Psychology Statistics Chapter 2 PDF
Document Details
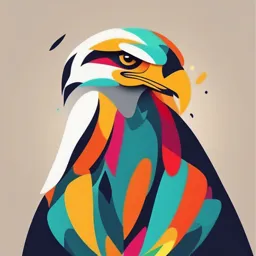
Uploaded by SolidElbaite6954
Ms. Rosian Mae S.P. Bermudo
Tags
Summary
This document covers concepts in central tendency and variability within psychological statistics. It includes definitions and examples of mean, median, mode, and different types of variability for a dataset. The document also includes practice questions on calculating mean, median, and mode.
Full Transcript
Chapter 2: Central Tendency and Variability Psyc115: Psychological Statistics Ms. Rosian Mae S.P. Bermudo, RPm. Objectives: At the end of this lesson, you will be going to learn the following: ❑ Central tendency ❑ Variability ❑ Z score ❑ The normal curv...
Chapter 2: Central Tendency and Variability Psyc115: Psychological Statistics Ms. Rosian Mae S.P. Bermudo, RPm. Objectives: At the end of this lesson, you will be going to learn the following: ❑ Central tendency ❑ Variability ❑ Z score ❑ The normal curve ❑ Sample and Population ❑ Probability The Central Tendency ✓ Central tendency is a statistical concept that refers to the measure that identifies the center or typical value of a data set. ✓ It provides a summary of the data by representing the "middle" or "average" value around which the data points are distributed. ✓ The most common measures of central tendency are Mean, Median and Mode The three measures of Central tendency The MEAN: The MEDIAN: The best measure of central The middle value in a data set when the tendency is the ordinary average, values are arranged in ascending or the sum of all the scores divided descending order. by the number of scores. If the data set has an even number of observations, the median is the average of The arithmetic average of all the the two middle numbers. data points. It is calculated by The MODE: adding all the values together and The value that occurs most frequently in a then dividing by the number of data set. A data set may have one mode, values. more than one mode, or no mode at all if no number repeats. Mean (Arithmetic Average) Suppose we have the following data set representing the number of books read by 5 people in a month: Data: 3, 7, 5, 10, 5, 9, 14 , 7, 3, 5 ,3, 3 Step 1: Add all the numbers together Step 2: Divide the sum by the number of data points What is the mean? M is a symbol for the mean. An alternative symbol, X (“X-bar”). ∑, the capital Greek letter sigma, is the symbol for “sum of.” It means “add up all the numbers for whatever follows.” It is the most common special arithmetic X stands for the scores in the distribution of the variable X. We could have picked any letter actually, However, if there is only one variable, it is usually called X. 2. Median (Middle Value) Try this new set: Data: 3, 7, 5, 10, 5, 9, 7, 3, 5 ,3, 3 Step 1: Arrange the numbers in ascending order: Step 2: Identify the middle value So, the median number of books read is ?. Median (Middle Value) Consider the same data set: Data: 3, 7, 5, 10, 5, 9, 14 , 7, 3, 5 ,3, 3 Step 1: Arrange the numbers in ascending order: Step 2: Identify the middle value So, the median number of books read is ?. Mode (Most Frequent Value) Consider the same data set: Data: 3, 7, 5, 10, 5, 9, 14 , 7, 3, 5 ,3, 3 Step 1: Identify the value that appears most frequently: The number 5 appears twice, while the others appear only once. There are some cases that a data set may have one mode, more than one mode, or no mode at all if no number repeats and here’s how to identify them 1. Unimodal - A data set is unimodal when there is only one mode, meaning a single value appears most frequently. Example: Data: 4, 2, 4, 6, 7 Mode: 4 (since it appears twice, more than any other value) 2. Bimodal - A data set is bimodal when there are two modes, meaning two different values appear with the same highest frequency. Example: Data: 4, 2, 4, 6, 2Modes: 4 and 2 (both appear twice) 3. Multimodal - A data set is multimodal when there are more than two modes, meaning three or more values appear with the same highest frequency. Example: Data: 4, 2, 4, 6, 2, 6 -- Modes: 4, 2, and 6 (all appear twice) 4. No Mode (Amodal) - A data set is considered to have no mode if no number repeats, meaning every value appears with the same frequency. Example: Data: 1, 2, 3, 4, 5 -- Mode: None (since no number repeats) Concept Check 1. Name and define three measures of central tendency. 2. Write the formula for the mean and define each of the symbols. 3.Why is the mean different from the median? Concept Check 1. Name and define three measures of central tendency. 2. Write the formula for the mean and define each of the symbols. 3.Why is the mean different from the median? 4. Data Set (representing the scores of 20 students on a math test): 78, 85, 92, 88, 73, 85, 95, 67, 88, 90, 77, 85, 82, 91, 88, 90, 85, 83, 88, 92 Variability: You can think of the variability of a distribution as the amount of spread of the scores around the mean. In other words, how close or far from the mean are the scores in a distribution? Variability, also known as dispersion (the action or process of distributing) Understanding variability helps to give a fuller picture of a data set beyond just the central tendency, as it reveals the degree of uncertainty, consistency, or diversity within the data. There are common measure of variability; 1. Range 2. Variance 3. Standard Deviation Range: The simplest measure of variability. It is the difference between the highest and lowest values in a data set. Formula: Range= Maximum Value−Minimum Value Example: For the data set 5, 8, 12, 15, 18, The range is 18−5=13, 18-8=10, 18-12=6, 18-15=3, 18-18=0 Variance: It measures how far each data point is from the mean and is calculated by averaging the squared differences from the mean. A higher variance indicates that the data points are more spread out from the mean. ❶ Subtract the mean from each score. (Deviation score) ❷ Square each of these deviation scores (multiply each by itself). This gives each score’s Squared deviation score. ❸ Add up the squared deviation scores. This total is called the sum of squared deviations. ❹ Divide the sum of squared deviations by the number of scores. This gives the average (the mean) of the squared deviations, called the variance. Variance: It measures how far each data point is from the mean and is calculated by averaging the squared differences from the mean. A higher variance indicates that the data points are more spread out from the mean. ❶ Subtract the mean from each score. This gives each score’s deviation score which is how far away the score is from the mean. ❷ Square each of these deviation scores (multiply each by itself). This gives each score’s squared deviation score. ❸ Add up the squared deviation scores. This total is called the sum of squared deviations. ❹ Divide the sum of squared deviations by the number of scores. This gives the average (the mean) of the squared deviations, called the variance. Standard Deviation The square root of the variance, it gives a measure of spread in the same units as the data, making it easier to interpret. ❶ Figure the variance. ❷ Take the square root. The standard deviation is the positive square root of the variance. (Any number has both a positive and a negative square root. For example, the square root of 9 is both+ 3 and - 3. But for the standard deviation we always use the positive square root.) 1. For the following scores, find the (a) mean, (b) median, (c) sum of squared deviations, (d) variance, and (e) standard deviation: 32, 28, 24, 28, 28, 31, 35, 29, 26 2. For the following scores, find the (a) mean, (b) median, (c) sum of squared deviations, (d) variance, and (e) standard deviation: 6, 1, 4, 2, 3, 4, 6, 6