Results (Part 7) - Results [Corrected 2].docx
Document Details
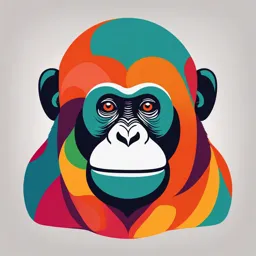
Uploaded by DiligentPolynomial
Open University
Tags
Full Transcript
**RESULTS** **[7 Extensive Results and Insights]** *Summary Overview of the Outcomes:* This chapter explores the responsiveness of gastric cancer cells to All-trans Retinoic Acid (ATRA), highlighting its capacity to limit cell proliferation. The first goal is to categorize 37 gastric-cancer cell-...
**RESULTS** **[7 Extensive Results and Insights]** *Summary Overview of the Outcomes:* This chapter explores the responsiveness of gastric cancer cells to All-trans Retinoic Acid (ATRA), highlighting its capacity to limit cell proliferation. The first goal is to categorize 37 gastric-cancer cell-lines into separate subgroups using the gene expression patterns obtained from the Cancer Cell Line Encyclopedia\'s RNA-sequencing data. In addition, the study involves evaluating ATRA-sensitivity in a significant number of these cell lines. Furthermore, we investigate the role of RARα in the anti-proliferative effects of ATRA and the genetic networks linked to ATRA sensitivity. Moreover, our research includes the assessment of ATRA\'s ability to limit growth in certain cell lines and tissue samples obtained from patients. **[7.1 Sensitivity Profiling of Gastric Cancer Cell Lines to ATRA:]** The project\'s objective is to create a model that characterizes the state and identity of gastric-cancer clinical samples as closely as possible. The heterogeneity of gastric-cancer^1,2^ is recapitulated by the large panel of gastric carcinoma cell-lines available in our laboratory. This offers notable advantages, such as the possibility of studying the biological characteristics of the tumour in a controlled way, testing the effectiveness of new drugs and targeted therapies, and understanding the molecular mechanism underlying cancer growth. In particular, the *CCLE (Cancer-Cell-Lines-Encyclopedia)* database contains RNA-Sequencing (*RNA-Seq*) data of the constitutive gene expression of all tumour cell lines. In particular, the gene-expression profiles of our 37 gastric cell-lines (**Fig. 10**), classify them into the G-INT and G-DIFF subgroups^3^. In order to determine the responsiveness of gastric-cancer cells to the growth-inhibitory effects of ATRA, a total of 27 cell-lines were selected and exposed to ascending doses of ATRA (ranging from 0.001 to 10 μM). The anti-proliferative effects of Retinoic Acid were assessed on day-3, day-6, and day-9 and the results obtained are exemplified in **Fig. 11** for the RERF-GC and IM95 cell-lines. Using an experimental version of the quantitative ATRA-score index^4^, cell-lines were classified according to their sensitivity to ATRA (**Fig. 12**). The revised *ATRA-score* value is derived from the AUCs calculated for each cell-line treated with Retinoic-Acid. It ranges from a minimum value of \"0.00\" to a maximum value of \"1.00\". *ATRA-scores* were computed based on the growth-curves observed on day-6, for each cell-line, as illustrated in **Fig. 13**. In order to categorize the 27 gastric-cancer cell-lines into two equally distributed groups (bimodal distribution) based on their sensitivity to the retinoid, we set threshold value of 0.55 for the *ATRA-score*. This value was chosen to fall above the median number of cell-lines. Indeed, the threshold value divides the cell-lines into two distinct groups, with 14 cell-lines defined by high ATRA-sensitivity (*ATRA-score* \> 0.55) and 13 cell-lines characterized by low ATRA-sensitivity (*ATRA-score* \< 0.55) (**Fig. 12**). The two most sensitive cell lines, GCIY and HGC-27, and the two least sensitive cell lines, HuG1-N and OCUM-1, exhibit a *G-DIFF* and a *G-INT* phenotype, respectively. The observed trend in our cell-line panel indicates that there is a significant increase in the occurrence of the *G-DIFF* and *G-INT* phenotypes in the high ATRA-sensitivity group (9 out of 14) and the low ATRA-sensitivity group (8 out of 13), respectively. **[7.2 Identification of the RAR/RXR Isoform Mediating ATRA\'s Anti-proliferative Effects:]** There are six known retinoid receptors, namely RARα, RARβ, RARγ, RXRα, RXRβ, and RXRγ. The active forms of these transcription factors are composed of RAR/RXR heterodimers, where RAR serves as the component that binds to the ligand^5,6^. ATRA is a compound that activates all three types of retinoic acid receptors (RARs), namely RARα, RARβ, and RARγ, with the same level of affinity and effectiveness. *7.2.1 Expression of the RAR/RXR mRNAs in gastric cancer cell lines and stomach tumors* In order to determine the specific RAR isoform(s) responsible for the retinoid\'s anti-proliferative effect, we first examined the baseline expression of *RARα, RARβ, RARγ, RXRα, RXRβ*, and RXRγ mRNAs in the CCLE panel of gastric cancer cell lines (**Fig. 14**). The CCLE RNA-seq results show that all the cell lines exhibit comparable amounts of *RARα, RARγ, RXRα*, and *RXRβ* mRNAs. On the other hand, the levels of *RARβ* mRNA exhibit variability, with most cell lines expressing just minimal quantities of the transcript. There is no apparent link between the levels of *RARβ* mRNA and the sensitivity to ATRA or the G-DIFF/G-INT phenotype. Furthermore, there is no cell-line that exhibits measurable levels of the *RXRγ* mRNA.  Interestingly, the expression patterns of the *RAR/RXR* in the cell-lines closely resemble those seen in primary gastric tumors, as shown by the *RNA-seq* data available in the TCGA-database (**Fig. 15**). *7.2.2 Effects of RAR agonists and antagonists on the growth of selected gastric cancer cell lines* Thereafter, a pharmacological strategy was implemented, including the administration of *RARα/RARβ/RARγ* agonists. The development of the *HGC-27 (ATRA-score = 1.00)*, *IM95* (*ATRA-score* = 0.88), *LMSU* (*ATRA-score* = 0.80), and *Hs747T* (*ATRA-score* = 0.59) cell lines, which are part of the high ATRA-sensitivity group, was assessed using this method. In order to achieve this objective, the 4 cell lines were challenged with increasing concentrations of ATRA, AM580 (*RARα* agonist)^7^, CD2314 (*RARβ* agonist)^8^ and BMS961 (a *RARγ* agonist) for six days, as illustrated in **Fig. 16**.  AM580 is the only *RAR* agonist with a concentration-dependent growth-inhibitory effect in all cell-lines, which is similar to the effect observed with ATRA. CD2314 and BMS961 have a minor impact on the proliferation of *HGC-27*, *LMSU*, *IM95*, and *Hs747T* cells. The anti-proliferative effects of the two compounds are noticeable only following challenge with maximal concentrations of CD2314 and BMS961. The findings acquired with the *RAR* agonists provide evidence that RARα is the main retinoid receptor responsible for the anti-proliferative effect of ATRA in gastric-cancer cell-lines. Lastly, we conducted experiments aimed at confirming the implied function of *RARα* in the growth inhibitory effects exerted by ATRA. This was done by using specific antagonists of *RARα* (ER50891), *RARβ/γ* (CD2665), and *RARγ* (MM11253) (**Fig. 17**). In order to perform these studies, *HGC-27* cells were challenged with ATRA, ER50891, CD2665, and MM11253, as well as the combinations of ATRA+ER50891, ATRA+CD2665, and ATRA+MM11253 for 9 days. In our experimental conditions, ATRA was shown to exert the expected growth-inhibitory effect, whereas ER50891, CD2665, and MM11253 did not show any anti-proliferative activity on *HGC-27* cells. Interestingly, the growth inhibitory action of ATRA was suppressed only by the RARα antagonist, ER50891. Indeed, the ATRA-dependent growth inhibition was observed also after exposure of *HGC-27* cells to ATRA+CD2665 and ATRA+MM11253. Overall, the findings collected from *HGC-27* cells using a pharmacological approach involving RAR agonists and antagonists support the notion that *RARα* activation is both necessary and sufficient to mediate the anti-proliferative effects of ATRA in responsive gastric-cancer cells. **[7.3 Identification of a Gene Transcriptomic Network Associated with ATRA Sensitivity:]** RARα is not an accurate biomarker for identifying gastric-cancer patients who might benefit from ATRA-based therapy. Therefore, it is crucial to develop a gene-expression model that can be used in clinical settings to identify individuals who would respond positively. To develop a prediction algorithm for selecting ATRA-sensitive gastric-cancer patients, the gene-expression patterns of our panel of 27 cell lines were determined using the *CCLE/RNA-seq* data. The computational method used identifies a small set of transcripts whose baseline expression levels show a positive or negative correlation with the experimentally established *ATRA-score* values in a quantitative manner (**Fig. 18**).  In fact, there are 26 protein-coding mRNAs showing a positive correlation with ATRA-sensitivity (*high-Basal-Expression-Levels/high-ATRA-scores*). Conversely, there are 16 protein-coding mRNAs showing a negative correlation with ATRA-sensitivity (*low-Basal-Expression-Levels/high-ATRA-scores*). An investigation conducted using the *STRING* database^9^ revealed that the products of these 42 genes coalesce to create four separate networks of interacting proteins (**Fig. 19**).  **Fig. 19** shows two distinct representations of the network under consideration. On the left, the types of interactions between genes and biological processes involved in the sub-networks are highlighted. On the right, it is possible to highlight the centrality of each gene, as the size of the node (gene) is directly proportional to the number of interconnections. In both representations, positive and negative correlations are shown by the red and blue colors, respectively. The most extensive network consists of 28 components, which include proteins associated with tissue development (*PITX2, PAX9, ALX3, MEOX1, SIX6, TLE3*) and the WNT pathway (*WNT2, TLE3, EGF, ERBB3*). The second network consists of six components that include proteins involved in myogenesis and collagen homeostasis (*TPM1/FLNC/NRAP/COL6A1/LOXL1/TLL1*). The third and fourth networks include factors that regulate pre-mRNA processing (*UPF3A/SRRM1/FIP1L1/NUP98*) as well as metabolic and mitochondrial homeostasis (*CPS1/BHMT/SIRT3/ING1*). **[7.4 Effects of ATRA on gene-expression in gastric-cancer cell-lines:]** In order to get a deeper understanding of the gene networks that are involved in the effects of ATRA in gastric-cancer, RNA-seq investigations were conducted on 13 of our cell lines, which are part of the total panel of gastric carcinoma cell lines of the CCLE used for the computation of the ATRA-score described in the previous paragraph (**Table 3**). *7.4.1 Transcriptomic Characterization and Clustering of Gastric-Cancer Cell-Lines:* Before performing the differential analysis between the samples of the cell-lines treated with the retinoid and the control (vehicle), an initial profiling was conducted to evaluate samples' clustering (**Fig. 20**).  As illustrated in **Fig. 20**, two distinct transcriptomic profiles emerge, which align with the classification by Tan et al.^3^. The two identified groups are "*Genomic Intestinal*" (*G-INT*), which includes 8 of our cell lines (*GSU, KATOIII, OCUM1, HuG1N, IM95, MNK45, AGS, NCI-N87*), and "*Genomic Diffuse*" (*G-DIFF*), which includes 7 of our cell lines (*GSS, GCIY, RERF-GC, MKN7, HGC-27, LMSU, Hs746T*). An additional step was to validate the reliability and consistency of our laboratory\'s panel of cell lines to ensure transcriptomic coherence with the cell lines deposited in the CCLE database. This validation was performed using only the control-treated (DMSO) cell lines from our panel, and a correlation analysis was conducted with the baseline expression of the cell lines from the CCLE (**Fig. 21**). *7.4.2 Differential Expression Analysis and Identification of the Effect of Retinoic Acid Treatment:* The ATRA-sensitivity of the seven cell-lines is classified as high (*ATRA-score* \> 0.55), while the sensitivity of the six cell-lines is characterized as low (*ATRA-score* \< 0.55) (**Fig. 20**).  In order to conduct these *RNA-seq* experiments, each cell-line was treated with either a vehicle or ATRA (1.0 µM) for a duration of 48 hours. This time period occurred before any indication of growth suppression. The link between the number of transcripts up- and down-regulated by ATRA in each cell-line (**Fig. 22**) and the *ATRA-scores* is directly demonstrated by the linear-regression *r-values* reported in **Fig. 23**. Indeed, there is a direct correlation between the *ATRA-score* measured in each cell-line and the amounts of mRNAs that are *up-* or *down-regulated* by ATRA. In order to get more information on the pathways that are altered by ATRA in the cell-lines that respond to it, a Gene Set Enrichment Analysis (*GSEA*) was conducted on the *RNA-Seq* data. The HALLMARK dataset was used for this analysis (**Fig. 24**).  The anti-proliferative effects of ATRA are based on a reduction in the expression of genes that govern the *G2M-checkpoint* and genes that are controlled by the *E2F transcription-factor*. ATRA seems to selectively decrease the expression of *c-myc* target-genes in cell-lines that are susceptible to the retinoid, resulting in the inhibition of cell proliferation (**Fig. 24**lower-right). The interactome with the highest number of elements, consisting of 28 factors, includes components that play a role in lipid metabolism. These factors include *MOGAT3, DGAT2, PCK1, NR1H3, SCARB1, PCSK9, PON2, CES2, CYP2C19, CYP4F12, CYP2C18, CYP2W1*, and *SLC16A1*. Curiously, a smaller network contains additional proteins that regulate lipid metabolism, such as *SPTLC3, DEGS2, UGT8*, and *GAL3ST1*. This finding provides evidence that the inhibitory effects of ATRA on the growth of the G-INT neoplastic cells are linked to the regulation of lipid homeostasis. The interactome of down-regulated gene-products (*CDH17/ITGA6/COL17A1/DSG3/TRIM29/SERPINB5/PLOD3/LAD1/P3H2/TMEM154/S100A14/S100A16/S100A4*), which is the second-largest, consists of proteins that play a role in cell adhesion and motility. The decrease in expression of these specific gene-products may be associated with a possible enhancement of the epithelial-polarization process, which was previously described in relation to the up-regulated *CYTH3* gene. In order to assess potential correlations with ATRA-sensitivity, similar investigations were performed on 5 *G-INT* cell-lines (*MKN45, AGS, NCI-N87, HuG1-N, and OCUM-1*) that are known to be endowed with low ATRA-sensitivity (*ATRA-score \ 0.55*). These cell-lines show a high sensitivity to ATRA. Additionally, research was conducted on *GSS* cells, the sole *G-DIFF* cell-line that exhibited poor sensitivity to ATRA (*ATRA-score = 0.50*). The expression of 6,063 genes in *HGC-27* cells and 5,557 genes in RERF-GC-1B cells is affected by ATRA. In comparison, a smaller number of genes, namely 1,886 genes in *LMSU* cells and 914 genes in GCIY cells, are up- or down-regulated. This information is shown in **Fig. 31**. Within these cell lines that are responsive to retinoids, ATRA induces an increase in the expression of 33 genes and a decrease in the expression of 10 genes, as shown in **Fig. 32**.  The STRING analysis conducted on the 33 up-regulated genes reveals that the relevant proteins form three distinct networks. The most extensive network, consisting of 13 components, is centered around IRF1. This network includes factors that regulate inflammation (*BIRC3/TLR3/USF1/CCL2*) and antigen presentation (*PSME1/PSMB10/TAPBPL2/ERAP1/ERAP2/CTSS*). The second-largest network comprises seven proteins (*CYP26B1/DHRS3/NRIP1/RARB/GPRC5A/RARRES3/APOL3*) that regulate retinoid metabolism and epithelial development. The 10 down-regulated genes do not encode any interacting protein. In retinoid-resistant *GSS* cells, ATRA induces a notable increase and decrease in the expression of 137 and 89 genes, respectively (**Fig. 33**). Interestingly, 4 specific genes (*CYP26B1, RARB, DHRS3, TINAGL1*) are significantly up-regulated in the retinoid-sensitive *G-DIFF* cell-lines (*GCIY, HGC-27, RERF-GC-1B*, and *LMSU*) (**Fig. 33**/black-squares). *7.5.3 ATRA-Modulated Gene Networks in Sensitive G-INT and G-DIFF Gastric-Cancer Cell-Lines:* In the final part of the studies, we focused our interest on the genes up- or down-regulated by ATRA in the *G-INT* and *G-DIFF* cell-lines, which are characterized by high ATRA-sensitivity (**Fig. 34**).  ATRA enhances the expression of 6 common genes, namely *IRF1, DHRS3, CTSS, PSMB10, CYP26B1*, and *TINAGL1*, in both *G-DIFF* and *G-INT* cell lines. However, ATRA specifically decreases the expression of *AHNAK2* in all *G-INT/G-DIFF* cell-lines. As previously noted, the *CTSS* and *PSMB10* gene-products have a direct interaction with *IRF1* and are included in the interactome centered on *IRF1* itself (**Fig. 31/Fig. 32**). In addition, the *CYP26B1* gene-product forms a connection with *DHRS3*. Both genes are network components that play a role in retinoid metabolism and epithelial development (as shown in **Fig. 31** and **Fig. 32**). *7.5.4 Epigenetic factors modulated by ATRA in G-INT e G-DIFF gastric-cancer cell-lines:* Epigenetic factors modulate gene expression modulating DNA methylation and causing histone modifications. They can be influenced by lifestyle and environmental factors. ATRA can modulate these epigenetic factors, affecting gene expression in cells. For example, ATRA has been shown to increase the expression of PD-L1 in gastric-cancer cells through epigenetic changes that influence protein stability and synthesis^12^. To identify the epigenetic factors which are regulated by ATRA in gastric carcinoma cells, a list of 720 epigenetic factors contained in the study of Medvedeva et al^13^ was used and implemented in our analysis. Among the Epigenetic factors modulated by ATRA in retinoid-sensitive G-INT gastric-cancer cell-lines it is possible to find the genes shown in **Fig. 35**. There is no clear clustering of these epigenetic factors, as only 3 of them are interconnected (**Fig. 35/rigth**). It is likely that these epigenetic factors play a positive/negative role in the growth inhibitory action exerted by ATRA in sensitive cells. Thus, induction or stimulation of these epigenetic factors may represent a novel strategy to: - Treat **G-INT** gastric-cancers - Enhance the anti-tumor action of ATRA and other therapeutic agents in **G-INT** gastric-cancers. Moreover, it is possible to observe that no epigenetic factor is up- or down-regulated by ATRA in retinoid-resistant *G-INT* and in retinoid-sensitive *G-DIFF* gastric-cancer cell-lines. Interestingly, only 3 genes were identified as epigenetic factor modulated by ATRA in *GSS*, the sole retinoid resistant *G-DIFF* gastric-cancer cell-line: *PADI1, RARA* (up-regulated) and *BRD7* (down-regulated). **[7.6 Definition of the Effects of ATRA on Antigen-Presentation Processes in GC Cell Lines:]** The activation of ATRA leads to an increase in the expression of genes implicated in IFN-dependent immune responses. This increase in expression is accompanied by an up-regulation of the HALLMARK gene-set associated with "*Allograft-Rejection*", as seen in **Fig. 24** and **Fig. 25**. The HALLMARK network comprises genes that play a role in presenting antigens and suppressing tumour development and metastasis via T-cell activity. This indicates that ATRA enhances the ability of gastric-cancer cells to trigger an immune response. Therefore, we considered the *REACTOME* \"*Folding-Assembly-and-Peptide-Loading-of-Class-I-MHC*\" gene-set, including 24 genes that have crucial functions in antigen-presentation. The gene-network is up-regulated by ATRA in most of our gastric-cancer cell-lines, independent of retinoid-sensitivity and *G-DIFF/G-INT* phenotype (**Fig. 36**).  ATRA enhances the activation of the *HLA-A/B/C* and *B2M genes*, which encode proteins central to the *Major Histocompatibility Complex (MHC)*. In order to determine if the changes in gene expression caused by ATRA result in an increase of the *HLA-A/B/C* surface antigens, *FACS (Fluorescence-Activated-Cell-Sorter)* analyses were conducted on various cell lines, including gastric-cancer *HGC-27, LMSU, KATO-III, AGS* and breast-cancer *SKBR3* (used as a positive control) cells. An anti-HLA-A/B/C antibody was used for this analysis (**Fig. 37A/Fig. 37B**). Consistent with the overall increase in the *REACTOME* gene-network, *HGC-27, LMSU*, and *KATO-III* cells exhibit a rise in the ATRA-dependent *HLA-A/B/C* surface-expression. Notably, ATRA does not modify the expression of *HLA-A/B/C* on the surface of *AGS* cells. However, in this particular cell-line, there is a minor decrease in the *REACTOME* gene-network. **[7.7 In-Vivo Validation through G-DIFF and G-INT Gastric Cancer Xenograft Models:]** In order to investigate the therapeutic potential of ATRA in gastric-cancer, in vivo experiments were conducted using xenografts of two cell lines: *LMSU* (*G-DIFF/ATRA-score=0.80*) and *NCI-N87* (*G-INT/ATRA-score=0.37*). Mice were administered either vehicle (DMSO) or ATRA intra-peritoneally for a period of 2 to 3 weeks. The growth of the tumour was measured at various time intervals (**Fig. 38**).  As for *LMSU*, there is already a noticeable decrease in tumour size after 5 days of therapy, which is dependent on ATRA. The maximum reduction is seen on day 15 (**Fig. 38**, left). The maximum impact of ATRA on *NCI-N87* xenografts is less significant and occurs afterwards, particularly on day-19 (**Fig. 38**, right). Under these circumstances, ATRA has no harmful effect on the body-weight of mice that have been transplanted with *LMSU* and *NCI-N87*, indicating a lack of systemic toxicity (**Fig. 39**).  In order to validate the results obtained in gastric-cancer cell-lines, an RNA-Seq analysis of the *LMSU* and *NCI-N87* xenografts was performed. In particular, a differential analysis was conducted comparing ATRA-treated and DMSO-treated cells, with the goal of performing a *Gene Set Enrichment Analysis* (*GSEA*), the results of which are shown in **Fig. 40**. The pathway-network highlights an up-regulation of 1 macro-clusters (*Signalling and Immune Response*) and the down-regulation of 1 macro-cluster (*Interactions and Signaling of the Extracellular Matrix \[EMC\]*). **[7.8 Validation of the Results using Tissue Cultures of Primary Tissue Specimens:]** *7.8.1 Gastric Cancer Patients Analysis from Tissue Cultures of Primary Tissue:* In order to confirm the findings of the cell-line experiments, the inhibitory effects of ATRA on the growth of gastric-cancer tissue-slices cultures^14,15^ collected from 13 patients were assessed (**Table 4**/patients-characteristics). Out of the total number of tumors, five are classified as *G-INT* and six are classified as *G-DIFF* based on the gene-expression patterns established by *RNA-seq* studies conducted on 13 cases (**Fig. 41**).  In order to classify the samples of these patients based on their ATRA-sensitivity, a *Gene Set Variation Analysis* (*GSVA*) was carried out using the signature predicting the response to ATRA identified in paragraph-3 (**Fig. 42**). Three thresholds were used to distinguish between low, medium, and high responses to ATRA. The table shown below combines the clustering results based on both the G-INT and G-DIFF profiles. The tissue slices were challenged with vehicle (DMSO) or ATRA for a duration of 48 hours. The suppressive impact of the retinoid on growth was evaluated by quantitatively assessing the *Ki-67* proliferation marker using immunohistochemical techniques. As an example, the results obtained in patient-1 (*G-INT*) and patient-2 (*G-DIFF*), as illustrated in **Fig. 43**.  The findings indicate that ATRA reduces *Ki-67* levels in 9 instances (*G-DIFF* = 5 cases; *G-INT* = 3 cases; *Not Defined* = 1 case) (**Fig. 44**). In order to validate the results obtained in the gastric-cancer cell-lines and in the gastric-cancer Xenograft models, the RNA-Seq analysis results, in particular the differential expression results (ATRA vs DMSO samples) were used to perform a *Gene Set Enrichment Analysis* (*GSEA*), the results of which are shown in **Fig. 45**.  The pathway-network highlights an up-regulation of 1 macro-clusters (*Interferon Response & Immune Regulation*) and the down-regulation of 1 macro-cluster (*Extracellular Matrix \[EMC\] and Cell Interaction*). *7.8.2 Gastric Cancer Patients Analysis from TCGA:* Firstly, a *Nearest Template Prediction Classification* (*NTP*) analysis was applied in order to quantitatively assess the distribution of the samples in the *G-INT* and *G-DIFF* groups. Notably, the *G-INT* profile is predominant in the 375 gastric carcinoma samples from TCGA (**Fig. 46**). In order to generate *Similarity-score* values from the RNA-seq of the 375 gastric-cancer cases available in the *TCGA-dataset*, the combined expression levels of the 42 transcripts identified in paragraph 3, which predict ATRA sensitivity, were used. These *Similarity-scores* were then applied to predict the corresponding *ATRA-score* values (**Fig. 47**).  The *ATRA-score* value is predicted to be above 0.55 in 165 instances, indicating a high level of ATRA-sensitivity. On the other hand, the remaining 210 cases show a predicted *ATRA-score* below 0.55, indicating a low level of ATRA-sensitivity. It is thus expected that 44% of stomach cancers are characterized by high ATRA-sensitivity. This is in accordance with the fraction of gastric-cancer cell lines that are experimentally sensitive to ATRA, which is 52% (**Fig. 12**). Patients characterized by an expected low ATRA-sensitivity (67%; **Fig. 47**) are more likely to exhibit a *G-INT* phenotype, as anticipated by the findings obtained from cell lines. **[BIBLIOGRAPHY:]** 1\. Berlth, F. Pathohistological classification systems in gastric cancer: Diagnostic relevance and prognostic value. *World J. Gastroenterol.* **20**, 5679 (2014).2. Wang, Q., Liu, G. & Hu, C. Molecular Classification of Gastric Adenocarcinoma. *Gastroenterol. Res.* **12**, 275--282 (2019).3. Tan, I. B. *et al.* Intrinsic Subtypes of Gastric Cancer, Based on Gene Expression Pattern, Predict Survival and Respond Differently to Chemotherapy. *Gastroenterology* **141**, 476-485.e11 (2011).4. Bolis, M. *et al.* Network-guided modeling allows tumor-type independent prediction of sensitivity to all-trans-retinoic acid. *Ann. Oncol.* **28**, 611--621 (2017).5. Garattini, E. *et al.* Retinoids and breast cancer: from basic studies to the clinic and back again. *Cancer Treat. Rev.* **40**, 739--749 (2014).6. Petkovich, M. & Chambon, P. Retinoic acid receptors at 35 years. *J. Mol. Endocrinol.* **69**, T13--T24 (2022).7. Delescluse, C. *et al.* Selective high affinity retinoic acid receptor alpha or beta-gamma ligands. *Mol. Pharmacol.* **40**, 556--562 (1991).8. Sun, S. Y. *et al.* Identification of receptor-selective retinoids that are potent inhibitors of the growth of human head and neck squamous cell carcinoma cells. *Clin. Cancer Res. Off. J. Am. Assoc. Cancer Res.* **6**, 1563--1573 (2000).9. Szklarczyk, D. *et al.* The STRING database in 2021: customizable protein--protein networks, and functional characterization of user-uploaded gene/measurement sets. *Nucleic Acids Res.* **49**, D605--D612 (2020).10. Zhou, Y. Q. *et al.* Tumor suppressor function of *BCSC-1* in nasopharyngeal carcinoma. *Cancer Sci.* **100**, 1817--1822 (2009).11. Kang, W. *et al.* Induction of DMBT1 expression by reduced ERK activity during a gastric mucosa differentiation-like process and its association with human gastric cancer. *Carcinogenesis* **26**, 1129--1137 (2005).12. Ma, Z.-L. *et al.* ATRA promotes PD-L1 expression to control gastric cancer immune surveillance. *Eur. J. Pharmacol.* **920**, 174822 (2022).13. Medvedeva, Y. A. *et al.* EpiFactors: a comprehensive database of human epigenetic factors and complexes. *Database J. Biol. Databases Curation* **2015**, bav067 (2015).14. van der Kuip, H. *et al.* Short term culture of breast cancer tissues to study the activity of the anticancer drug taxol in an intact tumor environment. *BMC Cancer* **6**, 86 (2006).15. Centritto, F. *et al.* Cellular and molecular determinants of all‐ *trans* retinoic acid sensitivity in breast cancer: *Luminal* phenotype and [RAR]{.smallcaps} α expression. *EMBO Mol. Med.* **7**, 950--972 (2015).