PUB 222 WEEK 1.pptx
Document Details
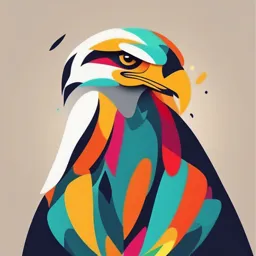
Uploaded by EngagingGhost
2024
Tags
Full Transcript
Introduction to Research Methodology in Public Administration Population and Sampling 22 July 2024 Population in Research Polit and Hungler (1999:37) refer to the population as an aggregate or totality of all the objects, subjects or members th...
Introduction to Research Methodology in Public Administration Population and Sampling 22 July 2024 Population in Research Polit and Hungler (1999:37) refer to the population as an aggregate or totality of all the objects, subjects or members that conform to a set of specifications. A population is the complete set of people or objects with a common characteristic of interest. The target population is the entire group the researcher wishes to generalize to. Importance of Defining the Population Helps determine the sampling frame and select an appropriate sample Ensures the sample is representative of the target population Allows for proper generalization of research findings Sampling Sampling is the act of selecting a group from a larger population to conduct measurements and gather data. The sample should accurately represent the population to ensure that the findings can be generalized. Researchers use sampling to draw conclusions about a population without needing to study every individual within that group, which is often impractical or impossible due to constraints like time, cost, and accessibility Types of Sampling: Probability sampling Probability sampling - also known as random sampling, is a sampling technique where the researcher selects a sample from a population based on the theory of probability. This means that every member of the population has a known, non-zero chance of being selected. Probability sampling is advantageous because it minimizes bias and allows for statistical inferences to be made about the population. However, it can be more time-consuming and expensive to implement compared to non-probability methods. Probability Sampling Techniques Simple Random Sampling: Each member of the population has an equal probability of being selected Systematic Sampling: Members are selected at regular intervals from a list Stratified Sampling: The population is divided into strata based on characteristics, then random samples are drawn from each stratum Cluster Sampling: The population is divided into clusters, then clusters are randomly selected and all members within the selected clusters are included Simple Random Sampling: Example Scenario The target population consists of all employees working at Raymond Mhlaba Municipality, say 1,000 employees. You (the researcher) decide to survey 100 (sample size) employees to gather insights into job satisfaction levels. A complete list of all 1,000 employees is compiled, ensuring that each employee's name is included. Each employee on the list is assigned a unique number from 1 to 1,000 Using a random number generator, the researcher selects 100 unique numbers corresponding to the employees. This ensures that each employee has an equal chance of being selected. Example Scenario: Systematic Sampling The target population consists of all employees within the Eastern Cape Department of Education, totaling 1000 employees. The researcher decides to survey 100 employees to assess their perceptions of the training programs. Each employee is assigned a unique number from 1 to 1000. The researcher calculates the sampling interval by dividing the total population size by the desired sample size. In this case, 1000/ 100 = 10 This means every 10th employee will be selected. The researcher randomly selects a starting point between 1 and 10. For example, if the random number chosen is 4, the first employee selected will be the one numbered 4. The researcher then selects every 10th employee from the starting point. This would include employees numbered 4, 14, 24, 34 Example Scenario: Stratified Sampling The target population consists of all registered second-year students at UFH totaling 2000 students. The researcher identifies key demographic characteristics to create strata. For instance, the population can be divided into strata based on age groups: 16-20 years (500) = 25%, 21-25 years (900) = 45%, 26-30 years (300) = 15%, 31 years and older (300) =15% The researcher decides to survey a total of 120 students to assess their satisfaction with the SRC The researcher examines the distribution of the population across the identified strata. For example, if the population distribution is as follows: 16-20 years (25% x120) = 30 21-25 years (45% x120) = 54 26-30 years (15% x120) = 18 31 years and older (15% x 120) = 18. The researcher then randomly selects individuals within each age group to ensure that the sample accurately reflects the demographic distribution of the 2nd year students. Example Scenario: Cluster Sampling Class exercise: As a public administration researcher, you have been tasked with evaluating the effectiveness of a new community policing initiative in a large city. The city is divided into 20 distinct neighborhoods. Based on the information above, in groups explain in depth the steps you would follow to conduct cluster sampling. Non Probability Sampling Non-probability sampling is a sampling technique where the researcher selects a sample based on subjective judgment rather than random selection. In this method, not all members of the population have an equal chance of being selected. Non-probability sampling is useful when probability sampling is not feasible, such as when studying hard-to-reach populations. However, it is more prone to bias and the findings cannot be generalized to the entire population. Non Probability Sampling Accidental or Convenience Sampling: Selecting readily available participants Purposive or Judgmental Sampling: Selecting participants based on the researcher's expertise Quota Sampling: Selecting participants to represent certain characteristics in the same proportion as the population Snowball Sampling: Participants recruit future participants from among their acquaintances