Digital Governance Notes PDF
Document Details
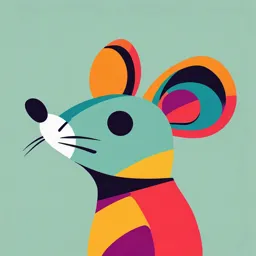
Uploaded by ThoughtfulRadium6251
Sorbonne Nouvelle
Tags
Related
- Maestría en Inteligencia Artificial y Educación Digital (PDF)
- Definition Of Terms PDF
- Demystifying AI: What Digital Transformation Leaders Can Teach You About Realistic Artificial Intelligence (PDF)
- Demystifying AI: What Digital Transformation Leaders Can Teach You About Realistic Artificial Intelligence (2019)
- Artificial Intelligence in Protecting Cyber Security (PDF)
- Investigating AI for Visual Accessibility PDF
Summary
These notes discuss the regulation of artificial intelligence (AI), exploring the reasons for regulation, including bias and inequality, accountability, and managing risks. They also examine how AI is regulated, including laws, government actions, and private sector initiatives. The notes touch on ethical and societal implications of AI.
Full Transcript
I. Why Regulate AI? 1. Core Reasons for Regulation Bias and Inequality: ○ Algorithms can unintentionally reflect and amplify biases found in their training data. ○ Example: A hiring algorithm trained on historical data might favor men over women for...
I. Why Regulate AI? 1. Core Reasons for Regulation Bias and Inequality: ○ Algorithms can unintentionally reflect and amplify biases found in their training data. ○ Example: A hiring algorithm trained on historical data might favor men over women for leadership roles due to past biase. ○ Impact: Such biases can worsen social inequalities, excluding marginalized groups from opportunities like employment, loans, or housing. Ensuring Accountability: ○ Regulations clarify who is responsible when AI fails, ensuring developers, companies, or users can be held accountabl. ○ Example: If a self-driving car causes an accident, regulations should specify whether the manufacturer, software developer, or driver is liable. Managing Risks: ○ Without regulation, AI can be used to spread misinformation (e.g., deepfakes) or facilitate election interferenc. ○ Example: Deepfake videos falsely portraying political figures can disrupt democratic processes. 2. Philosophy of Technology Technology Is Not Neutral: ○ AI reflects the choices and biases of its creators, making it a socio-technical system rather than a neutral too. ○ Example: Algorithms used in predictive policing may disproportionately target certain communities based on biased historical data. Hardening of Categories: ○ Metaphors like "cyberspace" shape how we think about technology and limit flexible problem-solving. ○ Example: Viewing the internet as a "space" encourages policies that treat it as separate from the real world, ignoring its integration into everyday lif. II. How Is AI Regulated? AI regulation is implemented through a combination of laws, government actions, private sector initiatives, and international agreements. By regulating AI, governments and organizations can ensure that technology supports societal progress, protects human rights, and fosters trust in emerging technologies. Each method addresses specific challenges of AI, such as bias, accountability, and security risks. 1. Laws (Legislative Governance) The EU AI Act: ○ This is a comprehensive legal framework categorizing AI systems into three risk levels: Low-Risk AI: Minimal regulations (e.g., chatbots). High-Risk AI: Used in critical sectors like healthcare and law enforcement, requiring strict rules for safety, fairness, and transparency. Unacceptable Risk AI: Banned applications, like AI used for social scoring or mass surveillance. Example: AI systems used for medical diagnoses must meet rigorous standards for accuracy and explainability. If an AI predicts a medical condition incorrectly, the system must provide an explanation of how it arrived at the diagnosis to prevent harm to patients. The U.S. Approach: ○ Unlike the EU, the U.S. has no unified federal AI regulation, leading to a patchwork of state-level rules. ○ The Algorithmic Accountability Act aims to reduce AI bias by requiring developers to test systems for fairness, especially those used in hiring or lending. Example: A state law might require transparency for AI systems in hiring processes, but another state might not, creating inconsistencies across the country. 2. Executive Actions Quick and Flexible Responses: ○ Governments use executive orders to respond quickly to emerging AI risks without waiting for legislation. ○ Example: The Biden administration issued an executive order mandating that all federal agencies assess AI risks before using such systems, ensuring government technology aligns with public safety and fairness standards. Strategic Focus Areas: ○ National Security: Monitoring global AI advancements to address threats like cyberattacks or autonomous weapon systems. ○ Workforce Adaptation: Creating training programs to help workers adapt to AI-driven changes in industries like manufacturing and customer service. Example: A government might fund AI-focused retraining programs for displaced workers in the automotive industry, ensuring they can transition into tech- driven roles. 3. Private Sector Regulation Self-Regulation: ○ Companies like Google and OpenAI establish internal ethical guidelines and conduct audits to address risks like bias or lack of transparency. ○ Why? Public trust is crucial for business success, and addressing ethical concerns can give companies a competitive edge. ○ Example: Google has an AI Ethics Board to review algorithms for fairness and avoid discrimination, particularly in sensitive applications like credit scoring. Call for Government Oversight: ○ Tech leaders recognize the limits of self-regulation and are increasingly calling for clear government frameworks. ○ Example: OpenAI’s CEO proposed a federal agency dedicated to overseeing powerful AI models, ensuring they are developed responsibly and do not harm society. 4. International Agreements OECD (Organisation for Economic Co-operation and Development ) AI Principles: International guidelines adopted by over 40 countries, including the U.S. and EU member states, to ensure ethical and human-centric AI development. These principles focus on fairness, transparency, accountability, and respect for democratic values, aiming to create shared global standards for responsible AI use. However, countries approach implementation differently: The EU: Focuses on strict privacy and data protection, exemplified by the GDPR, which regulates how personal data is collected and used. The U.S.: Emphasizes fostering innovation, allowing more flexibility in AI development to maintain global competitiveness. Significance: While the OECD Principles create a shared ethical foundation, regional differences—such as the EU's privacy focus versus the U.S.'s innovation-driven approach— make global harmonization challenging. These variations highlight the need for continued international collaboration to balance regulation and innovation effectively. III. Risks of AI 1. Algorithmic Bias and Inequality Algorithms trained on biased data replicate and scale these biases. Example: The COMPAS algorithm, used in criminal justice, showed racial bias by disproportionately labeling minority offenders as high risk, which perpetuates systemic discrimination in sentencing decisions. 2. Accountability Challenges AI decisions often involve "black box" systems, where it’s unclear how decisions are made. They are like mysterious machines, you put in data and get a result, but you don’t know what happens inside to produce that result. Impact: Lack of transparency makes it difficult to hold anyone accountable for harmful outcomes. 3. Emerging Challenges in AI Regulation Looking ahead, regulators face new and complex challenges as AI technology advances: 1. AI in Warfare: ○ The development of autonomous weapons raises ethical concerns about accountability, decision-making in conflict zones, and the potential for misuse by malicious actors. Example: AI-powered drones making life-and-death decisions without human intervention could lead to catastrophic consequences if misused. 2. Quantum Computing and AI: ○ Quantum computing has the potential to significantly enhance AI capabilities, accelerating breakthroughs but also magnifying risks, such as advanced cyberattacks and breaches of encrypted data. Example: Regulators must prepare for scenarios where quantum-enhanced AI systems outpace existing safeguards, requiring entirely new oversight mechanisms. 3. AI and Climate Change: ○ As AI models grow larger and require more computational power/energy, their environmental impact becomes a critical issue. Example: - Developing large language models like GPT-3 consumed enough energy to power multiple households for a yea. -Policymakers must incentivize the development of energy-efficient AI systems and renewable-powered data centers. 4. Deepening Social Inequalities: ○ Without proactive regulation, AI risks further entrenching existing inequalities, particularly in underrepresented communities. Example: Unequal access to AI-driven education tools or healthcare systems could widen the global wealth and opportunity gap. IV. Platform Governance 1. Challenges Balancing free speech with protecting users from harmful content (e.g., hate speech, misinformation). AI moderation tools often struggle to distinguish between harmful and harmless conten. 2. Decentralized Governance Platforms could use blockchain to give users voting power on decisions, like rule changes or fee structures. Risks: ○ Wealthy users could dominate decision-making. ○ Governance attacks by bad actors might manipulate outcome. Example : Platforms like Reddit already allow community-based moderation, where users vote on rules and content decisions. s t l e r e s e V. Digital Capitalism 1. What is Digital Capitalism? Digital capitalism is an economic system where companies profit by turning user data and online interactions into valuable resources. For example, platforms like Facebook collect data on user behavior and sell it to advertisers, making your personal activity part of their business model. 2. Impacts on Labor: Platforms create precarious work (e.g., gig economy jobs). Gig workers often lack stable income, job security, and legal protections like health insurance because platforms classify them as independent contractors rather than employees. Example: Uber drivers are managed by algorithms that determine pay and work condition. VI. Key Stakeholders and Their Interests Key stakeholders in AI governance include governments, which regulate to protect fairness and competition; tech companies, which seek innovation-friendly rules; and civil society, which advocates for transparency and ethical AI. I. PUBLIC SECTOR TECH REGULATION A. Attempts & Thoughts on Regulation A. Legislative governance: Oversight of AI: rules for arti cial intelligence Article: Future of Life Institute. “Pause Giant AI Experiments: An Open Letter.” March 22. 2023. Article: Senate Judiciary Sub-Committee on Privacy, Technology, and the Law. “Oversight of A.I.: Rules for Arti cial Summary: Advocates a six-month moratorium on AI training to assess risks and ethics in advanced AI systems. Intelligence.” Recording of Hearing, May 16th, 2023. Focus: Emphasizes the need for global cooperation to ensure ethical AI development and governance. Senate Judiciary Sub-Committee Hearing on AI: Oversight and Rules The Open Letter on AI Experiments - Main Ideas Context: A call for a moratorium on advanced AI training, endorsed by prominent tech leaders. Sam Altman (CEO of OpenAI): AI can solve major challenges, like curing diseases. Main Ideas: Addressing risks of uncontrolled AI development, such as inequality, safety concerns, and ethical Gary Marcus (Professor): AI threatens democracy and is unreliable. dilemmas. Senator Blumenthal: AI requires transparency, liability, and trust but faces slow regulation. Key Takeaways: - Key Takeaways ◦ A global governance framework is needed to regulate AI development. AI has transformative potential but requires safeguards to mitigate risks. ◦ The moratorium aims to balance innovation with societal safety. Transparency, accountability, and collaboration are essential for ethical governance. Conclusion: The pause on AI experiments underscores the need for measured, collaborative approaches to Regulation must align AI development with democratic values and public trust. governance. - Conclusion: Global cooperation is needed to ensure AI serves humanity responsibly / AI must be aligned with democratic values /Tight collaboration between independent scientist and governments / Collaboration of key B. Private sector Calls for Government Regulation actors on a global scale Article: Naughton, J. “When the tech boys start asking for new regulations, you know something’s up.”The Guardian, May 20. 2023. B. Executive Governance Summary: Highlights industry calls for oversight to ensure ethical AI. Article: “Big tech is very afraid of a very modest AI safety Bill” Focus: Examines the strategic and ethical implications of AI regulation, balancing innovation and risk mitigation. Summary: Examines legislative attempts to establish safety protocols for high-risk AI, highlighting regulatory pushback Sam Altman’s Call for AI Regulation from Big Tech. Context: OpenAI's CEO, Sam Altman, testi ed before the Senate advocating AI regulation, raising questions Focus: Discusses balancing innovation with public safety while navigating industry resistance. about the motivations behind his appeal. Safe and Secure Innovation Bill (SB1047) Main Ideas: Altman’s call is seen as either a strategic move to consolidate OpenAI's market dominance or a Context: A proposed legislative bill in California aiming to regulate high-risk AI models. genuine plea for oversight amidst AI's potential risks. Main Ideas: The bill would implement safety protocols but faced opposition from tech companies citing Key Takeaways: innovation barriers. ◦ AI's societal impact includes misinformation, loneliness, and risks to democracy. Key Takeaways: ◦ The need for licensing, collaboration with governments, and enforceable safety protocols. ◦ Public-private partnerships and accountability are vital for balancing innovation and safety. Conclusion: Without action, AI could spiral into uncontrollable threats, emphasizing the urgency of proactive ◦ The bill's veto highlights tensions between regulation and industry growth. regulation. Conclusion: AI governance requires collaboration across legislative, executive, and private sectors. II. INTERNATIONAL TRADE AGREEMENTS AND PLATFORM GOVERNANCE Enshiti cation = emmerdi cation A. International Trade agreement B. Platform governance Article: International “Digital Trade” Agreements: The Next Frontier Article: de Mesquita and Hale, "Platforms Need to Work with Their Users – Not Against Them" (2022). Summary: Explores how treaties shape global tech governance, often prioritizing corporate interests over transparency. Summary: Proposes blockchain-based governance to balance power between platforms and producers. Focus: Analyzes the role of trade policies in embedding corporate-friendly regulations and limiting government Focus: Discusses decentralization as a tool for enhancing user agency while noting implementation challenges. oversight. Platforms and Decentralized Governance Digital Trade Agreements and Big Tech Context: Analyzing the power imbalance between digital platforms and users. Context: Examining how digital trade agreements shape global tech regulation. Main Ideas: Blockchain-based governance could empower users against centralized platform control. Main Ideas: These agreements often favor corporate interests over public accountability. Key Takeaways: Key Takeaways: ◦ Decentralized governance distributes decision-making power but poses risks of low ◦ Algorithmic transparency and fair governance clauses are needed. participation and vote manipulation. ◦ Secrecy in negotiations limits public participation. ◦ Balancing innovation with fairness is essential. Conclusion: Progressive frameworks can ensure digital trade agreements serve broader societal interests. Conclusion: Blockchain o ers promise but requires careful implementation to ensure equity. III. AI, NEOLIBERALISM, AND THE PUBLIC INTEREST A. AI Innovation and Ideals of Technological Progress B. AI’s Impact on Knowledge Value and Understanding Article: The Techno-Optimist Manifesto by Marc Andreessen Article: Suchman, L. (2023). The uncontroversial ‘thingness’ of AI. Big Data & Society, 10(2), 20539517231206794. Summary: Advocates for embracing technology as a driver of progress while downplaying risks and ethical concerns. Summary: Critiques the anthropomorphization of AI, emphasizing its socio-technical roots. Focus: Highlights the transformative potential of tech while critiquing its simplistic optimism. Focus: Challenges assumptions that AI is independent, arguing it re ects human-made decisions and societal biases. Marc Andreessen’s Techno-Optimist Manifesto Lucy Suchman’s Critique of AI’s “Thingness” Context: Advocating technology as humanity's driver of progress. Context: Reframing AI not as an independent force but as a human-made socio-technical construct. Main Ideas: Highlighting the transformative potential of AI, energy innovation, and free markets. Main Ideas: AI re ects biases, societal values, and human decisions rather than being an autonomous entity. Key Takeaways: Key Takeaways: ◦ Technology must balance growth with ethics and sustainability. ◦ Treating AI as "magic" obscures its human-made biases. ◦ Critiques point to unchecked optimism ignoring societal inequalities. ◦ Transparent and ethical AI design is necessary. Conclusion: Optimism must be tempered with caution and ethical frameworks. Conclusion: AI must be understood as a tool shaped by human choices, aligning its development with societal values. IV. THEORIZING DIGITAL CAPITALISM AND ITS VARIETIES A. Theorizing digital capitalism and its varieties B. Varieties of Digital Capitalism Article: “Techno-Feudalism”:Watch Yanis Varoufakis, “Technofeudalism: Explaining To Slavoj Zizek Why I Think Article: Bircan, Tuba, and Emre Eren Korkmaz. "Big data for whose sake? Governing migration through arti cial Capitalism Has Evolved Into Something Else, Gane, Nicholas, "Capitalism is capitalism, not technofeudalism." Journal of intelligence." Humanities and Social Sciences Communications 8, no. 1 (2021): 1-5. Classical. Summary: Explores big data’s transformative potential while highlighting risks of bias, surveillance, and ethical Techno-Feudalism: A Critique of Modern Digital Economies dilemmas. Context: This article critiques the shift from a capitalist to a techno-feudal economy dominated by Big Tech Focus: Stresses the importance of transparency and regulation to ensure equitable use of data technologies. monopolies. Main Ideas: Techno-feudalism re ects a system where data and platform ownership supersede traditional Big Data’s Role in Society capital, concentrating power in a few hands. Context: This article explores the societal implications of big data, focusing on its dual-edged impact on Key Takeaways: governance, business, and personal privacy. ◦ Digital platforms function as “lords” in a feudal-like hierarchy, extracting value from users (the Main Ideas: Big data enhances decision-making and predictive analytics but poses risks such as surveillance, “serfs”) who contribute data. privacy erosion, and biases in algorithmic outcomes. ◦ This dynamic creates vast inequalities and reduces competition and innovation. Key Takeaways: ◦ Decentralization and regulation are proposed to dismantle feudal-like control. ◦ Big data is reshaping sectors from healthcare to urban planning, enabling e ciencies and Conclusion: The rise of techno-feudalism calls for structural interventions to ensure digital economies promote innovation. fairness and innovation rather than entrenching power. ◦ Risks include perpetuating systemic biases (e.g., biased algorithms in hiring) and invasive surveillance. ◦ Ethical guidelines and transparency are necessary to prevent misuse. Conclusion: Big data holds transformative potential, but careful regulation and ethical oversight are crucial to ensure it bene ts society equitably. V. POLITICAL AND ECONOMIC STRUCTURES A. Rethinking political structures B. Reinventing Economic structures Article: Risse, Mathias. "Arti cial Intelligence and the Past, Present, and Future of Democracy." (2022) Article: Where do you belong in crypto? Results from the Cryptopolitical Typology Quiz (2022). Summary: Examines how AI intersects with democracy, potentially improving or undermining governance structures. Summary: Surveys diverse political and economic perspectives within the crypto community, highlighting Focus: Explores philosophical, historical, and political dimensions of AI’s role in democracy. fragmentation. Focus: Examines governance debates, from total decentralization to hybrid and regulatory approaches. Mathias Risse on AI and Democracy Context: Exploring how AI interacts with democratic processes and power structures. Cryptopolitical Typology Quiz Main Ideas: AI could either bolster or undermine democracy, depending on its governance. Context: Understanding the crypto community’s fragmented opinions through a survey. Key Takeaways: Main Ideas: Divided views on decentralization, governance, and regulations within the crypto space. ◦ AI's potential to reinforce inequality vs. improve participation. Key Takeaways: ◦ Transparency and ethical standards are critical for democratic integration. ◦ Strong debates exist between total decentralization advocates and supporters of hybrid Conclusion: The future of democracy intertwined with AI hinges on intentional, ethical design. (need to adapt governance. to AI while preserving human values / How to design and regulate AI/ Finding balance) ◦ (4 major institution) Regulations are increasingly shaping the crypto landscape globally. ◦ On-chain + o chain Conclusion: Fragmentation within the community highlights the need for balanced, inclusive governance. Discussions Langdon Winner ideas 1. Embedded politics (tech and political are interconnected) 2. Inherently political => some technologies required speci c political structure => like nuclear power plant, concentration of power Ex: linguistic defranchisement, access to paid version (gatekepping/money) Ex: AI governed by cooperation and government MARX “Technology is the outcome of material conditions” => including capitalism System economic a ect technology fi ff fi fl ff fi ff fi fl fi fi fi fl ffi fi fi s 1. How can AI contribute to the dehumanization of migration processes, and what strategies could be adopted to counter this trend? 1. AI and Dehumanization in Migration Processes Key Contributions to Dehumanization: ◦ Automation of decision-making erases individual contexts (e.g., asylum applications). ◦ Use of biased datasets leading to discriminatory outcomes. ◦ Reduced human interaction, replacing empathy with algorithms. ◦ Surveillance tools treat migrants as security threats (e.g., biometric tracking). Strategies to Counter: ◦ Human oversight in decision-making processes. ◦ Inclusive, bias-free datasets. ◦ Ethical frameworks emphasizing dignity and rights. ◦ Transparent auditing of AI systems in migration. 2. Critically analyze the role of AI in strengthening or weakening democratic processes. Provide real-world examples. 2. AI and Democratic Processes Strengthening: ◦ Enhances participation through digital platforms (e.g., online petitions). ◦ Improves policymaking with data-driven insights (e.g., voter behavior analysis). Weakening: ◦ Disinformation campaigns (e.g., Cambridge Analytica). ◦ Algorithmic bias influencing elections (e.g., targeted ads). Examples: ◦ Strengthening: AI tools for civic engagement in Estonia's e-governance. ◦ Weakening: Russian interference in the 2016 U.S. elections. 3. To what extent should governments have access to private companies' AI algorithms to ensure fairness and accountability? Discuss the ethical and legal implications. 3. Government Access to AI Algorithms Arguments for Access: ◦ Ensures fairness and accountability in sensitive areas (e.g., hiring, credit scoring). ◦ Prevents harm caused by opaque systems. Ethical and Legal Implications: ◦ Risks of government overreach and privacy violations. ◦ Challenges in intellectual property protection. Balanced Approach: ◦ Independent oversight committees. ◦ Privacy-preserving audit mechanisms. 4. How can algorithmic transparency be achieved while protecting trade secrets and proprietary technology? Propose a balanced approach. 4. Algorithmic Transparency vs. Trade Secrets Challenges: ◦ Balancing corporate confidentiality and public accountability. ◦ Fear of intellectual property theft. Proposed Approach: ◦ Confidential third-party audits. ◦ Explainable AI frameworks. ◦ Limited disclosure agreements for sensitive algorithms. 5. Should AI systems be used to make decisions in high-stakes areas like criminal justice, health, or migration? Justify your answer with arguments and examples. 5. AI in High-Stakes Decisions Arguments for Use: ◦ Enhances efficiency and consistency (e.g., diagnosing diseases). ◦ Reduces human error in repetitive tasks (e.g., legal sentencing guidelines). Arguments Against Use: ◦ Risk of perpetuating biases (e.g., COMPAS in criminal justice). ◦ Lack of accountability in opaque decision-making. Examples: ◦ Success: AI in early cancer detection. ◦ Failure: Bias in predictive policing algorithms. 6. Discuss the key principles that should guide the regulation of AI at a global level. How can countries balance innovation with accountability? 6. Key Principles for Global AI Regulation Principles: ◦ Transparency, accountability, and inclusivity. ◦ Human-centric design and ethical frameworks. Balancing Innovation and Accountability: ◦ Encourage innovation via funding and incentives. ◦ Establish international agreements on ethical AI usage (e.g., UNESCO’s AI ethics guidelines). 7. How can the use of blockchain-based governance systems democratize decision-making on digital platforms? Discuss potential benefits and risks. 7. Blockchain in Digital Governance Potential Benefits: ◦ Decentralized decision-making reduces power concentration. ◦ Increases transparency in governance processes. Risks: ◦ Low participation due to technical complexity. ◦ Vulnerability to vote manipulation. Examples: ◦ Ethereum-based DAOs enabling community decision-making. 8. What are the most effective ways to ensure ethical AI development and deployment in areas like border control, hiring, and law enforcement? 8. Ethical AI in Sensitive Areas Challenges: ◦ Risk of discrimination and privacy violations. ◦ Accountability gaps in high-stakes decisions. Strategies: ◦ Human-AI collaboration in border control (e.g., manual review of flagged cases). ◦ Bias audits for hiring algorithms. ◦ Strict guidelines for law enforcement use. 9. To what extent does the lack of transparency in AI development affect public trust in technology? Propose strategies to build greater trust. 9. Transparency and Public Trust Impacts of Non-Transparency: ◦ Fuels mistrust and resistance to adoption. ◦ Reduces confidence in fairness and accountability. Strategies to Build Trust: ◦ Regular reporting on AI impact and performance. ◦ Public consultations in algorithm design. ◦ Independent review boards for contentious AI applications. 10. Do you believe that AI will increase or reduce inequality in society? Justify your answer using both theoretical arguments and real-world examples. 10. AI and Societal Inequality Arguments for Increased Inequality: ◦ Amplifies existing biases (e.g., hiring discrimination). ◦ Concentrates wealth and power among tech monopolies. Arguments for Reduced Inequality: ◦ Expands access to education and healthcare. ◦ Automates mundane tasks, creating opportunities for skilled labor. Examples: ◦ Increasing inequality: Bias in loan approvals. ◦ Reducing inequality: AI tools in rural healthcare delivery (e.g., diagnostics in India).