PSCI 2702 Chapter 4. PDF
Document Details
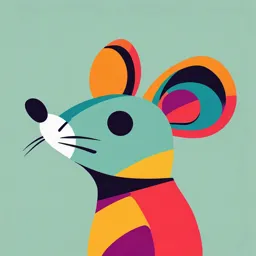
Uploaded by ConscientiousEvergreenForest1127
Toronto Metropolitan University
Tags
Summary
This document details the normal curve as a concept in statistics, used with the mean and standard deviation to describe empirical distributions. It's explained that the normal curve is a theoretical model that can be used to describe other distributions. The document covers hypothetical distributions of IQ scores and explains how areas under the normal curve correspond to proportions.
Full Transcript
Book Title: eTextbook: Statistics: A Tool for Social Research and Data Analysis, Fifth Canadian Edition Chapter 4. The Normal Curve 4.1. Introduction 4.1. Introduction 126 The normal curve is a concept of great importance in statistics. In combination wit...
Book Title: eTextbook: Statistics: A Tool for Social Research and Data Analysis, Fifth Canadian Edition Chapter 4. The Normal Curve 4.1. Introduction 4.1. Introduction 126 The normal curve is a concept of great importance in statistics. In combination with the mean and standard deviation, the normal curve can be used to construct precise descriptive statements about empirical distributions. In addition, as we shall see in Part 2, the normal curve is also central to the theory that underlies inferential statistics. This chapter will conclude our treatment of descriptive statistics in Part 1 and lay important groundwork for Part 2. The normal curve is a highly important theoretical model, a special kind of perfectly smooth frequency polygon that is unimodal (i.e., it has a single mode or peak) and symmetrical (unskewed) so that its mean, median, and mode all have the same value. The normal curve is also bell-shaped, with its tails extending infinitely in both directions. Even though no empirical distribution has a shape that perfectly matches this ideal model, many variables (e.g., test results from large classes, test scores such as the GRE, people’s height and weight) are close enough to permit the assumption of normality. In turn, this assumption makes possible one of the most important uses of the normal curve —to describe empirical distributions based on our knowledge of the theoretical normal curve. The crucial point about the normal curve is that distances along the abscissa (horizontal axis) of the distribution, when measured in standard deviations from the mean, always encompass the same proportion of the total area under the curve. In other words, on any normal curve, the distance from any given point to the mean (when measured in standard deviations) cuts off the same proportion of the total area. To illustrate, Figures 4.1 and 4.2 present two hypothetical distributions of IQ scores, one for a sample of children and one for a sample of adults (18+), both normally distributed (or nearly so), with the following means, standard deviations, and sample sizes: Children Adults (18+) ¯ ¯ X = 100 X = 100 s = 20 s = 10 N = 1,000 N = 1,000 Figures 4.1 and 4.2 are drawn with two scales on the horizontal axis or abscissa of the graph. The upper scale is stated in “IQ units” and the lower scale in standard deviations from the mean. These scales are interchangeable, and we can easily shift from one to the other. For example, for the children, an IQ score of 120 is one standard deviation (remember that, for the sample of children, s = 20 ) above the mean and an IQ of 140 is two standard deviations above (to the right of) the mean. Scores to the left of the mean are marked as negative values because they are less than the mean. An IQ score of 80 is one standard deviation below the mean, an IQ score of 60 is two standard deviations less 127 than the mean, and so forth. Figure 4.2 is marked in a similar way except that, because its standard deviation is a different value (s = 10) , the markings occur at different points. For the sample of adults, one standard deviation above the mean is an IQ of 110 , one standard deviation below the mean is an IQ of 90 , and so forth. Figure 4.1 IQ Scores for a Sample of Children Figure 4.2 IQ Scores for a Sample of Adults (18+) Recall that, on any normal curve, distances along the horizontal axis (or abscissa), when measured in standard deviations, always encompass the same proportion of the total area under the curve. Specifically, the distance between one standard deviation above the mean and one standard deviation below the mean (or ±1 standard deviation) encompasses exactly 68.26% of the total area under the curve. This means that in Figure 4.1, 68.26% of the total area lies between the score of 80 ( −1 standard deviation) and 120 ( +1 standard deviation). The standard deviation for the sample of adults is 10 , so the same percentage of the area (68.26%) lies between the scores of 90 and 110. If an empirical distribution is normal, 68.26% of the total area is 128 always encompassed between −1 and +1 standard deviation—regardless of the trait being measured and the number values of the mean and standard deviation. Taking the normal curve’s fixed relationship between the mean and standard deviation a little further, we see the following relationships between distances from the mean and areas under the curve: Between Lies −1 and +1 standard deviation 68.26% of the area −2 and +2 standard deviations 95.44% of the area −3 and +3 standard deviations 99.72% of the area These relationships are displayed graphically in Figure 4.3. Note that the relationships apply equally to normally distributed data in the population, but, instead, Greek letters are used to represent the mean, μ , and standard deviation, σ (see Section 3.5). So, 68.26% of all cases in a normally distributed population are contained within ±1σ of μ , 95.44% of all cases in a normally distributed population are contained within ±2σ of μ , and 99.72% of all cases in a normally distributed population are contained within ±3σ of μ. For the sake of brevity, we will refer to only sample data and symbols ( X and s) for the remainder of this chapter. ¯ 129 Figure 4.3 Areas under the Theoretical Normal Curve The relationship between distance from the mean and area allows us to describe an empirical distribution of a variable in the sample (or population), if it is at least approximately normal. The position of individual scores can be described with respect to the mean, the distribution as a whole, or any other score in the distribution. The areas between scores can also be expressed, if desired, in numbers of cases rather than percentage of total area. For example, a normal distribution of 1,000 cases will contain about 683 cases ( 68.26% of 1,000 cases) between −1 and +1 standard deviation of the mean, about 954 cases ( 95.44% of 1,000 cases) between −2 and +2 standard deviations, and about 997 cases ( 99.72% of 1,000 cases) between −3 and +3 standard deviations. Thus, for any normal distribution, only a few cases will be farther away from the mean than ±3 standard deviations. Book Title: eTextbook: Statistics: A Tool for Social Research and Data Analysis, Fifth Canadian Edition Chapter 4. The Normal Curve 4.2. Computing Z Scores (Standard Scores) 4.2. Computing Z Scores (Standard Scores) To find the percentage of the total area (or number of cases) above, below, or between scores in an empirical distribution, we must first express the original scores in units of the standard deviation or convert them to Z scores, which are also called standard scores. The original scores could be in any unit of measurement (metres, IQ, dollars), but Z scores always have the same values for their mean (zero) and standard deviation (one). Think of converting the original scores into Z scores as a process of changing value scales—like changing from metres to yards, kilometres to miles, or gallons to litres. These units are different but equally valid ways of expressing distance, length, or volume. For example, a mile is equal to 1.61 kilometres, so two towns that are 10 miles apart are also 16.1 kilometres apart and a 5 km race covers 3.11 miles. Although you may be more familiar with kilometres than miles, either unit works perfectly well as a way of expressing distance. In the same way, the original (or “raw”) scores and Z scores are two equally valid but different ways of measuring distances under the normal curve. In Figure 4.1, for example, we could describe a particular score in terms of IQ units (“Amal’s score was 120 ”) or standard deviations (“Amal scored one standard deviation above the mean”). When we compute Z scores, we convert the original units of measurement (IQ units, centimetres, dollars, etc.) to Z scores and, thus, “standardize” the normal curve to a distribution that has a mean of zero and a standard deviation of one. The mean of the empirical normal distribution is converted to zero, its standard deviation is converted to one, and all values are expressed in Z-score form. The formula for converting original scores in a sample to Z scores is: 130 Formula 4.1 ¯ Xi − X Z= s Formula 4.1 converts any score (Xi) from an empirical distribution to the equivalent Z score. To illustrate, consider the following sample of scores from Table 3.8: 10 , 20 , 30 , 40 , and 50. Their Z-score equivalents are presented in Table 4.1. Table 4.1 Computing Z Scores for a Distribution of Original Scores Score (Xi) ¯ Xi − X Z= s 10 10 − 30 = −1.414 14.14 20 20 − 30 = −0.707 14.14 30 30 − 30 = 0.000 14.14 40 40 − 30 = 0.707 14.14 50 50 − 30 = 1.414 14.14 Recall from Section 3.5 in Chapter 3 that X = 30 and s = 14.14. ¯ A Z score of positive 1.00 indicates that the original score lies one standard deviation unit above (to the right of) the mean. A Z score of negative 1.00 falls one standard deviation unit below (to the left of) the mean. Thus, in the above example, the Z score of 1.414 indicates that the original score of 50 lies 1.414 standard deviation units above the mean, while −1.414 indicates that the original score of 10 lies 1.414 standard deviation units below the mean. By inspection, you may notice that the distribution of Z scores in Table 4.1 has a mean of zero and a standard deviation of one. To substantiate this observation, the mean and standard deviation of the Z distribution are computed using Formulas 3.4 and 3.7. The results are shown in Table 4.2. (For practice in computing Z scores, see any of the problems at the end of this chapter.) Table 4.2 Computing the Mean and Standard Deviation for a Distribution of Z Scores One Step at a Time Finding Z Scores 1: Subtract the value of the mean (X ) from the value of the score ¯ (Xi). 2: Divide the quantity found in step 1 by the value of the standard deviation (s). The result is the Z-score equivalent for this raw score. Book Title: eTextbook: Statistics: A Tool for Social Research and Data Analysis, Fifth Canadian Edition Chapter 4. The Normal Curve 4.3. The Standard Normal Curve Table 4.3. The Standard Normal Curve Table 131 The theoretical normal curve has been thoroughly analyzed and described by statisticians. The areas related to any Z score have been precisely determined and organized in table format. This standard normal curve table or Z-score table is presented as Appendix A in this textbook, and a small portion of it is reproduced here in Table 4.3 for the purposes of illustration. 132 Table 4.3 An Illustration of How to Find Areas under the Normal Curve Using Appendix A (a)Z (b) Area between Mean and (c) Area beyond Z Z 0.00 0.0000 0.5000 0.01 0.0040 0.4960 0.02 0.0080 0.4920 0.03 0.0120 0.4880 ⋮ ⋮ ⋮ 1.00 0.3413 0.1587 1.01 0.3438 0.1562 1.02 0.3461 0.1539 1.03 0.3485 0.1515 ⋮ ⋮ ⋮ 1.50 0.4332 0.0668 1.51 0.4345 0.0655 1.52 0.4357 0.0643 1.53 0.4370 0.0630 ⋮ ⋮ ⋮ The standard normal curve table consists of three columns, with Z scores in the left-hand column (a), area between the Z score and the mean of the curve in the middle column (b), and area beyond the Z score in the right-hand column (c). To find the area between any Z score and the mean, go down the column labelled Z until you find the Z score. For example, go down column (a) either in Appendix A or in Table 4.3 until you find a Z score of +1.00. The entry in column (b) (“Area between Mean and Z”) is 0.3413. The table presents all areas in the form of proportions, but we can easily translate these into percentages by multiplying them by 100% (see Chapter 2). We could say either “a proportion of 0.3413 of the total area under the curve lies between a Z score of 1.00 and the mean,” or “ 34.13% of the total area lies between a Z score of 1.00 and the mean.” To illustrate further, find the Z score of 1.50 either in column (a) of Appendix A or the abbreviated table presented in Table 4.3. This score is 1.50 standard deviations to the right of the mean and corresponds to an IQ of 130 for the children’s IQ data (Figure 4.1). The area in column (b) for this score is 0.4332. This means that a proportion of 0.4332 —or a percentage of 43.32% —of all the area under the curve lies between this score and the mean. The third column in the table, column (c), presents “Area beyond Z.” These are areas above positive scores or below negative scores. This column is used when we want to find an area above or below certain Z scores, an application that is explained in Section 4.4. To conserve space, the standard normal curve table in Appendix A includes only positive Z scores. Because the normal curve is perfectly symmetrical, however, the area between the score and the mean (column (b)) for a negative score is the same as that for a positive score of the same numerical value. For example, the area between a Z score of −1.00 and the mean is also 0.3413 , or 34.13% , the same as the area we found previously for a score of +1.00. Notice that areas are always positive values, regardless of whether a Z score is positive or negative; however, as is repeatedly demonstrated below, the sign of the Z score is extremely important and should be carefully noted. For practice in using Appendix A to describe areas under an empirical normal curve, verify that the Z scores and areas given below are correct for the sample distribution of children’s IQ. For each IQ score, the equivalent Z score is computed using Formula 4.1, and then Appendix A is used to find areas between the score and the mean. ( X = 100 , s = 20 throughout.) ¯ IQ Score Z Score Area Between Z and Mean 110 +0.50 19.15% 125 +1.25 39.44% 133 +1.65 45.05% 138 +1.90 47.13% 133 The same procedures apply when the Z-score equivalent of an actual score happens to be a negative value (i.e., when the raw score lies below the mean). IQ Score Z Score Area between Z and the Mean 93 −0.35 13.68% 85 −0.75 27.34% 67 −1.65 45.05% 62 −1.90 47.13% Remember that the areas in Appendix A are the same for Z scores of the same numerical value regardless of sign. The area between the score of 138 ( +1.90 ) and the mean is the same as the area between the score of 62 ( −1.90 ) and the mean. (For practice in using the standard normal curve table, see any of the problems at the end of this chapter.) Book Title: eTextbook: Statistics: A Tool for Social Research and Data Analysis, Fifth Canadian Edition 4.4. Finding the Total Area Above and Below a Score 4.4. Finding the Total Area Above and Below a Score To this point, we have seen how the normal curve table can be used to find areas between a Z score and the mean. The information presented in the table can also be used to find other kinds of areas in an empirical distribution of a variable in the sample (or population), if it is at least approximately normal in shape. For example, suppose you need to determine the total area below the scores of two child subjects in the sample distribution described in Figure 4.1. The first subject has a score of 117 (X1 = 117) , which is equivalent to a Z score of +0.85 : ¯ Xi − X 117 − 100 17 Z= = = = +0.85 s 20 20 The plus sign of the Z score indicates that the score should be placed above (to the right of) the mean. To find the area below a positive Z score, the area between the score and the mean (given in column (b)) must be added to the area below the mean. As we noted earlier, the normal curve is symmetrical (unskewed), and its mean is equal to its median. Therefore, the area below the mean (just like the median) is 0.50 or 50%. Study Figure 4.4 carefully. We are interested in the shaded area. Figure 4.4 Finding the Area below a Positive Z Score By consulting the normal curve table, we find that the area between the score and the mean (see column (b)) is 0.3023 , or 30.23% , of the total area. The area below a Z score of +0.85 is therefore 0.8023 or 80.23% (50.00% + 30.23%). This subject scored higher than 80.23% of the sample tested. The second subject has an IQ score of 73 (X2 = 73) , which is equivalent to a Z score of −1.35 : ¯ X2 − X 73 − 100 −27 Z2 = = = = −1.35 s 20 20 134 To find the area below a negative score, we use the column labelled “Area beyond Z.” The area of interest is depicted in Figure 4.5, and we must determine the size of the shaded area. The area beyond a score of −1.35 is given as 0.0885 , which we can express as 8.85%. The second subject (X2 = 73) scored higher than 8.85% of the tested group. Figure 4.5 Finding the Area below a Negative Z Score In these examples, we use the techniques for finding the area below a score. Essentially the same techniques are used to find the area above a score. If we need to determine the area above an IQ score of 108 , for example, we first convert to a Z score: ¯ Xi − X 108 − 100 8 Z= = = = +0.40 s 20 20 and then proceed to Appendix A. The shaded area in Figure 4.6 represents the area we are interested in. The area above a positive score is found in the “Area beyond Z” column, and, in this case, the area is 0.3446 , or 34.46%. Figure 4.6 Finding the Area above a Positive Z Score These procedures are summarized in Table 4.4. To find the total area above a positive Z score or below a negative Z score, go down the “Z” column of 135 Appendix A until you find the standard score. The area you are seeking will be in the “Area beyond Z” column (column (c)). To find the total area below a positive Z score or above a negative score, locate the standard score and then add the area in the “Area between Mean and Z”(column (b)) to either 0.5000 (for proportions) or 50.00% (for percentages). These techniques might be confusing at first, and you will find it helpful to draw the curve and shade in the areas you are interested in. Table 4.4 Finding Areas above and below Positive and Negative Z Scores To Find Area: When the Z Score Is Positive Negative Above Z Look in column (c) Add column (b) area to 0.5000 or 50.00% Below Z Add column (b) area to Look in column (c) 0.5000 or 50.00% Book Title: eTextbook: Statistics: A Tool for Social Research and Data Analysis, Fifth Canadian Edition 4.4. Finding the Total Area Above and Below a Score Finding Raw Scores Finding Raw Scores Sometimes we want to work backward and find a raw score when only a percentile has been reported. (Percentiles identify the point below which a specific percentage of cases fall. We first encountered them in Chapter 3 in calculating the interquartile range, where we found three specific values: the first quartile, which is simply the 25th percentile; the second quartile, or 50 th percentile (the median); and the third quartile, or 75th percentile.) If a set of scores is normally distributed, we can use what we know about finding the area above (or below) a Z score to find the original, raw score. For example, let’s say that one of the adults whose IQ data are provided in Section 4.1 is told that their IQ is at the 98.5th percentile. In other words, 98.50% of all cases had a lower IQ score, as illustrated in Figure 4.7. They now want to know their raw IQ score. 136 Figure 4.7 Finding the Raw Score of the 98.50th Percentile Since we know that the mean of the IQ data for adults is 100 and the standard deviation is 10 , we only need to find the Z score of the 98.5th percentile to calculate the subject’s raw score, Xi. To do so, we must first find the area between the mean and the Z score. Scrolling down column (b) in Appendix A, we see that an area of 0.4850 (or 48.50% ) corresponds to a Z score value of 2.17. (We also know that the area below the mean contains 0.5000 , or 50.00% , of all scores.) Second, we insert the values of Z, X , and s into Formula 4.1 as follows: ¯ ¯ Xi − X Xi − 100 Z= = 2.17 = s 10 Then, through algebraic manipulation of this equation, we can find the raw score, Xi. Xi = (2.17)(10) + 100 = 121.70 The adult whose IQ is at the 98.5th percentile has a raw IQ score of 121.70. (For practice in finding areas above or below Z scores, see Problems 4.1, 4.2, 4.3, 4.4, 4.5, 4.6, and 4.7 at the end of this chapter. For practice in computing raw scores from percentiles, see Problems 4.8, 4.9, 4.10, and 4.11.) Book Title: eTextbook: Statistics: A Tool for Social Research and Data Analysis, Fifth Canadian Edition Chapter 4. The Normal Curve 4.5. Finding Areas between Two Scores 4.5. Finding Areas between Two Scores On occasion, you will need to determine the area between two scores rather than the total area above or below one score. In the case where the scores are on opposite sides of the mean, the area between the scores can be found by adding the areas between each score and the mean. Using the sample data of children’s IQ as an example, if we wished to know the area between the IQ scores of 93 and 112 , we would convert both scores to Z scores, find the area between each score and the mean from Appendix A, and add these two 137 areas together. The first IQ score of 93 converts to a Z score of −0.35 : ¯ Xi − X 93 − 100 −7 Z1 = = = = −0.35 s 20 20 The second IQ score (112) converts to +0.60 : ¯ X2 − X 112 − 100 12 Z2 = = = = 0.60 s 20 20 Both scores are placed on Figure 4.8. We are interested in the total shaded area. The total area between these two scores is 0.1368 , or 13.68% , + 0.2257 , or 22.57% , equaling 36.25%. Therefore, 36.25% of the total area or about 363 of the 1,000 cases lie between the IQ scores of 93 and 112. Figure 4.8 Finding the Area between Two Scores on Opposite Sides of the Mean When the scores of interest are on the same side of the mean, a different procedure must be followed to determine the area between them. For example, if we are interested in the area between the scores of 113 and 121 , we begin by converting these scores to Z scores: ¯ X1 − X 113 − 100 13 Z1 = = = = +0.65 s 20 20 ¯ X2 − X 121 − 100 21 Z2 = = = = +1.05 s 20 20 The scores are noted in Figure 4.9; we are interested in the shaded area. To find the area between two scores on the same side of the mean, find the area between each score and the mean (given in column (b) of Appendix A), and then subtract the smaller area from the larger. Between the Z score of +0.65 and the mean lies 0.2422 , or 24.22% , of the total area. Between +1.05 and the mean lies 0.3531 , or 35.31% , of the total area. Therefore, the area between these two scores is 35.31% − 24.22% , or 11.09% of the total area (or about 111 of the 1,000 cases). The same technique is followed if both scores are below the mean. 138 Figure 4.9 Finding the Area between Two Scores on the Same Side of the Mean The procedures for finding areas between two Z scores are summarized in Table 4.5 and in the One Step at a Time box. (For practice in finding areas between two scores, see Problems 4.3, 4.4, 4.5, 4.6, 4.7, and 4.12.) Table 4.5 Finding Areas between Scores Situation Procedure Scores are on the same side of Find the area between each the mean score and the mean in column Scores are on opposite sides of (b). Subtract the smaller area from the larger area. the mean Find the area between each score and the mean in column (b). Add the two areas together. Applying Statistics 4.1. Finding the Area below or above a Z Score You have just received your score on a driver’s licence test. If your score is 78 and you know that the mean score on the test is 67 with a standard deviation of 5 , how does your score compare with the distribution of all test scores? If you can assume that the test scores are normally distributed, you can compute a Z score and find the area below or above your score. The Z- score equivalent of your raw score is ¯ Xi − X 78 − 67 11 Z= = = = +2.20 s 5 5 Turning to Appendix A, we find that the “Area between Mean and Z” for a Z score of 2.20 is 0.4861 , which can also be expressed as 48.61%. Since this is a positive Z score, we need to add this area to 0.50 , or 50.00% , to find the total area below. Your score is higher than 48.61% + 50.00% , or 98.61% , of all the test scores. You did well! 139 One Step at a Time Finding Areas between Z Scores 1: Compute the Z scores for both raw scores. Note whether the scores are positive or negative. If the Scores Are on the Same Side of the Mean ⇓ 3: Subtract the smaller area from the larger area. Multiply this value by 100% to express it as a percentage. 2: Find the areas between each score and the mean in column (b). If the Scores Are on Opposite Sides of the Mean ⇓ 3: Add the two areas together to get the total area between the scores. Multiply this value by 100% to express it as a percentage. Applying Statistics 4.2. Finding the Area between Z Scores All sections of Political Science 101 at a large university were given the same final exam. Test scores were distributed normally, with a mean of 72 and a standard deviation of 8. What percentage of students scored between 60 and 69 (a grade of C), and what percentage scored between 70 and 79 (a grade of B)? The first two scores are both below the mean. Using Table 4.5 as a guide, we must first compute Z scores, then find areas between each score and the mean, and then subtract the smaller area from the larger: ¯ Xi − X 60 − 72 −12 Z1 = = = = −1.50 s 8 8 ¯ Xi − X 69 − 72 −3 Z2 = = = = −0.37 s 8 8 Using column (b), we see that the area between Z = −1.50 and the mean is 0.4332 and the area between Z = −0.37 and the mean is 0.1443. Subtracting the smaller from the larger (0.4332 − 0.1443) gives 0.2889. Changing to percentage format, we can say that 28.89% of the students earned a C on the test. (Of course, since we do not know the total number of students who wrote the final exam, we cannot calculate the exact number of students that this percentage represents.) To find the percentage of students who earned a B, we must add the column (b) areas together, since the scores ( 70 and 79 ) are on opposite sides of the mean (see Table 4.4): ¯ Xi − X 70 − 72 −2 Z1 = = = = −0.25 s 8 8 ¯ Xi − X 79 − 72 7 Z2 = = = = 0.87 s 8 8 Using column (b), we see that the area between Z = −0.25 and the mean is 0.0987 and that the area between Z = 0.87 and the mean is 0.3078. Therefore, the total area between these two scores is 0.0987 + 0.3078 , or 0.4065. Translating to percentages again, we can say that 40.65% of the students earned a B on this test. (We can only calculate the exact number of students that this percentage represents if we know the total number of students who wrote the final exam.) 140 Book Title: eTextbook: Statistics: A Tool for Social Research and Data Analysis, Fifth Canadian Edition 4.6. Using the Normal Curve to Estimate Probabilities 4.6. Using the Normal Curve to Estimate Probabilities To this point, we have thought of the theoretical normal curve as a way of describing the proportion or percentage of total area above, below, and between scores in an empirical distribution of interval-ratio variable scores. We have also seen that these areas can be converted into the number, proportion, or percentage of cases above, below, and between scores. In this section, we introduce the idea that the theoretical normal curve may also be thought of as a distribution of probabilities. Specifically, we may use the properties of the theoretical normal curve (Appendix A) to estimate the probability that a case randomly selected from an empirical normal distribution of interval-ratio variable scores has a score that falls in a certain range. In terms of techniques, these probabilities are found in the same way as areas are found. Before we consider these mechanics, however, let us examine what is meant by the concept of probability. Although we are rarely systematic or rigorous about it, we all attempt to deal with probabilities every day, and, indeed, we base our behaviour on our estimates of the likelihood that certain events will occur. We often ask (and answer) questions such as, “What is the probability of rain?” “What is the probability of drawing a king of hearts from a deck of cards?” “What is the probability of the worn-out tires on my car going flat?” “What is the probability of getting at least 80% on an exam?” Probability is simply defined as the likelihood that some event (like rain) will occur. To calculate the probability of an event, we must first be able to define what constitutes a “success.” The examples above contain several different definitions of a success (i.e., rain, drawing a certain card, flat tires, and exam grades). To determine a probability, a fraction must be established, with the numerator equalling the number of events that constitute a success and the denominator equalling the total number of possible events where a success could theoretically occur: # successes Probability = # events To illustrate, assume that we wish to know the probability of selecting the king of hearts in one draw from a well-shuffled deck of cards. Our definition of a success is quite specific (drawing the king of hearts), and with the information given, we can establish a fraction. Only one card satisfies our definition of success, so the number of events that constitute a success is 1 ; this value is the numerator of the fraction. There are 52 possible events (i.e., 52 cards in the deck), so the denominator is 52. The fraction is thus 1/52 , which represents the probability of selecting the king of hearts on one draw from a well-shuffled deck of cards. Our probability of success is 1 out of 52. We can leave the fraction established above as it is, or we can express it as a proportion by dividing the numerator by the denominator—the corresponding proportion is 0.0192 , which is the proportion of all possible events that satisfy our definition of a success. Probabilities are usually expressed as proportions, and we will follow this convention throughout the remainder of 141 this section. Using p to represent “probability,” the probability of drawing the king of hearts (or any specific card) can be expressed as # successes 1 p(king of hearts) = = = 0.0192 # events 52 As conceptualized here, probabilities have an exact meaning: over the long run, the events we define as successes will bear a certain proportional relationship to the total number of events. The probability of 0.0192 for selecting the king of hearts in a single draw really means that, over an infinite number of draws of one card at a time from a full deck of 52 cards, the proportion of successful draws will be 0.0192. Or, for every 10,000 draws, about 192 will be the king of hearts, and the remaining 9,808 or so selections will be other cards. Thus, when we say that the probability of drawing the king of hearts in one draw is 0.0192 , we are essentially applying our knowledge of what will happen over an infinite number of draws to a single draw. Like proportions, probabilities range from 0.00 (meaning that the event has absolutely no chance of occurrence) to 1.00 (a certainty). As the value of the probability increases, the likelihood that the defined event will occur also increases. A probability of 0.0192 is close to zero, and this means that the event (drawing the king of hearts) is unlikely or improbable. These techniques can be used to establish simple probabilities in any situation in which we can specify the number of successes and the total number of events. For example, a single die has six sides or faces, each with a different value ranging from one to six. The probability of getting any specific number (say, a four) in a single roll of a die is therefore 1 p (rolling a four) = = 0.1667 6 Further, if we list in a table the probability for each possible event, it is called a probability distribution. So, the probability distribution for rolling a single die is: Event 1 2 3 4 5 6 Probability 0.1667 0.1667 0.1667 0.1667 0.1667 0.16 Because all events are included in the distribution, the sum of the probabilities must equal 1.00 , within rounding error (e.g., 0.1667 + 0.1667 + 0.1667 + 0.1667 + 0.1667 + 0.1667 ≈ 1.00 ). Book Title: eTextbook: Statistics: A Tool for Social Research and Data Analysis, Fifth Canadian Edition 4.6. Using the Normal Curve to Estimate Probabilities Discrete vs. Continuous Probability Distributions Discrete vs. Continuous Probability Distributions At this point in our discussion of probability, it is important to distinguish between discrete and continuous variables because they require different methods to calculate probabilities. The die and deck-of-cards problems above are examples of discrete variables, and the list or table displaying the 142 probabilities associated with each event of a rolling a die or drawing a card is called a discrete probability distribution. Recall from Chapter 1 that nominal- or ordinal-level variables are considered discrete variables because they can only take on a finite number of distinct or discrete values, while interval-ratio- level variables are either discrete or continuous and can take on an infinite number of possible values. For instance, exam scores as a percentage, a continuous interval-ratio-level variable, can take on any value between 0 and 100 (e.g., 50.0 , 50.1 , 52.24 , 89.332 , 96.4242 …); however, the roll of a die with six sides can only take on one of six indivisible values—it can be one, two, three, four, five, or six but it cannot be in between these values. The die and deck-of-card illustrations show that calculating probabilities for discrete variables can be a straightforward mathematical process. (For interested readers, a supplement with a more detailed discussion of probability for discrete variables has been added to our website at www.cengage.com/healey5ce.) While the calculations for continuous variables are often more complex and require advanced mathematics, the underlying logic of probability is the same for discrete and continuous variables. What is more, as we will now see, it is often not necessary to do these calculations because published tables of some probabilities are readily available. Appendix A, for example, is just a list of probabilities (i.e., a probability distribution) for areas under the theoretical normal curve. So, whereas a discrete probability distribution describes the probability of occurrence of each event of a discrete variable like roll of a die, a continuous probability distribution like the normal distribution describes the probability of an area under the curve. That is, because of the infinite nature of continuous variables, probabilities are calculated for a range of values under the normal curve and not for a specific value. By combining our new understanding of probability (as the ratio of the number of successes to the number of possible events) with our knowledge of the normal curve, we can conveniently estimate the likelihood of selecting a case that has a score within a certain range for any continuous variable with a normal distribution. For example, suppose we wish to estimate the probability that a randomly chosen subject from the distribution of children’s IQ scores has an IQ score between 95 and the mean score of 100. Our definition of a success is the selection of any subject with a score in the specified range. Normally, we would next establish a fraction with the numerator equal to the number of subjects with scores in the defined range and the denominator equal to the total number of subjects. However, if the empirical distribution is normal in form, we can skip this step and avoid the intricate calculations and mathematics, since the probabilities, in proportion form, are already stated in Appendix A. To determine the probability that a randomly selected case has a score between 95 and the mean, we convert the original score to a Z score: ¯ Xi − X 95 − 100 5 Z= = =− = −0.25 s 20 20 143 Using Appendix A, we see that the area between this score and the mean is 0.0987. This is the probability we are seeking. The probability that a randomly selected case will have a score between 95 and 100 is 0.0987 (or, rounded off, 0.10 , or one out of ten). In the same fashion, the probability of selecting a subject from any range of scores can be estimated. Note that the techniques for estimating probabilities are the same as those for finding areas. The only new information introduced in this section is the idea that the areas in the standard normal curve table can also be thought of as probabilities. Consider an additional example: What is the probability that a randomly selected child has an IQ less than 123 ? We can find probabilities in the same way we found areas. The score (Xi) is above the mean, and, following the directions in Table 4.4, we find the probability we are seeking by adding the area in column (b) to 0.5000. First, we find the Z score: ¯ Xi − X 123 − 100 23 Z= = = = +1.15 s 20 20 Next, look in column (b) of Appendix A to find the area between this score and the mean. Then add the area (0.3749) to 0.5000. The probability of selecting a child with an IQ of less than 123 is 0.3749 + 0.5000 or 0.8749. Rounding this value to 0.88 , we can say that the probability is 0.88 (very high) that we will select a child with an IQ score in this range. Technically, remember, this probability expresses what would happen over the long run: for every 100 children selected from this group over an infinite number of trials, 88 would have IQ scores less than 123 and 12 would not. Let us close by stressing a very important point about probabilities and the normal curve. The probability is very high that any case randomly selected from a normal distribution will have a score close in value to that of the mean. The shape of the normal curve is such that most cases are clustered around the mean and decline in frequency as we move farther away—either to the right or to the left—from the mean value. In fact, given what we know about the normal curve, the probability that a randomly selected case will have a score within ±1 standard deviation of the mean is 0.6826. Rounding off, we can say that 68 out of 100 cases—or about two thirds of all cases—selected over the long run will have a score between −1 and +1 standard deviation or Z score from the mean. The probabilities are higher that any randomly selected case will have a score close in value to the mean. In contrast, the probability of the case having a score beyond three standard deviations from the mean is very small. Look in column (c) (“Area beyond Z”) for a Z score of 3.00 and you will find the value 0.0013. Adding the area in the upper tail (beyond +3.00 ) to the area in the lower tail (beyond −3.00 ) gives us 0.0013 + 0.0013 for a total of 0.0026. The probability of selecting a case with a very high score or a very low score is 0.0026. If we randomly selected cases from a normally distributed variable, we would select cases with Z scores beyond ±3.00 only 26 times out of every 10,000 trials. 144 Applying Statistics 4.3. Finding Probabilities The distribution of scores on a political science final exam used in Applying Statistics 4.2 had a mean of 72 and a standard deviation of 8. What is the probability that a student selected at random will have a score less than 61 ? More than 80 ? Less than 98 ? To answer these questions, we must first calculate Z scores and then consult Appendix A. We are looking for probabilities, so we will leave the areas in proportion form. The Z score for a score of 61 is ¯ Xi − X 61 − 72 −11 Z1 = = = = −1.37 s 8 8 This score is a negative value (below, or to the left of, the mean), and we are looking for the area below. Using Table 4.3 as a guide, we see that we must use column (c) to find the area below a negative score. This area is 0.0853. Rounding off, we can say that the probability of selecting a student with a score less than 61 is only 9 out of 100. This low value tells us this is an unlikely event. The Z score for the score of 80 is ¯ Xi − X 80 − 72 8 Z2 = = = = 1.00 s 8 8 The Z score is positive, and to find the area above (greater than) 80 , we look in column (c) (see Table 4.3). This value is 0.1587. The probability of selecting a student with a score greater than 80 is roughly 16 out of 100 , about twice as likely as selecting a student with a score of less than 61. The Z score for the score of 98 is ¯ Xi − X 98 − 72 26 Z1 = = = = 3.25 s 8 8 To find the area below a positive Z score, we add the area between the score and the mean (column (b)) to 0.5000 (see Table 4.3). This value is 0.4994 + 0.5000 , or 0.9994. It is extremely likely that a randomly selected student will have a score less than 98. Remember that scores more than ±3 standard deviations from the mean are very rare. The general point to remember is that cases with scores close to the mean are common and cases with scores far above or below the mean are rare. This relationship is central for an understanding of inferential statistics. (For practice in using the normal curve table to find probabilities, see Problems 4.12, 4.13, and 4.14 and 4.17.) One Step at a Time Finding Probabilities 1: Compute the Z score (or scores). Note whether the score is positive or negative. 2: Find the Z score (or scores) in column (a) of the standard normal curve table (Appendix A). 3: Find the area above or below the score (or between the scores), as you would normally (see the two previous One Step at a Time boxes in this chapter), and express the result as a proportion. Probabilities are expressed as a value between 0.00 and 1.00 and typically rounded to two digits beyond the decimal point. 145 Book Title: eTextbook: Statistics: A Tool for Social Research and Data Analysis, Fifth Canadian Edition Chapter 4. The Normal Curve Summary Summary 1. The normal curve, in combination with the mean and the standard deviation, can be used to construct precise descriptive statements about empirical distributions that are normally distributed. This chapter also lays some important groundwork for Part 2. 2. To work with the theoretical normal curve, raw scores must be transformed into their equivalent Z scores. Z scores allow us to find areas under the theoretical normal curve (Appendix A). 3. We considered three uses of the theoretical normal curve: finding total areas above and below a score, finding areas between two scores, and expressing these areas as probabilities. This last use of the normal curve is especially important because inferential statistics are centrally concerned with estimating the probabilities of defined events in a fashion very similar to the process introduced in Section 4.6. Summary of Formulas Z scores ¯ X1 − X Z= s Glossary Normal curve Probability Standard normal curve table Z scores Multimedia Resources Visit the companion website for the fifth Canadian edition of Statistics: A Tool for Social Research and Data Analysis to access a wide range of student resources: www.cengage.com/healey5ce. Problems 4.1 Scores on a quiz were normally distributed and have a mean of 10 and a standard deviation of 3. For each score below, find the Z score and the percentage of area above and below the score. Xi Z Score % Area % Area Above Below 5 6 7 8 9 11 12 14 15 16 18 146 SHOW ANSWER 4.2 Assume that the distribution of a graduate-school entrance exam is normal, with a mean of 500 and a standard deviation of 100. For each score below, find the equivalent Z score, the percentage of the area above the score, and the percentage of the area below the score. Xi Z Score % Area % Area Above Below 650 400 375 586 437 526 621 498 517 398 4.3 A class of final-year students at a university has been given a comprehensive examination to assess their educational experience. The mean on the test was 74 and the standard deviation was 10. What percentage of the students had scores a. between 75 and 85 ? b. between 80 and 85 ? c. above 80 ? d. above 83 ? e. between 80 and 70 ? f. between 75 and 70 ? g. below 75 ? h. below 77 ? i. below 80 ? j. below 85 ? SHOW ANSWER 4.4 For a normal distribution where the mean is 50 and the standard deviation is 10 , what percentage of the area is a. between the scores of 40 and 47 ? b. above a score of 47 ? c. below a score of 53 ? d. between the scores of 35 and 65 ? e. above a score of 72 ? f. below a score of 31 and above a score of 69 ? g. between the scores of 55 and 62 ? h. between the scores of 32 and 47 ? 4.5 GER A pension provider has hired a gerontologist to examine patterns in the demographic characteristics of its retirees. In their research on a sample (n) of 200 retirees, the gerontologist noted that the mean age at retirement was 72 and the standard deviation was 6. The following table presents the ages of 10 of the retirees when they retired. Convert each age to a Z score, and determine the number of people who retired at an older or a younger age than each of the 10 retirees listed here. (HINT: Determine the proportion of people in the usual way for each column below, multiply it by n, and round the result.) Xi Z Score Number of Number of Retirees Retirees Above Below 60 57 55 67 70 72 78 82 90 95 SHOW ANSWER 4.6 If a distribution of test scores is normal, with a mean of 78 and a standard deviation of 11 , what percentage of the area lies a. below 60 ? b. below 70 ? c. below 80 ? d. below 90 ? e. between 60 and 65 ? f. between 65 and 79 ? g. between 70 and 95 ? h. between 80 and 90 ? i. above 99 ? j. above 89 ? k. above 75 ? l. above 65 ? 4.7 A scale measuring ageism (age discrimination) has been administered to a large sample of human resources managers at major corporations. The distribution of scores is approximately normal, with a mean of 31 and a standard deviation of 5. What percentage of the sample had scores a. below 20 ? b. below 40 ? 147 c. between 30 and 40 ? d. between 35 and 45 ? e. above 25 ? f. above 35 ? SHOW ANSWER 4.8 At Matrix University, second-year co-op students are asked how many days they were required to work off-site during a four-month period. If the number of days is normally distributed, with a mean of 18 and a standard deviation of 3 , what is the raw score number of days of a student a. whose Z score is 3 ? b. whose Z score is 2 ? c. whose Z score is 1 ? 4.9 The average speed on a local residential street on a Monday afternoon was 59 km/h , and the standard deviation was 4. What is the probability that a randomly selected driver was going 1. between 55 and 65 ? 2. between 60 and 65 ? 3. above 65 ? 4. between 60 and 50 ? 5. between 55 and 50 ? SHOW ANSWER 4.10 On a career preparation aptitude test, a test writer’s score is at the 35 th percentile on mathematics and analytical reasoning. If the test scores are normally distributed, what is the test writer’s raw score if a. the mean is 1,000 and the standard deviation is 50 ? b. the mean is 100 and the standard deviation is 10 ? 4.11 On the same career preparation aptitude test as in Problem 4.10, a second test writer’s score is at the 50th percentile on mathematics and analytical reasoning. If the test scores are normally distributed, what is the second test writer’s raw score if a. the mean is 1,000 and the standard deviation is 50 ? b. the mean is 100 and the standard deviation is 10 ? SHOW ANSWER 4.12 The average burglary rate for a jurisdiction has been 311 per year with a standard deviation of 50. What is the probability that next year the number of burglaries will be a. less than 250 ? b. less than 300 ? c. more than 350 ? d. more than 400 ? e. between 250 and 350 ? f. between 300 and 350 ? g. between 350 and 375 ? 4.13 What is the probability of finding a score greater than 80 in a normal distribution of scores with a mean of 65 and a standard deviation of 15 ? SHOW ANSWER 4.14 On the scale mentioned in Problem 4.7, if a score of 40 or more is considered “highly discriminatory,” what is the probability that a human resources manager selected at random has a score in that range? 4.15 The local police force gives all applicants an entrance exam and accepts only those applicants who score in the top 15% on the exam. If the mean score this year is 87 and the standard deviation is 8 , will an individual with a score of 110 be accepted? SHOW ANSWER 4.16 After taking a city’s examinations for the positions of social worker and employment counsellor, you receive the following information on the tests and on your performance. On which of the tests did you do better? Social Worker Employment Counsellor ¯ ¯ X = 118 X = 27 s = 17 s=3 Your score = 127 Your score = 29 4.17 In a distribution of scores with a mean of 35 and a standard deviation of 4 , which event is more likely: that a randomly selected score is between 29 and 31 or that a randomly selected score is between 40 and 42 ? SHOW ANSWER 4.18 To be accepted into a university’s co-op education program, students must have GPAs in the top 10% of the school. If the mean GPA is 2.78 and the standard deviation is 0.33 , which of the following GPAs qualify? 3.20 , 3.21 , 3.25 , 3.30 , 3.35 You Are the Researcher Using SPSS to Produce Histograms and Compute Z Scores with the 2018 CCHS 148 The demonstrations and exercises below use the shortened version of the 2018 CCHS data set supplied with this textbook. Start SPSS, and open the CCHS_2018_Shortened.sav file. SPSS Demonstration 4.1 The Histogram Before we can compute and use Z scores and the standard normal curve table (Appendix A), we need to find out if a variable has a normal, bell-shaped curve. The histogram, discussed in Chapter 2, provides a convenient method to display the distribution of a variable. Here we will use the Histogram command to show the distribution of hwtdgcor (BMI or body mass index). (BMI is a measure of body fat based on a person’s weight and height. It is interpreted using a weight classification system as follows: less than 18.5 = underweight ; 18.5 − 24.9 = normal ; 25.0 − 29.9 = overweight ; and 30.0 and over = obese.) The SPSS procedure for producing a histogram is very similar to that for the bar chart illustrated in Demonstration 2.2. Click Graphs, Legacy Dialogs, and then Histogram. The Histogram dialog box will appear. Select hwtdgcor from the variable list on the left, and then click the arrow button at the top of the screen to move hwtdgcor to the Variable box. Click OK in the Histogram dialog box, and the histogram for hwtdgcor will be produced. 149 151 150 The distribution of hwtdgcor is approximately normal in shape. No empirical distribution is perfectly normal, but hwtdgcor is close enough to permit the assumption of normality. We can proceed to use the normal curve to convert the original scores of hwtdgcor into Z scores. SPSS Demonstration 4.2 Computing Z Scores The Descriptives command introduced in Demonstration 3.2 can also be used to compute Z scores for any variable. These Z scores are then available for further operations and may be used in other tasks. SPSS will create a new variable consisting of the transformed scores of the original variable. The program uses the letter Z with the letters of the variable name to designate the standardized scores of a variable. In this demonstration, we will have SPSS compute Z scores for hwtdgcor. First, click Analyze, Descriptive Statistics, and then Descriptives. Find hwtdgcor in the variable list, and click the arrow to move the variable to the Variable(s) box. Find the “Save standardized values as variables” option below the variable list, and click the checkbox next to it. With this option selected for Descriptives, SPSS will compute Z scores for all variables listed in the Variable(s) box. Click OK, and SPSS will produce the usual set of descriptive statistics for hwtdgcor. It will also add the new variable (called Zhwtdgcor), which contains the standardized scores for hwtdgcor, to the data set. To verify this, run the Descriptives command again and you will find Zhwtdgcor in the variable list. Transfer Zhwtdgcor to the Variable(s) box with hwtdgcor, and then unclick the checkbox next to “Save standardized values as variables.” Finally, click OK. The following output will be produced: Descriptive Statistics N Minimum Maximum Mean Std. Deviat BMI 1730 15.50 51.93 27.2597 5.0808 Z Score: 1730 −2.31450 4.85551.0000000 1.0000 BMI Valid N 1,716 (listwise) Like any set of Z scores, Zhwtdgcor has a mean of zero and a standard deviation of one. The new variable Zhwtdgcor can be treated just like any other variable and used in any SPSS procedure. If you would like to inspect the scores of Zhwtdgcor, use the Case Summaries procedure. Click Analyze, Reports, and then Case Summaries. Move both hwtdgcor and Zhwtdgcor to the Variable(s) box. Be sure the “Display cases” checkbox at the bottom of the window is selected. Find the “Limit cases to first” option. This option can be used to set the number of cases included in the output. By default, the system lists only the first 100 cases in your file. You can raise or lower the value for “Limit cases to first” n cases in your file or deselect this option to list all cases. For this exercise, let’s set a limit of 20 cases. Make sure the checkbox to the left of the option is checked, and type 20 in the textbox to the right. Click OK, and the following output will be produced : Scan the list of scores, and note that the scores that are closer in value to the mean of hwtdgcor (27.26) are closer to the mean of Zhwtdgcor (0.00) , and the farther away the score is from 27.26 , the greater the numerical value of the Z score. Also note that, of course, scores below the mean (less than 27.26 ) have negative signs, and scores above the mean (greater than 27.26 ) have positive signs. Exercises (using CCHS_2018_Shortened.sav) 4.1 Use the Histogram command to get a histogram of hwtdghtm (height in metres). How close is this curve to a smooth, bell-shaped normal curve? Write a sentence or two of interpretation for the graph. 4.2 Using Demonstration 4.2 as a guide, compute Z scores for hwtdghtm. Use the Case Summaries procedure to display the normalized and raw scores for each variable for 20 cases. Write a sentence or two summarizing these results. Cumulative Exercises Cumulative exercises provide practice in choosing, computing, and analyzing statistics. These online exercises present only data sets and research questions. You can choose appropriate statistics as part of the exercises. Cumulative exercises can be found on the student companion website at www.cengage.com/healey5ce. Book Title: eTextbook: Statistics: A Tool for Social Research and Data Analysis, Fifth Canadian Edition Chapter 4. The Normal Curve Summary of Formulas Summary of Formulas Z scores ¯ X1 − X Z= s