SIBD Notes PDF
Document Details
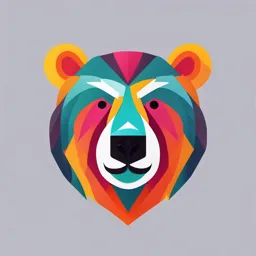
Uploaded by AmenableCobalt7313
Tags
Summary
These notes provide an introduction to the measures of location, also known as measures of central tendency in statistics. The content explains concepts like mean, median, and mode, along with calculation methods for both individual observations and grouped frequency distributions.
Full Transcript
MODULE – 1 Q. What do you understand by measures of location or measures of central tendency? Ans. - We first note that Statistics deals with collection of numerical observations. Any such set of values is usually considerably large in size and hence it is not easy to understand the nature of the...
MODULE – 1 Q. What do you understand by measures of location or measures of central tendency? Ans. - We first note that Statistics deals with collection of numerical observations. Any such set of values is usually considerably large in size and hence it is not easy to understand the nature of the data in respect of the common character of the data that is represented by it. To have a birds’ eye view, the first and the foremost work is to present the data in tabular form. We usually transfer the data in the form of frequency distribution. To be briefer, we need to locate the central point of the data. As the centre of a data can be defined in a number of ways, we have a number of statistical tools to locate the central point of a data and we call each of them a measure of location or a measure of central tendency. Q. What are the requisites of a good measure of central tendency? Ans. – According to Prof. R. A. Fisher, the following are the requisites of a good measure of central tendency: (i) It should be rigidly defined. (ii) It should be easily graspable and easy to calculate. (iii) It should be based on all the observations. (iv) It should be least affected by extreme values. (v) It should be capable of further algebraic treatments. (vi) It should be least affected by fluctuations of sampling. VARIOUS MEASURES OF CENTRAL TENDENCY Or MEASURES OF LOCATION MEAN The mean of a data is defined as the arithmetic average of the given set of observations. Thus if we have n observations X 1, X2, X3, - - - - - Xn, then their mean is given as Mean = 1 /n (X1 + X2 + X3 + - - - - - + Xn). On the other hand, in case of frequency distribution, we have, Mean = 1/ N ∑ f i X i , where X i denotes value or mid-value of class interval, fi is frequency and N = ∑ f i. MEDIAN The median of a data is defined as the middle-most value in the given set of observations. Thus for individual observations, Median = ( n + 1) th value, when n is an odd number 2 = 1.n. th value +.n. + 1 th value , when n is an even number. 2 2 2 For grouped frequency table, when we are supplied with the values of variable and their corresponding frequencies, calculation of median needs the following steps: Step-I → The less than cumulative frequencies of the values are firstly computed. Step-II → The value of N = ∑ f i = sum of the frequencies is computed and then N / 2 is also computed. Step-III→ The cumulative frequency just including (N / 2) is computed and the corresponding ‘value’ is taken as median. For frequency distribution in the form of class intervals, the following steps are needed for the calculation of median: Step-I → The less than cumulative frequencies of the class-intervals are firstly computed. Step-II → The value of N = ∑ f i = sum of the frequencies is computed and then N / 2 is also computed. Step-III→ The cumulative frequency just including (N / 2) is computed and the corresponding class-interval is taken as median class of the data. Step-IV→ Median of the data can now be computed by using the following formula Median = l + h N –C f 2 where, l = lower limit of median class, h = magnitude of median class, f = frequency of median class, C = cumulative frequency of the class just preceding median class. MODEg Mode of a data is defined as the most frequent value in the data. Thus for individual observations, mode is that value in the data that occurs the largest number of times. For grouped frequency table, when we are supplied with the values of variable and their corresponding frequencies, the ‘value’ corresponding to the largest frequency is taken as mode of the data. For frequency distribution in the form of class intervals, we first locate the largest frequency and call the corresponding class-interval as modal class. Now mode is computed by the following formula Mode = l + ( f 1 – f o ) h 2 f 1–f o–f 2 where, l = lower limit of modal class, h = magnitude of modal class, f1 = frequency of modal class, fo = frequency of the class just before modal class, f2 = frequency of the class just after modal class. GEOMETRIC MEAN (G. M.) The geometric mean of a given set of n observations is defined as the nth root of the product of n observations. For individual observations, the G. M. of n observations X1, X2, X3, - - - - - - - Xn, denoted by G, is given by G. M. = Antilog [ 1 / n ∑ log X i ] For frequency distribution, the geometric mean G is given by G = Antilog [ 1 / N ∑ log X i ], where N = ∑ f i and X i = value or mid-value of class interval HARMONIC MEAN ( H. M.) The harmonic mean of a data is defined as the reciprocal of the average of reciprocals of the values. Thus for individual observations, harmonic mean H of n values X1, X2, X3, - - - - - - Xn is given by 1/ H = 1/ n ( 1/X) For frequency distribution, ( X i / f i ) , the harmonic mean H is given by 1/ H = 1/ N ( f /X) MEASURES OF DISPERSION Introduction: - Before explaining the measures of dispersion, we first note that any measures of central tendency gives us a rough idea regarding the size of values in the given data. But this is not enough, especially when we need to compare two or more set of observations. The very next step in the direction of knowing the nature of the data is to have some idea regarding the variations in the values of the given data. We have a number of statistical formulas meant to explain the nature of any given data in terms of variations among the values and we call these as the measures of dispersion or the measures of variation. RANGE This is the simplest measure of dispersion and is rarely used. The range of a data is defined as the difference between the largest and the smallest value in the given set of observations. Thus, Range = L – S, where L is the largest observation and S, the smallest observation in the given data. INTERQUARTILE RANGE AND QUARTILE DEVIATION This measure of dispersion is based on quartiles. In usual notations, if Q1 and Q3 denote respectively the lower and upper quartiles of any given data, then we define the following: INTERQUARTILE RANGE = Q3 – Q1 SEMI INTERQUARTILE RANGE = QUARTILE DEVIATION (Q. D.) = ½ (Q3 – Q1) MEAN DEVIATION ( M. D.) OR AVERAGE DEVIATION ( A. D.) The mean deviation of a data about a value A is defined as the average of absolute values of deviations of observations from the value A. Thus for individual observations X1, X2, X3, - - - - - -, Xn, the mean deviation about A is given as, Mean Deviation (M. D.) about A = 1/n | X i – A |. For frequency distribution, M. D. about A = 1 / n f i | X i – A | , where notations have their usual meanings. STANDARD DEVIATION ( S. D.) The standard deviation of any given set of values is defined as the positive square root of the average of squares of deviations of observations from their mean. Thus for individual observations X1, X2, X3, - - - - , Xn, we have, S. D. = 1/ n ( X i – X ) 2 , where X = mean of the given observations. For frequency distribution, S. D. = 1 / n f i ( X i – X )2, where N = ∑ f i and X = mean of given data. We now enumerate some more measures of dispersion: MODULE – 2 Bivariate data:- For any given group of similar units, having two common characters, if observations are taken in respect of each of the two characters, then on each unit, we get a pair of observations, one observations in respect of each character. The collection of all such pairs of observations for the entire group is taken as a bivariate data. SCATTER DIAGRAM Introduction: - This is a diagrammatic representation of any given bivariate data and provides us a rough idea regarding co- variation of two variables of the given bivariate data. To plot this diagram for any given bivariate data, we need to consider the values or measurements in respect of the two variables along the two axes of co-ordinates. If X, Y denotes the two variables of the bivariate data, usually these are to be considered along horizontal and vertical axes respectively. Now corresponding to each pair of observations in the bivariate data, we can plot a point on the graph paper. Plotting points for all the pairs of values, we get a diagram of points, called scatter diagram of the data. CORRELATION Introduction: -The term correlation simply means ‘co-variation’. In fact this term is used in studying any given bivariate data. Under correlation analysis of a bivariate data, we need to get answers to the following questions: (i) The two variables in the bivatiate data are changing in the same direction ot in opposite directions? (ii) What is the ratio of such change? To get answers to the following questions, we make use of a statistical tool named after its inventor as Karl Pearson’s coefficient, which is denoted by r (X,Y) and has been defined as r (X,Y) = cov ( X, Y ) √ σx2. σy2 Where, cov (X, Y) = 1/ n ∑ (X – X ) (Y –Y ), σx2 = 1/ n ∑ ( X – X ) 2, σy2 = 1/ n ∑ ( Y – Y ) 2, X = 1/ n ∑ X , Y = = 1/ n ∑ Y. RANK CORRELATION Introduction: -Before explaining the concept of rank correlation, we first note the fact that under simple correlation we need to consider two such characters of a group of units, which can be measured quantitatively. But we sometimes need to consider certain qualitative characters in a bivariate study. In this case we cannot have numerical observations in respect of the two characters. In such a situation we make use of the concept of what we call as ‘Rank’. In fact, on the basis of a particular qualitative character a given group of units can be positioned as having ranks 1,2,3, -----. If the units are allotted ranks in respect of each of the two characters then we have a pair of characters for each unit in the group. The simple correlation between such pairs of ranks is taken as rank correlation. Measurement of Rank correlation: - It is interesting to note here that rank correlation can be measured by Karl Pearson’s coefficient of correlation, however there is a formula named after its inventor as Spearman’s formula for rank correlation. This formula is given as: 6 S di 2 r ( C, U ) = 1 - n3 – n Where, r ( C, U ) denotes rank correlation coefficient between characters X and Y, di = Xi -Ui, Xi and Yi being ranks of i th unit in respect of characters X and Y, n = number of pairs of ranks. REGRESSION ANALYSIS Introduction: - The term regression literally means ‘stepping back towards the average’. More clearly, under regression analysis, we present average or approximate relationship between two or more variables of a bivariate data. Such a relation is usually expressed in the form of some equation and we call it the regression equation of the given bivariate or multivariate data. In case of bivariate data, the regression equation is usually in the form of equation of a straight line and we call it the regression line. Such a regression line is the line of best fit to the scatter diagram of the bivariate data. Here the principle of least squares is used to minimize the sum of squares of deviations of the values from their estimates. Such deviations are taken either along X-axis or along Y- axis and consequently we have two regression lines called respectively as ‘line of regression of X on Y’ and ‘line of regression of Y on X’. MODULE - 3 Time-series: - A set of observations arranged in chronological order is called a ‘time-series data’ or simply a time-series. In other words, a time-series is an arrangement of observations in respect of time. Some common examples of time-series are (i) Yearly production of steel of certain steel plant. (ii) Daily sale of tickets at a particular cinema hall. (iii) Weekly payments to workers by a contractor. Components of a time-series: - Before explaining the components of a time-series, we first note that the most common kind of data is what we call a cross-sectional data, in which observations are taken from different units at the same point of time. The measures of locations or central tendency and measures of dispersion, etc. reflect the nature of such data. But these measures fail to throw light upon the nature of a time-series. We would now discuss the way of studying a time-series. A time- series is usually seen to vary in some irregular manner. Such variations in a time- series are considered to be caused by several forces, some of which obey certain regular pattern whereas there are certain irregular forces too. These forces of variations are called components of a time-series. The following are the components of a time-series. 1. Trend or secular trend, 2. Periodic variation; further classified as [A] seasonal variations and [B] cyclical variations. 3. Irregular variations. Trend or secular trend:- The term ‘trend’ means the long-term general tendency of a time-series to increase, decrease or to remain almost unchanging. Thus trend of a time-series provides a rough idea regarding the nature of the series. In forecasting future values, trend plays a vital role. Periodic variations: - Certain rhythmic or periodic forces cause part of variations in a time-series. Such forces are seen to repeat their effects after almost a fixed interval of time. This interval may be of a few days, a few weeks or months or several years. Variations in a time-series due to such periodic forces are called periodic variations and have been classified as: (i) Seasonal variations: - Periodic variations in a time-series due to natural seasons or certain man made conventions like festivals, vacations, marriage seasons, etc. are taken as seasonal variations. One more important character of such variations is that the period of variations is always less than a year. (ii) Cyclical variations: - Periodic variations in a time-series not caused by natural seasons or man made conventions, and having a considerably large period of almost 8 –12 years are called cyclical variations. This kind of periodic variations in a time-series is assumed to be caused by so called ‘business cycles’. Irregular variations: - Apart from all the above-mentioned forces of variations in a time-series, certain part of variations still remains to be explained. This happens because of a component, which we call irregular component and this component is likely to affect almost all the time-series data. Such variations in a time-series are caused by accidental reasons like flood, drought, war, earthquake, strike, etc. Analysis of time-series: - We note that the nature of a time-series can be explained by its components, namely, trend, seasonal component, cyclical component and the irregular component. The time-series values of a given data are expressed in terms of some mathematical model of suitable kind. The kind of model to be considered depends upon the prevailing situation. We usually considered multiplicative model as the components of a time- series are of multiplicative nature. But we sometimes make use of additive model or a mixed model as well, if the situations compel us. The next step here is to measure the components of the given time-series by suitable methods, whenever possible. Some components can be measured directly, whereas some can be measured only by certain indirect method. In particular, the irregular component cannot be measured by any direct or indirect method, however its effect can be almost eliminated by certain available methods. The above mentioned works of firstly expressing a given time-series by a suitable model and then providing measurements of its components are taken as analysis of time-series. Thus analysis of time-series provide us an idea regarding its nature and this idea is of much use, especially for forecasting purposes. Measurement of components of a time-series I. Measurement of trend: - The following are the four usual methods of measuring trend: (i) Graphical or Free hand method: - This method of measuring trend is quite simple, but at the same time, it is perfectly subjective. So it is not of much practical utility. Under this method, the actual time-series is firstly plotted on a graph paper. Next, a free hand smoothing of the above graph is carried out and the resulting estimates are taken as trend values in this case. (ii) Method of moving averages: - This method of measuring trend consists in finding averages of successive groups of time-series values and plotting these averages against the centres of the periods to which the values correspond. To explain this method more clearly, let the period of moving average be m. Then the following steps are to be followed: Step 1: -The given time-series data is arranged in a tabular form writing points of time in the very first column and corresponding time-series values in the 2nd column. Step 2: -The total of first m time-series values is computed and written in a fresh column against the centre of these m values. Next the total of m time-series values starting from 2 nd to [m+1]th value is computed and written similarly on this very third column. This process of computing and entering the totals of m values in column three is carried out up to the last group of m values. The entries in third column are called m point moving totals. Step 3: - Each moving total is now divided by m to get corresponding moving averages and are written in 4 th column. These m point moving averages are taken as moving average trend values. Step 4: - This step is meant only for the case when the period of moving average is even. In this case, the average of first two moving averages is computed and is written in the middle of these two entries in a fresh column, i. e. column number five. Then the average of second and third entries of 4 th column is computed and written likewise in 5th column. This process is repeated till the last pair of values of 4 th column. These entries in the 5th column are called centered moving averages. Step 5: - The moving averages are plotted on the same graph paper on which actual time-series is plotted. Joining these moving averages by free hands, usually by a dotted curve form, we get the moving the actual time-series values. (iv) Method of least squares: - This method of measuring trend of a time-series is based on the principle of least squares. This principle is used here to minimize the sum of squares of deviations of observations from their estimates. This minimization is achieved by applying the method of Maxima and Minima of Calculus, which gives a number of equations in terms of the parameters of the line or curves to be fitted and we call these ‘normal equations’. It may be noted here that the number of normal equations must be equal to the number of parameters to be estimated, as the derivatives to be equated to zero are obtained with respect to each unknown parameter; a simultaneous solution of all these normal equations provide the estimates of unknown parameters. Using these estimates, the equation of line or curve representing the time-series in terms of time is obtained. This equation of line or curve is now used to obtain trend value in respect of each point of time. These trend values are finally plotted on a graph paper along with The regular components of a time-series like trend, seasonal fluctuations, cyclical fluctuations can be measured directly or indirectly, but the random component cannot be measured, even its variance cannot be measured. We now discuss the methods of measuring various components of a time-series. Fitting of straight line: - If there be n pairs of observations on two variables, say X and Y, then the equation of straight line to be fitted to this data is of the form Y = a + b X , where a and b are the two parameters to be estimated by the principle of least squares. The normal equations to be use here are: Y = n a + b X and XY = a X + b X 2. Solving the above two equations simultaneously, we can obtain the values of a and b and thus the equation of the straight line will be obtained. Using this equation, the estimates of Y for given values of X can be estimated. Fitting of second degree curve or parabola: - If there be n pairs of observations on two variables, say X and Y, then the equation of second degree curve to be fitted, also called parabola, is of the form Y = a + b X + c X 2, where a, b and c are the three parameters to be estimated by making use of the principle of least squares. The normal equations to be used in this case are given below: Y = n a + b X + c X 2, XY = a X + b X 2.+ c X 3 and X 2 Y = a X 2 + b X 3.+ c X 4. II. Measurement of seasonal variations Method of simple averages: - This method of measuring seasonal component is quite simple. The method can be explained in terms of the following steps: Step 1: - The given data is written in tabular form writing years and seasons, one along rows and the other along columns. Step 2: - The totals and averages for entries for different years of each season is computed and is written against each season. Step 3: - Average of all seasonal averages obtained in step 2 is computed Step 4: - Seasonal index for each is computed by using the following formula Seasonal index for a particular season = Average of the season x 100 Average of seasonal averages Step 5: - The seasonal indices for all seasons must come to be a total of 400 in case of quarterly data and 1200 in case of monthly data. This sum is usually not seen to come to the above totals, so an adjustment is done at this step. The seasonal indices are multiplied by a factor to get adjusted seasonal indices. This factor is ‘400 total of seasonal indices’ for quarterly data and similarly for monthly data, the factor is ‘1200 total of seasonal indices’. Index numbers Under the subject matter of economics, we come across certain variables which we call economic variables. Examples of such variables are prices of commodities, demands or supplies of commodities in a market, cost of living of persons of a certain class of people, etc. Each of such variables is seen to vary with passage of time, but the changes in any such variable as seen directly may not be taken to be the change in actual change as it is a result of economic forces working at that time. So we must study any such change only after evaluating it on the basis of prevailing economic system. The Statistical tool called ‘Index Number’ is meant to serve this very purpose. More clearly, index numbers may be considered as statistical devices meant to measure the effect of economic pressure on the change some economic variable and hence sometimes index numbers are called economic barometers. We now also note the fact that the measure of actual change in any economic variable needs two points of time and we call these as ‘base period’ or ‘base year’ and ‘current period’ or ‘current year’. The point of time of study of such change is termed as current whereas the previous point of time with respect of which the comparison is being made is taken to be current. One more point to note here is that the study of such change is usually done for certain group of commodities taken together. The reason behind this is that due to nature of use as well as taxation patterns etc. the prices of commodities in certain groups are seen to vary in almost the same pattern. Certain common examples of such groups are ‘cereals’, ‘cosmetics’, ‘petroleum products’, ‘stationeries’, etc. The notations in the context of index numbers are as follows: In general, the base year denoted by suffix ‘0’ and current year denoted by suffix ‘1’ and the index number with base year ‘0’ and current year ‘1’ is usually denoted by I 01. On the similar manner the price index with base year ‘ 0’ and current year ‘1’ is denoted by P01 , whereas the quantity index number with the same base year and current year is denoted by Q 01. Important formulae for Price indices Simple (unweighted) Aggregative method: - In this case the Price index number for base year denoted by suffix 0 and current year by suffix 1 is given by P01 = ∑p1 x100 ∑p0 Simple Average of price relatives: - In this case the Price index number is given by P01 = (∑P) / n, where n = number of items and P = Price relative = p1 x 100 p0 Weighted Aggregative method: - In this case the Price index number is given by P01 = ∑p1 w x100 , where w denotes weight ∑p0w Weighted Average of price relatives: - In this case the Price index number is given by P01 = (∑Pw) / ∑w Lespeyre’s method: - In this case the Price index number is given by P01 = ∑p1 q0 x100 ∑p0 q0 Paasche’s method: - In this case the Price index number is given by P01 = ∑p1 q1 x100 ∑p0 q1 Fisher’s method: - In this case the Price index number is given by P01 = 100 ∑p1 q0 ∑p1 q1 √ ∑p0 q0 ∑p0 q1 MODULE – 4 PROBABILITY THEORY. {It is a measure of certainty in the sphere of uncertainty} Introduction: - The concept of probability can be developed by noting the fact that there are several kinds of experiments and we broadly classify them into the following two categories: (i) Deterministic experiments: - Under such experiments, the result is unique and can be predicted in advance. Almost all physical and chemical experiments may be taken as examples of such experiments. (ii) Non-deterministic / Probabilistic / Random experiments: - The result of this kind of experiment is not unique; rather it is one of the several possible results and cannot be predicted in advance. Tossing a coin, throwing two dice, selecting four cards from a well-shuffled pack of cards, etc. are some common examples of random experiments. Some useful terms 1. Random experiment: - If an experiment is repeated under essentially identical and homogenous conditions but the result is not unique rather it is one of the several possible results, it is called a random experiment. 2. Trial: - Performing a certain random experiment is taken as a trial. Thus throwing a die or tossing two coins, etc. are some common examples of trial. 3. Event: - Particular result of a certain random experiment is taken as an event. Thus in a single die throwing experiment, getting 5 or getting an even number are some examples of event. 4. Sample space: - The set of all possible outcomes (results) of a certain random experiment is taken as sample space and is usually denoted by S. Thus in tossing three coins, the sample space is given by S = {(TTT), (TTH), (THT), (HTT), (THH), (HTH), (HHT), (HHH)}, where H and T denotes respectively the occurrence of head and tail. 5. Simple event: - An event that specifies a single point in the sample space is taken as a simple event or an elementary event. Thus in single die throwing experiment, getting 3 is a simple event. 6. Compound event: -An event that specifies two or more points in the sample space is taken as a compound event. 7. Exhaustive cases: - The totality of all events in a certain random experiment are taken as exhaustive events. 8. Favourable cases: - The cases favouring the occurrence of an event are taken as favourable cases for the event. 9. Mutually exclusive events: - Several events of a certain random experiment are said to be mutually exclusive, if happening of any one of them precludes the happening of all other events. 10. Equally likely events: - Several events of a certain random experiment are said to be equally likely, if taking into consideration all relevant evidences, there is no reason to expect one in preference to the other. 11. Independent events: - Several events of a certain random experiment are said to be independent, if happening or non-happening of any one of them is not affected by the supplementary knowledge regarding happening or non- happening of any number of the remaining events. 12. Probability of an event: - (Classical / Mathematical / a priory definition): The probability of an event is defined as the ratio of ‘the number of cases favourable to the event’ to ‘the total number of mutually exclusive and equally likely cases’. The probability of an event E is usually denoted by P(E). Thus we have the formula P(E) = n(E) , where n(E) = number of cases favourable to E and n(S) n(S) = total number of mutually Connection of Probability Theory with Set Theory If A, B, C denote any three events connected with certain random experiment and S denotes the sample space, then A, B, C are denoted by three sets and S is taken as the universal set. Hence the sets A, B, C may be considered as subsets of S. The following connections of Set Theory with Probability Theory are important: Complementary event of A is A At least one of the events A or B occurs Þ w Î A È B Both the events A and B occur Þ w Î A Ç B Neither A nor B occurs Þ w Î A Ç B Event A occurs but B does not Þ w Î A Ç B Event B occurs but A does not Þ w Î A Ç B Exactly one of the events A or B occurs Þ w Î [( A Ç B ) È ( A Ç B )] Not more than one of the events A or B occurs Þ w Î [( A Ç B ) È ( A Ç B ) È ( A Ç B )] If event B occurs so does B Þ AÌ A Events A and B are mutually exclusive Þ A Ç B = f Exactly one of the events A, B, C occurs Þ w Î [( A Ç B Ç C ) È ( A Ç B Ç C ) È ( A Ç B Ç C )] Exactly two of the events A, B, C occurs Þ w Î [( A Ç B Ç C ) È ( A Ç B Ç C ) È ( A Ç B Ç C )] More than one of the events A, B, C occur Þ w Î [(A Ç B Ç C)È(A Ç B Ç C) È(A Ç B Ç C) È(A Ç B Ç C)] Theorems on Probability. A general rule: - If A and B be any two mutually exclusive events connected with certain random experiment, then P(A B) = P(A) + P(B). Theorem 1: - If S denotes the sample space, then P(S) = 1. Proof: - We know that the probability of an event E is defined as P(E) = n(E) , n(S) where, n(E) = number of cases favourable to E and n(S) = total number of mutually exclusive and equally likely cases. Thus, we have P(S) = n(S) = 1 and hence the result. n(S) Theorem 2: - If denotes the impossible event, then P() = 0. Theorem 3: - If A denotes non-happening of the event A, then P( A ) = 1 – P(A). Theorem 4: - To prove that P(A B) = P(A) – P(A B). P(A B) = P(A) – P(A B) and hence the theorem. Theorem 5: - To prove that P(A B) = P(B) – P(A B). Theorem 6: - (Addition theorem for two events) Statement: - For any two events A and B connected with a certain random experiment, P(A B) = P(A) + P(B) – P(AB). Theorem 7: - (Addition theorem for three events) Statement: - For any three events A, B and C connected with a certain random experiment, P(A B C) = P(A) + P(B) + P(C) – P(A B) – P(A C) – P(B C) + P(A B C). Theorem 8: -Multiplication theorem on probability. Statement: - For any two events A and B connected with a random experiment (i) P(A B) = P(A). P(B / A) (ii) P(A B) = P(B). P(A / B) Theorem 9: - Baye’s theorem. Statement: - If E1, E 2, E 3, - - - - - -, E k be k mutually exclusive and exhaustive events and A be an event that can occur in combination with any E i, then P(E i / A) = P(E i) P(A / E i) , where summation ranges from 1 to k. P(E i) P(A / E i) Random variable: - Roughly speaking, random variables are real numbers associated with the outcomes (results) of a certain random experiments. More precisely, a random variable is some real valued function defined on sample space of all outcomes of a certain random experiment. Kinds of random variables 1. Discrete random variable: - A random variable is said to be discrete if its values can be put in one-to-one correspondence with the set of all natural numbers. 2. Continuous random variable: - A random variable is said to be continuous if can assume infinitely many values between certain limits. 3. Probability function of a random variable: - A mathematical rule which can yield the probability of each possible value of a random variable is called probability function of that random variable. Note: - The probability function of a discrete random variable X is called probability mass function and is usually denoted by p(x). On the other hand the probability function of a continuous random variable X is called probability density function and is usually denoted by f(x). For any discrete or continuous random variable, we must have, total probability = 1. Thus in usual notations, Σ p(x) = 1 and ∫ f(x) dx = 1, in discrete and continuous cases respectively, where summation or integration is to be extended over the entire range of values of the random variable X. EXPECTATION Introduction: - The expectation, mathematical expectation, or expected value of a random variable may be considered as theoretical average of the random variable. The expected value of a random variable X is usually denoted by E (X) and is defined as below: (i) If X be a discrete random variable with probability mass function p (x), then E (X) = ∑ x. p(x) (ii) If X be a continuous random variable with probability density function f (x), then E (X) = ∫ x. f (x) dx. Here summation or integration is to be extended over the entire range of values of the random variable X. BINOMIAL DISTRIBUTION Introduction: - Experiments that consist of exactly two disjoint results, called ‘success’ and ‘failure’ and the probability of success in each trial be constant, are called Bernaullian experiments. In n independent trials of a Bernaullian experiment, the probability of success is a random variable and we call it a binomial random variable. If p denotes the fixed probability of success in each trial, then the number x of successes in n trials of such experiment is given by P(x) = nCx p xq n – x , x = 0,1,2,3, - - - - - - -n. q = 1 – p = probability of failure in each trial. Here n and p are called parameters of the distribution. Definition: - A discrete random variable X is said to follow a binomial distribution with parameters n and p, if it assumes only non-negative values and its probability mass function is given by P(x) = nCx p xq n – x , x = 0,1,2,3, - - - - - - -n. q = 1 p. Note: - In this case we write X ~ B(n, p), read as X follows a binomial distribution with parameters n and p. Moreover, in this case, Mean = np, variance = npq and S.D.(Standard deviation) = npq. POISSON’S DISTRIBUTION Introduction: - This probability distribution rule is an approximation to binomial probability distribution law. The conditions under which Poisson’s law is used as an approximation to binomial law are enumerated below: (i) n → ∞, i.e. the number of trials is too large, (ii) p → 0, i.e. the probability of successes is too small, (iii) np = λ, is finite. Under the above mentioned conditions, the Poisson’s probability law is given by p(x) = e λ , x = 0,1,2,3, - - - - - -to ∞, where λ is the only parameter of the distribution. —λ x x! Definition: - A discrete random variable x is said to follow a Poisson’s distribution with parameter λ, if it assumes only non- negative values and its probability mass function is given b p(x) = e λ , x = 0,1,2,3, - - - - - -to ∞. —λ x x! Note: - In this case, we write X ~ P( λ), read as X follows a Poisson’s distribution with parameter λ. Moreover, for this distribution, Mean = Variance = λ, and S.D. (Standard deviation) = λ NORMAL DISTRIBUTION Definition: - A continuous random variable X is said to follow a normal distribution with parameters μ and , called respectively mean and variance if its probability density function is given by ² f(x) = 1 e ½ (x μ) / ², ∞ < X < ∞. In this case we write X N ( , 2), which is read as “ X follows a normal distribution √2ח with mean and variance 2 ”. SAMPLING THEORY USEFUL DEFINITIONS Population: - Population means the collection or aggregate of objects having certain common character. Thus the term population does not refer to a collection of human being only; rather it may refer to a any group of objects, living or non- living, which have some common character. Thus students of a certain class, fishes in a pond, or books in a library, etc. may be taken as some examples of population. Elements of a population: - The smallest, identical and non-overlapping sections of a population are called elements of the population. Character under study: - In any statistical investigation, we are interested in a common character of the group of units or the population and we call it the ‘character under study’. Sample: - A small part of the population that may represent the entire population in respect of the character under study is called a sample. Parameter: - Statistical constants of a population are called parameter. Thus population mean, population median, population standard deviation, etc. are some common examples of parameters. Sampling unit: - The smallest, identical and non-overlapping sections of the population on which observations can be made are taken as sampling units. Clearly, sampling units are usually elements of the population, but sometimes some groups of elements of population are to be taken as sampling units. Statistic: - Any statistical measure defined on sample observations is called a statistic. Thus sample mean, sample variance, etc. are some examples of statistic. Sampling: - Sampling is the method of selecting a small part of the population that may represent the entire population in respect of the character under investigation. This small part of the population is called a sample and on the basis of observations on this part only, inferences are drawn regarding the entire population. Thus sampling is some kind of guessing. Census method or the method of complete enumeration or inquiry: - The method of statistical investigation in which observations are taken on each unit of the population and then only inferences are drawn about the entire population in respect of the character under investigation, is called census method of statistical investigation or the method of complete enumeration or inquiry. Sampling distribution. The subject matter of Statistics is to deal with ‘population’, which is a collection of objects possessing certain common character. Under any statistical investigation, one needs to find out find out the value of certain population parameter. As the size of population under any statistical investigation is usually quite large, a technique known as ‘sampling method of statistical investigation’ is usually adopted. Under this technique, firstly a small part of population is selected that may represent the entire population in respect of the character under study and it is called a sample. On the basis of observations on a sample, an approximate value of the population parameter is obtained and we call it an estimate of the population parameter under consideration. Clearly, this is a kind of guessing, but due to numerous reasons, the technique is universally accepted and preferred to the method of complete enumeration method of statistical investigation. It is a very real fact that the result of sampling method of statistical investigation depends on the observations contained in the sample that we are using. Moreover, it is also obvious that from any given population, several samples of some given size can be drawn. These samples usually differ from one another in respect of the observations contained. Hence a particular statistic is likely to yield differing estimates of the same population parameter. This gives rise to a distribution of the statistic and we call it the sampling distribution of that statistic concerned. It is impractical to study the actual distribution of any statistic as the actual number of samples and hence that of the estimates is too much large, usually larger than the population size. That is why often follow theoretical approach by making use of probability theory for studying sampling distribution of any statistic. Methods of sampling: - The schedule according to which a sample is to be selected from any given population is called a sampling method. Such methods have broadly been classified into the following two categories: (i) Probability sampling and (i) Non-probability sampling. Under probability sampling, each unit in the population has a pre-assigned probability of being selected in a sample of some given size. On the other hand, under non-probability sampling, no assignment of probability is made to the units in the population of being selected in a sample. In fact, in the later case, the selection of units is a sample depends on the discretion of desire of the concerned investigator. However, in certain non-probability sampling methods, restrictions are imposed on the desire of the investigator. Clearly, a non-probability sampling method is subjective and hence suffers from personal bias and it is also likely to be affected by lack of skill of the investigator concerned. On contrary, as the probability sampling methods are not subjective, rather such methods are objective and thereby well accepted for almost all purposes. The following are the important kinds of probability sampling: (i) Simple Random Sampling, (ii) Stratified Random Sampling, (iii) Systematic Sampling, (iv) Cluster Sampling, (v) Multistage Sampling, (vi) Ratio and Regression methods of sampling, etc. Some of the important kinds of non-probability sampling methods may be enumerated as: (i) Purposive of Judgement Sampling, (ii) Quota Sampling, etc. Simple Random Sampling. Introduction: - This is the simplest case of probability sampling. Under this method of sampling, each unit in the population is given an equal and independent chance of being selected in a sample of some given size. To provide such equal chance to units, one usually follows any kind of lottery scheme. Sometimes, one makes use of conventional ‘lottery procedures’, whereas in some cases the use of lottery machines, conventional of electronic, is made. The most sophisticated and quick way is to follow ‘Mechanical Randomization Method’ or ‘Random Numbers Table Method’. The methods can be explained as below: 1. Lottery method: - Under this method, as appears from the name of the method itself, we make use of certain lottery scheme. To follow conventional method, firstly serial numbers 1, 2, 3, - - - - - -, N are allotted to each unit in the population. These numbers are then written on N similar chits. These chits are then folded in similar way, put in an urn and mixed thoroughly. Finally, chits are drawn one by one from the same urn, unfolded and the numbers on them are noted. This process is continued till we get desired number of such numbers. The units in corresponding to the above noted numbers constitute the required simple random sample. After providing serial numbers to the units in the population as mentioned above, one may make use of lottery machines for the purpose of selecting numbers from this list. Thus the method becomes quite convenient in this case. 2. Mechanical randomization method: - Under mechanical randomization method, the use of a table, random numbers table, is made. This table has been prepared in such a way that each digit occurs with almost the same frequency. To make use of such table, firstly the units in the population are allotted serial numbers 1,2,3,- - - - -, N and then from any page of a suitable random numbers table, one can start with any one row or column of that page. The numbers of the selected row or column are noted down serially and the numbers corresponding to these numbers are considered for the required sample. This process is to be continued till the desired number of units for the sample are obtained. STRATIFIED RANDOM SAMPLING Introduction: - Before explaining this method of sampling, we first note that under simple random sampling method the variance of sample mean is given by var ( yn ) = N – n. S2. N n It is thus clear that the amount of the variance depends on n, the sample size, and S2, the population heterogeneity. Increasing the sample size can obviously reduce the variance of sample mean. But increasing sample size indefinitely large makes the sampling costly and time taking. We therefore need to reduce the effect of heterogeneity of the population and this is done by subdividing the population into homogeneous subgroups, which we call as strata. Dividing any given population into a number of strata is taken as stratification of population and the method of sampling based on stratification of population is what we call as stratified random sampling. Under this method of sampling, independent simple random samples are taken from each of the strata and the estimates of certain population parameter are finally pooled together to draw any inference about the population parameter. Here, the observations of samples from all the strata taken together are said to constitute the stratified random sample and the method of selecting such sample is called stratified random sampling method. Systematic sampling. Introduction: - This sampling method, appears from its name, is based on some system or schedule. To have a clear idea of this method, let us consider a population of size N and let the desired sample size be n. Then we first need to assign numbers 1, 2, 3, - - - - - -, N to each unit in the population. Next we find N / n = k (let), which should preferably be a whole number. Now out of the numbers 1, 2, 3, - - - - - -, k, one number is randomly chosen. If this chosen number be I, the desired sample consists of the units of the population corresponding to the numbers i, i + k, i + 2k, i + 3k, - - - - - - -, i + k and call this a systematic sample of size n with random start i. Notation: - The following are the usual notations under systematic sampling: N = population size, n = sample size, Yi = measure of character under study for i th unit in the population, Cluster sampling Introduction: - Before explaining this sampling method, we first note the fact that the smallest, identical and non- overlapping sections of a population are called elements of the population. Certain groups of elements of population, which represent certain pockets of population, are called clusters and the method of sampling based on clusters is taken as cluster sampling. In fact, under cluster sampling scheme, the entire population is divided into a finite number of clusters. The actual sampling procedure here consists in randomly selecting a few clusters from the population. The sampling units falling under these selected clusters constitute the required cluster sample. The sizes of different clusters in the population may or may not be equal. But in usual practice, we consider the case of equal cluster sizes. To explain the idea of this sampling method mathematically, let us consider the following: N = Number of clusters in the population M = Number of sampling units in each cluster n = sample size (number of clusters selected in the sample.) Then under cluster sampling method, our work is to select a random sample of n clusters out of the available N clusters in the population. The nM sampling units in the selected sample of clusters constitute the requires cluster sample. MODULE - 5 ESTIMATION The branch of Statistics called “Statistical Inference” deals with the business of drawing inferences regarding population parameters on the basis of observations on certain sample from that very population. Estimation is a technique that falls under the heading of ‘Statistical Inference’. The work of estimation has broadly been classified in two categories, namely, ‘Point estimation’ and ‘Interval estimation’. Let us now discuss these two techniques. Under ‘Point estimation’, one needs to find a value that may be taken to lie very near to the true value of the unknown population parameter. Such an approximate value of the population parameter is called an estimate of the concerned population parameter. To find any such estimate, one needs to use a suitable statistic defined on sample observations and it is called an estimator of the concerned parameter. When an estimator is operated on observations of a particular sample, an estimate of the population parameter can be obtained. It may be noted here that several samples of some given size can be taken from a fixed population and these samples usually differ from one another in terms of the observations contained, so the estimates obtained on the basis of different samples, though using the same estimator, are usually seen to differ. This gives rise to a distribution of estimates, which we call sampling distribution of the concerned statistic. To have a more clear idea of the technique, let us consider to be the unknown population parameter. Also let we agree to take samples of size n. If (X1, X2, - - - - - -, Xn) denotes a sample of n observations, then we need to find a statistic n , based on n sample observations. If this statistic n is likely to yield approximate value of , we call n as an estimator of . Under ‘Interval estimation’, the aim is to find an interval within which the unknown population parameter may be ascertained to lie with some specified degree of confidence. Such confidence is expressed in probabilistic terms and the above interval is called ‘confidence interval’. The two values, which constitute such an interval, are called confidence limits and the degree of confidence is taken as confidence coefficient. The confidence interval obtained on the basis of observations on a sample is taken as an interval estimate of the parameter. Mathematically speaking, if θ be an unknown population parameter, then under interval estimation, one needs to find two values a and b, such that P(a < < b) = 100(1 – ) %. Here a and b are confidence limits, the interval [a, b] is interval estimate of or the confidence interval for θ. Moreover, 100(1 – ) % is confidence coefficient. TESTING OF HYPOTHESES Introduction: - Testing of hypothesis should rightly be called as ‘Statistical testing of statistical hypotheses’. So we firstly need to explain the term hypothesis. A hypothesis is any statement that can be verified. A particular kind of hypothesis, which is regarding probability distribution of an observable random variable, is called ‘statistical hypothesis’. Statistical hypotheses have been classified into the following important categories: 1. Parametric (statistical) hypothesis: This is a kind of statistical hypothesis, which is regarding parameter of an observable random variable. 2. Non-parametric (statistical) hypothesis: This kind of statistical hypothesis is not regarding any parameter rather it is regarding form of some distribution. Parametric hypotheses have further been categorized as: # Simple (parametric statistical) hypothesis – This kind of statistical hypothesis specifies a single point in the parameter space. # Composite (parametric statistical) hypothesis-A parametric hypothesis, which specifies so many points in the parameter space, is taken as a composite hypothesis. The following diagram can summarize the above-mentioned information: Hypothesis (A statement that can be verified) Statistical hypothesis Non-statistical hypothesis ( A hypothesis regarding probability distribution ( Not regarding a random variable.) of an observable random variable.) Parametric (statistical) hypothesis Non-parametric (statistical) hypothesis (A hypothesis regarding parameter (A hypothesis which is not regarding parameter of a random of a random variable.) variable rather it is regarding only the form of the distribution.) Simple (parametric statistical) hypothesis Composite (parametric statistical) hypothesis (A parametric hypothesis that specifies (A parametric hypothesis, which specifies so a single point in the parameter space.) many points in the parameter space.) EXAMPLES Hypothesis: - (i) Cloudy days are warmer than clear days. (ii) Smoking is injurious to health. Statistical hypothesis: - (i) The sample comes from a population whose mean is 50. (ii) The sample comes from a binomial population. Parametric (statistical) hypothesis: - (i) For a normal population σ2 > 10. (ii) For a Poisson’s population λ = 3 Non-parametric (statistical) hypothesis: - (i) The sample comes from some normal population. (ii) Two samples have the same distribution, i. e. f1(.) = f2(.). Simple (parametric statistical) hypothesis: - (i) λ = 2, λ being the parameter of a Poisson’s distribution. (ii) μ = 100, for a normal variate. Composite (parametric statistical) hypothesis: - (i) λ ≠ 3, where λ is parameter of a Poisson’s variate. (ii) p > 0.6, where p is parameter of a binomial variate. Null and alternative hypotheses: - In statistical testing of hypotheses, we are mainly concerned with a hypothesis of no or null difference, hence we call it a null hypothesis. This kind of hypothesis is also called ‘hypothesis under test’. In framing such a hypothesis, one must remain impartial towards the facts under consideration. It is logical to think that a test may not always result in acceptance of the null hypothesis. Clearly, whenever a test results in rejection of the null hypothesis, one must conclude otherwise. To serve this purpose, there is another kind of hypothesis that we call an ‘alternative hypothesis’. This hypothesis must always be rival in sense to the null hypothesis. The common understanding regarding these two hypotheses is like this – whenever the null hypothesis is accepted, the alternative hypothesis gets rejected and vice-versa. Usually a null hypothesis is denoted by Ho and the alternative hypothesis by H1. Tests of significance Introduction: - A group of units possessing certain common character is called a population. Any statistical constant of a population is taken as a parameter. The actual value of a population parameter cannot practically be known. However, on the basis of a small part of the population, i.e. a sample, one can find an approximate value of certain population parameter. Such approximate value is called an estimate of the concerned parameter and it usually differs from the actual value of the parameter. Under the branch of Statistics called ‘Statistical Inference’, an important work is to assume certain value of a parameter on the basis of reasonable grounds and then to compare this value with its sample estimate. If these two values are not much different, the assumed value of the parameter is taken to be true; on the other hand, when the difference is significant, assumed value of the parameter is not taken to be true. The above work is taken as ‘Test of significance’ and should rightly be called as ‘Statistical tests of significance of difference between assumed value of population parameter and its sample estimate’. The work of tests of significance has broadly been classified into the following two categories: I. Large sample tests and II. Small sample tests. Large sample tests. Introduction: - Under the heading ‘Large sample tests’, we consider only those cases when the sample size exceeds 30. In this case, we make use of the area property of normal curve and also the fact that for large sample, any statistic obeys normal distribution law with the same mean as population but having variance 1/nth time the population variance. Thus for any statistic t, based on n sample observations, for large value of n we have, t – E(t) ~ N(0, 1), where S. E.(t) = √ var(t). S. E.(t) Thus by making use of this property, several kinds of test statistic Z has been proposed to be used in differing situations. The main steps to be followed under any large sample test may be enumerated as below: 1. Framing the null and alternative hypotheses: - Here, the null hypothesis is to be framed in an impartial manner and hence the terms under comparison are taken to be not significantly different. The alternative hypothesis must also be framed keeping in mind the object of the test and the fact that it must be rival in sense to the null hypothesis. 2. Choosing suitable test statistic: - Depending upon the hypothesis to be tested, we need to select suitable test statistic Z. The value of Z is then computed on the basis of the given sample observations and population values. 3. Drawing inference: - Comparing the computed value of test statistics Z with standard critical value, a decision regarding the acceptance or rejection of the null and alternative hypotheses is taken. The critical value Z depends upon the level of significance and is to be computed using normal probability table. The test criteria are like this; (i) if |Z| < Zα, the null hypothesis is accepted. (ii) if |Z| > Zα, the null hypothesis is rejected in favour of the alternative hypothesis. Here Zα is standard critical value obtained from normal probability table on the basis of the level of significance. Different cases in large sample tests. Test for specified mean: - Here we need to test the significance of difference between sample mean and population mean. The null hypothesis to be tested here is: Ho: μ = μo (specified value) The test to be used in this case is Z = x – μ , where n = sample size, x = sample mean, μ = population mean and σ = population standard deviation. σ √n Small sample tests. Introduction: - Statistical tests of significance based on samples whose sizes are less than 30 are taken as small sample tests. Such tests are based on the probability distribution of certain statistic like t, 2, F, etc. The following are the main steps to be followed in any small sample tests: 1. Framing the null and alternative hypotheses: - Here, the null hypothesis is to be framed in an impartial manner and hence the terms under comparison are taken to be not significantly different. The alternative hypothesis must also be framed keeping in mind the object of the test and the fact that it must be rival in sense to the null hypothesis. 2. Choosing suitable test statistic: - Depending upon the hypothesis to be tested, we need to select suitable test statistic from amongst the possible cases of t, F and 2. 3. Observing the value of the test statistic from table at desired level of significance and for specified d. f.. 4. Drawing inference: - Comparing the computed value of test statistics with tabulated value, a decision regarding the acceptance or rejection of the null and alternative hypotheses is taken. The test criterion is: (i) If calculated value < tabulated value, the null hypothesis is to be accepted. (ii) If calculated value > tabulated value, the null hypothesis is to be rejected in favour of the alternative. Different cases in small sample tests. t – test. Introduction: - Small sample tests based on the probability distribution of t – statistic are called t – tests. The following are the three kinds of t – tests: t – test for specified mean: - This kind of t – test is meant to test the significance of difference between sample mean and population mean. Thus the null hypothesis to be tested in this case is Ho: μ = μo (specified value) t = x – μ = x – μ where n = sample size, x = sample mean, μ = population mean and S = sample mean square. 2 S √n s √n –1 we have S2 = 1/(n-1) ∑( xi – x )2 and s2 =1/n ∑( xi –x )2 = sample variance, so ns2 = (n –1) S2. The degrees of freedom for the test is n – 1. 2 - test. Introduction: - This test is based on probability distribution of 2-statistic. Here the area property of - probability curve is used to test the null hypothesis. For acceptance or rejection of the concerned hypothesis, chi- square probability table is used. The main kinds of chi-square test may be enumerated as: - test for goodness of fit: - Under this test one can compare the theoretical facts with corresponding data. The test statistic to be used in this case is given by n where Oi = observed frequency = (Oi Ei) 2 , Ei = expected frequency i =1 Ei [The degree of freedom for the test is (n – 1).] Introduction to non-parametric tests. The branch of Statistics that deals with drawing inferences on the basis of some sample of observations from the population under investigation is called ‘Statistical Inference’. The technique of non-parametric tests falls under the heading of Statistical Inference. To have a clear idea of this technique, let us first note the fact that the usual statistical tests of significance are meant to test the hypotheses regarding certain parameters of some given population and hence we call these as ‘Parametric tests’. On the other hand, we have statistical tests which have nothing to do with parameters and the hypotheses to be tested here are only regarding form of the distributions and hence we call these as ‘Non-parametric tests’ or ‘Distribution-free tests’. Under such tests, we usually take into consideration a hypothesis of the form f 1(. ) = f 2(. ), where f 1(. ) and f 2(. ) represent the probability density functions of the two populations under consideration. But sometimes we need to consider only one sample and we test the hypothesis of randomness of such sample. The following are some important Non-parametric tests: Wald-Wolfowitz Run Test This is a case of non-parametric test meant to test the hypothesis of the form f 1(. ) = f 2(. ), where f 1(. ) and f 2(. ) represent the probability density functions of the two populations under consideration. As appears from the name of the test itself, it is based on the concept of the term ‘run’. To have a clear idea of this term, let us consider (x 1, x 2, - - - - - -, x n 1) and (y 1, y 2, - - - - - -, y n 2) as two samples from the two populations under consideration. If we combine these n 1 + n 2 observations of the two samples and arrange these in ascending or descending order, we have a sequence of x i’s and yj’s. Here the characters of one type surrounded by those of the other type is taken as a run. Thus if we have an arrangement of the sample observations in the combined sample of the type x 1, x 2, y 1, x 3, y 2, y 3, y 4, x 4, - - - - -, then x 1, x 2 is a run, y 1 is another run, x 3 is next run, y 2, y 3, y 4 is the other run, and so on. Let us now explain the actual test procedure, which consists of the following steps: Step 1: - Consider the Null hypothesis of the form H0 : f 1(. ) = f 2(. ) Step 2: -Combine the observations in the two samples having n1 and n 2 observations and arrange these (n1 + n 2) observations in ascending order of their magnitudes. Step 3: - Count the number of runs in the above mentioned combined ordered sample. Let this number of runs be U. Step 4: - Evaluate E (U) and Var (U) by using the formula mentioned below E (U) = 2n1 n 2 + 1 and Var (U) = 2n1 n 2 (2n1 n 2 – n1 – n 2) n1 + n 2 (n1 + n 2) 2 (n1 + n 2 – 1) Step 5: - Compute the value of the test statistic Z given by Z = U – E (U) N (0, 1) Var (U) Step 6: - Compare the value of the test statistic Z with standard critical value and this leads to acceptance or rejection of the null hypothesis under consideration. The test criteria is like this: Whenever Z > Z , the null hypothesis is rejected, otherwise it is accepted. Here Z denotes the standard critical value at % level of significance. Median test This is a case of non-parametric test meant to test the hypothesis of the form f 1(. ) = f 2(. ), where f 1(. ) and f 2(. ) represent the probability density functions of the two populations under consideration. As appears from the name of the test itself, it is based on the median of the combined sample. To explain this method in detail, let us consider (x 1, x 2, - - - - - -, x n 1) and (y 1, y 2, - - - - - -, y n 2) as two samples from the two populations under consideration. We combine these n1 + n 2 observations of the two samples and arrange these in ascending or descending order to find median of the combined sample. The actual test procedure consists of the following steps: Step 1: - Consider the Null hypothesis of the form H0 : f 1(. ) = f 2(. ) Step 2: -Combine the observations in the two samples having n1 and n 2 observations and arrange these (n1 + n 2) observations in ascending order of their magnitudes. Step 3: - Compute the median of the combined sample and let it be M. Step 4: - Count the number of observations of the first sample exceeding the above median M and let this number be m1. Step 5: - Evaluate E (m1) and Var (m1) by using the formula mentioned below E (m1) = n1 , if N = n1 + n 2 is even 2 =n1. N – 1 , if N is odd. 2 N Var (m1) = n1 , if N = n1 + n 2 is even 2 = n1. N – 1 , if N is odd. 2 N Step 6: - Compute the value of the test statistic Z given by Z = U – E (U) N (0, 1) Var (U) Step 7: - Compare the value of the test statistic Z with standard critical value and this leads to acceptance or rejection of the null hypothesis under consideration. The test criteria is like this: Whenever Z > Z , the null hypothesis is rejected, otherwise it is accepted. Here Z denotes the standard critical value at % level of significance. Sign test This non-parametric test is meant for a specific situation when one desires to compare two things or materials under various sets of conditions. The circumstances under which such an experiment is conducted may be enumerated as below: (i) When there are pairs of observations on two things under comparison. (ii) For any given pair of observations, each of the two observations must be taken under similar extraneous conditions. (iii) Different pairs are observed under different conditions. The application of Sign test is subjected to the following assumptions: 1. Measurements of characters should such that the deviations of observations of the form di = x i – y i, can be expressed in terms of positive or negative signs. 2. Variables have continuous distribution. 3. The values di’s, for different values of i are independent. The steps to be followed under this test based on n pairs of observation of the form (xi, yi), may be enumerated as below: Step 1: - Consider the Null hypothesis of the form H0 : f 1(. ) = f 2(. ) Step 2: - Count the number of positive deviations and denote it by U. Step 3: - Evaluate E (U) and Var (U) by using the formula mentioned below E (U) = n, Var (U) = n 2 4 Step 4: - Compute the value of the test statistic Z given by Z = U – E (U) N (0, 1) Var (U) Step 5: - Compare the value of the test statistic Z with standard critical value and this leads to acceptance or rejection of the null hypothesis under consideration. The test criteria is like this: Whenever Z > Z , the null hypothesis is rejected, otherwise it is accepted. Here Z denotes the standard critical value at % level of significance.