Trust AI or Gut: 2018 HBR Case Study PDF
Document Details
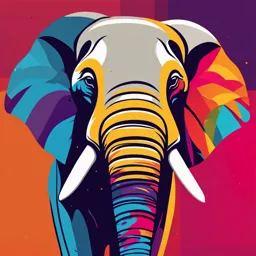
Uploaded by CleanestPopArt3963
New York University
2018
Jeffrey T. Polzer
Tags
Summary
This HBR case study analyzes a VP's dilemma in choosing between a data-driven algorithm and gut feeling in hiring a marketing director. The VP must decide between a highly performing existing employee and a candidate recommended by a new HR algorithm, leading an examination of the strengths and weaknesses of each candidate, the biases of human judgment, and the role of data analytics in organizational decisions.
Full Transcript
CASE STUDY TRUST THE ALGORITHM OR YOUR GUT? A VP DECIDES WHICH CANDIDATE TO PROMOTE. BY JEFFREY T. POLZER Aliyah Jones was having trouble paying attention to the farewell toas...
CASE STUDY TRUST THE ALGORITHM OR YOUR GUT? A VP DECIDES WHICH CANDIDATE TO PROMOTE. BY JEFFREY T. POLZER Aliyah Jones was having trouble paying attention to the farewell toasts. Although she was sad to see her longtime colleague Anne Bank go, she was more consumed JEFFREY T. POLZER is the with trying to figure out who should replace her. UPS Foundation As a VP of sales and marketing for Becker-Birnbaum International, a Professor of Human Resource Management global consumer products company, Aliyah knew she needed a talented in the organizational marketing director to support her division’s portfolio of 34 products. behavior unit at Harvard After working with HR to narrow down the list of candidates, she had two Business School. finalists, both internal: Molly Ashworth, a brand manager on her team in the cleaning division, and Ed Yu, a rising star from BBI’s beauty division. HBR’s fictionalized case studies present problems Aliyah liked Molly and respected her work. Two years earlier, Molly faced by leaders in real had spearheaded a new subscription service for BBI cleaning products, companies and offer which had shown strong growth in the past two quarters. Customers solutions from experts. seemed to love the convenience, and the R&D, marketing, and executive This one is based on the teams had gotten excited about the service as a platform to test new HBS Case Study “Susan Cassidy at Bertram Gilman offerings. Having mentored Molly through the pitch and launch of the International” (case no. CASE STUDY service, Aliyah was intimately familiar with her protégé’s strengths and 417-053), by Jeffrey T. Polzer CLASSROOM NOTES weaknesses and was certain that she was ready for the next challenge. and Michael Norris. Companies use algorithms But soon after the position had been posted, Christine Jenkins, a in people-related decisions corporate VP of HR, had come to Aliyah with Ed’s résumé. Like Molly, for many reasons, including consistency, Ed had joined BBI right out of business school and been quickly tapped reduced bias, casting as a high potential. He also had his own BBI success story: As a brand a broader net, and manager in the beauty group, he had revived its 20-year-old FreshFace efficiency. How might makeup-removal product line, increasing sales by 60% in three years. the recommendations an Perhaps more important to Christine, he’d been recommended as a algorithm makes differ from those of a hiring 96% match for the job by HR’s new people-analytics system, which manager who is not she had championed. (Molly had been an 83% match.) The goal using data analytics? of the initiative was to expand the use of data analytics to human MAY–JUNE 2018 HARVARD BUSINESS REVIEW 147 CASE STUDY TRUST THE ALGORITHM OR YOUR GUT? resources, to inform even his questions seemed a bit stiff. hiring, promotion, Aliyah didn’t sense the dynamism or Does using algorithms for any type of people analytics and compensation entrepreneurial mindset that she knew violate employees’ privacy? decisions. Aliyah was glad to see two Molly had. Maybe he’s nervous, she New laws—in particular, insiders in contention—she’d come up thought. Or maybe that’s just who he is. the EU’s General Data the ranks herself—but that made the Aliyah didn’t doubt Ed could do the Protection Regulation decision harder. job. But she didn’t feel excited (GDPR)—are setting limits on what information As the COO made a toast to Anne, about hiring him. employers can and cannot Aliyah considered her interviews with collect, and how employees Ed and Molly. MOLLY’S “INTERVIEW” must be notified. Setting Molly’s interview MEETING ED YU up for the same day as Ed’s “I’m sorry I’m so late,” Ed said, looking had seemed like a great idea a little discombobulated. “My Uber when she’d suggested it to driver insisted he knew a shortcut from Christine, and given the noon Heathrow—but he was wrong.” time slot, it had been only natural to Aliyah couldn’t help drawing an meet at their usual lunch spot near the Research shows that immediate comparison with Molly, office. But as soon as Aliyah walked into hiring managers typically who was always steady and calm, but the café, she realized how unfair these form opinions about a she tried to keep an open mind. back-to-backs were to Ed. Managers tend to candidate’s personality and “No problem,” she said. “Shall we It was impossible not to hug Molly hire people similar to competence in the first 30 get started?” hello and ask for a quick update on her themselves, studies show. seconds of an interview. For example, Kellogg “Absolutely,” Ed said eagerly. projects and family. They even ordered School of Management “What interests you about the job?” the same thing: curried egg salad. But professor Lauren Rivera Ed explained that while he was as soon as the waitress left, Molly got found that managers prefer proud of the growth FreshFace had seen down to business: “I know we e‑mail recruits who have the under his leadership, he was ready for 10 times a day, but I’d like to treat this most potential to become a new challenge. He’d enjoyed diving as a formal interview.” friends, even over those who are more qualified. deep into one product but felt his Aliyah smiled. “Of course.” Should Aliyah worry that skills were better suited for a position As Christine had advised her to do, she’s choosing Molly that would allow him to work across she asked questions that were the same because she likes her? programs and direct a larger portfolio. or similar to the ones she’d asked Ed. Sharp, clear answer, Aliyah thought. “Tell me why you’re interested in “What have you learned in beauty that this job,” she started. It was awkward. Unstructured interviews would apply in cleaning?” she asked. Aliyah knew the answer already, but are the default method for most hiring managers, This was an important question. to Molly’s credit, she proceeded as if but numerous studies BBI’s top team had directed the they weren’t close colleagues. With have found them to be divisions to share more best practices each response, she demonstrated deep poor predictors of actual and improve collaboration. In fact, her knowledge of the business, and she on-the-job performance. boss wanted her to work more closely had good ideas for collaborating across with her peers in other divisions. programs and building on the success Ed explained how he thought of the subscription program. She was his division’s approach to in-field as polished and thoughtful as Ed, but customer research, which he credited she also seemed warmer and more with boosting FreshFace sales, could self-aware. work in cleaning. Partnering with Knocked it out of the park, Aliyah anthropologists was something thought, as they walked back to the Aliyah’s team had talked about but office. Looking at the smile on Molly’s hadn’t yet tried out. face, Aliyah knew her protégé was He also asked about the feeling confident that she’d done well. new subscription program, referencing a recent THE ALGORITHM white paper on trends The day after Anne’s farewell party, in subscription business Aliyah met with Christine and Brad models. He’d clearly done Bibson, a data scientist on the people his homework, was smart and analytics team. ambitious, knew BBI’s business “I know you were leaning well, and seemed eager to toward Molly after we debriefed the learn. But his answers and interviews,” Christine said, “but we 148 HARVARD BUSINESS REVIEW MAY–JUNE 2018 wanted to share some about the methodology, despite the more data.” companywide training sessions. Brad handed over two colorful “I’d be happy to talk more about how diagrams. “These are network analyses the algorithm works,” Christine replied, of Molly’s and Ed’s e‑mail and meeting “but right now you should focus on the Network analyses can history at BBI. With their permission two candidates. The point of the system reveal patterns that are and without looking at the content of isn’t to replace your judgment. The otherwise hard to see—for their e‑mails or calendars, we analyzed aim is to surface qualified people you example, by identifying who they’d been in contact with across wouldn’t otherwise know about so you which employees are the firm over the past six months.” can make a more informed decision.” most central to informal information flows. It was clear from the diagrams that “It’ll help you make a less-biased Ed was connected to not just his beauty decision too,” Brad chimed in, “by division colleagues but also key people relying more on the data and less on in other groups. Molly’s network was gut instinct.” mainly within cleaning products. Aliyah wondered whether Brad “I didn’t know we were doing this thought she was unfairly favoring kind of analysis,” Aliyah said. Molly. She worried about that herself “We’ve just started looking at and cared deeply about making an networks,” Brad said, “and we think objective decision. Would trusting the they can reveal useful insights.” new system help her do that? “I know one chart isn’t going “But the algorithm’s not completely to sway your decision,” Christine neutral either, right?” she said. said, “but better to have the data, “You’re still relying on information— right? You wouldn’t launch a new performance reviews, résumés—that product or a new campaign without conceivably has bias baked into it.” data. HR decisions should be “Fair point,” Christine conceded, Data scientist Cathy approached the same way.” It “and we’ve worked hard to control for O’Neil warns in her was a pitch that Christine had that. But as a data-driven firm, we have book Weapons of Math made countless times while to extend our approach to the most Destruction that although stumping for the new initiative. important part of our business: people.” algorithms are fairly easy to create using historical “We’re confident that decisions “It feels like you’re pushing Ed for data and can improve made using our algorithms this position,” Aliyah said. the efficiency of decision are reasoned, strong, and less “Remember, I have to take a making, people often biased by personal feelings toward broader view,” Christine said. “We ran rely on them without employees,” she said. analysis to show which high potentials understanding the biases they may be propagating. Aliyah turned to Brad. “I assume are at risk of leaving BBI, and Ed was you agree?” near the top of the list. There is not “Of course,” he said, watching for likely to be an opening in beauty Studies have revealed How does using Christine’s reaction. “But as a data products, and we want to keep him.” algorithms to analyze a phenomenon called “algorithm aversion.” scientist, I also encourage healthy “But what about Molly?” Aliyah customers differ from Even when data-driven skepticism. Our algorithm is brand- said. “She’ll be devastated if she doesn’t using them on employees? predictions yield higher new. We’ve used it to inform three get this job, and I’m sure she’d start Should companies success rate than intuitive be more cautious in promotion decisions so far, but it’s looking too.” human forecasts, people implementing these too early to tell how those people “Our analysis didn’t flag her as a flight methodologies internally? often prefer to rely on the latter. And if they learn are doing. I don’t want to give the risk,” Brad said. “But you could be right.” an algorithm is imperfect, impression that we’re 100% confident.” they simply won’t use it. Christine looked annoyed. “I DECISION TIME Under what conditions appreciate your caution, Brad, but A week later, Aliyah wasn’t any closer to would you base a decision we’ve heard from the hiring managers a decision. She’d been avoiding Molly on data analysis? that the type of recommendations the and had put Brad’s analyses in a drawer. algorithm provides is changing the Ed was clearly qualified, and he’d Along with managers, way they think about positions and impressed her. But she knew intuitively many applicants are candidates. And we’ve been testing the that Molly was ready for the job. skeptical of algorithms, system for months now.” Did she prefer Molly because of their according to Pew. A Aliyah sighed. “I’d trust the close relationship? Would Molly stay at majority of Americans algorithm more if I understood it BBI even if she was passed over? (76%) say they would not want to apply for jobs that better.” She knew she wasn’t alone Aliyah needed to make a use a computer program in her hesitation: Christine’s team decision. Should she trust the SEE COMMENTARIES ON THE to make hiring decisions. had gotten a lot of questions algorithm or her instincts? NEXT PAGE MAY–JUNE 2018 HARVARD BUSINESS REVIEW 149