Research Statistics Finals Study Guide PDF
Document Details
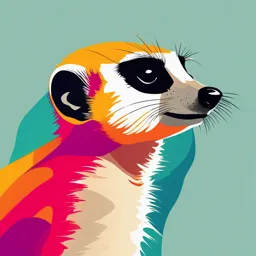
Uploaded by MeritoriousMajesty5217
Tags
Summary
This study guide covers research statistics, including probability, sample spaces, events, trials, permutations, combinations, factorials, expected value, percentiles, simulations, and more. It has sections on the fundamental principle of counting, Venn diagrams, independent and dependent events, mutually exclusive events and more.
Full Transcript
Research Statistics Finals Study Guide Crash Course Unit 1: Probability Probability The mathematics of chance behavior Sample space The set of all possible outcomes Outcomes The elements of a sample space Event Some subset of outcomes from the sample space Trial Any proce...
Research Statistics Finals Study Guide Crash Course Unit 1: Probability Probability The mathematics of chance behavior Sample space The set of all possible outcomes Outcomes The elements of a sample space Event Some subset of outcomes from the sample space Trial Any procedure that can be infinitely repeated and has a well-defined sample space Permutations An arrangement of items in a particular ORDER P(n, r) = n! / (n - r)! for 0 ≤ r ≤ n Combinations A selection in which ORDER DOES NOT MATTER C(n, r) = n! / r! (n - r)! for 0 ≤ r ≤ n Factorials n! = n * (n-1) * (n-2) * (n-3) *... * 3 * 2 * 1 0! = 1 Expected Value The long-term average value achieved by a numerical random process (outcome 1 * probability of outcome 1) + (outcome 2 * probability of outcome 2) +... Percentile Each of the 100 equal groups into which a population can be divided according to a distribution of values of a particular variable Simulation An artificial representation of a random process used to study the process’s long-term properties Steps in creating a simulation 1. Identify the real world activity that is to be repeated 2. Link the activity to one or more random numbers 3. Describe how you will use the random number assignment to complete a full trial 4. State the response variable 5. Run several trials 6. Collect and summarize the results of all of the trials 7. State your conclusion The Fundamental Principle of Counting Also known as the “Multiplication Principle” If one event has m possible outcomes and a second event as n possible outcomes, then there are m*n possible outcomes for the two events together. Venn diagrams S (rectangle) represent a sample space A and B (circles) represent specific events in the sample space S Not: involves complements and is designated AC or A’. The probability that an event will fail to occur. And: involves set intersection and is designated A∩B. The probability that both events will occur. Or: involves set union and is designated A∪B. The probability that either of the events will occur. Union “Or.” The probability of either one of the events occurring. Intersection “And.” The probability of both events occurring. Independent Events The occurrence of one event does not affect the occurrence of a second event Dependent Events The occurrence of one event affects the occurrence of a second event Mutually Exclusive Events Also known as “disjoint events” Two events that cannot happen at the same time. The probability of an event E P(E) = number of outcomes in the event E / number of outcomes in the sample space Multiplication Rule General Multiplication Rule: P(A∩B) = P(A) * P(B|A) Multiplication Rule for Independent Events: A and B are independent events if and only if P(A∩B) = P(A) * P(B). Addition Rule General Addition Rule: P(A∪B) = P(A) + P(B) - P(A∩B) Addition Rule for Mutually Exclusive Events: P(A∪B) = P(A) + P(B) Complement Rule P(A) + P(AC) = 1 Conditional Probability Rule The probability of an event, given that another has already occurred. For any two events A and B with P(A) ≠ 0, P(B|A) = P(A∩B) / P(A) Random phenomenon An event with an uncertain outcome Random variable The numerical outcome of a random phenomenon Probability histogram of a random variable X-axis: the possible values of x (the random variable) Y-axis: the probability of x Theoretical (exact) probability Ratio of the number of favorable outcomes to the total number of possible outcomes Empirical (Estimate of) probability Ratio of the number of favorable outcomes to the total number of trials IN AN EXPERIMENT Cumulative Relative Frequency Ratio of, CUMULATIVELY, how many times an event occurs to the maximum amount of times it could have occurred Tables Groups observational units based on two categorical variables. (ex. Education and Salary) Tree Diagrams The first set of branches describe the probability that A will happen. The second set of branches describe the probability that given A, B will happen. The probability of a certain event is 1 ( Sum of all outcomes in the sample space is 1). If two events with nonzero probabilities are MUTUALLY EXCLUSIVE EVENTS, then they cannot be INDEPENDENT because P(A|B) = 0 when A and B are mutually exclusive. Binomial Distributions A frequency distribution of the possible number of successful outcomes in a given number of trials Conditions for a binomial experiment: ○ TWO possible outcomes (typically referred to as “success” and “failure”) An observational study of past data Sampling frame An actual list of every member of the population to sample from Simple random sample (SRS) A way to avoid a biased sampling method. Gives every member of the population the same chance of being selected for the sample. Ensures that every possible sample has an equal chance of being in the sample ultimately selected. Treatment An explanatory variable group the researcher controls/ imposes Unbiased statistic Values of the statistic from different random samples are centered at the actual parameter value. Unit 4: Normal Distributions Normal distribution curves Shape: Symmetric, single-peaked, and bell-shaped The mean, median, and mode are equal The area under the curve is 1 The curve approaches, but never touches the x-axis as it extends farther and farther from the mean. Normal quantile plots Z score Z = (x - μ) / σ Also known as “standardized score” How many standard deviations above or below the mean a particular value falls. The Empirical Rule 68-95-99.7 Rule In a normal distribution, 68% of the values lie within 1 standard deviation of the mean, 95% of the values lie within 2 standard deviations of the mean, and 99.7% of the values lie within 3 standard deviations of the mean. normalcdf() Finds the area between the lower and upper bound, given the population mean and standard deviation ShadeNorm() normalcdf(), but graphs the area invNorm() Given an area (as well as the population mean and sd), it finds the z value. Central Limit Theorem for a sample mean For a SRS of size n (sampling distribution) taken from a large population in which the variable of interest has mean μ and standard deviation σ ○ Shape: the distribution will be approximately normal if the population distribution is normal OR the sample size is larger than 30. ○ Center: the mean will equal μ, regardless of whether or not the population distribution is normally distributed. ○ Spread: the standard deviation will equal σ / √(n). Normal distribution A theoretical model used to approximate distributions Population distribution The distribution of all members of a population Sample distribution The distribution of a single sample of a population Sampling distribution The distribution of the sample means of all or many possible samples of a population Standardization Calculating a z score Converts data to a common scale so you can compare observations from apparently disparate distributions Unbiased estimator An accurate statistic used to estimate a population parameter The sampling distribution of the sample mean is approximately normal if n is large, no matter what the distribution of the parent population. Unit 5: Significance Testing Sampling distribution of x̄: Unbiased estimator of μ (μx̄ = μ) The variability of the sampling distribution decreases as the sample size n increases, and by a factor of 1/√(n). ○ σx̄ = σ / √(n) CLT (shape of the sampling distribution of x̄) If the population distribution is normally distributed, than the sampling distribution of x̄ is normally distributed, regardless of the sample size. If the population distribution is not normally distributed, then the sampling distribution of x̄ will be approximately normal if n is sufficiently large (n ≥ 30). Sources of variation between the value of the population mean and the sample statistic calculated from collected data: 1. BIAS in the sampling procedure 2. CHANCE ERROR: the variation occurred strictly due to chance 3. A SIGNIFICANT EVENT: the difference between the population and sample means is so great that we can rule out chance error, and the observed result is said to cast doubt on the validity of the population mean. a. The basis for statistical significance Statistical significance A sample result is said to be statistically significant if it is unlikely to occur due to random sampling variability alone. (P < 0.05) Although you cannot use a sample statistic to determine a population parameter exactly, you can be reasonably CONFIDENT that the sample statistic falls within a certain distance of the population parameter. The standard deviation of the sampling distribution of the sample mean (x̄) σx̄ = σ / √(n) Standard error Provides a close estimate of σx̄ because we almost never know σ σx̄ = s / √(n) Confidence intervals Point estimate ± margin of error Margin of error = critical value * standard error ○ The margin of error is the half width of the confidence interval Confidence interval for a sample mean x̄ ± z * ( σ / √(n) ) When σ is unknown: x̄ ± t * ( s / √(n) ) Interpretation of a confidence INTERVAL I am __% confident that the true population mean falls within ___ and ___. Interpretation of a confidence LEVEL If I created confidence intervals for all possible samples of sample size n from the population, then the population mean will fall in __% of them. Statistical confidence (ex. 95%) and statistical significance (ex. 5%) are opposites. (100 - statistical confidence = statistical significance) Significance testing 1. Parameter = description of parameter (means are used as an example, because we didn’t use other parameters) a. One sample test i. μ = description of parameter b. Two sample test i. μ1 = description of parameter of first population ii. μ2 = description of parameter of second population c. Paired test i. μd = description of parameter of differences of pairs ( population 1 - population 2 or population 2 - population 1) 2. Null + alternative hypothesis a. One sample test i. H0: μ = x ii. HA: μ (, ≠) x b. Two sample test i. H0: μ1 = μ2 ii. HA: μ1 (, ≠) μ2 c. Paired test i. H0: μd = 0 ii. HA: μd (, ≠) 0 3. Technical conditions a. SRS? i. Is it a simple random sample? (eliminates reason to believe bias caused error in significance test) ii. If not, is it representative of the population? b. Independent? i. Is there sampling with replacement? (the observational units are independent of each other) ii. Is the sample size less than 10% of the population size? (the sample is small enough so that the sampling without replacement has a minimal effect on the end result) c. Normal? i. Is the population distribution normal? (if it is, then the sampling distribution is normal, regardless of the sample size) ii. Is the sample size greater than 30? (30 is a large enough number so that if the population distribution were not normal, the sampling distribution will be approximately normal) iii. Look at the data. Does the normal probability plot show an approximately straight line? 4. Test statistic a. One sample i. Z-test: z = ( x̄ - μ ) / ( σ / √(n) ) ii. T-test: t = ( x̄ - μ ) / ( s / √(n) ) b. Two sample i. Z-test: ((x̄1 - x̄2) - (μ1 - μ2)) / √(( σ12 / n1 ) + ( σ22 / n2 )) ii. T-test: ((x̄1 - x̄2) - (μ1 - μ2)) / √(( s1 2 / n1 ) + ( s22 / n2 )) c. Paired i. T-test: t = ( x̄d ) / ( sd / √(n) ) 5. P-value a. Use normalcdf() or tcdf() b. Or use z- or t- table 6. Conclusion a. I (fail to) reject the null hypothesis at the __% significance level. There is strong/weak evidence supporting the null hypothesis that... IF YOU MADE IT ALL THE WAY DOWN HERE I APPLAUD YOUR DEDICATION TO A GOOD GRADE IN RESEARCH STATS. GOOD LUCK ON THE FINAL.