PR Lectures_Class II.pptx
Document Details
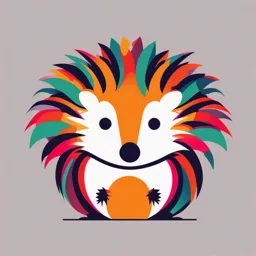
Uploaded by mxrieen
CSJMU Kanpur, India
Full Transcript
Metabolic Flux Anaysis (MFA) Type of Metabolic Pathways Branched pathway A primary module of metabolic networks is the branched pathway which, together with the linear pathway, constitutes the major building blocks of most metabolic networks. The splitting ratios of branching fluxes at key nodes...
Metabolic Flux Anaysis (MFA) Type of Metabolic Pathways Branched pathway A primary module of metabolic networks is the branched pathway which, together with the linear pathway, constitutes the major building blocks of most metabolic networks. The splitting ratios of branching fluxes at key nodes essentially determine the overall distribution of metabolic fluxes in most biological systems. Fig. Branched pathway models. Four types of branched biosynthetic pathways consisting of three metabolites: (a) simple irreversible branched pathway; (b) branched pathway with a reversible reaction (ν2); (c) branched pathway with feedback inhibition; and (d) branched pathway with feedback inhibition and crossover activation. Metabolite Balancing Stoichiometric matrix for Metabolite Balancing in MFA • Stoichiometric modeling framework is mostly characterized by MFA where a set of measured extracellular metabolite concentration rates of change are fitted to relatively simple mass balance models using the stoichiometric mass balance analysis to derive comprehensive metabolic flux maps. • Such quantitative maps have turned out to be extremely useful for comparing the effects of various stressors on metabolism, as they provide a global picture and understanding of the changes in relevant metabolic pathways. The flux distribution is calculated using the basic idea of MFA. The mass balances of all internal metabolites can be written as follows: Where, X is vector of metabolite concentrations, v is the flux distribution vector, and S is the stoichiometric matrix, where rows correspond to the metabolites and columns represent the reaction rates. Stoichiometric matrix for Metabolite Balancing in MFA where rows correspond to the metabolites and columns represent the reaction rates. P Q Fig. A small metabolic network The network includes four internal reactions (v1, v2, v3, and v4) and four external reactions (v5, v6, v7 and v8), which are given as “output” fluxes. The stoichiometric matrix (S) is constructed according to material balance equations. It is generally assumed that the internal metabolites are at a pseudo-steady state, because metabolic transients are rapid compared to environmental changes. Therefore, the mass balance is rewritten as follows: Metabolite Balancing Formulation of an FBA (a) A metabolic network reconstruction consists of a list of stoichiometrically balanced biochemical reactions. (b) This reconstruction is converted into a mathematical model by forming a matrix (labeled S), in which each row represents a metabolite and each column represents a reaction. Growth is incorporated into the reconstruction with a biomass reaction (yellow column), which simulates metabolites consumed during biomass production. Exchange reactions (green columns) are used to represent the flow of metabolites, such as glucose and oxygen, in and out of the cell. (c) At steady state, the flux through each reaction is given by Sv = 0, which defines a system of linear equations. As large models contain more reactions than metabolites, there is more than one possible solution to these equations. (d) Solving the equations to predict the maximum growth rate requires defining an objective function Z = cTv (c is a vector of weights indicating how much each reaction (v) contributes to the objective). In practice, when only one reaction, such as biomass production, is desired for maximization or minimization, c is a vector of zeros with a value of 1 at the position of the reaction of interest. In the growth example, the objective function is Z = vbiomass (that is, c has a value of 1 at the position of the biomass reaction). (e) Linear programming is used to identify a flux distribution that maximizes or minimizes the objective function within the space of allowable fluxes (blue region) defined by the constraints imposed by the mass balance equations and reaction bounds. The thick red arrow indicates the direction of increasing Z. As the optimal solution point lies as far in this direction as possible, the thin red arrows depict the process of linear programming, which identifies an optimal point at an edge or corner of the solution space Figure: Formulation of an FBA problem (Orth et al., 2010, Nature Biotechnology) Tracer Experiments, MS and NMR in labelling measurement • Metabolic flux can be inferred through the use of isotopic tracers along with Mass Spectrometry (MS) or Nuclear Magnetic Resonance (NMR). Stable-isotope-assisted methods like 13C-MFA (Metabolic Flux Analysis) is a gold standard for accurate and precise flux information. • The workflow for 13C-MFA first involves designing an optimal tracer experiment or metabolite labeling that is measured by MS or NMR. • To quantify fluxes at the systems levels i.e., at very large scales the tracer data is synthesized using computational models. That means labeling measurements are fit to a metabolic network model constructed based on large network databases like KEGG or BioCyc. • There are some computational programs that help reach the best global fit for flux estimation and this is followed by statistical analysis to evaluate the fit. This is an iterative process. Based on the result, if the solution is not optimal, the network could be modified or further labeling experiments could be proposed. • Other methods for quantifying metabolic fluxes in biological systems is the Classic MFA without tracer analysis, Flux Balance Analysis (FBA) and a broad range of constraint-based reconstruction and analysis (COBRA) methods. These techniques require no 13C-labeling experiments, so involves fewer experimental data. But, to constrain metabolic fluxes Bibliography de Falco B, Giannino F, Carteni F, et al (2022) Metabolic flux analysis: a comprehensive review on sample preparation, analytical techniques, data analysis, computational modelling, and main application areas. RSC Adv 12:25528–25548. https://doi.org/10.1039/d2ra03326g Emwas AH, Szczepski K, Al-Younis I, et al (2022) Fluxomics - New Metabolomics Approaches to Monitor Metabolic Pathways. Front Pharmacol 13:1–13. https://doi.org/10.3389/fphar.2022.805782 Kwilas AR, Donahue RN, Tsang KY, Hodge JW (2015) HHS Public Access. Cancer Cell 2:1– 17. https://doi.org/10.1016/j.cell.2018.03.055.Metabolomics Nielsen J (2014) Bioreaction Engineering Principles Orth JD, Thiele I, Palsson BO (2010) What is flux balance analysis? Nat Biotechnol 28:245– 248. https://doi.org/10.1038/nbt.1614 https://biochem.web.utah.edu/iwasa/metabolism/chapter5.html Exercise ite the stoichiometric matrix for the given reaction networks. (A) (B)