PSCI 2702 Chapter 2 - Statistics PDF
Document Details
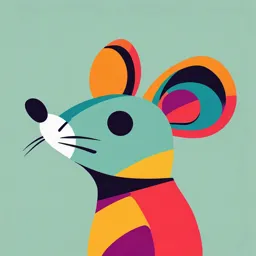
Uploaded by ConscientiousEvergreenForest1127
Toronto Metropolitan University
Tags
Summary
This chapter from an eTextbook introduces the concepts of percentages and proportions in social research and data analysis. It explains how to calculate percentages and proportions using formulas and provides examples.
Full Transcript
Book Title: eTextbook: Statistics: A Tool for Social Research and Data Analysis, Fifth Canadian Edition Chapter 2. Basic Descriptive Statistics: Percentages, Ratios and Rates, Tables, Charts, and Graphs 2.1. Percentages and Proportions 2.1. Percentages and Proportions Consider the follo...
Book Title: eTextbook: Statistics: A Tool for Social Research and Data Analysis, Fifth Canadian Edition Chapter 2. Basic Descriptive Statistics: Percentages, Ratios and Rates, Tables, Charts, and Graphs 2.1. Percentages and Proportions 2.1. Percentages and Proportions Consider the following statement: “Of the 113 survey respondents, 56 are female.” While there is nothing wrong with this statement, the same fact could have been more clearly conveyed if it had been reported as a percentage: “About 50% of survey respondents are female.” Percentages and proportions supply a frame of reference for reporting research results in the sense that they standardize the raw data: percentages to the base 100 and proportions to the base 1.00. The mathematical definitions of proportion and percentage are Formula 2.1 f Proportion (p) = n Formula 2.2 f Percentage(%) = ( ) × 100% n where f = frequency, or the number of cases in any category n = the number of cases in all categories To illustrate the computation of percentages, consider the data presented in Table 2.1. How can we find the percentage of males in the sample? Note that 42 there are 53 males (f = 53) and a total of 113 cases in all (n = 113). So, f 53 Percentage % = ( ) × 100% = ( ) × 100% = 0.4690 × 100% = 4 n 113 Table 2.1 Gender of Respondents Using the same procedures, we can find the percentage of females: f 56 Percentage % = ( ) × 100% = ( ) × 100% = 0.4956 × 100% = 4 n 113 and the percentage of other genders: f 4 Percentage % = ( ) × 100% = ( ) × 100% = 0.0354 × 100% = 3 n 113 All three results could have been expressed as proportions. For example, the proportion of females in Table 2.1 is 0.4956 : f 56 Proportion(p) = = = 0.4956 n 113 Percentages and proportions are easier to read and comprehend than frequencies. This advantage is particularly obvious when attempting to compare groups of different sizes. For example, Table 2.2 provides data on major fields of study from the 2019 Postsecondary Student Information System, an annual Statistics Canada survey of postsecondary students and institutions. Gender comparisons, however, are difficult because the total numbers of male (243,405) and female (319,935) students are very different. It is not evident which gender has the higher relative number of, say, majors in business, management, and public administration. Calculating percentages eliminates the difference in size of the two groups by standardizing both distributions to the base of 100. The same data are presented in percentages 43 in Table 2.3, making it easier to identify both differences and similarities 44 between the genders. We now see that a higher percentage of males (25.57%) than females (23.33%) study business, management, and public administration at Canadian postsecondary institutions, even though the absolute number of males (62,229) is considerably smaller than that of females (74,640). How would you describe the differences in the other major fields? (For practice in computing and interpreting percentages and proportions, see Problems 2.1 and 2.2.) Table 2.2 Major Field of Study for Female and Male Postsecondary Students Source: Statistics Canada. (2019). Postsecondary Student Information System. Table 2.3 Major Field of Study for Female and Male Postsecondary Students (Based on Table 2.2) Applying Statistics 2.1. Communicating with Statistics Not long ago, in a large social service agency, the following conversation took place between the executive director of the agency and a supervisor of one of the divisions: Executive director: Well, I don’t want to seem abrupt, but I’ve only got a few minutes. Tell me, as briefly as you can, about this staffing problem you claim to be having. Supervisor: Unfortunately, I just don’t have enough people to handle our workload. Of the 177 full-time employees of the agency, only 50 are in my division. Yet 6,231 of the 16,722 cases handled by the agency last year were handled by my division. Executive director (smothering a yawn): Very interesting. I’ll certainly get back to you on this matter. How could the supervisor have presented the case more effectively? Because the supervisor wants to compare two sets of numbers (the supervisor’s staff versus the total staff and the workload of the supervisor’s division versus the total workload of the agency), proportions or percentages would be a more forceful way of presenting results. What if the supervisor had said, “Just over 28% of the staff is assigned to my division, but we handle more than 37% of the total workload of the agency”? Is this a clearer message? The first percentage is found by f 50 %=( ) × 100% = × 100% n 177 = 0.2825 × 100% = 28.25% and the second percentage is found by f 6,231 %=( ) × 100% = ( ) × 100% n 16,722 = 0.3726 × 100% = 37.26% Here are some further guidelines on the use of percentages and proportions: 1. When working with a small number of cases (say, fewer than 20 ), it is usually preferable to report the actual frequencies rather than percentages or proportions. With a small number of cases, the percentages can change drastically with relatively minor changes in the data. For example, if you begin with a data set that includes 10 engineers and 10 sociologists (i.e., 50% of each of the two professions) and then add another sociologist, the percentage distributions will change noticeably to 52.38% sociologists and 47.62% engineers. Of course, as the number of observations increases, each additional case will have a smaller impact. If we started with 250 engineers and 250 sociologists and then added one more sociologist, the percentage of sociologists would change by only a tenth of a percent (from 50% to 50.1% ). 2. The first guideline leads to a follow-up, general best practice guideline about reporting results. Always report the number of observations along with proportions and percentages. This permits the reader to judge the adequacy of the sample size and, conversely, helps prevent the researcher from lying with statistics. Statements like “two out of three 45 people questioned prefer courses in statistics to any other course” might impress you, but the claim would lose its gloss if you learned that only three people were tested. You should be extremely suspicious of reports that fail to state the number of cases that were tested. 3. Percentages and proportions can be calculated for variables at the ordinal and nominal levels of measurement, even though they require division. This is not a violation of the level-of-measurement guideline (see Table 1.2). Percentages and proportions do not require the division of the scores of the variable (as would be the case in computing the average score on a test, for example) but rather the number of cases in a particular category (f) of the variable by the total number of cases in the sample (n). When we make a statement like “ 43% of the sample is female,” we are merely expressing the relative size of a category (female) of the variable (gender) in a convenient way. One Step at a Time Finding Percentages and Proportions 1: Determine the values for f (number of cases in a category) and n (number of cases in all categories). Remember that f will be the number of cases in a specific category and n will be the number of cases in all categories and that f will be smaller than n, except when the category and the entire group are the same. Therefore, proportions cannot exceed 1.00 , and percentages cannot exceed 100.00%. 2: For a proportion, divide f by n. 3: For a percentage, multiply the value you calculated in step 2 by 100%. Book Title: eTextbook: Statistics: A Tool for Social Research and Data Analysis, Fifth Canadian Edition 2.2. Ratios and Rates Ratios Ratios Ratios are especially useful for comparing categories of a variable in terms of relative frequency. Instead of standardizing the distribution of the variable to the base 100 or 1.00 , as we did in computing percentages and proportions, we determine ratios by dividing the frequency of one category by the frequency of another. Mathematically, a ratio can be defined as Formula 2.3 f1 Ratio = f2 where f1 = the number of cases in the first category f2 = the number of cases in the second category 46 To illustrate the use of ratios, we will use actual information from the 2019 Canadian Election Study, which is an inter-university project that regularly conducts a survey of Canadian voters on a variety of political issues. One of the survey questions asked, “Thinking about government spending, should the federal government spend more or about the same as now or less on crime and justice.” Of those polled, 1,442 people said that the federal government should spend more, while 1,890 said it should spend about the same as now or less. What is the relative size of these two groups? To find the ratio of those who think the government should spend more (f1) to those who think the government should spend about the same as now or less (f2) , divide 1,442 by 1,890 : f1 1,442 Ratio = = = 0.76 f2 1,890 The resultant ratio is 0.76 , which means that for every Canadian who thinks the government should spend about the same as now or less on crime and justice, there are 0.76 Canadians who think the government should spend more. Ratios can be very economical ways of expressing the relative predominance of two categories. In our example, it is obvious from the raw data that Canadians who think the government should spend more on crime and justice are outnumbered by Canadians who think the government should spend about the same as now or less. Percentages or proportions could have been used to summarize the overall distribution (e.g., “ 43.28% of Canadians think more should be spent on crime and justice, compared to 56.72% who think spending should remain the same as now or less”). In contrast to these other 47 methods, ratios express the relative size of the categories: they tell us exactly how much one category outnumbers (or is outnumbered by) the other. Applying Statistics 2.2. Ratios Indigenous Peoples are one of the fastest growing populations in Canada. One way to express this growth is to calculate the ratio of Indigenous (f1) to non-Indigenous (f2) persons at different points in time. For example, there were 1,172,785 Indigenous and 30,068,240 non-Indigenous persons in Canada in 2006, or a ratio of f1 1,172,785 = = 0.039 f2 30,068,240 By 2016, census data showed that there were 1,673,785 Indigenous and 32,786,285 non-Indigenous persons in the population. The ratio is f1 1,673,785 = = 0.051 f2 32,786,285 So, for every non-Indigenous person, there were 0.039 and 0.051 Indigenous persons in the population in 2006 and 2016 respectively. To eliminate the decimal points, we can multiply these ratios by 100 and report the values as 3.9 and 5.1 —for every 100 non-Indigenous persons in the population, the number of Indigenous persons grew from 3.9 to 5.1 over this 10-year period. Source: Data from Statistics Canada, 2006 and 2016 Census of Population. Ratios are often multiplied by some power of 10 to eliminate decimal points. For example, the ratio computed above might be multiplied by 100 and reported as 76 instead of 0.76. This would mean that, for every 100 Canadians who think the government should spend about the same as now or less on crime and justice, there are 76 Canadians who think more should be spent. To ensure clarity, the comparison units for the ratio are often expressed as well. Based on a unit of ones, the ratio of Canadians who think more should be spent to Canadians who think about the same as now or less should be spent would be expressed as 0.76:1. Based on hundreds, the same statistic might be expressed as 76:100. (For practice in computing and interpreting ratios, see Problems 2.1 and 2.2.) Book Title: eTextbook: Statistics: A Tool for Social Research and Data Analysis, Fifth Canadian Edition 2.2. Ratios and Rates Rates Rates Rates provide still another way of summarizing the distribution of a single variable. Rates are defined as the number of actual occurrences of some phenomenon divided by the number of possible occurrences per some unit of time. Formula 2.4 factual Rate = fpossible where factual = the number of actual occurrences of a phenomenon fpossible = the number of possible occurrences of the phenomenon Rates are usually multiplied by some power of 10 to eliminate decimal points. For example, the crude death rate for a population is defined as the number of 48 deaths in that population (actual occurrences) divided by the number of people in the population (possible occurrences) per year (the term “crude” is used because it is not adjusted for any underlying characteristics of the population such as the age or sex distribution). This quantity is then multiplied by 1,000. The formula for the crude death rate can be expressed as Number of deaths Crude death rate = × 1,000 Total population Applying Statistics 2.3. Rates In 2020, there were 374,885 births in Canada, within a population of 38,005,238. In 1972, when the population of Canada was only 22,218,463 , there were 351,256 births. Is the birth rate rising or falling? Although this question can be answered from the preceding information, the trend in birth rates is much more obvious if we compute birth rates for both years. Like crude death rates, crude birth rates are usually multiplied by 1,000 to eliminate decimal points. For 1972: 351,256 Crude birth rate = × 1,000 = 15.81 22,218,463 In 1972, there were 15.81 births for every 1,000 people in Canada. For 2020: 374,885 Crude birth rate = × 1,000 = 9.86 38,005,238 In 2020, there were 9.86 births for every 1,000 people in Canada. With the help of these statistics, the decline in the birth rate is clearly expressed. Source: Statistics Canada. Table 17-10-0008-0 Estimates of the components of demographic growth, annual. One Step at a Time Finding Ratios and Rates To Find Ratios 1: Determine the values for f1 and f2. The value for f1 is the number of cases in the first category, and the value for f2 is the number of cases in the second category. 2: Divide the value of f1 by the value of f2. 3: You may multiply the value you calculated in step 2 by some power of 10 when reporting results. To Find Rates 1: Determine the number of actual occurrences. This value is the numerator of the formula. 2: Determine the number of possible occurrences. This value is usually the total population for the area in question and is the denominator of the formula. 3: Divide the number of actual occurrences (step 1) by the number of possible occurrences (step 2). 4: Multiply the value you calculated in step 3 by some power of 10. Conventionally, birth rates and death rates are multiplied by 1,000 and crime rates are multiplied by 100,000. 5: Remember to state the period of time (e.g., per year) on which the rate is based. In 2020, a total of 296,373 deaths were registered in Canada. With a population of 38,005,238 , Canada’s crude death rate for that year was 296,373 Crude death rate = × 1,000 = 0.00779 × 1,000 = 7.80 38,005,238 Or, for every 1,000 Canadians, there were 7.8 deaths in 2020. Rates are often multiplied by 100,000 when the number of actual occurrences of some phenomenon is extremely small relative to the size of the population, such as homicides in Canada. Canadian police reported 743 homicides in 2020, so the homicide rate was 743 Homicide rate = × 100,000 = 0.0000195 × 100,000 = 1.95 38,005,238 Or, for every 100,000 Canadians, there were 1.95 homicides in 2020. (For practice in computing and interpreting rates, see Problems 2.3 and 2.4.) 49 Book Title: eTextbook: Statistics: A Tool for Social Research and Data Analysis, Fifth Canadian Edition Chapter 2. Basic Descriptive Statistics: Percentages, Ratios and Rates, Tables, Charts, and Graphs 2.3. Frequency Distributions: Introduction 2.3. Frequency Distributions: Introduction Table 2.1 is an example of what is formally called a frequency distribution. A frequency distribution is a table that summarizes the distribution of a variable’s values by reporting the number of cases contained in each category of the variable. It is a very helpful and commonly used way of organizing and working with data. In fact, the construction of a frequency distribution is almost always the first step in any statistical analysis. To illustrate the construction of frequency distributions and to provide some data for examples, let’s assume a hypothetical situation in which students who recently visited the health and counselling services centre at a university were sent an email asking them to complete a brief patient-satisfaction survey. Any realistic evaluation research would collect a variety of information from a large group of students, but for the sake of this example, we will confine our attention to just four variables and 20 students. The data are reported in Table 2.4. Table 2.4 Data from Health and Counselling Services Survey Student Gender Type of Satisfaction Age Health with Professional Services Seen A Male Medical 1 18 doctor B Male Counsellor 2 19 C Female Medical 4 18 doctor D Female Medical 2 19 doctor E Other Counsellor 1 20 F Male Medical 1 20 doctor G Female Counsellor 4 18 H Female Medical 3 21 doctor Student Gender Type of Satisfaction Age Health with Professional Services Seen I Male Medical 2 19 doctor J Other Other 3 23 K Female Medical 3 24 doctor L Male Counsellor 3 18 M Female Medical 1 22 doctor N Female Counsellor 4 26 O Male Medical 3 18 doctor P Male Counsellor 4 19 Q Female Counsellor 2 19 R Male Other 1 19 Student Gender Type of Satisfaction Age Health with Professional Services Seen S Female Other 4 21 T Male Medical 2 20 doctor Note that even though the data in Table 2.4 represent an unrealistically low number of cases, it is difficult to discern any patterns or trends. For example, try to ascertain the general level of satisfaction of the students from Table 2.4. You may be able to do so with just 20 cases, but it will take some time and 50 effort. Imagine the difficulty with 50 cases or 100 cases presented in this fashion. Clearly the data need to be organized in a format that allows the researcher (and their audience) to easily understand the distribution of the variable’s values. One general rule that applies to all frequency distributions is that the categories of the frequency distribution must be exhaustive and mutually exclusive. In other words, the categories must be stated in a way that permits each case to be counted in one and only one category. This basic principle applies to the construction of frequency distributions for variables measured at all three levels of measurement. Beyond this rule, there are only guidelines to help you construct useful frequency distributions. As you will see, the researcher has a fair amount of discretion in stating the categories of the frequency distribution (especially with variables measured at the interval-ratio level). We will identify the issues to consider as you make decisions about the nature of any particular frequency distribution. Ultimately, however, the guidelines we state are aids for decision making, nothing more than helpful suggestions. As always, the researcher has the final responsibility for making sensible decisions and presenting their data in a meaningful way. Book Title: eTextbook: Statistics: A Tool for Social Research and Data Analysis, Fifth Canadian Edition 2.4. Frequency Distributions for Variables Measured at the Nominal and Ordinal Levels 2.4. Frequency Distributions for Variables Measured at the Nominal and Ordinal Levels Nominal-Level Variables For nominal-level variables, construction of the frequency distribution is typically very straightforward. For each category of the variable being displayed, the occurrences are counted, and the subtotals, along with the total number of cases (n) , are reported. Table 2.5 displays a frequency distribution for the variable “gender” from the health and counselling services survey. For purposes of illustration, a column for tallies has been included in this table to illustrate how the cases are sorted into categories. (This column is not included 51 in the final form of the frequency distribution.) Take a moment to notice several other features of the table. Specifically, it has a descriptive title, clearly labelled categories (male, female, and other), and a report of the total number of cases at the bottom of the frequency column. These items must be included in all tables, regardless of the variable or level of measurement. Table 2.5 Gender of Respondents, Health and Counselling Services Survey The meaning of the table is quite clear. There are nine males, nine females, and two people of other genders in the sample, a fact that is much easier to comprehend from the frequency distribution than from the unorganized data presented in Table 2.4. For some nominal variables, the researcher might have to make some choices about the number of categories they wish to report. For example, the distribution of the variable “type of health professional seen” could be reported using the categories listed in Table 2.4. The resultant frequency distribution is presented in Table 2.6. Although this is a perfectly fine frequency distribution, it may be too detailed for some purposes. For example, the researcher might want to focus solely on “non–medical doctor” as distinct from “medical doctor” as the type of health professional seen by respondents during their visit to the health and counselling services centre. That is, the researcher might not be concerned with the difference between respondents who saw a “counsellor” and respondents who saw any “other” type of health professional but may want to treat both as simply “non–medical doctor.” In that case, these categories could be grouped together and treated as a single entity, as in Table 2.7. Notice that, when categories are collapsed like this, information and detail are lost. This latter version of the table does not allow 52 the researcher to discriminate between the two types of non–medical doctor health professionals. Table 2.6 Type of Health Professional Seen by Respondents, Health and Counselling Services Survey Table 2.7 Type of Health Professional Seen by Respondents, Health and Counselling Services Survey Book Title: eTextbook: Statistics: A Tool for Social Research and Data Analysis, Fifth Canadian Edition 2.4. Frequency Distributions for Variables Measured at the Nominal and Ordinal Levels Ordinal-Level Variables Ordinal-Level Variables Frequency distributions for ordinal-level variables are constructed following the same routines used for nominal-level variables. Table 2.8 reports the frequency distribution of the “satisfaction” variable from the health and counselling services survey. Note that a column of percentages by category has been added to this table. Such columns heighten the clarity of the table (especially with larger samples) and are common adjuncts to the basic frequency distribution for variables measured at all levels. Table 2.8 Satisfaction with Services, Health and Counselling Services Survey This table reports that students were neither satisfied nor dissatisfied with health and counselling services. Students were just as likely to be “satisfied” as “dissatisfied.” (For practice in constructing and interpreting frequency distributions for nominal- and ordinal-level variables, see Problem 2.5.) Book Title: eTextbook: Statistics: A Tool for Social Research and Data Analysis, Fifth Canadian Edition 2.5. Frequency Distributions for Variables Measured at the Interval-Ratio Level 2.5. Frequency Distributions for Variables Measured at the Interval-Ratio Level Basic Considerations In general, the construction of frequency distributions for variables measured at the interval-ratio-level is more complex than for nominal and ordinal variables. Interval-ratio variables usually have a large number of possible scores (i.e., a wide range from the lowest to the highest score). The large number of scores requires some collapsing or grouping of categories to produce reasonably compact frequency distributions. Note that a frequency distribution constructed from collapsed or grouped categories of interval-ratio variable values will closely resemble the frequency distribution of an ordinal variable. When we talk of collapsing or grouping interval-ratio variable values in order to create a frequency distribution, we are looking for a way to summarize the interval-ratio variable values in table form. In other words, we are not replacing the actual variable values with the grouped values, as would be the case with a level of measurement 53 transformation. To construct frequency distributions for interval-ratio-level variables, you must decide how many categories to use and how wide these categories should be. For example, suppose you wish to report the distribution of the variable “age” for a sample drawn from a community. Unlike the university data reported in Table 2.4, a community sample would have a very broad range of ages. If you simply reported the number of times that each year of age (or score) occurred, you could easily wind up with a frequency distribution that contained 70 , 80 , or even more categories. Such a large frequency distribution would not present a concise picture. The scores (years) must be grouped into larger categories to heighten clarity and ease of comprehension. How large should these categories be? How many categories should be included in the table? Although there are no hard-and-fast rules for making these decisions, they always involve a tradeoff between more detail (a greater number of narrow categories) and more compactness (a smaller number of wide categories). Book Title: eTextbook: Statistics: A Tool for Social Research and Data Analysis, Fifth Canadian Edition 2.5. Frequency Distributions for Variables Measured at the Interval-Ratio Level Constructing the Frequency Distribution Constructing the Frequency Distribution To introduce the mechanics and decision-making processes involved, we will construct a frequency distribution to display the ages of the students in the health and counselling services centre survey. Because of the narrow age range of a group of university students, we can use categories of only one year (these categories are often called intervals when working with interval-ratio data). The frequency distribution is constructed by listing the ages from youngest to oldest, counting the number of times each score (year of age) occurs, and then totalling the number of scores for each category. Table 2.9 presents the information and reveals a concentration or clustering of scores in the 18 and 19 intervals. Table 2.9 Age of Respondents, Health and Counselling Services Survey (interval width = one year of age) Even though the picture presented in this table is fairly clear, assume for the sake of illustration that you desire a more compact (less detailed) summary. To 54 do this, you have to group scores into wider intervals. By increasing the interval width (say to two years), you can reduce the number of intervals and achieve a more compact expression. The grouping of scores in Table 2.10 clearly emphasizes the relative predominance of younger respondents. This trend in the data can be stressed even more by the addition of a column displaying the percentage of cases in each category. Table 2.10 Age of Respondents, Health and Counselling Services Survey (interval width = two years of age) Note that the intervals in Table 2.10 are stated with an apparent gap between them (i.e., the intervals are separated by a distance of one unit). At first glance, these gaps may appear to violate the principle of exhaustiveness, but because age has been measured in whole numbers, the gaps actually pose no problem. Given the level of precision of the measurement (in years, as opposed to tenths or hundredths of a year), no case could have a score falling between these intervals. In fact, for these data, the set of intervals contained in Table 2.10 constitutes a scale that is exhaustive and mutually exclusive. Each of the 20 respondents in the sample can be sorted into one and only one age category. However, consider the difficulties that might have been encountered if age had been measured with greater precision. If age had been measured in tenths of a year, into which interval in Table 2.10 would a 19.4-year-old subject be placed? You can avoid this ambiguity by always stating the limits of the intervals at the same level of precision as the data. Thus, if age were being measured in tenths of a year, the limits of the intervals in Table 2.10 would be stated in tenths of a year. For example: 17.0–18.9 19.0–20.9 21.0–22.9 23.0–24.9 25.0–26.9 To maintain mutual exclusivity between categories, do not overlap the intervals. If you state the limits of the intervals at the same level of precision as the data (which might be in whole numbers, tenths, hundredths, etc.) and maintain a “gap” between intervals, you will always produce a frequency 55 distribution for which each case can be assigned to one and only one category. Book Title: eTextbook: Statistics: A Tool for Social Research and Data Analysis, Fifth Canadian Edition 2.5. Frequency Distributions for Variables Measured at the Interval-Ratio Level Midpoints Midpoints On occasion, you will need to work with the midpoints of the intervals, for example, when constructing or interpreting certain graphs such as the frequency polygon (see Section 2.6). Midpoints are defined as the points exactly halfway between the upper and lower limits and can be found for any interval by dividing the sum of the upper and lower limits by two. Table 2.11 displays midpoints for two different sets of intervals. (For practice in finding midpoints, see Problems 2.8b and 2.9b.) Table 2.11 Midpoints Interval Width = 3 Interval Midpoint 0–2 1.0 3–5 4.0 6–8 7.0 9–11 10.0 Interval Width = 6 Interval Midpoint 100–105 102.5 106–111 108.5 112–117 114.5 118–123 120.5 One Step at a Time Finding Midpoints 1: Find the upper and lower limits of the lowest interval in the frequency distribution. For any interval, the upper limit is the highest score included in the interval and the lower limit is the lowest score included in the interval. For example, for the top set of intervals in Table 2.11, the lowest interval (0–2) includes scores of 0 , 1 , and 2. The upper limit of this interval is 2 and the lower limit is 0. 2: Add the upper and lower limits and divide by 2. For the interval 0–2: (0 + 2)/2 = 1. The midpoint for this interval is 1. 3: Midpoints for other intervals can be found by repeating steps 1 and 2 for each interval. As an alternative, you can find the midpoint for any interval by adding the value of the interval width to the midpoint of the next lower interval. For example, the lowest interval in Table 2.11 is 0–2 and the midpoint is 1. Intervals are 3 units wide (i.e., they each include three scores), so the midpoint for the next higher interval (3–5) is 1 + 3 , or 4. The midpoint for the interval 6–8 is 4 + 3 , or 7 , and so forth. 56 Book Title: eTextbook: Statistics: A Tool for Social Research and Data Analysis, Fifth Canadian Edition 2.5. Frequency Distributions for Variables Measured at the Interval-Ratio Level Real Limits Real Limits For certain purposes, you must eliminate the “gap” between intervals and treat a distribution as a continuous series of categories that border each other. This is necessary, for example, in constructing some graphs, such as the histogram (see Section 2.6). To illustrate, let’s begin with Table 2.10. Note the “gap” of one year between intervals. As we saw before, the gap is only apparent: Scores are measured in whole years (i.e., 19 or 21 vs. 19.5 or 21.3 ) and cannot fall between intervals. These types of intervals are called stated limits, and they organize the scores of the variable into a series of discrete, non-overlapping intervals. To treat the variable as continuous, we must use the real limits. To find the real limits of any interval, divide the distance between the stated limits (the “gap”) in half, and then add the result to all upper stated limits and subtract it from all lower stated limits. This process is illustrated below with the intervals stated in Table 2.10. The distance between intervals is one, so the real limits can be found by adding 0.5 to all upper limits and subtracting 0.5 from all lower limits. Stated Limits Real Limits 18–19 17.5–19.5 20–21 19.5–21.5 22–23 21.5–23.5 24–25 23.5–25.5 26–27 25.5–27.5 Note that when conceptualized with real limits, the intervals overlap with each other, and the distribution can be seen as continuous. Table 2.12 presents additional illustrations of real limits for two different sets of intervals. In both cases, the “gap” between the stated limits is one. (For practice in finding real limits, see Problems 2.7c and 2.8d.) Table 2.12 Real Limits Stated Limits Real Limits 3–5 2.5–5.5 6–8 5.5–8.5 9–11 8.5–11.5 Stated Limits Real Limits 100–105 99.5–105.5 106–111 105.5–111.5 112–117 111.5–117.5 118–123 117.5–123.5 One Step at a Time Finding Real Limits 1: Find the distance (the “gap”) between the stated class intervals. In Table 2.10, for example, this value is 1. 2: Divide the value found in step 1 in half. 3: Add the value found in step 2 to all upper stated limits and subtract it from all lower stated limits. 57 Book Title: eTextbook: Statistics: A Tool for Social Research and Data Analysis, Fifth Canadian Edition 2.5. Frequency Distributions for Variables Measured at the Interval-Ratio Level Cumulative Frequency and Cumulative Percentage Cumulative Frequency and Cumulative Percentage Two commonly used adjuncts to the basic frequency distribution for interval- ratio-level and ordinal-level data are the cumulative frequency and cumulative percentage columns. Their primary purpose is to allow the researcher (and their audience) to tell at a glance how many cases fall below a given score or interval in the distribution. To construct a cumulative frequency column, begin with the lowest interval (i.e., the interval with the lowest scores) in the distribution. The entry in the cumulative frequency column for that interval is the same as the number of cases in the interval. For the next higher interval, the cumulative frequency is all cases in the interval plus all the cases in the first interval. For the third interval, the cumulative frequency is all cases in the interval plus all cases in the first two intervals. Continue adding (or accumulating) cases until you reach the highest interval, which has a cumulative frequency of all the cases in the interval plus all cases in all other intervals. For the highest interval, cumulative frequency equals the total number of cases. Table 2.13 shows a cumulative frequency column added to Table 2.10. Table 2.13 Age of Respondents, Health and Counselling Services Survey The cumulative percentage column is quite similar to the cumulative frequency column. Begin by adding a column for percentages to the basic frequency distribution as in Table 2.10. This column shows the percentage of all cases in each interval. To find cumulative percentages, follow the same addition pattern explained above for cumulative frequency. That is, the cumulative percentage for the lowest interval is the same as the percentage of 58 cases in the interval. For the next higher interval, the cumulative percentage is the percentage of cases in the interval plus the percentage of cases in the first interval, and so on. Table 2.14 shows the age data with a cumulative percentage column added. Table 2.14 Age of Respondents, Health and Counselling Services Survey These cumulative columns are quite useful in situations where the researcher wants to make a point about how cases are spread across the range of scores. For example, Tables 2.13 and 2.14 show quite clearly that most students in the health and counselling services survey are 21 years of age or younger. If the researcher wishes to impress this feature of the age distribution on their audience, then these cumulative columns are quite handy. Most realistic research situations are concerned with many more than 20 cases and/or many more categories than our tables have. Because the cumulative percentage column is clearer and easier to interpret in such cases, it is normally preferred to the cumulative frequencies column. One Step at a Time Adding Cumulative Frequency and Percentage Columns to Frequency Distributions To Add the Cumulative Frequency Column 1: Begin with the lowest interval (the interval with the lowest scores). The entry in the cumulative frequency column is the same as the number of cases in this interval. 2: Go to the next interval. The cumulative frequency for this interval is the number of cases in the interval plus the number of cases in the lower interval. 3: Continue adding (or accumulating) cases from interval to interval until you reach the interval with the highest scores, which has a cumulative frequency equal to n. To Add the Cumulative Percentage Column 1: Compute the percentage of cases in each category one at a time, and then follow the pattern for the cumulative frequencies. The entry for the lowest interval is the same as the percentage of cases in the interval. 2: For the next higher interval, the cumulative percentage is the percentage of cases in the interval plus the percentage of cases in the lower interval. 3: Continue adding (or accumulating) percentages from interval to interval until you reach the interval with the highest scores, which has a cumulative percentage of 100%. 59 Book Title: eTextbook: Statistics: A Tool for Social Research and Data Analysis, Fifth Canadian Edition 2.5. Frequency Distributions for Variables Measured at the Interval-Ratio Level Unequal Intervals Unequal Intervals As a general rule, the intervals of frequency distributions should be equal in size in order to maximize clarity and ease of comprehension. For example, note that all of the intervals in Tables 2.13 and 2.14 are the same width (two years). However, there are two other possibilities for stating intervals, and we will examine each situation separately. The first option is to use “open-ended” intervals. For instance, what would happen to the frequency distribution in Table 2.13 if we added one more student who was 47 years of age? We would now have 21 cases and there would be a large gap between the oldest respondent (now 47) and the second oldest (age 26). If we simply added the older student to the frequency distribution, we would have to include nine new intervals (28–29, 30–31, 32–33, etc.) with zero cases in them before we got to the 46–47 interval. This would waste space and probably be unclear and confusing. An alternative way to handle the situation where we have a few very high or low scores is to add an open-ended interval to the frequency distribution, as in Table 2.15. Table 2.15 Age of Respondents, Health and Counselling Services Survey (n = 21) The open-ended interval in Table 2.15 allows us to present the information more compactly than listing all of the empty intervals from “28–29” to “44–45.” We could handle an extremely low score by adding an open-ended interval as the lowest interval (e.g., “17 and younger”). There is a small price to pay for this efficiency, which is that there is no information in Table 2.15 about the exact scores included in the open-ended interval, so this technique should not be used indiscriminately. The second option for stating intervals is to use intervals of unequal size. On some variables, most scores are tightly clustered together, but others are strewn across a broad range of scores. Consider, as an example, the distribution of personal before-tax income in 2017, when most Canadians (52%) reported an income between $25,000 and $99,999 , and a sizable grouping (39%) earned less than that. The problem (from a statistical point of view) comes with more affluent individuals, those with incomes of $100,000 and above. The number of these individuals is typically quite small, of course, but we must still account for them. 60 If we tried to use a frequency distribution with equal intervals of, say, $10,000, we would need 30 or 40 or more intervals to include all of the more affluent individuals, and many of our intervals in the higher income ranges—especially those over $150,000—would have few or zero cases. In situations such as this, we can use intervals of unequal size to summarize the variable more efficiently, as in Table 2.16. Table 2.16 Distribution of Before-Tax Income by Individuals, Canada, 2017 Some of the intervals in Table 2.16 are $5,000 wide, others are $10,000 , $20,000 , or $25,000 wide, and two (the lowest and highest intervals) are open-ended. Tables that use intervals of mixed widths might be a little confusing for the reader, but the tradeoff in compactness and efficiency can be considerable. Book Title: eTextbook: Statistics: A Tool for Social Research and Data Analysis, Fifth Canadian Edition 2.5. Frequency Distributions for Variables Measured at the Interval-Ratio Level Procedures for Constructing Frequency Distributions for Interval-Ratio Variables Procedures for Constructing Frequency Distributions for Interval-Ratio Variables We covered a lot of ground in the preceding section, so let’s pause and review these principles by considering a specific research situation. Below are hypothetical data on the number of hours each student in an Introduction to Sociology course spent studying for the final exam (n = 105). 17 20 21 26 35 10 12 12 15 22 20 15 18 15 12 21 20 11 12 12 14 35 5 7 27 18 2 12 35 12 20 21 20 18 35 10 16 10 35 36 32 23 7 14 15 35 10 35 16 35 20 14 18 27 10 35 19 27 7 15 30 25 14 28 35 20 25 29 18 14 3 30 23 20 17 35 25 3 7 12 18 35 9 14 24 10 12 3 15 20 15 18 10 6 25 20 34 18 3 15 20 33 14 7 30 61 Listed in this format, the data are a hopeless jumble from which no one could derive much meaning. The function of the frequency distribution is to arrange and organize these data so that their meanings will be made obvious. First, we must decide how many intervals to use in the frequency distribution. Following the guidelines presented in the One Step at a Time: Constructing Frequency Distributions for Interval-Ratio Variables box, let’s use about 10 intervals (k = 10). By inspecting the data, we can see that the lowest score is 2 and the highest is 36. The range of these scores (R) is 36 −2 , or 34. To find the approximate interval size (i) , divide the range (34) by the number of intervals (10). Since 34/10 = 3.4 , we can set the interval size at 3. The lowest score is 2 , so the lowest interval is 2–4. The highest interval is 35–37 , which will include the high score of 36. All that remains is to state the intervals in table format, count the number of scores that fall in each interval, and report the totals in a frequency column. These steps have been taken in Table 2.17, which also includes columns for the percentages and cumulative percentages. Note that this table is the product of several relatively arbitrary decisions. The researcher should remain aware of this fact and inspect the frequency distribution carefully. If the table is unsatisfactory for any reason, it can be reconstructed with a different number of categories and interval sizes. Table 2.17 Number of Hours Studied for Final Exam in Introduction to Sociology Course Now, with the aid of the frequency distribution, some patterns in the data can be discerned. There are three distinct clusterings of scores in the table. The 62 single largest interval, with 17 cases, is 14–16. Nearly as many students, 15 cases, spent between 20 and 22 hours studying for the final exam. Combined with the interval between them ( 17–19 hours ), this represents quite a sizable grouping of cases ( 43 out of 105 , or 40.95% of all cases). The third grouping is in the 35–37 interval with 13 cases, showing that a rather large percentage of students (12.38%) spent relatively many hours studying for the final exam. The cumulative percentage column indicates that the majority of the students (55.24%) spent less than 20 hours studying for the exam. (For practice in constructing and interpreting frequency distributions for interval- ratio-level variables, see Problems 2.5 to 2.9 and 2.11.) 63 One Step at a Time Constructing Frequency Distributions for Interval-Ratio Variables 1: Decide how many intervals (k) you wish to use. One reasonable convention suggests that the number of intervals should be about 10. Many research situations may require fewer than 10 intervals (k < 10) , and it is common to find frequency distributions with as many as 15 intervals. Only rarely will more than 15 intervals be used, since the resultant frequency distribution would be too large for easy comprehension. 2: Find the range (R) of the scores by subtracting the low score from the high score. 3: Find the size of the intervals (i) by dividing R (from step 2) by k (from step 1): i = R/k Round the value of i to a convenient whole number. This is the interval size or width. 4: State the lowest interval so that its lower limit is equal to or below the lowest score. By the same token, your highest interval is the one that contains the highest score. Generally, intervals should be equal in size, but unequal and openended intervals may be used when convenient. 5: State the limits of the intervals at the same level of precision as you have used to measure the data. Do not overlap intervals. You will thereby define the intervals so that each case can be sorted into one and only one category. 6: Count the number of cases in each interval, and report these subtotals in a column labelled “Frequency.” Report the total number of cases (n) at the bottom of this column. The table may also include a column for percentages, cumulative frequencies, and cumulative percentages. Book Title: eTextbook: Statistics: A Tool for Social Research and Data Analysis, Fifth Canadian Edition 2.6. Charts and Graphs 2.6. Charts and Graphs Researchers frequently use charts and graphs to present their data in ways that are visually more dramatic than frequency distributions. These devices are particularly useful for conveying an impression of the overall shape of a distribution and for highlighting any clustering of cases in a particular range of scores. Many graphing techniques are available, but we will examine just five. The first two, pie and bar charts, are appropriate for discrete variables at any level of measurement. The next two, histograms and frequency polygons, are used with both discrete and continuous interval-ratio variables but are particularly appropriate for the latter. The fifth, boxplots, examined in Chapter 3, are also appropriate for both discrete and continuous interval-ratio variables. The sections that follow explain how to construct graphs and charts by hand. These days, however, computer programs are almost always used to produce graphic displays. Graphing software is sophisticated and flexible but also relatively easy to use, and, if such programs are available to you, you should familiarize yourself with them. The effort required to learn these programs will be repaid in the quality of the final product. SPSS Demonstration 2.2 at the end of this chapter shows how to produce bar charts. Book Title: eTextbook: Statistics: A Tool for Social Research and Data Analysis, Fifth Canadian Edition 2.6. Charts and Graphs Pie Charts Pie Charts To construct a pie chart, begin by computing the percentage of all cases that fall in each category of the variable. Then divide a circle (the pie) into segments (slices) proportional to the percentage distribution. Be sure that the chart and all segments are clearly labelled. Figure 2.1 is a pie chart that displays the distribution of the “type of health professional seen” variable from the health and counselling services survey. The frequency distribution (see Table 2.6) is reproduced as Table 2.18, with a 64 column added for the percentage distribution. Because a circle’s circumference is 360° , we will apportion 180° (or 50% ) for the first category, 126° (35%) for the second, and 54° (15%) for the last. The pie chart visually reinforces the relative preponderance of respondents who saw a medical doctor and the relative absence of respondents who saw other types of health professionals in the health and counselling services survey. Figure 2.1 Pie Chart: Type of Health Professional Seen by Respondents, Health and Counselling Services Survey Table 2.18 Type of Health Professional Seen by Respondents, Health and Counselling Services Survey Book Title: eTextbook: Statistics: A Tool for Social Research and Data Analysis, Fifth Canadian Edition 2.6. Charts and Graphs Bar Charts Bar Charts Like pie charts, bar charts are relatively straightforward. Conventionally, the categories of the variable are arrayed along the horizontal axis (or abscissa), and frequencies, or percentages if you prefer, are arranged along the vertical axis (or ordinate). For each category of the variable, construct (or draw) a rectangle of constant width and with a height that corresponds to the number of cases in the category. The bar chart in Figure 2.2 reproduces the data for the “type of health professional seen” variable from Figure 2.1 and Table 2.18. Figure 2.2 Bar Chart: Type of Health Professional Seen by Respondents, Health and Counselling Services Survey (n = 20) This chart is interpreted in exactly the same way as the pie chart in Figure 2.1, and researchers are free to choose between these two methods of displaying data. However, if a variable has more than four or five categories, the bar chart is preferred. With too many categories, the pie chart gets very crowded and loses its visual clarity. 65 Bar charts are also particularly effective ways to display the relative frequencies for two or more categories of a variable when you want to emphasize some comparisons. Suppose, for example, that you wished to compare males, females, and people of other genders on satisfaction with health and counselling services. Figure 2.3 displays these data, derived from Table 2.4, in an easily comprehensible way. The bar chart shows that females are most likely to be very satisfied with services and males are most likely to be very dissatisfied with services. Figure 2.3 also shows the special usefulness of bar charts for ordinal variables since the placement of the variable values on the abscissa preserves the rank order of an ordinal variable’s values. (For practice in constructing and interpreting pie and bar charts, see Problems 2.5b and 2.10.) Figure 2.3 Satisfaction with Services by Gender, Health and Counselling Services Survey (n = 20) Book Title: eTextbook: Statistics: A Tool for Social Research and Data Analysis, Fifth Canadian Edition 2.6. Charts and Graphs Histograms Histograms Histograms look a lot like bar charts and, in fact, are constructed in much the same way. However, histograms use real limits rather than stated limits; also, the categories or scores of the variables are contiguous, meaning that they border each other, as if they merged into each other in a continuous series. Therefore, these graphs are most appropriate for continuous interval-ratio- level variables, although they are commonly used for discrete interval-ratio- level variables as well. To construct a histogram from a frequency distribution, follow these steps: 1. Array the real limits of the intervals or scores along the horizontal axis (abscissa). 2. Array frequencies along the vertical axis (ordinate). 3. For each category in the frequency distribution, construct a bar with height corresponding to the number of cases in the category and with width corresponding to the real limits of the intervals. 4. Label each axis of the graph. 5. Title the graph. 66 As an example, Table 2.19 presents the real limits, along with midpoints, of the intervals for the frequency distribution shown in Table 2.17. This information was used to construct a histogram of the distribution of study hours, as presented in Figure 2.4. Note that the edges of each bar correspond to the real limits and that the middle of each bar is directly over the midpoint of the interval. Overall, the histogram visually reinforces the relative concentration of students in the middle of the distribution, as well as the relatively large grouping of students who spent between 35 and 37 hours studying for the final exam in the Introduction to Sociology course. 67 Table 2.19 Real Limits and Midpoints of Intervals for Frequency Distribution Shown in Table 2.17 Figure 2.4 Histogram of Study Hours for Final Exam in Introduction to Sociology Course (n = 105) Book Title: eTextbook: Statistics: A Tool for Social Research and Data Analysis, Fifth Canadian Edition Chapter 2. Basic Descriptive Statistics: Percentages, Ratios and Rates, Tables, Charts, and Graphs Summary Summary 1. We considered several different ways of summarizing the distribution of a single variable and, more generally, reporting the results of our research. Our emphasis throughout was on the need to communicate our results clearly and concisely. You will often find that, as you strive to communicate statistical information to others, the meanings of the information will become clearer to you as well. 2. Percentages, proportions, ratios, and rates represent several different techniques for enhancing clarity by expressing results in terms of relative frequency. Percentages and proportions report the relative occurrence of some category of a variable compared with the distribution as a whole. Ratios compare two categories with each other, and rates report the actual occurrences of some phenomenon compared with the number of possible occurrences per some unit of time. 3. Frequency distributions are tables that summarize the entire distribution of some variable. It is very common to construct these tables for each variable of interest as the first step in a statistical analysis. Columns for percentages, cumulative frequencies, and/or cumulative percentages often enhance the readability of frequency distributions. 4. Pie and bar charts, histograms, and frequency polygons are graphic devices used to express the basic information contained in the frequency distribution in a compact and visually dramatic way. Summary of Formulas Proportion p= f n Percentage %=( f ) × 100% n Ratio Ratio = f1 f2 Rate Rate = factual fpossible Glossary Bar charts Cumulative frequency Cumulative percentage Frequency distribution Frequency polygon Histograms Intervals Midpoints Percentage Pie chart Proportion Rates Ratios Real limits Stated limits Multimedia Resources 70 Visit the companion website for the fifth Canadian edition of Statistics: A Tool for Social Research and Data Analysis to access a wide range of student resources: www.cengage.com/healey5ce. Problems 2.1 SOC The tables that follow report the marital status of 20 respondents in two different apartment complexes. (HINT: Make sure you have the correct numbers in the numerator and denominator before solving the following problems. For example, Problem 2.1a asks for “the percentage of the respondents who are legally married in each complex”; the denominators are 20 for these two fractions. Problem 2.1d, however, asks for “the percentage of the single respondents who live in Complex B”; the denominator for this fraction is 4 + 6 , or 10.) Status Complex A Complex B Legally married 5 10 Common-law 8 2 Single 4 6 Separated 2 1 Widowed 0 1 Divorced 1 0 20 20 a. What percentage of the respondents in each complex are legally married? SHOW ANSWER b. What is the ratio of single to legally married respondents at each complex? SHOW ANSWER c. What proportion of each sample are widowed? SHOW ANSWER d. What percentage of the single respondents live in Complex B? SHOW ANSWER e. What is the ratio of not legally married to legally married persons at each complex? SHOW ANSWER 2.2 SOC At Algebra University, the genders of students in the various major fields of study are as follows: Major Male Female Other Total Humanities 114 83 3 200 Social sciences 97 130 2 229 Natural 71 20 1 92 sciences Business 156 139 0 295 Nursing 3 32 3 38 Education 25 15 5 45 Total 466 419 14 899 71 Read each of the following problems carefully before constructing the fraction and solving for the answer. (HINT: Be sure you place the proper number in the denominator of the fractions. For example, some problems use the total number of students as the denominator, but others use the total number of majors.) a. What percentage of social science majors are male? b. What proportion of business majors are female? c. For the humanities, what is the ratio of males to females? d. What percentage of the total student body are males? e. What is the ratio of people with other genders to females for the entire sample? f. What proportion of the nursing majors are male? g. What percentage of the sample are social science majors? h. What is the ratio of humanities majors to business majors? i. What is the ratio of female business majors to female nursing majors? j. What proportion of the males are education majors? 2.3 CJ A city in Ontario had a population of 211,732 and experienced 47 bank robberies, 13 murders , and 23 auto thefts during a recent year. Compute a rate for each type of crime per 100,000 population. (HINT: Make sure you set up the fraction with size of population in the denominator.) SHOW ANSWER 2.4 CJ The numbers of homicides in five US states and five Canadian provinces for the years 1997 and 2019 are as follows: 1997 2012 State/Province Homicides Population Homicides Popu New Jersey 338 8,053,000 287 8,88 Iowa 52 2,852,000 80 3,15 Alabama 426 4,139,000 587 4,90 Texas 1,327 19,439,000 1,694 28,62 California 2,579 32,268,000 1,794 39,51 Nova Scotia 24 936,100 6 96 Quebec 132 7,323,600 77 8,50 Ontario 178 11,387,400 246 14,54 Manitoba 31 1,137,900 72 1,36 British 116 3,997,100 90 5,09 Columbia Source: Centers for Disease Control and Prevention, US Census Bureau, and Statistics Canada. Calculate the homicide rate per 100,000 population for each state and each province for each year. Relatively speaking, which state and which province had the highest homicide rates in each year? Which country seems to have the higher homicide rate? Write a paragraph describing these results. 72 2.5 SOC The scores of 15 respondents on four variables are reported below. The numerical codes for the variables are as follows: Gender Stricter Gun Level of Education Laws 1 = Male 1 = In favor 0 = Less than high school 2 = Female 2 = Opposed 1 = High school 3 = Other 2 = Community college 3 = Bachelor′s 4 = Graduate Case Gender Support Level of Age Number for Gun Education Control 1 2 1 1 45 2 1 2 1 48 3 2 1 3 55 4 1 1 2 32 5 2 1 3 33 6 1 1 1 28 7 2 2 0 77 8 1 1 1 50 9 1 2 0 43 10 2 1 1 48 11 1 1 4 33 12 1 1 4 35 Case Gender Support Level of Age Number for Gun Education Control 13 1 1 0 39 14 2 1 1 25 15 1 1 1 23 a. Construct a frequency distribution for each variable. Include a column for percentages. b. Construct pie and bar charts to display the distributions of gender, support for stricter gun laws, and level of education. SHOW ANSWER 2.6 SOC Concerned with the growing number of unemployed young adults in the community, a local employment service agency developed an innovative education and training program aimed at improving the employability of this group. A sample of 15 unemployed youth from the community participated and completed the program. To measure the success of the program, each participant was asked about the number of job interviews they had in the six-month period before the program (pretest) and six-month period after the program (post-test). The numbers are as follows: Case Pretest Post-Test A 8 12 B 7 13 C 10 12 D 15 19 E 10 8 F 10 17 G 3 12 H 10 11 I 5 7 J 15 12 K 13 20 L 4 5 M 10 15 Case Pretest Post-Test N 8 11 O 12 20 Construct frequency distributions for the pretest and post-test numbers. Include a column for percentages. (HINT: The maximum range for these scores is 20. If you use 10 intervals to display these scores, the interval size is 2. Because there are no scores of 0 or 1 for either test, you may state the first interval as 2–3. To make comparisons easier, both frequency distributions should have the same intervals.) 2.7 SOC Sixteen students in their final year of undergraduate studies completed a class to prepare them for the GRE (Graduate Record Examination). Their scores on the GRE are reported below. 420 345 560 650 459 499 500 657 467 480 505 555 480 520 530 589 These same 16 students were given a test of math and verbal ability to measure their readiness for graduate-level work. The following scores are reported in terms of the percentage of correct answers for each test. Math Test 67 45 68 70 72 85 90 99 50 73 77 78 52 66 89 75 73 Verbal Test 89 90 78 77 75 70 56 60 77 78 80 92 98 72 77 82 a. Display each of these variables in a frequency distribution with columns for percentages and cumulative percentages. b. Construct a histogram and a frequency polygon for these data. c. Find the upper and lower real limits for the intervals you established. 2.8 GER The numbers of times 25 residents of a community for older adults left their homes for any reason during the past week are displayed below. 0 2 1 7 3 7 0 2 3 17 14 15 5 0 7 5 21 4 7 6 2 0 10 5 7 a. Construct a frequency distribution to display these data. b. What are the midpoints of the intervals? c. Add columns to the table to display the percentage distribution, cumulative frequency, and cumulative percentage. d. Find the real limits for the intervals you selected. e. Construct a histogram and a frequency polygon to display these data. f. Write a paragraph summarizing this distribution of scores. 2.9 SOC Twenty-five students completed a questionnaire that measured their attitudes toward interpersonal violence. Respondents who scored high believed that in many situations a person could legitimately use physical force against another person. Respondents who scored low believed that in no situation (or very few situations) could the use of violence be justified. 52 47 17 8 92 53 23 28 9 90 17 63 17 17 23 19 66 10 20 47 20 66 5 25 17 a. Construct a frequency distribution to display these data. b. What are the midpoints of the intervals? c. Add columns to the table to display the percentage distribution, cumulative frequency, and cumulative percentage. d. Construct a histogram and a frequency polygon to display these data. e. Write a paragraph summarizing this distribution of scores. SHOW ANSWER 2.10 PA/CJ As part of an evaluation of the efficiency of your local police force, you have gathered the following data on police response time to calls for assistance during two different years. (Response times were rounded off to whole minutes.) Convert both frequency distributions into percentages, and construct pie charts and bar charts to display the data. Write a paragraph comparing the changes in response time between the two years. Response Time, 2000 Frequency (f) 21 minutes or more 35 16–20 minutes 75 11–15 minutes 180 6–10 minutes 375 Less than 6 minutes 275 940 Response Time, 2020 Frequency (f) 21 minutes or more 45 16–20 minutes 95 11–15 minutes 155 6–10 minutes 350 Less than 6 minutes 250 895 2.11 In a survey conducted by a telecommunications company, 15 people age 40 and under and 16 people over age 40 were asked how many years they have been in their existing mobile phone plan. Their answers are reported below. Display each of these variables in a frequency distribution with columns for percentages and cumulative percentages. 40 and under 6 5 6 6 2 5 2 3 5 3 1 4 5 6 1 Over 40 4 1 3 7 7 2 3 8 7 2 4 2 5 2 8 8 74 SHOW ANSWER You Are the Researcher Using SPSS to Produce Frequency Distributions and Graphs with the 2018 GSS The demonstrations and exercises below use the shortened version of the 2018 GSS data set supplied with this textbook. Click the SPSS icon to start SPSS. Load the 2018 GSS by clicking the file name (2018_GSS_Shortened.sav) on the first screen, or by clicking File, Open, and Data in the SPSS Data Editor window. In the Open Data dialog box, you may have to change the drive specification to locate the 2018 GSS data. Double-click the file name (2018_GSS_Shortened.sav) to open the data set. You are ready to proceed when you see the message “IBM SPSS Statistics Processor is ready” on the status bar at the bottom of the SPSS Data Editor window, as shown in Figure 2.6. 75 Figure 2.6 SPSS Data Editor Window: Data View The SPSS Data Editor window can actually be viewed in one of two modes. The Data View mode (Figure 2.6), which is the default mode when you start SPSS, displays the data in the data set. Each row represents a particular case and each column a particular variable. By contrast, the Variable View mode (Figure 2.7) shows the variables in that data set, where each row represents a particular variable and each column a particular piece of information (e.g., name, label) about the variable. When analyzing variables, be careful not to mix up the variable name and the variable label, or your analysis might not be performed by SPSS. To change from one mode to the other, click the appropriate tab located at the bottom of the SPSS Data Editor window. Figure 2.7 SPSS Data Editor Window: Variable View Before we begin our demonstrations, it is important to note that SPSS provides the user with a variety of options for displaying information about the data file and output on the screen. We highly recommend that you tell SPSS to display lists of variables by name (e.g., agegr10) rather than labels (e.g., age group of respondent (groups of 10)). Lists displayed in this way will be easier to read and to compare to the GSS and CCHS codebooks in Appendix G. To do this, click Edit on the main menu bar at the top of the SPSS Data Editor window, and then click Options from the drop-down submenu. A dialog box labelled Options will appear with a series of tabs along the top. The General options should be displayed, but, if not, click on the General tab. On the General screen, find the box labelled Variable Lists; if they are not already selected, click Display names, then Alphabetical, and then OK. If you make changes, a message may appear on the screen that tells you that changes will reset all dialog box settings and close all open dialog boxes. Click OK. 76 SPSS DEMONSTRATION 2.1 Frequency Distributions In this demonstration, we will use the Frequencies procedure to produce a frequency distribution for the variable marstat (marital status). From the menu bar on the SPSS Data Editor window, click Analyze. From the menu that drops down, click Descriptive Statistics and then Frequencies. The Frequencies dialog box appears, with the variables listed in alphabetical order in the left-hand box. Find marstat in the left-hand box by using the slider button or the arrow keys on the right-hand border to scroll through the variable list. As an alternative, type “m,” and the cursor will move to the first variable name in the list that begins with the letter “m.” In this case, the variable is marstat, the variable that we are interested in. Once marstat is highlighted, click the arrow button in the centre of the screen to move the variable name to the Variable(s) box. The variable name marstat should now appear in the box. Click the OK button at the bottom of the dialog box, and SPSS will create the frequency distribution you requested. The output table (frequency distribution) will be in the SPSS Output, or Viewer, window, which will now be “closest” to you on the screen. As illustrated in Figure 2.8, the output table, along with other information, is in the right-hand box of the window, while the left-hand box contains an “outline” log of the entire output. To change the size of the SPSS Output window, click on the Maximize button, the middle symbol (shaped like either a square or two intersecting squares) in the upper right corner of the window. The actual output can also be edited by double-clicking on any part of the table. (See Appendix F.6 for more information on editing output.) Let’s briefly examine the elements of the output table in Figure 2.8. The variable description, or label, is printed at the top of the output (“Marital status of the respondent”). The various categories are printed on the left. Moving one 77 column to the right, we find the actual frequencies, or the number of times each score of the variable occurs. We see that 768 of the respondents were married, 156 had common-law status, and so forth. Figure 2.8 SPSS Viewer (Output) Window Next are two columns that report percentages. The entries in the “Percent” column are based on all respondents in the sample. In this case, the denominator includes any respondents, even the ones coded as missing. The “Valid Percent” column eliminates all cases with missing values. When there are missing cases, we should focus on the “Valid Percent” column because it ignores missing values—the denominator includes only respondents with non- missing values. The final column is a “Cumulative Percentage” column. For nominal-level variables like marstat, this information is not meaningful because the order in which the categories are stated is arbitrary. The output table in the SPSS Output window can be printed or saved by selecting the appropriate command from the File menu. You can also transfer the table to a word processor document: Right-click on any part of the table, choose Copy, right-click on the spot in the word processor document where you want to place the table, and choose Paste. Appendix F.7 provides more information on these topics. As a final note, the total number of cases in the GSS data set is 1,500. This can be verified by scrolling to the bottom of the SPSS Data Editor window, where you will find a total of 1,500 rows. However, the “total” number of cases in the output table above is 1,575. The reason for the difference is that the GSS data set is weighted to correct for sampling bias. Like many social surveys, the GSS under- and over-samples various groups of individuals—some individuals are more likely than others to be included in the sample. The weight variable included with the GSS, wght_per, corrects for this bias. Once you open the 2018_GSS_Shortened.sav file, the weight variable is automatically turned on, as confirmed by the message “Weight On” on the status bar at the bottom of the SPSS Data Editor window. So, you are really analyzing 1,575, not 1,500, individuals when you are using this data file. (See Appendix G.5 for more information on this topic.) SPSS DEMONSTRATION 2.2 Graphs and Charts SPSS can produce a variety of graphs and charts, and we will use the program to produce a bar chart in this demonstration. To conserve space, we will keep the choices as simple as possible, but you should explore the options for yourself. For any questions you might have that are not answered in this demonstration, click Help on the main menu bar. To produce a bar chart, first click Graphs on the main menu bar, then Legacy Dialogs, and then Bar. The Bar Charts dialog box will appear, with three choices for the type of graph we want. The Simple option is already highlighted, and this is the one we want. Make sure that Summaries for groups of cases in the Data in Chart Are box is selected, and then click Define at the bottom of the dialog box. The Define Simple Bar dialog box will appear with variable names listed on the left. Choose dh1ged (education—highest degree) from the variable list by moving the cursor to highlight this variable name. Click the arrow button in the middle of the screen to move dh1ged to the Category Axis text box. Note that the Bars Represent box is above the Category Axis box. The options in this box give you control over the vertical axis of the graph, which can be 78 calibrated in frequencies, percentages, or cumulative frequencies or percentages. Let’s choose N of cases (frequencies), the option that is already selected. Click OK in the Define Simple Bar dialog box, and the following bar chart will be produced. (Note that, to save space, only the output graph, and not the whole SPSS Output window, is shown (see Figure 2.9), and it has been slightly edited for clarity and will not exactly match the output on your screen.) Figure 2.9 Output Window* *This output has been slightly edited for clarity and will not exactly match the output on your screen. The bar chart reveals that the most common level of education for this sample is “Post-secondary Diploma.” The least common level is “Less than High School.” Don’t forget to Save or Print the chart if you wish. EXERCISES (using 2018_GSS_Shortened.sav) 2.1 Make frequency distributions for five nominal or ordinal variables in the GSS data set. Write a sentence or two summarizing each frequency distribution. Your description should clearly identify the most and least common scores and any other noteworthy patterns you observe. 2.2 Make a bar chart for lrcc20 (length of time in city or local community) and hsdsizec (household size). Write a sentence or two of interpretation for each chart. 79 Book Title: eTextbook: Statistics: A Tool for Social Research and Data Analysis, Fifth Canadian Edition Chapter 2. Basic Descriptive Statistics: Percentages, Ratios and Rates, Tables, Charts, and Graphs Summary of Formulas Summary of Formulas Proportion p= f n Percentage %=( f ) × 100% n Ratio Ratio = f1 f2 Rate Rate = factual fpossible