Quiz 1 Preparation.pdf
Document Details
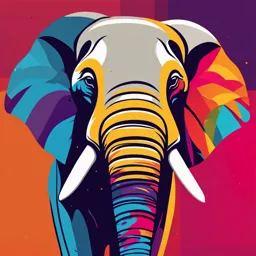
Uploaded by CostSavingWildflowerMeadow
Northwestern University in Qatar
Tags
Full Transcript
Quiz 1 Preparation – Key Concepts Week 1: Course Introduction & The Importance of AI in Society 1. Digital Humanities: Intersection of digital technology and humanities. 2. AI in Society: How AI impacts various aspects of life. 3. Ethics in AI: Moral implications of AI technologies....
Quiz 1 Preparation – Key Concepts Week 1: Course Introduction & The Importance of AI in Society 1. Digital Humanities: Intersection of digital technology and humanities. 2. AI in Society: How AI impacts various aspects of life. 3. Ethics in AI: Moral implications of AI technologies. 4. AI Applications: Examples of AI in everyday life. 5. Digital Literacy: Importance of understanding digital tools. 6. Human-Machine Interaction: How humans and AI systems interact. 7. Future of AI: Potential developments and their societal impact. Week 2: History of AI & Core Concepts of AI 11. Early AI: Initial concepts and developments in AI. 12. Turing Test: A measure of a machine’s ability to exhibit intelligent behavior. 13. Symbolic AI: Early AI focused on logic and symbols. 14. Machine Learning: AI systems that learn from data. 15. Neural Networks: AI inspired by the human brain. 16. AI Winter: Periods of reduced funding and interest in AI. 17. AI Hype: Overestimations of AI capabilities. 18. AI Milestones: Key achievements in AI history. 19. AI Limitations: Current challenges and limitations of AI. 20. AI Ethics: Ethical considerations in AI development. 21. Expert Systems: AI systems that mimic the decision-making abilities of a human expert. 22. Knowledge Representation: How information is formally structured for AI to process. 23. Inference Engines: Components of AI that apply logical rules to the knowledge base to deduce new information. 24. Heuristics: Techniques used to solve problems faster when classic methods are too slow. 25. Genetic Algorithms: Optimization algorithms inspired by the process of natural selection. 26. Fuzzy Logic: A form of logic that deals with reasoning that is approximate rather than fixed and exact. 27. Robotics: The branch of technology that deals with the design, construction, operation, and application of robots. 28. Cognitive Computing: Systems that simulate human thought processes in a computerized model. 29. Swarm Intelligence: The collective behavior of decentralized, self-organized systems, typically natural or artificial. 30. Artificial General Intelligence (AGI): The hypothetical ability of an AI to understand, learn, and apply knowledge across a wide range of tasks, similar to human intelligence. Week 3: Introduction to NLP & Text Representation Techniques 31. Natural Language Processing (NLP): AI’s ability to understand and generate human language. 32. Syntax and Semantics: Structure and meaning in language. 33. Tokenization: Breaking text into smaller units. 34. Part-of-Speech Tagging: Identifying parts of speech in text. 35. Named Entity Recognition: Identifying proper names in text. 36. Language Models: Predicting the next word in a sequence. 37. Bag of Words: Simple text representation method. 38. TF-IDF: Term frequency-inverse document frequency for text analysis. 39. Word Embeddings: Representing words as vectors. 40. Contextual Embeddings: Advanced word representations considering context. 41. Sentiment Analysis: The process of determining the emotional tone behind a series of words. 42. Machine Translation: The use of AI to automatically translate text from one language to another. 43. Speech Synthesis: The artificial production of human speech. 44. Text Summarization: The process of creating a short and coherent version of a longer document. 45. Question Answering Systems: AI systems designed to answer questions posed by humans in natural language. 46. Information Retrieval: The process of obtaining relevant information from a large repository. 47. Coreference Resolution: The task of determining which words refer to the same entity in a text. 48. Discourse Analysis: The study of the ways in which language is used in texts and contexts. 49. Pragmatics: The study of how context influences the interpretation of meaning. 50. Morphological Analysis: The study of the structure and formation of words. Week 4: Introduction to Speech Processing & Overview of LLMs 51. Speech Processing: Converting speech to text and vice versa. 52. Automatic Speech Recognition (ASR): Technology that converts spoken language into text. 53. Text-to-Speech (TTS): Technology that converts text into spoken language. 54. Phonetics and Phonology: Study of sounds in speech. 55. Acoustic Models: Models that represent the relationship between audio signals and phonetic units. 56. Language Models in ASR: Predicting word sequences in speech. 57. Large Language Models (LLMs): Advanced AI models for understanding and generating text. 58. Transformers: A type of model architecture for NLP tasks. 59. BERT: Bidirectional Encoder Representations from Transformers. 60. GPT: Generative Pre-trained Transformer models. 61. Prosody: The patterns of rhythm and sound used in poetry and speech. 62. Voice Activity Detection (VAD): The process of detecting the presence of human speech in audio. 63. Speaker Recognition: The process of identifying a person based on their voice. 64. Speech Synthesis Markup Language (SSML): A standard for specifying the pronunciation, volume, pitch, and speed of synthesized speech. 65. End-to-End Models: AI models that learn to map input directly to output without intermediate steps. 66. Attention Mechanisms: Techniques that allow models to focus on specific parts of the input when making predictions. 67. Sequence-to-Sequence Models: Models that transform sequences from one domain to sequences in another domain. 68. Pre-training and Fine-tuning: The process of training a model on a large dataset and then fine-tuning it on a specific task. 69. Zero-shot Learning: The ability of a model to perform a task without having seen any examples of that task during training. 70. Transfer Learning: The practice of reusing a pre-trained model on a new, related problem. Week 5: LLMs Applications & AI Chatbots 71. Applications of LLMs: Uses of large language models in various fields. 72. Media Industry: Role of AI in media and content creation. 73. Content Generation: AI’s ability to create text, images, and videos. 74. Personalization: Tailoring content to individual preferences using AI. 75. AI Chatbots: Automated conversational agents. 76. Dialogue Systems: Systems designed to converse with humans. 77. Natural Language Understanding (NLU): AI’s ability to understand human language. 78. Conversational AI: AI designed for human-like interactions. 79. Innovations in Chatbots: Recent advancements in chatbot technology. 80. Ethical Considerations in Chatbots: Ensuring ethical use of conversational AI. 81. Conversational Agents: AI systems designed to engage in dialogue with humans. 82. Multimodal AI: AI that can process and understand multiple types of data, such as text, images, and audio. 83. Contextual Understanding: The ability of AI to understand the context in which a conversation is taking place. 84. Dialogue Management: The component of a conversational agent that manages the flow of conversation. 85. User Intent Recognition: The process of identifying the user’s goal or intention in a conversation. 86. Response Generation: The process of generating appropriate responses in a conversation. 87. Personal Assistants: AI systems designed to assist users with tasks such as scheduling, reminders, and information retrieval. 88. Customer Support Bots: AI systems used to provide customer service and support. 89. Interactive Voice Response (IVR): Technology that allows humans to interact with a computer-operated phone system through voice and DTMF tones. 90. Ethical AI in Chatbots: Ensuring that chatbots operate within ethical guidelines, respecting user privacy and avoiding harmful behavior.