Prospective Sectoral GHG Benchmarks (PDF)
Document Details
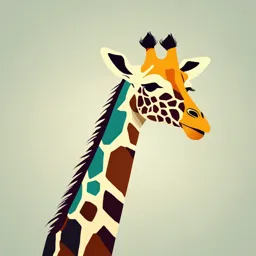
Uploaded by WittyAloe
Polytechnique Montréal
Anne-France Bolay, Anders Bjørn, Olaf Weber, Manuele Margni
Tags
Summary
This paper examines prospective sectoral greenhouse gas (GHG) benchmarks based on corporate climate mitigation targets. It analyzes the harmonization process of scope 1 and 2 emission targets of numerous companies across various sectors, using 2017 as a common reference year. The study highlights the importance of a sectoral approach and a consistent reference year to assess corporate climate mitigation targets accurately.
Full Transcript
Journal of Cleaner Production 376 (2022) 134220 Contents lists available at ScienceDirect Journal of Cleaner Production...
Journal of Cleaner Production 376 (2022) 134220 Contents lists available at ScienceDirect Journal of Cleaner Production journal homepage: www.elsevier.com/locate/jclepro Prospective sectoral GHG benchmarks based on corporate climate mitigation targets Anne-France Bolay a, *, Anders Bjørn b, c, d, Olaf Weber e, Manuele Margni a, f a Department of Mathematical and Industrial Engineering, Polytechnique Montréal, 2500 chemin de Polytechnique, Montréal, Québec, H3T 1J4, Canada b Department of Management, John Molson School of Business, Concordia University, 1450 Guy St., Montréal, Québec, H3H 0A1, Canada c Department of Geography, Planning and Environment, Concordia University, 1455 de Maisonneuve Blvd. W., Montréal, Québec, H3G 1MB, Canada d Loyola Sustainability Research Centre, Concordia University, 1455 de Maisonneuve Blvd. W., Montréal, Québec, H3G 1MB, Canada e Faculty of Environment, School of Environment, Enterprise and Development (SEED), University of Waterloo, 200 University Avenue West, Waterloo, Ontario, N2L 3G1, Canada f Institute of Sustainable Energy, School of Engineering, University of Applied Sciences and Arts Western Switzerland (HES-SO), Rue de l’Industrie 23, Sion, 1950, Switzerland A R T I C L E I N F O A B S T R A C T Handling Editor: Jian Zuo As climate change becomes firmly acknowledged as a financial risk, shareholders must consider corporate GHG performance to inform responsible investment decisions. To mitigate future climate risks, prospective bench Keywords: marks based on corporate climate mitigation targets are increasingly demanded by shareholders. However, Climate risk existing benchmarks often lack sufficient sectoral coverage or fail to appropriately harmonize target metrics, Corporate climate mitigation target scope, and corresponding timeframes. A harmonization process was developed which allows the calculation of Prospective benchmark sector-level prospective GHG benchmarks in terms of absolute or intensity GHG metrics. This process was applied Target ambition Responsible investment to harmonize scope 1 and 2 emission targets of 1697 companies reporting in 2018 CDP questionnaires across 13 Target progress sectors using 2017 as the common reference year. Results indicate the importance of using a sectoral approach and applying a common reference year to avoid considering reductions occurring before the target was launched due to company choice of target reference year. In 2030, the lowest and highest median reduction rates values are − 9% and − 36% for fossil fuels and power generation sectors respectively. The findings obtained strongly suggest targeted percentage of reductions should not be the only metric considered when comparing corporate climate mitigation targets amongst peers. Target progress when the target was announced and at a common reference year was found to be an important metric to assess potential greenwashing or poor ambition. In 14% of cases, targets were already achieved when company launched them. Funding sources Climate-related financial risks come from two main sources: physical risks and transition risks (Campiglio et al., 2018; Giuzio et al., 2019; This research did not receive any specific grant from funding TCFD, 2017). Physical risks involve climate induced weather events agencies in the public, commercial, or not-for-profit sectors. causing damage to physical assets, natural capital, and/or human capital (Monasterolo et al., 2017). These events may have significant financial 1. Introduction impacts to countries (Hsiang et al., 2017; Kompas et al., 2018; Nordhaus, 2017), companies (Bernstein et al., 2019; Dessaint and Matray, 2017; Climate change is increasingly recognized as a financial risk by Goldstein et al., 2019; Pankratz et al., 2019), and citizens (Hasegawa practitioners and academics (Battiston et al., 2017; Carney, 2015; Dietz et al., 2016; McMichael et al., 2006; Vicedo-Cabrera et al., 2021). et al., 2016). If not anticipated and correctly accounted for, climate risks To mitigate the financial impacts associated with physical climate- may become systemic risks and cause financial instability across global induced events, a transition to a low carbon economy is required markets (Bolton et al., 2020; Dafermos et al., 2018; Saha and Viney, (Campiglio et al., 2018). However, this process presents another set of 2020). financial risks and uncertainties i.e., transition risks (Monasterolo et al., * Corresponding author. E-mail address: [email protected] (A.-F. Bolay). https://doi.org/10.1016/j.jclepro.2022.134220 Received 7 March 2022; Received in revised form 25 June 2022; Accepted 18 September 2022 Available online 25 September 2022 0959-6526/© 2022 Elsevier Ltd. All rights reserved. A.-F. Bolay et al. Journal of Cleaner Production 376 (2022) 134220 2017; TCFD, 2017), stemming from climate-mitigation policies (such as (2017) compared 989 companies from Europe and United States, 75% of carbon pricing), technology obsolescence, and changes in consumer which reported targets, and compared targeted percentage reductions preference (Feyen et al., 2020; Semieniuk et al., 2020). between BY and TY, as previously done by Ioannou et al. (2016). Wang Climate policies have grown rapidly in number throughout the globe, and Sueyoshi (2018) analysed 1495 companies, of which 26.5% did not passing from 72 in 1997 to 1500 in 2018 (Nachmany and Setzer, 2018). have a target, and took differences in timeframe into account by Additionally, the ambition and effectiveness of such policies are ex comparing annualized targeted reductions. None of these two studies pected to increase carbon constraints on both the consumption and harmonized the target timeframes by using a common BY and TY, the production sides (Erickson et al., 2018). Furthermore, carbon prices are target metrics, and the scopes. Reported absolute and intensity reduc expected to increase from a median value of USD$ 10/tCO2e in 2020 tion targets are directly compared (Wang, 2017; Wang and Sueyoshi, (World Bank, 2020) to a minimum value of USD$ 50–100/tCO2e for a 2018), even when a percentage reduction in emission intensity generally two-degree pathway by 2030 (Stiglitz et al., 2017); up to an average translates to a different percentage reduction in absolute emissions. price of USD$ 179/tCO2e for a 1.5◦ pathway by 2030 (Rogelj et al., Accordingly, harmonizing the target to an equivalent unit is essential 2018). when using reduction targets to assess climate change risks. Reported Shareholders increasingly demand forward-looking approaches and targets with a different scope are also directly compared (Wang, 2017; industry data to effectively assess climate transition risks (Monnin, Wang and Sueyoshi, 2018). The target can be incomparable when they 2018; NGFS, 2021, 2019). Corporate GHG benchmarks have previously do not have the same scope coverage (Day et al., 2022) unless they are focused on current or historical performance, which is insufficient for harmonized in this regard (Institut Louis Bachelier, 2020). assessing future risks (Byrd et al., 2013; Sueyoshi and Wang, 2014; Van Building on the methodology of Dietz et al. (2018), the Transition Atten et al., 2021; Wiedmann et al., 2009; Y. P. Yu et al., 2016). How Pathway Initiative (TPI) has harmonized target timeframes by calcu ever, prospective benchmarks based on corporate GHG performance are lating an annualized reduction rate using 2019 as a common BY for useful to mitigate climate financial risks (Climate Action 100+, 2019; projections to 2030 and 2050 (Dietz et al., 2021a) and the target scope TCFD, 2021) and facilitate responsible investment strategies (Busch (Dietz et al., 2021b). If a target does not cover the same scope as the et al., 2011). Indeed, Boiral et al. (2012) found a significant positive link benchmark, the remaining emissions (not covered by the target scope) between corporate climate mitigation targets, corporate GHG perfor are assumed to be constant (Dietz et al., 2021b). However, TPI only mance, and financial performance. provided physical GHG intensity metrics, i.e. absolute GHG emissions Corporate climate mitigation targets are commonly expressed as a divided by a unit of production (e.g. kWh, ton of steel, etc.) which can percentage reduction of GHG emissions within a stated emission scope1 only by applied to homogeneous sectors (Koellner et al., 2005). As of between a base year (BY) and target year (TY). Corporate climate 2021, 401 companies have been analysed by TPI, of which 66% reported mitigation targets can be reported as absolute or intensity targets quantitative climate mitigation targets (Dietz et al., 2021a). (relative to a measure of activity or economic value) (Dahlmann et al., Another metric often used is economic GHG intensity (Institut Louis 2017; Herzog et al., 2006; Kolk and Pinkse, 2004). Corporate climate Bachelier, 2020; TCFD, 2021). Economic intensity metrics include ab mitigation targets and future corporate GHG performance are difficult to solute GHG emissions divided by a financial measure (Hoffmann and compare across organizations without harmonization of scopes, target Busch, 2008; P. Y. Yu et al., 2016). At the time of writing, no studies metrics, and timeframes (Institut Louis Bachelier, 2020). developed economic GHG intensity benchmarks based on reported Existing studies on corporate climate mitigation targets have focused corporate climate mitigation targets. Intensity targets pursue perfor on target determinants (Dahlmann et al., 2017; Ioannou et al., 2016; Yin mance improvements which do not necessarily lead to substantial re et al., 2017), target-setting approaches (Bjorn et al., 2021; Krabbe et al., ductions in absolute emissions (Dahlmann et al., 2017). The most 2015; Randers, 2012), and more recently, science-based targets (Gie commonly used financial measures are market capitalization, revenue, sekam et al., 2021a; Kuo and Chang, 2021). Other studies have done and enterprise value (Thoma et al., 2018). Revenue and enterprise value specific sector and country prospective GHG benchmarks based on are recommended by Net-Zero Asset Owner Alliance, 2020). Economic corporate climate mitigation targets. For example, Gouldson and Sulli intensity metrics can be applied to highly diversified sectors. However, van (2013) scrutinized targets from supermarkets in the UK, while they are highly susceptible to the price volatility associated with the Sullivan and Gouldson (2016) compared targets from US and UK com denominator used (Thoma et al., 2018). In contrast, physical intensity panies in the retail sector. Giesekam et al. (2018) examined targets from metrics are recommended for homogenous sectors (Koellner et al., the UK construction sector, while Immink et al. (2018) focused on the 2005) to avoid the influence of economic price volatility (TCFD, 2021). mining sector in South Africa. These type of studies are limited in the Previous studies either show a gap in their sectoral coverage (only broadness of firms included (Wang and Sueyoshi, 2018). considering a single sector or homogeneous sectors) or do not suffi Prospective GHG benchmarks based on corporate climate mitigation ciently harmonize scopes, timeframes, and target metrics. Accordingly, targets, including different sectors and a broad number of firms, have the main objective of this study is to establish absolute and intensity been previously developed (Dietz et al., 2021a; Wang, 2017; Wang and benchmarks with harmonized scopes, target metrics and timeframes Sueyoshi, 2018). Wang (2017) and Wang and Sueyoshi (2018) used based on self declared corporate climate mitigation targets i.e., absolute targeted percentage reductions without passing by absolute GHG GHG emission, economic GHG intensity (per revenue and per enterprise- emissions. Absolute GHG metrics are expressed as the total amount of value), and physical GHG intensity targets (per activity measure for carbon equivalent emissions and are useful to ensure respect of the homogeneous sectors). Once comparability is enabled through harmo global carbon equivalent budget (Hoffmann and Busch, 2008). How nized metrics, some targets may already be achieved at the chosen ever, misleading variations may occur due to a simple decrease in ac common BY. To better assess these, further analysis is needed regarding tivity, or events such as mergers and acquisitions; both of which tend to target progress and achievement. Accordingly, the second objective of influence intensity metrics less (Hoffmann and Busch, 2008). Wang this study is to assess companies progress towards their GHG reduction targets. 1 2. Methodology Scope 1 represent direct GHG emissions of the corporation (Greenhouse Gas Protocol, 2004). Scope 2 is GHG emissions generated from purchased electricity which could be reported as location-based (regional grid-average emission Sector-specific benchmarks are calculated for 2020, 2025 and 2030. factor data) or market-based (specific emission factors from contractual The main displayed statistical metrics are the first quartile (Q1) and agreement) (Greenhouse Gas Protocol, 2015). Scope 3 is all the remaining value median which help determine if a company is targeting emission re chain GHG emissions (GreenHouse Gas Protocol, 2011). ductions greater than 75% or 50% of their peers. Target progress is 2 A.-F. Bolay et al. Journal of Cleaner Production 376 (2022) 134220 assessed at different points in time by calculating whether targets are Targets were harmonized to the same unit as the benchmark by already achieved, absolute emissions have reduced since the company- transforming intensity targets into absolute targets, or the opposite specified base year, or if those emissions have increased. depending on the benchmark unit. The following units are defined for each benchmark: tCO2e for absolute GHG emissions, tCO2e per million US dollars of revenue and enterprise-value for economic intensity, and 2.1. Benchmarks development tCO2e per activity measure for physical intensity. The methodological process to develop absolute and intensity GHG 2.1.2. Data preprocessing of emission target disclosure benchmarks consists of multiple steps as presented in Fig. 1 and Step 2 consists of ensuring target data completeness and accuracy. explained in the following subsections. Note that Steps 4b and 4c are Table 1 identifies the completeness of company data required and cor only needed to adjust intensity benchmarks when the unit of reduction responding data sources for each benchmark. The two main sources of target is not the same as the intensity benchmark metric. Sensitivity company GHG emissions data and intensity metrics used here are Car analysis is described in Section 2.2. Fictious examples are provided in bon Disclosure Project (CDP) and Bloomberg (Bloomberg L.P., 2020; supplementary information. CDP, 2018). The data identified in Table 1 were extracted from the corresponding sources and compiled into a consolidated dataset. 2.1.1. Harmonization of scopes, target metrics and timeframes To ensure robustness, any data entry that is incomplete or judged to Harmonization is required to allow target comparisons as previously be unsuitable has been removed. The units or classifiers adopted in this discussed. As such, step 1 involves harmonization of choices regarding study are identified in brackets in Table 1. Reported targets that do not scope of emissions, reference year, and target metrics. adhere to the specified unit or classifier type are considered unsuitable This study only harmonized target on scope 1 and 2 and excluded and excluded from the benchmark of concern. For example, targets re scope 3. As shown by Klaaβen and Stoll (2021), reported scope 3 ported with the status ‘expired’ are unsuitable and thus excluded. emissions for most organizations are often found to be either too Some company data required for this study are subject to harmoni incomplete or inconsistent. In addition, most companies only report zation choices such as scope covered and 2017 emissions. The presented scope 1 and 2 emissions. When the reported scope 2 market-based procedure can also be applied to different harmonization choices lead emissions were available, they have been used over scope 2 ing to different company data required. location-based data. The next part of Step 2 involves evaluating data consistency for each The company-specified base year significantly vary from one orga company across years, target type, and between data sources. Because nization to another, ranging from 1990 to 2018. In this study, 2017 is multiple inconsistencies were found in the reported data, targets have selected as the common BY across all organizations for two reasons. been disregarded if they did not meet the following conditions: First, 2017 is the latest year available in the dataset. Second, 2017 is the year with the most data available. Indeed, 98.8% of companies have 1. The reported period given for the BY and 2017 must cover a full year. data available for 2017, compared to 95% for 2016. The presented harmonization procedure can also be applied to more recent and future datasets and to different target scopes. Fig. 1. Flowchart of the methodological process to determine absolute and intensity GHG benchmarks. 3 A.-F. Bolay et al. Journal of Cleaner Production 376 (2022) 134220 Table 1 ( ) Input data required to derive sectorial benchmarks. εTY = εBY − %RSC SC BY × %SC × εBY Equation1 Benchmark’s Data type Company data required (unit or Sources Where: type classifier) All Target BY (year) 2018 CDP εTY TY annual emissions for scope 1 and 2 in tCO2e TY (year) Target scope (scope 1, scope 2 εBY BY annual emissions for scope 1 and 2 in tCO2e location-based, scope 2 market- %RSCBY targeted percentage reduction from the BY for given scope based, scope 1 & 2 location- covered based, or scope 1 & 2 market- %SC percentage of given scope covered based) Target status (underway or εSC BY BY annual emissions for given scope covered in tCO2e new) Targeted reduction from BY 2.1.4. Linear interpolation until the target year (%) Step 4a establishes a reduction trajectory by calculating the emis Scope covered (%) BY emissions covered by target sions in every year between the common BY (2017) and TY. Since no (tCO2e) information is given by companies for their reduction trajectory, a linear GHG BY emissions for scope 1 2010–2018 interpolation is applied as follows: emissions (tCO2e) CDP BY emissions for scope 2 εTY − εCBY εy = × (y − CBY) + εCBY Equation 2 market-based or location-based TY − CBY (tCO2e) 2017 emissions for scope 1 2017–2018 Where: (tCO2e) CDP 2017 emissions for scope 2 εy annual emissions for scope 1 & 2 in tCO2e in year y market-based or location-based (tCO2e) TY target year Sector CDP’s Activity Classification 2018 CDP CBY common BY System y given calendar year Absolute GHG Target Corresponding absolute 2018 CDP εCBY common BY annual emissions for scope 1 and 2 in tCO2e emission emissions change for intensity targets (%) Economic GHG Target Corresponding absolute 2018 CDP The linear interpolation is only carried out if the common BY emis intensity emissions change for intensity sions εCBY is higher than the TY emissions. Hence, if a company has targets (%) reduced emissions between its company-specified BY and the common Economical Company Ticker (Ticker value) 2018 CDP BY sufficiently to already meet its reduction target, it is assumed that the Up to 1990 to 2019 revenues Bloomberg ($M US current 2019 value) absolute emissions remain constant until the TY. The only exception Up to 1990 to 2019 enterprise Bloomberg from this assumption is when companies explicitly report an expected value ($M US current 2019 increase in absolute emissions when disclosing an intensity target. value) If a company has multiple target years, linear interpolation is applied Physical GHG Target Activity metric of intensity 2018 CDP intensity target (homogeneous sectoral from the common BY to the first TY, from the first TY to second TY, and activity measure unit) so on. In Equation (2), ‘CBY’ and ‘εCBY ’ are replaced by the first TY and Physical Up to 1990 to 2017 activity 2010–2018 emissions for the first TY, respectively, etc. This allows emissions re values (homogeneous sectoral CDP ductions to be calculated and compared for years before the TY. activity measure unit) 2.1.5. Linear extrapolation of the activity measure value until the target 2. The sum of scope 1 and 2 emissions for the BY and the sum of scope 1 year and intensity calculation and 2 emissions for 2017 (common BY) must be above zero (entries Steps 4b and 4c establish intensity values up to the TY via the of zero annual emissions were assumed to be errors). extrapolation of linear regression models established from historical 3. The BY emissions reported must be within±20% of the adjusted data. These steps are only necessary for intensity benchmarks when value with the percentage of scope covered by the target and the field reported targets do not match the homogeneous unit for a given sector. ‘Base year emissions covered by target’ in CDP questionnaire. That is, for absolute emission targets where activity information such as production or revenue are also known. Step 4b extrapolates the activity Finally, when companies report multiple targets for the same TY, measure value for the corresponding intensity type. typically covering different scopes, only the most comprehensive/in Step 4c calculates the emission intensities by combining the results of clusive target has been included. For instance, if a company reports two Step 4a and Step 4b as described in Equation (3). Note that a negative targets for the year 2025, one covering scope 1 + 2 and the other only activity measure value for some years may occur from Step 4b if the covering scope 2, only the former was included. linear extrapolation based on historical data results in a negative slope. For such cases, emission intensities for those years are not included 2.1.3. Calculation of emissions in target year because the result is a model artefact. Step 3 calculates the TY emissions as expressed in Equation (1), εIy = εy ÷ Iy Equation 3 where the part in brackets calculates the reduced emissions relative to the BY. %SC × εSCBY correspond to the value found in the field ‘Base year Where: emissions covered by target’ reported in the CDP questionnaires (See Table 1). Targets included cover scope 1, scope 2 location-based, scope 2 εIy annual emission intensity in tCO2e per activity measure market-based, scope 1 & 2 location-based, or scope 1 & 2 market-based. Iy annual activity measure value in unit of the corresponding Note that when a target does not fully cover the harmonized scope (1 & benchmark 2), the uncovered emissions are assumed to remain constant between the BY and TY, following Dietz et al. (2021b). For instances in which intensity targets are reported in the homo 4 A.-F. Bolay et al. Journal of Cleaner Production 376 (2022) 134220 geneous unit, Steps 4b and 4c are excluded, and Steps 1 through 4 apply targets were considered complete and accurate, i.e., 964 companies and with a unit modification for the emissions variable ‘ε’. i.e., tCO2e will 1325 targets. Exclusions were due to incomplete information (39%), become tCO2e per activity measure. Physical activity unit measures for unsuitable target status such as expired targets (33%), targets with homogeneous sectors can be MWh for the power generation sector, MJ overlapping scopes and same TY (13%), and data inconsistencies (15%). for the fossil fuels sector, and so on. 36% of data were removed due to inconsistencies involving contradic tory information reported for different questions in the same CDP 2.1.6. Normalization of emission targets to a common base year questionnaire. Step 5 calculates the relative reduction of GHG emissions normalized to the common BY (2017) as given by Equation (4). 3.2. Final sample description ̂ y = Xy ÷ X2017 − 1 R Equation 4 For the 964 companies with useable target data, total revenue and enterprise values exceed 16.3 trillion and 28.5 trillion USD$, respec Where: tively. Scope 1 emissions for 2017 was 2.6 GtCO2e, which represents 7% of global fossil fuels and industrial emissions (Jackson et al., 2018). R ̂ y percentage of normalized reduction Scope 2 emissions for 2017 was 0.6 GtCO2e. Emissions in 2017 per Xy annual emissions for scope 1 & 2 in the benchmark target metric sector and their distribution are available in supplementary information. unit their headquarters in the European region, 26% in the USA, 10% in X2017 annual emissions for scope 1 & 2 for the common BY (2017) in Japan, and 6% in China. The number of companies per region or country the benchmark target metric unit is available in supplementary information. For the 1325 targets included, 70% cover at least 95% of their scope 1 and 2 emissions. A sectoral benchmark is then determined for the 13 sectors reported Table 2 presents the number of companies and targets by sector in the CDP Activity Classification System by consolidating targets for included in the final sample, and general descriptive statistics for the each company through a statistical representation (median, 1st, and 3rd target timeframe. The timeframe represents the difference between quartiles). company-specified BY and TY. The results are separated between targets reported as absolute or intensity by companies and include their number 2.2. Sensitivity analysis by sector. On average, reported absolute targets tends to have longer timeframes than intensity. Sensitivity analysis is performed on methodological Steps 4a and 4b Table 3 shows the number of companies included in each benchmark to evaluate the impact of various assumptions and linear extrapolation according to sector and year, which represents the number of data points techniques on final benchmark results. The first sensitivity analysis is included in further results as all duplicate targets per year has been applied for all benchmark types. The default case assumes that absolute excluded (see Section 2.1.2). Year 2017 includes all the companies emissions will stay constant if TY emissions are already achieved in having an ongoing target with a TY later than 2017, while other years 2017, unless specified otherwise by an intensity target. The sensitivity represent the numbers of companies included with a target for that year analysis case involves performing a linear interpolation from the com or later. mon BY to the TY i.e., the company of concern may have already ach The number of companies reporting data are not uniform across ieved the TY emissions in 2017 which would lead to an increase of sectors and years. Some sectors are underrepresented, which means interpolated values. potentially lower sectoral benchmark robustness. For example, Apparel, The second sensitivity analysis evaluates two linear extrapolation Hospitality, Mineral extraction have less than 20 companies with targets methods and two data exclusions conditions. The default case applies a extending to 2020. Long-term targets are rare across all sectors. Whereas linear extrapolation and includes all resulting data regardless of how 91% of companies have targets until 2020, only 30% have until 2030, well the linear model fits historical data values. The sensitivity analysis 9% until 2050, and two companies past 2050. case applies a two-part discontinuous piecewise linear regression model to the data and extrapolates based on the last linear segment. This 3.3. Absolute GHG sectoral benchmark method identifies trend reversals that may occur throughout historical data and bases the extrapolation on the most recent trend. The breakout The percentage of change per sector including median and Q1 for year for each company is determined by maximizing the coefficient of from 2017 to 2020, 2025 and 2030 are presented in Table 4. The values determination (R2 ) for each model independently. Adjusted cases displayed show a substantial difference in reduction between sectors. In exclude companies that produce an R2 value less than 0.4. 2030, the median values for fossil fuels and power generation sectors are − 9% and − 36% respectively; a difference of 27 percentage points. Q1 2.3. Target progress results for 2030 ranges from − 14% for apparel to − 62% for materials; a difference of 48 percentage points. These differences show the impor The target progress of companies is assessed from the start year tance of taking a sectoral approach when developing benchmarks based (when a target was announced) and 2017 (the common BY) considering on corporate climate mitigation targets to guide investment decisions. three cases: 1) target already achieved, 2) absolute emissions reduced, 3) Targets with longer timeframes do not necessarily have higher absolute emissions increased. ambition (Callery and Kim, 2021). For the apparel sector, for example, the percentage of change for the median and Q1 is higher in 2025 than in 3. Results 2030. The targets of this sector cover four companies in 2025 and two in 2030, implying a different subset (See Table 2). 3.1. Data completeness and accuracy 3.3.1. Sensitivity analysis In total, 2607 companies reported information in the 2018 CDP Fig. 2 presents the sectoral results for the default and sensitivity questionnaire. Of these, 27% did not report emission reduction targets, analysis cases in 2020, 2025, and 2030. 8% reported targets for scope 1, 2, and 3 emissions, and 65% for scope 1 The main difference between the two cases is for the third quartile and/or 2 emissions. 1697 companies reported 3009 targets for scope 1 (Q3) and the maximum values as illustrated in Fig. 3. When a target is and/or 2. already achieved, the common base year emissions are lower than the After data preprocessing (step 2), only 44% of scope 1 and/or 2 target year emissions resulting in an increase in the sensitivity analysis. 5 A.-F. Bolay et al. Journal of Cleaner Production 376 (2022) 134220 Table 2 Number of companies and targets by sector included in the final sample and descriptive statistics on the target timeframe. The target timeframes are separated depending on whether companies reported them as absolute or intensity targets. No C. represents the number of companies while No T. is the number of targets, and Med. the median. General Timeframe of target reported as absolute Timeframe of target reported as intensity Sector/Metrics Nbr C. Nbr T. Nbr Min. Med. Max. Mean Std. Nbr Min. Med. Max. Mean Std. Apparel 13 14 10 5 8.5 17 9.60 4.55 4 2 5 13 6.25 4.72 Biotech, health care & pharma 48 60 39 1 10 40 11.31 8.68 21 3 7 18 7.81 3.89 Food, beverage & agriculture 55 71 41 1 12 37 14.39 9.20 30 4 10 25 9.37 4.54 Fossil Fuels 23 31 21 4 8 20 9.29 4.99 10 4 11 38 13.50 10.00 Hospitality 16 23 8 5 10 39 14.00 12.04 15 3 15 40 16.13 10.85 Infrastructure 70 100 65 3 10 60 14.23 11.59 35 3 9 43 12.00 10.39 Manufacturing 316 414 232 1 11 60 14.21 10.24 182 1 7 40 8.73 5.69 Materials 52 61 25 1 10 45 14.00 11.60 36 1 14.5 40 16.33 11.76 Mineral extraction 13 20 12 3 10 33 12.50 9.32 8 2 10 15 8.50 4.07 Power generation 28 44 25 3 15 45 16.96 10.91 19 1 12 40 15.68 10.34 Retail 31 51 32 4 10.5 44 16.59 11.17 19 4 9 15 8.68 3.06 Services 265 392 272 1 11 45 14.63 10.37 120 1 7 34 8.83 5.88 Transportation services 34 44 14 1 12 45 14.57 13.44 30 4 9.5 39 11.37 8.01 Overall 964 1325 796 1 11 60 14.19 10.32 529 1 8 43 10.16 7.50 Table 3 Number companies per year and sector included for each benchmark. The acronym Abs. corresponds to the absolute GHG benchmark, Rev. to the economic intensity per revenue, E.V. to the economic intensity per enterprise value, and Ph. to the physical intensity one. 2017 2020 2025 2030 Sector/Benchmark Abs. Rev. E.V Ph. Abs. Rev. E.V. Ph. Abs. Rev. E.V. Ph. Abs. Rev. E.V. Ph. Apparel 13 10 9 0 12 9 8 0 4 4 3 0 2 2 2 0 Biotech, health care & pharma 48 46 42 0 43 42 39 0 15 14 12 0 10 9 8 0 Food, beverage & agriculture 55 50 48 0 54 50 48 0 22 20 19 0 16 14 12 0 Fossil Fuels 23 23 23 0 23 23 23 0 12 12 12 0 8 8 8 0 Hospitality 16 16 15 0 15 15 14 0 8 8 7 0 8 8 7 0 Infrastructure 70 65 62 0 65 59 57 0 30 28 28 0 26 24 24 0 Manufacturing 316 259 251 0 274 225 218 0 117 101 97 0 75 64 62 0 Materials 52 50 46 0 48 46 43 0 24 22 20 0 16 16 12 0 Mineral extraction 13 13 13 0 10 9 10 0 4 4 4 0 3 3 3 0 Power generation 28 27 25 32 27 26 24 31 22 21 19 24 20 20 17 22 Retail 31 31 31 0 31 31 30 0 17 17 17 0 9 9 9 0 Services 265 240 177 0 243 214 156 0 122 108 81 0 91 81 58 0 Transportation services 34 31 29 0 31 28 25 0 10 9 9 0 7 6 6 0 Overall 964 861 771 32 876 777 695 31 407 368 328 24 291 264 228 22 in Section 3.6. Table 4 The Q1 and the median values of the default case and the sensitivity Absolute GHG benchmarks results. All displayed values are harmonized to analysis are similar. Of the 39 observations for Q1 (13 sectors for three cover scope 1 and 2 emissions and refer to 2017 as common BY. years), Q1 values are the same in 34 cases. The same number of differ 2020 2025 2030 ences occur for the median. The average annualized difference between Sector/Metrics Median Q1 Median Q1 Median Q1 the two cases is 0.32% for the median and 0.2% for Q1. Apparel − 4% − 11% − 28% − 58% − 9% − 14% Biotech, health − 3% − 16% − 18% − 30% − 27% − 36% care & pharma 3.4. Economic intensity sectoral benchmarks Food, beverage & − 6% − 15% − 16% − 24% − 21% − 33% agriculture Economic intensity benchmarks have been calculated with respect to Fossil Fuels − 4% − 12% − 4% − 13% − 9% − 19% Hospitality − 4% − 8% − 12% − 14% − 21% − 27% revenue and enterprise-value. The percentage of change per sector Infrastructure − 6% − 13% − 16% − 23% − 29% − 37% including median and Q1 from 2017 to 2020, 2025 and 2030 are pre Manufacturing − 5% − 15% − 15% − 23% − 27% − 35% sented in Table 5. Since financial information was not available for all Materials − 5% − 23% − 12% − 26% − 29% − 62% companies in the final sample (964), the revenue-based intensity Mineral 5% 12% 20% 30% 29% 34% benchmark builds on 868 companies, while enterprise value-based in − − − − − − extraction Power generation − 7% − 11% − 22% − 29% − 36% − 45% tensity benchmark builds on 771 companies. The number of companies Retail − 11% − 19% − 14% − 34% − 25% − 41% included in each sector and year is available in Table 2. Services − 4% − 13% − 15% − 34% − 24% − 42% From the results displayed in Table 5, 2030 median values for the Transportation 5% 18% 9% 23% 22% 35% − − − − − − revenue benchmark range from − 12% for apparel to − 67% for power services Overall − 5% − 15% − 15% − 26% − 25% − 39% generation; a difference of 55%. Q1 value varies from − 26% for apparel to − 73% for materials; a difference of 47%. For the enterprise value benchmark, median values vary from − 1% for apparel to − 63% for While in the default case, the common base year emissions are assumed mineral extraction and from − 29% for apparel to − 75% for mineral to remain constant until the target year (conditional interpolation) extraction for Q1 value; a difference of 62% and 46% respectively. which explains the difference between the two cases for the Q3 and Notable differences are occurring between absolute GHG emissions maximum values. The percentage of targets already achieved is detailed and economic intensity benchmarks. Inflation and other economic 6 A.-F. Bolay et al. Journal of Cleaner Production 376 (2022) 134220 Fig. 2. Absolute benchmarks scenario results for default case and the sensitivity analysis. The default case is a conditional interpolation between the common base year emissions and target year emissions if the target is already achieved, the emissions are assumed to stay constant unless an expected increase in absolute emissions is reported by an intensity target. The sensitivity analysis is a linear interpolation between the common base year and the target year even if the target is already achieved. All displayed values are harmonized to cover scope 1 and 2 emissions and refer to 2017 as common BY. In the boxplot, the bottom of the box represents the first quartile (Q1), the line is the median while the top of the box is the third quartile (Q3). The whiskers are at 1.5 times the interquartile range. Fig. 3. Revenue-based intensity benchmarks results for default case and the sensitivity analysis. The default case is a financial extrapolated values based on a linear regression model and the sensitivity analysis is a financial extrapolated values based on the last segment of a discontinuous piecewise linear regression model. All displayed values are harmonized to cover scope 1 and 2 emissions and refer to 2017 as common BY. In the boxplot, the bottom of the box represents the first quartile (Q1), the line is the median while the top of the box is the third quartile (Q3). The whiskers are at 1.5 times the interquartile range. parameters were assumed to be already considered by companies in 3.4.1. Sensitivity analysis their targets. Perhaps this is not always the case and that can explain A sensitivity analysis was performed on the financial extrapolation some of these differences. However, differences in sector ranking occur method corresponding to Step 4b (see Section 2.2). The results for the between the benchmarks. For example, 2030 median value for the fossil default and sensitivity analysis cases are presented in Figs. 3 and 4 for sector in the revenue benchmark is − 60% (sector ranking: 2nd position revenue and enterprise value respectively. The adjusted cases are pre on 13 sectors) while value for the enterprise value benchmark is − 41% sented in supplementary information. (sector ranking: 11th position on 13 sectors). In the absolute GHG Differences between cases are substantial as indicated by the large emission benchmark, the fossil fuels sector has a 2030 median value of difference in data dispersion displayed in Figs. 3 and 4. The discontin − 9% (sector ranking: 13th position on 13 sectors). These differences uous piecewise linear regression captures the last trend of a company show the importance of adequately choosing the benchmark type to historical financial values which leads to an increased number of guide appropriate investment decisions. extrapolated financial values based on a negative growth (negative slope) compared to the default case which captures the global trend. For 7 A.-F. Bolay et al. Journal of Cleaner Production 376 (2022) 134220 Table 5 Economic intensity benchmarks results. All displayed values are harmonized to cover scope 1 and 2 emissions and refer to 2017 as common BY. Revenue (tCO2e/$M USD of revenue) Enterprise Value (tCO2e/$M USD of enterprise value) 2020 2025 2030 2020 2025 2030 Sector/Metrics M. Q1 M. Q1 M. Q1 M. Q1 M. Q1 M. Q1 Apparel − 9% − 18% − 40% − 64% − 12% − 26% − 11% − 19% − 44% − 49% − 1% − 29% Biotech, health care & pharma − 18% − 24% − 36% − 48% − 50% − 59% − 11% − 18% − 38% − 53% − 44% − 64% Food, beverage & agriculture − 18% − 31% − 32% − 43% − 41% − 55% − 8% − 20% − 22% − 36% − 37% − 51% Fossil Fuels − 36% − 44% − 47% − 56% − 60% − 68% − 20% − 34% − 29% − 40% − 41% − 53% Hospitality − 17% − 22% − 34% − 35% − 44% − 48% − 10% − 17% − 25% − 30% − 35% − 43% Infrastructure − 25% − 33% − 39% − 50% − 55% − 66% − 18% − 29% − 35% − 46% − 43% − 62% Manufacturing − 19% − 31% − 35% − 46% − 52% − 59% − 5% − 23% − 25% − 40% − 44% − 56% Materials − 23% − 38% − 36% − 43% − 50% − 73% − 9% − 28% − 16% − 43% − 45% − 72% Mineral extraction − 26% − 35% − 50% − 57% − 59% − 64% − 32% − 43% − 55% − 68% − 63% − 75% Power generation − 31% − 41% − 53% − 59% − 67% − 73% − 19% − 30% − 26% − 50% − 51% − 65% Retail − 25% − 30% − 38% − 48% − 49% − 61% − 20% − 35% − 46% − 51% − 57% − 60% Services − 18% − 27% − 36% − 50% − 48% − 63% − 14% − 23% − 33% − 47% − 46% − 62% Transportation services − 19% − 33% − 33% − 38% − 48% − 57% − 16% − 36% − 31% − 40% − 45% − 52% Overall − 20% − 31% − 37% − 49% − 50% − 63% − 12% − 26% − 29% − 46% − 45% − 61% Fig. 4. Enterprise value-based intensity benchmarks results for default case and the sensitivity analysis. The default case is a financial extrapolated values based on a linear regression model and the sensitivity analysis is a financial extrapolated values based on the last segment of a discontinuous piecewise linear regression model. All displayed values are harmonized to cover scope 1 and 2 emissions and refer to 2017 as common BY. In the boxplot, the bottom of the box represents the first quartile (Q1), the line is the median while the top of the box is the third quartile (Q3). The whiskers are at 1.5 times the interquartile range. the sensitivity analysis, the mix of an increasing number of negative for the power generation sector includes 19 companies with reported slopes and lower number of positive slopes leads to substantial differ intensity targets, and 13 companies with 22 absolute targets converted ences and an extended interquartile range as shown in Figs. 3 and 4. using production data into physical intensity targets. Both economic benchmarks are very sensitive to the financial The percentage of change including median and Q1 from 2017 to extrapolation methods used. Some sectors are more sensitive than others such as infrastructure, mineral extraction, and power generation which have at least 3% differences per year for both statistical metrics (median Table 6 and Q1) in both economic benchmarks. For the revenue benchmark, the Benchmark results for the power generation sector. All displayed values are average annualized difference is 2% for the median and 1.87% for Q1. harmonized to cover scope 1 and 2 emissions and refer to 2017 as common BY. For the enterprise value benchmark, the average annualized difference 2020 2025 2030 is 3.38% for the median and 2.84% for Q1. However, the mineral Sector/Metrics Median Q1 Median Q1 Median Q1 extraction sector is skewing the average difference results by contrib Physical intensity − 7% − 16% − 19% − 29% − 35% − 47% uting to a much larger proportion. Absolute GHG − 7% − 11% − 22% − 29% − 36% − 45% emission Economic − 31% − 41% − 53% − 59% − 67% − 73% 3.5. Physical intensity sectoral benchmark intensity (per revenue) Only the power generation sector had a relevant number of com Economic − 19% − 30% − 26% − 50% − 51% − 65% panies (more than 20) reporting physical intensity benchmarks with intensity (per consistent physical units (TCO2e/MWh) and represents 25% of all 2017 enterprise value) emissions from the data in this study. The physical intensity benchmark 8 A.-F. Bolay et al. Journal of Cleaner Production 376 (2022) 134220 2020, 2025 and 2030 for all benchmarks regarding the power genera (GHG protocol). The use of REC is reported by 352 companies studied tion sector are presented in Table 6. No substantial difference occurs for a total of 90.7 TWh while the total energy purchased by the 964 between the physical intensity and absolute GHG emission benchmarks. companies studied represents 3213.6 TWh. However, some companies However, notable differences occur between those two benchmarks and may have not shared information about the use of offsets or RECs and the two economic intensity benchmarks. Economical intensity bench these numbers are potentially underestimated. Nevertheless, companies marks include financial growth which further diminish the intensity with a scope 2 market-based targets tend to have more ambitious targets reduction. Nevertheless, the sector ranking is similar with 1st position in than those with a scope 2 location-based target (Bjorn et al., 2021), the absolute GHG emissions and economic intensity per revenue indicating that companies with market-based targets generally intent to benchmarks, and 3rd position in the economic intensity per enterprise purchase RECs. value. Depending on the company choice of target BY, emission reductions may occur before the target was announced (start year). As such, target 3.5.1. Sensitivity analysis progress results have been analysed between the company-specified BY A sensitivity analysis was performed on the extrapolation method and the target start year, and are presented in Fig. 6. In 14% of cases, corresponding to Step 4b (see Section 2.2). Only the default case and targets were already achieved in the year they were announced which adjusted default case have been applied since there was insufficient data indicates a potential lack of ambition (Fig. 6). The fossils fuels sector had to establish a piecewise model. In total, the default case includes 32 30% of companies with achieved targets in 2017, while an analysis of companies while the adjusted case includes 27 companies. No substan target progress at the start year shows that 20% of sector targets were tial difference was found to occur between these two cases. The average achieved when launched demonstrating the importance of such analysis. annualized differences between the two cases are 0.09% for the median Differences between the company-specified BY and target start year and 0.15% for Q1. is one year for the median and two years on average. On average, 27% of target emission reductions occurred between the company-specified BY 3.6. Target progress and the target start year. Nevertheless, 36% of targets have the same BY and start year, meaning that those companies did not have reductions For the 964 companies, 22% had already achieved their most long- caused by their choice of target BY. term target in 2017, 46% have reduced their absolute emissions compared to the company-specified BY, and 32% have increased them 4. Discussion since. The median annualized absolute emission reductions across all sectors between the reported BY and 2017 is 1.76%. Differences be 4.1. Comparison to existing literature tween 2017, the common BY defined in this study, and the BY reported by companies in the CDP is 3 years for the median and 4.36 years on In total, 2607 reporting companies were assessed in this study, which average. is a substantial increase compared to previous studies (989 companies The progress on climate mitigation targets per sector in 2017 are for Wang, 2017, 1495 companies for Wang and Sueyoshi, 2018, and 401 presented in Fig. 5. Some sectors have more companies with achieved companies for Dietz et al., 2021). The share of companies not reporting a targets than others as shown in Fig. 5. The Hospitality, Fossil Fuels, and climate mitigation target (27%) is consistent with previous studies using Infrastructure sectors have more than 30% of their companies with earlier CDP questionnaires (25% for Wang, 2017 and 26.5% for Wang achieved targets. and Sueyoshi, 2018) or more recent database, (34% for Dietz et al., Some progress may be due to the use of offsets by companies if they 2021). have improperly included them in their absolute emissions reporting. Only 44% of the 3009 scope 1 and/or 2 targets reported in CDP Nevertheless, 152 of the 964 companies studied reported purchasing questionnaires were considered complete and accurate. Some exclusions 10.5 MtCO2e of compliance carbon credits or voluntary offsets. The such as unsuitable target status or duplicate entries can not be avoided. reduced emissions from all the company-specified BY to 2017 represent However, 46% of the excluded targets could have been avoided by more 302.3 MtCO2e. Another part of the progress may be due to the use of diligent reporting. Giesekam et al. (2021a) which studied the renewable energy certificates (RECs) as they are allowed in the scope 2 science-based emission reduction targets (final sample of 133 targets) market-based accounting approach (Greenhouse Gas Protocol, 2015) mentioned an exclusion rate of 21% due to insufficient information Fig. 5. Target progress in 2017 by percentage of companies per sector. Results presented by the highest percentage of achieved targets. 9 A.-F. Bolay et al. Journal of Cleaner Production 376 (2022) 134220 Fig. 6. Target progress at the start years by percentage of target per sector. Results presented by the highest percentage of achieved targets. being available. Here, the exclusion rate due to incomplete information by the targets included in each sample and the sampling year: TPI covers is 39%. Since both science-based and non-science-based targets were targets reported in 2020, while 2018 is covered here. As should be ex included, non-science-based targets may be more prone to having pected, some companies have more ambitious targets which will incomplete information. decrease Q1 sectoral results. Differences in targeted absolute emission reductions between sectors Regarding progress on climate mitigation targets, 22% of companies in 2030 were found to range from − 9% to − 62% depending on the have achieved their most long-term target, while 46% have reduced metrics used (see Section 3.3). Dietz et al., 2021; Wang (2017); Wang from the company-specified BY, and 32% have increased. This is and Sueyoshi (2018) used a different sectoral classification, but sub consistent with Wang (2017) and Kuramochi et al. (2021) for the com stantial differences in targeted emission reductions between sectors also bined results of achieved and reduced from their BY results and Giese occurred in those studies. Wang (2017) used the target percentage kam et al. (2021a) for the achieved target. However, Wang (2017) and reduction without annualizing it between the BY and TY, making it Kuramochi et al. (2021) only defines whether a target is on track or not difficult to compare with our results. Wang and Sueyoshi (2018) annu compared to the linear reduction trajectory between the BY and TY, alized the percentage of reduction from the BY to the TY but did not while Giesekam et al. (2021a) also assess if the target is achieved. Our present aggregated results at the sector level, instead focusing on approach to evaluate the progress in respect to climate mitigation tar regional differences. To allow comparison with the results of Wang and gets avoids the linear trajectory assumption while still differentiating Sueyoshi (2018), the results here have been annualized and aggregated achieved, reduced, or increased compared to the company-specified BY. per region (see Supplementary Information). The percentage of absolute Due to their low sample size, Giesekam et al. (2021b) did not include a emission reductions obtained are generally lower than that of Wang and sectoral comparison. Our results show substantial difference between Sueyoshi (2018), even though the data used in this study is 5 years more sectors for the target progress ranging from 15% to 31% for achieved recent and companies should be expected to have increased their targets, to 29%–62% for the one reducing, and 23%–54% for targets ambition. For example, the US annualized average reduction rate in increasing their absolute emissions compared to the company-specified Wang and Sueyoshi (2018) is − 3.14% while here is 2.4% for 2020, 2.5% BY (see Fig. 6). While these achievements are promising for some sec for 2025 and 2.2% for 2030. As explained in Section 3.6, part of the tors, further research is needed to assess whether the targets progression reduction occurred before the target start year (14% of targets were actually correspond to more emission reduction than companies’ already achieved by that time) and between the target start year and the business-as-usual trajectory, as mentioned by Kuramochi et al. (2021). common BY, which can explain some of the difference with Wang and Giesekam et al. (2021a) mentioned some targets were achieved Sueyoshi (2018) results. Further studies should correct for the reduction before official SBT approval. In this study, it was examined whether occurring before the target start year which represents 27% of the targets were achieved before their start year. Such cases were found to reduction target on average. Nevertheless, it is interesting to note that correspond to 14% of the final sample. This raises questions concerning amongst developed countries, Canadian firms have the lowest annual poor ambition by companies as also indicated by Giesekam et al. ized average reduction rate in 2025 (− 0.7%) and 2030 (− 1%), in (2021a). However, most of those targets are not science-based and their agreement with the findings of Wang and Sueyoshi (2018). achievement is primarily due to the choice of the BY. Choosing targets Dietz et al., 2021 displayed an annual average percentage of emis parameters in such a manner can be considered ‘greenwashing’ as sion reductions with a 2019 BY for their sectoral physical intensity defined by Delmas and Burbano (2011), and also, Lyon and Montgomery benchmarks. The data used by TPI come from the latest version of FTSE (2015). Russell (TPI, 2022), while data for this study comes from 2018 CDP academic package. To allow a better comparison, a normalization with a 2017 BY has been performed using the TPI, 2021 database for power 4.2. Limitations and future research generation companies assessed in 2019 (see Supplementary Informa tion). The TPI sample included 31 companies while our sample included The main limitations of the present study are the exclusion of scope 3 32. Median targeted reductions are identical for TY 2025 and 2030, targets, the data accuracy of reported information, the assumption of while TPI finds lower median targeted reductions for TY 2020 and lower linear reductions for benchmark results and the emissions not covered Q1 of targeted reductions for all TY. These differences can be explained by a target, the high sensitivity to the extrapolation method used for economic-intensity targets, and the lack of physical production data in 10 A.-F. Bolay et al. Journal of Cleaner Production 376 (2022) 134220 CDP questionnaires for most sectors. adjusting projected reductions with an assessment of target progress Scope 3 targets were not assessed in this study since scope 3 emis performance determinants and past target achievements. Furthermore, sions for company-specified BY were generally incomplete or inaccu 21% of the 2000 biggest companies in the world have committed to a rate, as also found by Busch et al. (2020), Klaaβen and Stoll (2021), and net-zero target by 2050 (Hale et al., 2022). Since most of these net-zero GRAIN and Institute for Agriculture and Trade Policy, 2018. Blanco et al. targets were not included in this study, it is important to update the (2016) assessed scope 3 emissions disclosed by US companies and found analysis in the upcoming years with a more recent CDP dataset or with that on average, only 22% reported scope 3 values. In this study, only another database. 8% of companies were found to disclose scope 1, 2 and 3 targets. These Additional work to this study has been conducted (see Supplemen results are consistent with previous studies that found scope 3 targets are tary Information) regarding the comparison of benchmarks developed much less common than targets for direct emissions (Gouldson and here to the Paris Agreement goal and to determine if high-emitting Sullivan, 2013; Wang, 2017; Wang and Sueyoshi, 2018). However, companies are planning to reduce more in the coming years compared scope 3 emissions can make up for over 75% of the total company to their peers. Nevertheless, further work is still needed on these topics. emissions (Huang et al., 2009). As such, it is important that more companies disclose scope 3 targets and BY emissions. 4.3. Contributions and recommendations This study is based on reported information from companies which may lack accuracy. One of the main issue concerns BY emissions, which The present study contributes to the scientific literature on corporate may change over time due to mergers and acquisitions or changes in climate mitigation targets. First, the extent and breadth concerning the company emissions coverage. The third criteria in Section 2.1.2 was number of companies considered has made it the largest study on the designed to minimize this issue. Additional data accuracy criteria should matter, with 2607 companies being assessed. Second, the methodolog be developed to prevent such problems in future studies. In 26% of ical approach allows the harmonization of targets with respect to scope, cases, companies did not report BY emissions corresponding to their timeframe, and target metrics, to produce a uniform and coherent target in the same questionnaire where they report their target. Com analysis structure. A comparison with Wang and Sueyoshi (2018) shows panies should report the same BY used for their target in the ‘Emissions that annualizing reductions from a company-specified BY to the TY can Methodology’ section of the CDP Questionnaire. In our study, 91 com overestimate the percentage of reduction, as reductions occurring before panies were excluded due to data mismatch between the ‘Targets’ and the target is launched are also integrated. This reveals the importance of the ‘Methodology’ sections in the 2018 CDP questionnaires. Therefore, a common BY in the development of benchmarks based on corporate companies should update their BY emissions in order to match the in climate mitigation targets. Third, target progress results indicate the formation reported in the ‘Targets’ section of the CDP questionnaires. relevance of tracking such indicators at the common BY and a target Since no information was given on the reduction trajectory of re start year to determine poor ambition or greenwashing. ported targets, a linear reduction was assumed when developing Results obtained from the study have produced insights leading to benchmarks as done in previous studies (see Section 4.1). The integra recommendations for practitioners such as companies, investors, and tion of intermediary targets as done in Step 4a help to diminish conse governments. Companies need to improve the quality of their GHG quences of this assumption. Accordingly, companies should report emissions and target data reporting. To track target ambition more intermediary targets when they have long-term targets. Ideally, com accurately and avoid linear reduction trajectory assumptions, com panies should give more information on their reduction trajectory to panies should report more intermediary targets. Investors and govern avoid the need for assuming a linear pathway. Since no information was ments should pressure companies to do so in order to maximize the provided on emissions not covered by targets, these emissions were quality and accuracy of analysis results. assumed to stay constant over time, following Dietz et al. (2021b). To For investors, a sectoral perspective should be taken when reduce uncertainties on benchmarks, companies should report addi comparing the ambition of corporate climate mitigation targets. Sectoral tional information on the expected trajectory of these emissions. results from this study can help investors assess companies ambition An important limitation of economic intensity benchmarks devel compared to their peers in order to apply a best-in-class responsible oped in this study is high sensitivity to the extrapolation method used for investment strategy. These results can also be useful for an engagement economic values i.e., average annualized differences ranging from and voting responsible investment strategies by giving more suitable 1.87% to 3.38% were observed depending on the statistical metric and and achievable targets coming from best-in-class peers for lagging benchmark (see Section 3.4.1). Unfortunately, no relevant studies were companies. Investors should also track target progress through time and found to allow a comparison. Nevertheless, intensity-metrics are often engage for more ambitious reductions once companies have achieved used by investors to perform portfolio GHG footprint assessments. their target, since 22% of companies kept their target active even if they Extrapolation methods used for long-term economical values should be were already achieved. To identify poor ambition and greenwashing, decided by the investor as different forecasting methods may be investors should also assess the target progress at the start year, since preferred from one investor to another for various reasons. 14% of companies have achieved their target before announcing them. The present study shows the limitations of relying on CDP data for These results can also help investors better understand their position to developing benchmarks for intensity-based reduction targets, given its avoid financial climate risks or to determine their own reduction tra lack of physical production data and standardized measures. Only the jectory based on corporate climate mitigation targets. However, not all power generation sector had enough intensity targets with similar companies report a target. For such cases, a precautionary approach as physical units or production data available to surpass 20 companies. suggested by Hoepner and Rogelj (2021) should be taken to estimate the Therefore, other information sources such as corporate sustainability trajectory. reporting should be used to complement CDP questionnaires where For governments and policymakers, the results can help to better possible. However, physical intensity metrics can only be applied to understand how much could be achieved through corporate climate homogeneous sectors (Koellner et al., 2005). mitigation targets if realized. However, the reduction resulting from The benchmarks developed here can be used to compare companies’ corporate climate mitigation targets will not be sufficient to respect stated emission reduction intentions with those of peers. However, national mitigation targets (Kuramochi et al., 2020). Nevertheless, un companies do not always achieve their targeted emission reductions. For derstanding where sectors are projecting themselves can be useful to example, considering that the materials industry contains “hard to policymakers to know where more efforts and regulations are required. abate” emissions (e.g., from cement and steel production), it is not a In supplementary information, the annualized reduction rates of firms given that the relatively high abatement ambition of that sector will be aggregated per countries are valuable information for comparing firms realized. More advanced models could alleviate this problem by from one country to another. For example, Canadian firms have the 11 A.-F. Bolay et al. Journal of Cleaner Production 376 (2022) 134220 lowest annualized average reduction rate amongst developed countries Bjorn, A., Lloyd, S., Matthews, D., 2021. From the Paris Agreement to corporate climate commitments: evaluation of seven methods for setting “science-based” emission in 2025 and 2030. Monitoring target progress from corporate climate targets. Environ. Res. Lett. 16 https://doi.org/10.1088/1748-9326/abe57b. mitigation targets is also important, considering that 32% of companies Blanco, C., Caro, F., Corbett, C.J., 2016. The state of supply chain carbon footprinting: have increased their absolute emissions since setting their reduction analysis of CDP disclosures by US firms. J. Clean. Prod. 135, 1189–1197. https://doi. targets. org/10.1016/j.jclepro.2016.06.132. Bloomberg, L.P., 2020. Market Capitalisation and Enterprise Value for Company 01/01/ 1990 to 01/01/2019 [WWW Document]. URL Bloomberg terminal (accessed 5. Conclusion 8.7.21). Boiral, O., Henri, J.-F., Talbot, D., 2012. Modeling the impacts of corporate commitment on climate change. Bus. Strat. Environ. 21, 495–516. This study is the largest study establishing benchmarks based on Bolton, P., Despres, M., Awazu, L., Da, P., Samama, F., Svartzman, R., 2020. The Green corporate climate mitigation targets by assessing 2607 companies. After Swan: Central Banking and Financial Stability in the Age of Climate Change. Bank the target data preprocessing step, the final sample included 964 com for International Settlements. Busch, T., Weinhofer, G., Hoffmann, V.H., 2011. The carbon performance of the 100 panies for absolute GHG emission benchmarks, 868 for the economic largest US electricity producers. Util. Pol. 19, 95–103. https://doi.org/10.1016/j. intensity benchmark per revenue, 771 for the economic intensity jup.2010.12.002. benchmark per enterprise value, and 32 for the power generation Busch, T., Johnson, M., Pioch, T., 2020. Corporate carbon performance data : Quo vadis. J. Ind. Ecol. 1–14. https://doi.org/10.1111/jiec.13008. physical intensity benchmark. Byrd, J., Bettenhausen, K.L., Cooperman, E.S., 2013. Context-based sustainability and Results confirm the importance of taking a sectoral perspective when corporate CO2 reduction targets : are companies moving fast enough? Int. Rev. determining or comparing target ambitions. The methodological Account. , Bank. Financ. 5. Callery, P.J., Kim, E.-H., 2021. Moving targets: aggressiveness, attainment, and temporal approach consisting of harmonizing every target to the same scope, dynamics in corporate carbon targets. Acad. Manag. Proc. 2021, 10873 https://doi. timeframe, and target metric show the importance of having a common org/10.5465/ambpp.2021.10873abstract. base year to avoid overestimating the percentage of reductions by Campiglio, E., Dafermos, Y., Monnin, P., Ryan-collins, J., Schotten, G., Tanaka, M., 2018. Climate change challenges for central banks and financial regulators. Nat. Clim. considering those occurring before the target was launched due to Change 8, 462–468. https://doi.org/10.1038/s41558-018-0175-0. company choice of target base year. Carney, M., 2015. Breaking the Tragedy of the Horizon – climate change and financial Furthermore, the percentage of reductions should not be the only stability. In: Speech Given by Governor of the Bank of England, pp. 1–16. metric considered. Target progress is an important parameter to assess CDP, 2018. CDP Academic Data Package (2010 to 2018) [WWW Document]. URL. https://www.cdp.net/en/data/corporate-data#84637b799821f4e01a65f96 potential greenwashing or poor ambition. In 2017, 22% of companies 37ecb3548. had achieved their most long-term target, 46% have reduced their ab Climate Action 100+, 2019. 2019 Progress Report. solute emissions compared to the company-specified base year, and 32% Dafermos, Y., Nikolaidi, M., Galanis, G., 2018. Climate change , financial stability and monetary policy Climate change , financial stability and monetary policy. Ecol. Econ. have increased their absolute emissions since. Substantial sectoral dif 152, 219–234. ferences occurred in those rates. Nevertheless, 14% of targets were Dahlmann, F., Branicki, L., Brammer, S., 2017. Managing carbon aspirations: the achieved before the target was even announced. influence of corporate climate change targets on environmental performance. J. Bus. Ethics 158. https://doi.org/10.1007/s10551-017-3731-z. Although the results of this study have produced valuable contri Day, T., Mooldijk, S., Smit, S., Posada, E., Hans, F., Fearnehough, H., Kachi, A., butions, further work should be performed to develop more advanced Warnecke, C., Kuromochi, T., Hohne, N., 2022. Corporate Climate Responsibility models including a readjustment of corporate climate mitigation target Monitor 2022. Delmas, M.A., Burbano, V.C., 2011. The drivers of greenwashing. Calif. Manag. Rev. 54, reductions based on an assessment of target progress determinants and 64–87. past target achievements to increase the accuracy and applicability of Dessaint, O., Matray, A., 2017. Do managers overreact to salient risks? Evidence from such benchmarks. hurricane strikes. J. Financ. Econ. 126, 97–121. Dietz, S., Bowen, A., Dixon, C., Gradwell, P., 2016. Climate value at risk of global financial assets. Nat. Clim. Change 6, 676–679. https://doi.org/10.1038/ CRediT authorship contribution statement nclimate2972. Dietz, S., Fruitiere, C., Garcia-manas, C., Irwin, W., Rauis, B., Sullivan, R., 2018. An assessment of climate action by high-carbon global corporations. Nat. Clim. Change Anne-France Bolay: Conceptualization, Methodology, Formal 8, 1072–1075. https://doi.org/10.1038/s41558-018-0343-2. analysis, Investigation, Visualization, Writing – original draft, Writing – Dietz, S., Bienkowska, B., Gardiner, D., Hastreiter, N., Jahn, V., Komar, V., Scheer, A., review & editing. Anders Bjørn: Writing – review & editing, Supervi