Portfolio Theory Lecture 6 PDF
Document Details
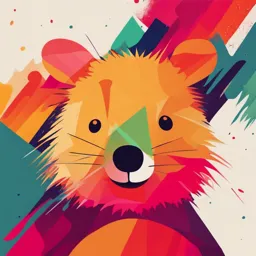
Uploaded by ImpeccableMorningGlory980
University of Groningen
2024
Tags
Summary
This University of Groningen lecture discusses Portfolio Theory, including the single index model, market models, and assumptions about the random error term. The lecture also covers shrinkage and covariance matrix estimation methods.
Full Transcript
1 Portfolio Theory 2024-2025 LECTURE 6 1 2 Agenda Index Models CAPM 2 3 What you will learn Using historical estimates of expected return, variances and...
1 Portfolio Theory 2024-2025 LECTURE 6 1 2 Agenda Index Models CAPM 2 3 What you will learn Using historical estimates of expected return, variances and covariance is potentially bad for (future) performance. Several ways of improving the model: – Shrink the covariance matrix: use an index model or the average correlation model to estimate the covariance matrix – Use the CAPM to generate expected stock returns, gives a reasonable estimate of the risk premium. 3 4 The Single Index Model Two basic version of the Single Index model: 1. The market model (Markowitz/Sharpe/Treynor) 𝑟𝑖𝑡 = 𝛼𝑖 + 𝛽𝑖 𝑟𝑚𝑡 + 𝜀𝑖𝑡 2. The Excess return form (Jensen) 𝑟𝑖𝑡 − 𝑟𝑓 = 𝛼𝑖 + 𝛽𝑖 𝑟𝑚𝑡 − 𝑟𝑓 + 𝜀𝑖𝑡 4 5 Single index model: The market model The market model (Markowitz/Sharpe/Treynor) 𝑟𝑖𝑡 = 𝛼𝑖 + 𝛽𝑖 𝑟𝑚𝑡 + 𝜀𝑖𝑡 The conditional expectation given a realization of the market index is: 𝐸 𝑟𝑖𝑡 |𝑟𝑚𝑡 = 𝛼𝑖 + 𝛽𝑖 𝑟𝑚𝑡 5 6 Assumptions about the random error term 1. The expected value of the random error term is zero 2. The variance of the random error term is constant over time (homoscedastic) 3. The error terms are uncorrelated with 𝑟𝑚𝑡 , that is 𝐶𝑂𝑉 𝜀𝑖𝑡 , 𝑟𝑚𝑡 = 0 4. The random error terms are serially uncorrelated 5. The random error terms of one asset are uncorrelated with those of any other asset: 𝐶𝑂𝑉 𝜀𝑖𝑡 , 𝜀𝑗𝑡 = 0 6 Systematic versus Unsystematic return 𝑟𝑖𝑡 = 𝛼𝑖 + 𝛽𝑖 𝑟𝑚𝑡 + 𝜀𝑖𝑡 Systematic return Unsystematic return The return decomposition can also be used to make a decomposition of variance / risk: 𝜎𝑖2 = 𝑏𝑖2 𝜎𝑚 2 + 𝜎2 𝑖𝑒 Systematic Unsystematic variance variance 8 Covariance between two stocks The covariance between two stocks can be written as: 2 +𝜎 𝜎𝑖,𝑗 = 𝛽𝑖 𝛽𝑗 𝜎𝑚 2 𝜀𝑖 𝜀𝑗 = 𝛽𝑖 𝛽𝑗 𝜎𝑚 The residual covariance is (by assumption) equal to zero. 8 9 Advantage of Market Model: Markowitz / Full historical Market Model E[r] 𝑛 𝛼𝑖 n 1 𝛽𝑖 𝑛 Cov. Matrix 𝑛+ 𝑛2 − 𝑛 2 𝜎𝜀𝑖 𝑛 1 𝐸 𝑟𝑚 1 Total: 2𝑛 + 𝑛2 − 𝑛 2 𝜎𝑚 1 Fewer estimates needed. Total: 3𝑛 + 2 9 10 Data requirements: Full Historical vs Market Model 25000 of parameter estimates Vertical axis: required number 20000 15000 10000 5000 0 0 50 100 150 200 250 Full Historical Market Model On the horizontal axis: number of securities 10 11 How to get the covariance matrix? › Sample covariance between two stocks 𝑇 𝑟𝑡1 − 𝐸 𝑟1 𝑟𝑡2 − 𝐸 𝑟2 𝜎12 = , 𝑇−1 𝑡=1 where 𝑇 it the number of observations 1 Matrix algebra: Ω = E 𝐑𝐓 𝐑 = 𝐑𝐓 𝐑 𝑇−1 where R is the matrix of returns in excess of their means. 11 12 Why is the covariance matrix important ? It affects the number of parameters to estimate However, it has other implications too: 1. It determines the future performance of the portfolios on the efficient frontier 2. It determines the sensitivity of the composition of the portfolios to changes / errors in estimating future expected returns 12 13 Full historical covariance matrix 1. Enter covar(series1;series2) pair by pair 2. Use the analysis toolpack, which is an Add-In in Excel (Data, Data Analysis) 3. Matrix formula: =MMULT( transpose(Datarange); Datarange)/T in a N by N array. Where Datarange represents the deviations from the mean See: tab “covariance matrix” 13 14 How to estimate the Market Model › Use the command ‘slope’ from Excel: this estimate the slope of a regression line. › Diagonal has the same variances as in the Historical model. › Off-diagonal elements: 𝜎𝑖,𝑗 = 𝛽𝑖 𝛽𝑗 𝜎𝑚2 + 𝜎𝜀𝑖 𝜀𝑗 = 𝛽𝑖 𝛽𝑗 𝜎𝑚2 Full historical Single index model covariance matrix 14 15 The step-by-step approach The approach that I propose the use here is much simpler than the one presented in 8.2. I use the same example as presented in ‘Example 8.4’: E[r] beta alpha Std Var Res std Res var 1 2.40% 0.959 1.488% 45.84% 21.01% 39% 15.42% 2 1.37% 1.055 0.035% 47% 22.27% 39% 15.50% 3 0.76% 1.065 -0.025% 49.40% 24.41% 42% 17.51% Market 0.954% 1 0.954% 24.66% Target 1.200% 15 16 Lagrange constraints We use the Lagrange objective function in the same way as in Lecture 2 We substitute the returns and covariances consistent with the Market Model. The objective function starts with the expression for portfolio variance and adds the two constraints multiplied with a multiplier, resp. λ and γ 𝑛 𝑛 𝑛 𝑛 1 𝐿 = 𝑤𝑖 𝑤𝑗 𝜎𝑖𝑗 + 𝜆 𝐸 𝑟𝑝 − 𝑤𝑖 𝐸 𝑟𝑖 + 𝛾 1 − 𝑤𝑖 2 𝑖=1 𝑗=1 𝑖=1 𝑖=1 16 17 Matrix representation C (Jakobian) x = b Francis & Kim, p 137/138 17 18 1: Covariance matrix with SI model. Market Model Covariance Matrix The diagonal presents the 1 2 3 variances of the stock 1 0.21012 0.06151 0.06210 returns as in the full 2 0.06151 0.22267 0.06831 historical covariance matrix 3 0.06210 0.06831 0.24406 The off-diagonal elements are 𝛽𝑖 𝛽𝑗 𝜎𝑚2 For example, the covariance between stock 2 and 3 is calculated as 1.055 × 1.065 ×0.24662 18 19 Create the covariance matrix for the SI Model: If you have a column b vector with beta’s for each stocks and a column vector of ε with the residual standard deviation of each stock and 𝜎𝑚 the standard deviation of the market index. Then, the covariance matrix for the market model can be created by: 𝚺 = 𝐛𝐛′ 𝝈𝟐𝒎 + 𝛆𝛆′ 𝐈 19 20 2. Create C matrix (Jakobian) C. (Jakobian) 1 0.21012 0.06151 0.06210 0.0240 1 2 0.06151 0.22267 0.06831 0.0137 1 3 0.06210 0.06831 0.24406 0.0076 1 Cond 1 0.02400 0.01370 0.00760 0 0 Cond 2 1 1 1 0 0 20 21 Step 3: Take the inverse and multiply it with vector b SI Model Inverse (C) Solution 1 0.5636 -1.5154 0.9517 65.8199 -0.6640 0 12.6% 2 -1.5154 4.0741 -2.5587 -13.0239 0.5393 0 38.3% 3 0.9517 -2.5587 1.6070 -52.7960 1.1247 0 49.1% Cond 1 65.8199 -13.0239 -52.7960 -1185.2514 18.6958 1.20% 4.47 Cond 2 -0.6640 0.5393 1.1247 18.6958 -0.4122 1 -0.19 The answer is close to Francis & Kim get at the end of Example 8.4 on page 186. The differences are because of the rounding errors. 21 22 Multi Index Models Multi Index Models can also be used to create optimal portfolios. Ri = i + i1I1 + i 2 I 2 +..... + iL I L + i 2 2 2 2 2 2 𝜎𝑖2 = 𝑏𝑖,1 𝜎𝑖,1 + 𝑏𝑖,2 𝜎𝑖,2 +...... +𝑏𝑖,𝐿 2 𝜎𝑖,𝐿 + 𝜎𝜀,𝑖 2 2 2 𝜎𝑖𝑗 = 𝑏𝑖,1 𝑏𝑗,1 𝜎𝐼,1 + 𝑏𝑖,2 𝑏𝑗,2 𝜎𝐼,2 +.... +𝑏𝑖,𝐿 𝑏𝑗,𝐿 𝜎𝐼,𝐿 + 𝑒𝑖,𝑗 Covariance between residuals is zero by assumption: 2 2 2 𝜎𝑖𝑗 = 𝑏𝑖,1 𝑏𝑗,1 𝜎𝐼,1 + 𝑏𝑖,2 𝑏𝑗,2 𝜎𝐼,2 +.... +𝑏𝑖,𝐿 𝑏𝑗,𝐿 𝜎𝐼,𝐿 22 23 The average correlation model The Market Model results in better future portfolio performance in relative to the full historical covariance matrix. Simpler models seem to have an advantage in the reality of everyday stock returns. An even simpler solution may even be to reduce that covariance matrix by using the average correlation between all individual stocks, and use that to calculate the covariance between individuals' stocks: 𝜌𝑖𝑗 = 𝐸[𝜌] …. 𝜎𝑖𝑗 = 𝐸[𝜌]𝜎𝑖 𝜎𝑗 23 This is the so-called average (or constant) correlation model. 23 24 Shrinkage Shrinking the covariance matrix is the practice of using fewer parameters to estimate the covariance matrix. This practice refers to the use of Single Index models, Multi Index models and the average correlation model. 24 25 An example Five potential investments with the following returns E[r] Std Covariance Matrix (Full Historical) A 13.0% 50.00% 0.2500 -0.0420 0.0900 0.0540 0.0360 B 6.9% 42.00% -0.0420 0.1764 -0.0126 0.0680 0.0504 C 8.2% 30.00% 0.0900 -0.0126 0.0900 0.0432 0.0288 D 6.0% 36.00% 0.0540 0.0680 0.0432 0.1296 0.0086 E 9.9% 48.00% 0.0360 0.0504 0.0288 0.0086 0.2304 M 8.0% 25.00% 25 26 An example: Average correlation model covariance matrix Correlation Matrix Average correlation covariance matrix 0.25 0.0441 0.0315 0.0378 0.0504 1 -0.2 0.6 0.3 0.15 -0.2 1 -0.1 0.45 0.25 0.0441 0.1764 0.02646 0.031752 0.042336 0.6 -0.1 1 0.4 0.2 0.0315 0.02646 0.09 0.02268 0.03024 0.3 0.45 0.4 1 0.05 0.0378 0.031752 0.02268 0.1296 0.036288 0.15 0.25 0.2 0.05 1 0.0504 0.042336 0.03024 0.036288 0.2304 The average correlation can be calculated from the correlation matrix: ሺ−0.2 + 0.60 + 0.30 … + 0.05ሻ/10 = 0.21 The diagonal elements are the same as in the full historical covariance matrix. The off-diagonal elements are calculated as 0.21𝜊𝑖 𝜊𝑗 For example, element 2,1 is calculated as 0.21× 0.50×0.42 = 0.0441 26 27 Comparing optimal portfolios We can use the Lagrange method for finding optimal portfolios with an expected return of 8% as before: Optimal Portfolio Average Correlation Model C: Jakobian b 0.2500 0.0441 0.0315 0.0378 0.0504 0.13 1 0 0.0441 0.1764 0.0265 0.0318 0.0423 0.069 1 0 0.0315 0.0265 0.0900 0.0227 0.0302 0.082 1 0 0.0378 0.0318 0.0227 0.1296 0.0363 0.06 1 0 0.0504 0.0423 0.0302 0.0363 0.2304 0.099 1 0 0.13 0.069 0.082 0.06 0.099 0 0 8% 1 1 1 1 1 0 0 1 27 28 Results Solution E[r] = 8% Full Historical Avg Correlation Results are very A 3.5% 8.0% different. B 29.9% 15.1% C 53.1% 42.8% Average Correlation D 5.3% 24.9% model also averages E 8.2% 9.3% portfolio weights. Lambda 0.044 -0.016 Gamma -0.055 -0.052 Cross. Std 19% 13% E[r] 8.0% 8.0% Std. dev (1) 22.8% 23.8% Std. dev (2) 24.8% 23.1% 28 29 The overall result (literature) Descriptive ability Predictive ability 1. Historical covariance matrix 1. Average correlation 2. Multi-index model 2. Single-index model 3. Single-index model 3. Multi-index model 4. Average correlation model 4. Historical covariance matrix 29 30 Why does the average correlation model perform better? The average correlation model and the index model dramatically reduce the need for estimating parameters. Estimation error is a large source of problems in portfolio optimization. The impact of an error in estimating expected stock return on the portfolio composition is larger if the correlation between two assets is high. Consider the following example, with two assets B and D, which are highly correlated 30 31 E[r] Std Correlation Matrix D A 13.0% 50.00% 1 -0.1 0.6 -0.1 0.2 B 6.9% 42.00% -0.1 1 -0.1 0.99 -0.1 C 8.2% 30.00% 0.6 -0.1 1 -0.1 0.2 D 6.9% 42.00% -0.1 0.99 -0.1 1 -0.1 E 9.9% 48.00% 0.2 -0.1 0.2 -0.1 1 M 8.0% 25.00% RF 4% Average Correlation 0.139 The optimal Full Hist Avg cor Full Hist Avg cor MVP MVP TP TP portfolios A -0.3% 10.4% 33.3% 32.9% We have calculated a B 16.1% 17.6% 13.4% 5.2% minimum variance portfolio C 51.4% 42.7% 17.0% 37.7% D 16.1% 17.6% 13.4% 5.2% and a tangency portfolio E 16.7% 11.8% 22.8% 19.0% for rf=4% for both covariance matrices E[r] 8.05% 8.44% 9.84% 9.96% 31 32 Example with high correlation (continued) Suppose that you estimate the expected return of B A E[r] 13.0% Std 50.00% is 0.5% higher than that of B. B 7.4% 42.00% Note: this is a small difference, it is often difficult to C D 8.2% 6.9% 30.00% 42.00% find a difference of 0.5% to be statistically significant. E 9.9% 48.00% M 8.0% 25.00% Full Hist Avg cor Full Hist Avg cor MVP MVP TP TP The solution for the full historical A -0.3% 10.4% 32.7% 32.0% covariance matrix turns D in a large B 16.1% 17.6% 174.3% 8.4% C 51.4% 42.7% 16.8% 36.5% short position, whereas the average D 16.1% 17.6% -146.3% 4.8% correlation model leads to a minor E 16.7% 11.8% 22.5% 18.3% change. E[r] 8.13% 8.53% 10.66% 9.92% 32 33 Estimation error Minor differences in estimations can result in huge fluctuations in the composition of the optimal portfolio. Historical estimates of expected stock returns are very vulnerable to estimation errors. There is strong evidence that the stocks with the highest returns in the past 5 years underperform in the next 5 years, and the worst performing stocks will outperform (DeBondt and Thaler, 1985) 33 34 DeBondt and Thaler, 1985 34 35 Use CAPM to generate expected returns Replacing the full historical covariance matrix with the covariance matrix from the Single Index model or the Market Model improves the stability of the model. Also improve the out-of-sample performance (the ‘real’ life implementation). Another big gain can be made by replacing the historical expected returns with those from the CAPM. 𝐸 𝑟𝑖𝑡 = 𝑟𝑓 + 𝛽𝑖 𝑟𝑚 − 𝑟𝑓 A practical approach is to estimate the beta using a standard regression model with Jensen’s version of the Single Index Model. Next you plug in the current risk-free rate, and a reasonable estimate for the market premium. 35 36 Conclusions The single index model and the average correlation model simplify the process of portfolio optimization because they require dramatically fewer parameters to be estimated As an additional benefit of this, these models perform much better as they reduce the negative side effects of estimation errors. Using CAPM generated expected returns also reduce the impact of estimation errors 36 37 Francis & Kim: Chapter 12 CAPITAL ASSET PRICING MODEL 37 38 Assumption underlying the CAPM 1. Investors in capital assets are risk-averse one-period expected-utility-of-wealth maximisers. 2. Investors make their decisions exclusively on the basis of the mean and standard deviation of returns. This is equivalent to expected wealth and its standard deviation. 3. The mean and standard deviation of returns (wealth) are finite numbers that do exist. 4. Perfect markets: securities are infinitely divisible, taxes do not impact security prices, there are no transaction costs, investors are not able to influence prices, etc.. 38 39 Assumptions (continued) 5. Borrowing and lending is possible at the same risk-free rate 6. All assets are marketable (include human capital, collector’s items, …) 7. Perfect capital markets: Information is freely and instantly available to every one, no margin requirements and no limitations on short sales. 8. Investors have homogeneous expectations over the same one-period investment horizon, and the same expectations with respect to returns, variances and covariances. (Idealized uncertainty). Communist Asset Pricing Model 39 40 Capital Market Line (CML) The CML represents the efficient frontier as viewed by all investors (due to homogeneous expectations) The Capital Market Line intersects the y-axis at the risk-free rate The slope of the Capital Market Line is equal to the market price per unit risk, also known as the Sharpe ratio: (𝑅𝑚 − 𝑅𝑓 ) 𝑆= σ𝑚 40 41 The capital market line 30.0% 25.0% 20.0% 15.0% Expected Return 10.0% 5.0% 0.0% 0.0% 10.0% 20.0% 30.0% 40.0% 50.0% 60.0% -5.0% -10.0% Standard Deviation Eff. Frontier with only risky assets Capital Market Line The CML represents combinations of the risk-free fund and the market portfolio. 41 42 The CML specification: 𝐸 𝑟𝑚 −𝑟𝑓 E𝑟 𝑝∗ = 𝑟𝑓 + 𝜎𝑝 ∗ , 𝜎𝑚 rp* is the return on any efficient portfolio rf is the risk-free rate rm is the return on the market portfolio σp* is the standard deviation of any efficient portfolio σm is the standard deviation of the market portfolio 42 43 CAPM is an equilibrium model CAPM is an equilibrium model: it describe the behavior of all investors simultaneously as well as their impact on security prices. All investors choose portfolios on the Capital Market Line (CML) However, all investors together own the market portfolio. The CML does not describe the relation between risk and return of inefficient portfolios and individual securities. 43 44 Individual securities If the market portfolio is well diversified, then its idiosyncratic risk tends to zero. We assume that it doesn’t exist Risk averse investors will minimize the risk of a portfolio given a particular return level. Beta is the index of systematic risk measured relative to the (efficient) market portfolio. Without idiosyncratic risk, there is only systematic risk. So Beta is a complete measure of risk. | 44 44 45 Security Market Line Eri = rf + i (Erm − rf ) ri is the return on any portfolio or security rf is the risk-free rate rm is the return on the market portfolio βi is the beta relative to the market portfolio All individual securities and portfolio - whether efficient or not - are on the Security Market Line 45 46 The Security Market Line R 16 13.6 R m R F ß 0 1 0.8 46 47 Example ABC company has a beta equal to 1.5 In 2006, the realized return on the market portfolio was 12%. In 2006, the risk-free rate was 4% The realized return on the stocks of ABC was 20% Was ABC undervalued or overvalued? Does the CAPM hold? 47 48 Answer We cannot say whether this stock is overvalued or undervalued. The SML specifies the relation between the expected return of an individual portfolio or stock and the expected return of the market. (The example was given in realized return!) There is also idiosyncratic risk! 48 49 Another example Suppose that we have the following information on the stock market. The expected return of the stock market is 6% and its standard deviation is 30%. The relevant risk- free rate is 3%. Suppose that we find an asset A that has an expected return of 7.5% and a standard deviation of 50%. Does the existence of asset A rejects the CAPM? Why? 49 50 Answer A is not on the Capital Market line. If it was on the Capital Market Line, it would have had an expected return of 3%+0.1 × 50% = 8%. However, this does not mean that CAPM should be rejected. A is an individual security, so we should check whether it is on the SML. Since we do not know its Beta, we cannot reject the CAPM. 50 51 Main prediction of CAPM Investors have the same portfolio of risky assets. The market portfolio reflects the collective expectations of the homogenous investors. Investors use all the potential for diversification. | 51 51 52 Do investors invest in the market portfolio? By definition: Yes. The sum of the holdings of all investors equals the market portfolio Most individual investors invest in poorly diversified portfolios of individual stocks mutual funds – Most mutual funds are actively managed ETFs and index funds Institutional investors invest a considerable amount of money in a passive way 52 53 Passive versus active investing Source: ICI Factbook 2024 53 54 Mutual market concentration ICI Factbook, 2024 54 55 ESG oriented funds 55 56 Conclusions The CAPM relies on a long list of assumptions Simple in its predictions – Everyone owns the market portfolio – Linear relation between beta and expected return Predictions are highly relevant for practitioners Big impact on the asset management industry. Indexation / ETFs. 56