Statistics Notes PDF
Document Details
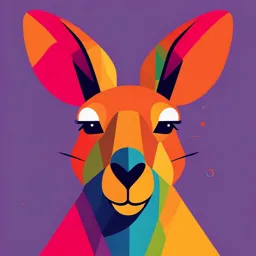
Uploaded by VirtuousBeryllium4719
New York University
Tags
Summary
This document contains notes on statistical concepts, including aggregation, information measurement, and data analysis techniques. These notes cover a range of topics, from basic statistical principles to more advanced concepts. The content explores methods for summarizing and analyzing data as well as discussing the relationship between data and accuracy.
Full Transcript
Session 1 & 2 readings notes: Intro: 7 pillars of stats: 1. Aggregation – basically just finding the mean or average from observations 2. Information (information measurement) – information in data can be measured, accuracy is related to the amount of data 3. Likelihood – the...
Session 1 & 2 readings notes: Intro: 7 pillars of stats: 1. Aggregation – basically just finding the mean or average from observations 2. Information (information measurement) – information in data can be measured, accuracy is related to the amount of data 3. Likelihood – the calibration of inferences with the use of probability 4. Intercomparison – statistical comparisons do not need to be made w respect to an exterior standard but can often be made in terms interior to the data themselves 5. Regression – basically bivariate normal distribution, 6. Design – design of experiments, super important imo 7. Residual – kind of “everything else,” but not really ?? To oversimplify: 1. The value of targeted reduction or compression of data 2. The diminishing value of an increased amount of data 3. How to put a probability measuring stick to what we do 4. How to use internal variation in the data to help in that 5. How asking questions from different perspectives can lead to revealingly different answers 6. The essential role of the planning of observations 7. How all these ideas can be used in exploring and comparing competing explanations in science Ch. 1: Aggregation - Aggregation was referred to as the “combination of observations” - The taking of a mean of any sort is a sort of radical idea, being that statisticians must discard some data information to do so - Contrasting observations to arrive at a result, not a combination of essentially equivalent observations – the average was a “before minus after” contrast - midrange=the mean of the largest and the smallest – while this is an arithmetic mean of two observations, there is scarcely any other way of effecting a compromise between two values - Common problem: how to summarize a set of similar, but not identical, measurements. The way the problem was dealt with in each situation reflects the intellectual difficulty involved in combination, one that persists today. - Many ways suggested for reconciling inconsistent measures taken under different conditions through some form of aggregation–the most successful was the method of least squares, which is formally a weighted average of observations and had the advantage over methods of being easily extendable to more complicated situations in order to determine more than two unknowns - Conclusion: aggregation has taken many forms, from simple addition to modern algorithms that are opaque to casual inspection. However, the principle of using summaries in place of full enumeration of individual observations, of trying to gain info by selectively discarding information, has remained the same. - Here’s what Sisi thinks: stats (specifically aggregation/finding the mean of observations) is applicable across many disciplines, and can also be used to help formulate policies ? Ch. 2 notes: Information - The second pillar, information measurement, is logically related to the first: if we gain info by combining observations, how is the gain related to the number of observations? How can we measure the value and acquisition of info? - (this might sound crazy but it lowkey does make sense:) Laplace came to the same conclusion in regard to the total or mean of observations (such as the weights of a sample of coins), where the individual observations (or errors in observations) followed pretty much any distribution - The effect of correlation on the amount of information in the data—basically the main theme of this chapter - In any event, the idea that information in data could be measured, that accuracy was related to the amount of data in a way that could be made precise in some situations, was clearly established by 1900. - Correct argument that sounds silly but actually works: it would be expected that many people continued to believe that the second 20 observations were at least as valuable as the first 20. But an interesting claim from sources that was even more extreme goes in the opposite direction—in some situations, it is better, when you have two observations, to discard one than to average the two! - Conclusion: the statistical assessment of the accumulation of information can be quite a complex task, but with care and attention to correlations and scientific objectives, the measurement of information in data—the comparative information in different data sets and the rate of increase in information with an increase in data—has become a pillar of stats - In Sisi's words: measurement of information → using stats to analyze correlation but with a special focus and consideration of the size of study groups and increase in numbers with increase in data….. And also remember the objective of the study to not get lost in the sauce Journal 1: Aggregation **DUE 2/10** Impact (tulsa regional demographics)t: demographics – snapshot of thing and size of city; bassline general stats for the city 9b Neighborhood explorer: more about trend differences – over time Equality indicator: Highlight group that is most disadvantaged, compare w group that is most advantaged; systemic disparities → does a good job showing differences between the groups (really incorporates the range when calculating, differentiating themselves from the other 2 orgs)