Critical And Creative Thinking Lecture 4 PDF
Document Details
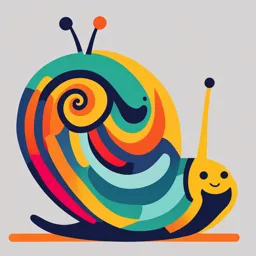
Uploaded by WonderfulConnemara587
VinUniversity
Tags
Summary
This document is a lecture on critical and creative thinking, specifically focusing on causal inference. The lecture covers a variety of topics including inductive reasoning, general claims, and the nature of causation. It also features examples, activities, and discussions meant for a university-level audience.
Full Transcript
Critical And Creative Thinking Lecture 4 Causal Inference IMPORTANT NOTICE There is substantial evidence from research in cognitive psychology and neuroscience that distraction can negatively affect learning. Distractions interfere with one’s ability to process and retain information, leading to...
Critical And Creative Thinking Lecture 4 Causal Inference IMPORTANT NOTICE There is substantial evidence from research in cognitive psychology and neuroscience that distraction can negatively affect learning. Distractions interfere with one’s ability to process and retain information, leading to decreased focus, reduced comprehension, and impaired memory consolidation. For this reason, laptops and tablets are strictly prohibited in this class (unless otherwise instructed). If your laptop or tablet is visible you will be marked as having an unauthorized absence from the class. Each unauthorized absence will reduce your overall grade. Four or more unauthorized absences mean an automatic grade of 0 for the class. All slides will be made available on Canvas after the class. If you like to take notes, you may use pen and paper. 2 Activity This graph charts the energy consumption of different countries against their GDP per capita. (’GDP’ stands for ‘Gross Domestic Product’, and is a measure of the total monetary value of the goods and services produced within a country.) What does this graph tell us about the causal relationship between energy consumption and wealth? Recap: Inductive Generalization 4 Recap: Inductive Generalization Example: P1) 5% of sampled mosquitos carry dengue fever C) Therefore, 5% of mosquitos carry dengue fever 5 General Claims and Causation However, even if we know that, in general, As are Bs, this doesn’t tell us whether being an A causes something to be a B. Knowing that economic activity is correlated with high energy consumption doesn’t tell us that high energy consumption is a cause of wealth (or vice versa). Knowing that increased social media use is correlated with declining mental health doesn’t tell us that increased social media use causes poor mental health (or vice versa). Knowing that marijuana use is correlated with schizophrenia doesn’t tell us that marijuana use causes schizophrenia (or vice versa). 6 Causation Causes are difference-makers. If A causes B, then A makes a difference as to whether B happens. For instance, if striking a match causes it to light, then striking the match is what makes a difference as to whether the match lights. 7 Causation Causal knowledge is essential to life because it underpins subjunctive and counterfactual reasoning. A subjunctive is a claim of the form If it were the case that A, then it would be the case that B. If I were to turn the wheel clockwise, the car would turn right. If I were to eat peanuts, I would go into anaphylactic shock. If I didn’t care so much about others’ opinions, I wouldn’t feel so anxious. 8 Causation Causal knowledge is essential to life because it underpins subjunctive and counterfactual reasoning. A counterfactual is a claim of the form If it had been the case that A, then it would have been the case that B. If I had turned the wheel clockwise, the car would have turned right. If I had eaten peanuts, I would have gone into anaphylactic shock. If I hadn’t cared so much about others’ opinions, I wouldn’t have felt so anxious. 9 Causation Typically, the causes we care about are not complete or total causes. Notice that one of the causes of a match lighting is the presence of oxygen. When we say that striking the match caused it to light, we are not describing the complete causes of it lighting (which would also include (e.g.) the causal contribution of oxygen). 10 Causation Causal claims are very common, but often we don’t use the word ‘cause’. Rain prevented the game from taking place. The cat killed the mouse. I fed the dog. The policy improved the economy. The dish was ruined with too much salt. 11 Causation Some causal claims are particular. For example: Striking this match caused it to light. Dropping the vase caused it to break. The assassination of Archduke Franz Ferdinand caused the First World War. Other causal claims are general For example: Smoking causes cancer. Vaccinations prevent rabies. Carbon dioxide causes climate change. 12 Activity: Identifying Causes 13 Activity: Identifying Causes Discussion question: Why do women with very high IQs usually marry a partner with a lower IQ? In fact, this question can be answered without appealing to any cause. It is (more or less) statistically inevitable that anyone with a very high IQ who gets married will marry someone with a lower IQ. If someone has an IQ ≥ 145, then ≥ 99.9% of people will have a lower IQ than that person. 14 Identifying Causes System 1 is geared towards attributing causes, even when this is not justified. Seeing a striking correlation between As and Bs can make it very intuitive to hold that A causes B. But there are other possibilities: The correlation is by chance There is a structural (non-causal) explanation for the correlation B causes A There is some third factor C that causes both A and B 15 Identifying Causes Holding that A causes B merely because A is correlated with B is the post hoc ergo propter hoc fallacy (Latin for ‘after this, therefore because of this’). 16 Identifying Causes As the Scottish Enlightenment philosopher David Hume (1711-1776) argued, we cannot perceive causation. Imagine one billiard ball striking another. We see one ball move, touch another, then the other ball move. We perceive one event followed by another (correlation), but we do not perceive the cause itself. 17 Identifying Causes Problem: a) We shouldn’t infer causation from correlation. b) But our only evidence for causation could be from correlation. Solution: Particular patterns of correlations are evidence of causation. 18 Identifying Causes There are three fundamental means of identifying causes: the method of difference the method of agreement the method of concomitant variation 19 Identifying Causes Imagine you occasionally show symptoms of a food intolerance (e.g. stomach pain, nausea, fatigue) when eating. You want to know if dairy foods (milk, cheese etc.) cause these symptoms. Dependent variable B:= symptoms of food intolerance Independent variable A:= eating dairy 20 Method of Difference The method of difference involves comparing cases where the dependent variable B occurs (you experience the symptoms) to cases where B doesn’t occur. If the independent variable A (eating diary) is what is different about the cases where B occurs, this is evidence that A causes B. 21 Method of Agreement The method of agreement involves comparing multiple cases where dependent variable B occurs (you experience the symptoms). If the independent variable A (eating diary) is what all or many cases of B have in common, this is evidence that A causes B. 22 Method of Concomitant Variation The method of concomitant variation involves varying the amount of the independent variable A (eating dairy) and examining the amount of the dependent variable B (the symptoms). If the amount of dependent variable B (the symptoms) covaries with the amount of the independent variable A (eating dairy) this is evidence that A causes B. 23 Confounding Variables The role of the methods of difference, agreement, and concomitant variation is to rule out confounding variables. A confounding variable (or confound) is some factor C that correlates with either A or B, and might (for all we know) be the cause of B. 24 Confounding Variables Shoe size (independent variable A) correlates with reading ability in children (dependent variable B). Is this good evidence that large feet cause better reading ability? Question: What confounding variable C might there be in this case? 25 Confounding Variables Shoe size (independent variable A) correlates with reading ability in children (dependent variable B). Is this good evidence that large feet cause better reading ability? Question: What confounding variable C might there be in this case? Answer: The age of a child correlates with shoe size, and explains differences in reading ability. 26 Activity For each of the following correlations, identify (i) the suggested causal link, and (ii) a possible confound. 1. Breastfed babies have a reduced risk of sudden infant death syndrome. 2. People who listen to rock music when driving tend to drive faster. 3. People who exercise more are less likely to be depressed. 4. Those who use a sauna one a week live longer. 5. Students who study philosophy score higher on intelligence metrics. 27 Identifying Causes: Laboratories Some branches of science use laboratories to collect evidence for causal claims. The goal of a laboratory experiment is to remove, as far as possible, any potential confounds, by constructing a controlled environment. When well constructed, laboratory experiments allow scientists to isolate the cause of the dependent variable B. 28 Identifying Causes: Laboratories Research Question: Does the amount of light a plant receives affect its growth? Hypothesis: Plants that receive more light will grow taller than plants that receive less light. Experimental Setup: 1.Independent Variable A: The amount of light the plants receive (e.g., full light, half light, no light). 2.Dependent Variable B: The height of the plants after a set period (e.g., 2 weeks). 3.Control Variables: Factors that are kept constant to ensure fair comparison, such as: i. The type of plant (same species). ii. Amount of water given. iii. Type of soil. iv. Temperature of the room. v. Size of the pots. 29 Identifying Causes: Laboratories Prepare three groups of identical plants: Group 1: Plants receive full light (control group). Group 2: Plants receive half light (experimental group: partial exposure). Group 3: Plants receive no light (experimental group: complete darkness). Place the plants under their respective lighting conditions, ensuring that all other factors like water, soil, and temperature are kept the same. Measure the growth (height) of the plants every few days for two weeks. At the end of the experiment, compare the average height of plants in each group to determine the effect of different light levels on growth. Expected Outcome: If the hypothesis is correct, the plants exposed to full light will grow the tallest, while the plants with no light will show the least growth. 30 Identifying Causes Often though, we want to know whether causal claims are true, but cannot test them directly in a laboratory. For example: Smoking causes cancer. Vaccination A prevents disease B. Carbon dioxide causes climate change. Economic policy A causes GDP to grow. Here we must use other techniques to determine whether A makes a difference to B There is no confounding variable C that causes B (instead of A) 31 Activity Consider the following study. There is a new experimental medication for depression. The medication is given to a large number of people who report being depressed in January. After three months taking the medication, more than half the patients report an improvement in mood. 1. Think of three possible confounding variables that might explain the improvement in mood. 2. What could be done to eliminate these confounds? 32 Controlling for Confounds Consider the previous experiment. How might we control for possible confounds? How might we gain evidence that A (the medication) makes a difference to B (depression)? 33 Controlling for Confounds The experiment should contain: an experimental group (who take the medication), and a control group (who do not take the medication) It is then possible to measure whether there are different results for the experimental group and the control group. 34 Controlling for Confounds We need to prevent selection biases that may act as confounds. For example, it may be that people who are more optimistic are more likely to opt to take an experimental medication. But perhaps people who are more optimistic are also more likely to recover from depression within three months. If the experimental group is more optimistic than the control group, this could skew the results. 35 Controlling for Confounds The experimenter can mitigate selection bias by randomly assigning people to the control group and experimental group. If this is not possible, the experimenter can mitigate selection bias by comparing like-for-like (e.g. comparing the results of non-optimistic people in the control group and non-optimistic people in the experimental group). (Notice that we can only control for possible confounds that we have thought of. This is one reason critical thinking requires imagination and creative thinking.) 36 Controlling for Confounds Another possible confound that should be controlled for is the placebo effect. The placebo effect: It is well documented that just thinking you have taken a medication can effect a person’s medical outcome; the expectation that they will get better can itself cause them to get better. 37 Controlling for Confounds To control for this possible confound, subjects in the control group and the experimental group should not know which group they are in. This is called a single-blind experiment. Typically, subjects in the control group will be given a placebo control: a fake medication that looks identical to, and is administered in an identical way to the real medication. 38 Controlling for Confounds The experimenters knowing which groups the subjects are in can also act as a confound. The experimenters may interpret the subjects’ behaviour differently. The experimenters may (perhaps inadvertently) influence the subjects’ behaviour. 39 Controlling for Confounds To control for this possible confound, the experimenters should not know which groups the subjects are in. When neither the subjects nor the experimenters know which groups the subjects are in, it is called a double-blind experiment. 40 Very Weak Support for a Causal Claim ANECDOTAL CORRELATION It seems to me that there is a correlation between A and B. 41 Weak Support for a Causal Claim STUDY WITHOUT CONTROLS A study has carefully collected data (from an appropriately large group) showing that A correlates with B, but without controlling for confounds. 42 Modest Support for a Causal Claim STUDY WITH SOME CONTROLS A study has carefully collected data (from an appropriately large group) showing that A correlates with B, and attempting to control for some confounds. 43 Stronger Support for a Causal Claim STUDY WITH MORE EXTENSIVE CONTROLS A study has carefully collected data (from an appropriately large group) showing that A correlates with B, has randomly assigned subjects to the control group and experimental group, has used placebos and double-blind experimental design to attempt to control for confounds. 44 Activity Design a controlled experiment to decide whether caffeine consumption causes better memory retention. Although you won’t actually carry out the experiment, you can use the VinUni population as your subjects. Think about: i. What data you will collect ii. What variables you can control for (and how) iii. How you would assess whether caffeine consumption causes better memory retention 45