Samenvatting Quantitative.docx
Document Details
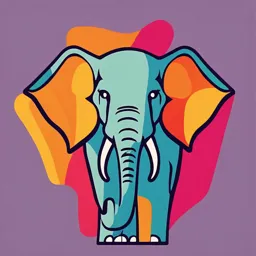
Uploaded by RealisticTaylor
Vrije Universiteit Amsterdam
Full Transcript
Samenvatting Quantitative What is quantitative research - - Quantitative research methods resolve around answering a particular research question by collecting numerical data that are analyzed by the use of mathematical methods (in particular statistics. Types of quantitative research -...
Samenvatting Quantitative What is quantitative research - - Quantitative research methods resolve around answering a particular research question by collecting numerical data that are analyzed by the use of mathematical methods (in particular statistics. Types of quantitative research - - - - - - Why do we need quantitative research - - - - When do we need quantitative research? Good research questions: - - - Example - Talhem et al 2014 - - - - What is a theory? - - Elements of a good theory - Whetten 1989 - - - - What is theory - H. Poincaré - What is a theory - Model  Summary - - The Data Generating Process (DGP) - - Example We hypothesized that founders gender positively/negatively affects funding because more men founder are asked promotion-focused questions (which allow them to boast). - - -  Alternative explanations We call that alternative explanations - - We will need to find clever ways to rule out these alternative explanations to identify (credible test) our proposed theoretical relation with the data at hand. This a large part of the scientific review process. Research design A good research design helps us achieve this by: 1. 2. 3. To do so, we want to optimize the validity and reliability of our study Construct validity A measure is valid to the degree that it represents what you are trying to measure - - - Internal vs external validity - - Threats of validity - - - - Omitted variable bias - - - Excurse: Gender pay gap:... is a complex and important phenomenon. It is also real! - Reverse causality Reverse causality occurs when the direction of the arrow in our theoretical model goes the other way. - Very difficult to empirically rule this out (beyond the scope of this course) Sometimes logical reasoning can help us Sample Section: It is almost never possible to obtain data from the full population of interest. - - You need to make sure that your sample is representative of you population of interest. - - (Sample) selection bias = the selection of data for analysis in such a way that proper randomization is not achieved, leading to an unrepresentative sample of the population intended to be analzed To avoid these issues, we need to draw an independent and identically distributed sample from the population = Random sample - - Measurement error - - - - - - Reliability Reliability is the degree to which a measure produces stable/repeatable and consistent results Inter-item reliability..... measures if items on a scale are internally consistent Cronbach's alpha - - - - Reliability vs validity Reliability measures precision whereas validity measures accuracy  Types of variables Statistics 101  Conditional means It's quite cumbersome to describe the relation between two variables by looking at the full distribution. In principle, we could examine how the different moments of the distribution change We will focus on the conditional mean: When you condition on a discrete (categorical, binary) variable, calculating the conditional mean is relatively straightforward. But what if we wanted to measure the relationship (continuous) extroversion on (continuous) happiness? Not so straightforward.... Enter correlation - - - - The more two variable covary, meaning the more they vary together in a consistent way, the stronger their linear relationship is, as indicated by the magnitude of their correlation coefficient. Regression versus correlation Linear regression coefficient and correlation coefficient are computationally very similar. In fact, identical when using standardized variables. The difference is conceptual in that the correlation quantifies the strength and direction of a linear relationship between two variables, whereas the regression estimates how one variable affects another and predicts values based on this relationship. LOESS - locally estimated scatterplot smoothing Line - fitting Instead of predicting the outcome (dependent) variable using local values of explanatory (independent) variable, we could assume that their relation is represented by a certain shape (i.e., a straight lane). For example: Y = 1.53 + 0.01X This is what call line-fitting, also known as regression  Extraversion = 1.53 + 0.01Height Great, now what does that actually mean? - - From sample to population - - The true population model  y = dependent variable x = independent or explanatory variable b1 = slope parameter = how much we would expect y to change given a one-unit change in x b0 = intercept parameter = what value we would expect y to have when x = 0 e = error = other factors than x that affect y (unobserved)  Assumptions of OLS to be "BLUE" 1. 2. 3. 4. 5. TLD'R - - - - Violations to the assumptions In practice, with real world data, BLUE assumptions are frequently violated: - - - Only assumption 1 (exogeneity) is truly problematic. We say IV is endogenous when our estimate on average gives us the wrong answer, we call that a bias in our estimate! When E (e\|x) ≠ 0 we say there is omitted variable bias Omitted variable bias Omitted variable bias occurs when you leave out (omit) an independent variable that is a determinant of the dependent variable and correlated with one or more of the included independent variables. In this case, leaving out this independent variable will lead to an over- or underestimation of the relation between your variables of interest In our analysis we need to control or adjust for these variables! Omitted variable bias and the DGP Omitted variables are part of the alternative explanations that we covered earlier. When our model (b0 + b1x + e) differs from the "real" data generating process such that it violates the exogeneity assumption, we might make incorrect claims about the direction and the strength of the relation of interest between X and Y If we want to make unbiased claims about the relation between our explanatory and outcome variable we need to reduce concerns of omitted variable bias. Controlling for other variables\ When we believe a variable z may cause omitted variable bias if we exclude it from our model, we need to "control" or ("adjust") for it by explicitly integrating it Now the exogeneity assumption becomes: E(e\|x, z = 0) Conditional conditional means ("controlling") What do we do when we "control" for a variable Z? 1. 2. 3. 4. 5. Simple linear regression  Multiple linear regression What if x is binary or categorical?  T-test What to do if you have variables with multiple categories? Simple: make a binary variable for all but one of the different categories. (Else, we fall in the dummy variable trap!) You can interpret the coefficient of each of these variables as the proportionate difference in the outcome relative to the reference category (= the category for which you did not make a dummy)  Logit Regression: DV binary, IV whatever, more than one IV/Control Linear regression vs logistic regression  Binary dependent variables: Logit Regression - - - Hypothesis testing -  - Regression inference  1 Get the slope 2 Get standard error of the slope The standard error of Bk is the standard deviation of the sampling distribution of bk (I omit the formula here; it doesn't add much) - - - 3 Calculate test statistic: t-value (again) As we already mentioned, the default test that is conducted by any statistical software is:  The p-value.... When to reject H0? What about p = 0.49 vs p = 0.51 Interpretation: If what we observe is too unlikely to happen under the null hypothesis, it means that this null hypothesis is likely to be false When p is (groter of gelijk aan) a we say that the result is significant at a certain significance level a - Decision: If the p-value falls below the cutoff a, we "reject the null hypothesis" at the significance level a." P-values notation conventions  Important from teacher: - - - Significant vs meaningful Statistical significance only provides information about whether a particular null value is unlikely. It doesn't say anything about whether the effect you have found matters. That is why I teach you to also look at the coefficients to understand the magnitudes of the relation between variables. Use common sense. Moderation - - - - Example: - -  - Moderation - - - - - - - - - Mediation - -  Example mediation: Micro damage Mediation a la Baron & Kenney (1986)  Moderator mediation