Cohen's D Effect Size Statistics PDF
Document Details
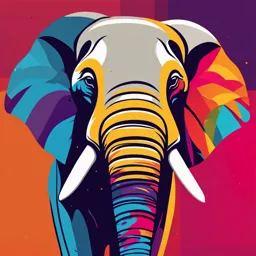
Uploaded by LargeCapacityAntigorite4770
Tata Memorial Centre
Danielle J. Navarro
Tags
Summary
This PDF document is a chapter from a book likely on statistics. The chapter discusses Cohen's d as an effect size measure, its applications in t-tests (including paired-samples, and Welch tests), and different ways to interpret it.
Full Transcript
set up to handle data in long form. Instead it expects to be given two separate variables, x and y, and you need to specify paired=TRUE. And on top of that, you’d better make sure that the first element of x and the first element of y actually correspond to the same person! Because it doesn’t ask fo...
set up to handle data in long form. Instead it expects to be given two separate variables, x and y, and you need to specify paired=TRUE. And on top of that, you’d better make sure that the first element of x and the first element of y actually correspond to the same person! Because it doesn’t ask for an “id” variable. I don’t know why. So, in order to run the paired samples t test on the data from Dr Chico’s class, we’d use this command: > t.test( x = chico$grade_test2, # variable 1 is the "test2" scores + y = chico$grade_test1, # variable 2 is the "test1" scores + paired = TRUE # paired test + ) and the output is Paired t-test data: chico$grade_test2 and chico$grade_test1 t = 6.4754, df = 19, p-value = 3.321e-06 alternative hypothesis: true difference in means is not equal to 0 95 percent confidence interval: 0.9508686 1.8591314 sample estimates: mean of the differences 1.405 Yet again, these are the same numbers that we saw in Section 13.5. Feel free to check. 13.8 E↵ect size The most commonly used measure of e↵ect size for a t-test is Cohen’s d (Cohen, 1988). It’s a very simple measure in principle, with quite a few wrinkles when you start digging into the details. Cohen himself defined it primarily in the context of an independent samples t-test, specifically the Student test. In that context, a natural way of defining the e↵ect size is to divide the di↵erence between the means by an estimate of the standard deviation. In other words, we’re looking to calculate something along the lines of this: (mean 1) ´ (mean 2) d“ std dev and he suggested a rough guide for interpreting d in Table 13.1. You’d think that this would be pretty unambiguous, but it’s not; largely because Cohen wasn’t too specific on what he thought should be used as the measure of the standard deviation (in his defence, he was trying to make a broader point in his book, not nitpick about tiny details). As discussed by McGrath and Meyer (2006), there are several di↵erent version in common usage, and each author tends to adopt slightly di↵erent notation. For the sake of simplicity (as opposed to accuracy) I’ll use d to refer to any statistic that you calculate from the sample, and use to refer to a theoretical population e↵ect. Obviously, that does mean that there are several di↵erent things all called d. The cohensD() function in the lsr package uses the method argument to distinguish between them, so that’s what I’ll do in the text. My suspicion is that the only time that you would want Cohen’s d is when you’re running a t-test, and if you’re using the oneSampleTTest, independentSamplesTTest and pairedSamplesTTest() functions to run your t-tests, then you don’t need to learn any new commands, because they automatically produce - 412 - Table 13.1: A (very) rough guide to interpreting Cohen’s d. My personal recommendation is to not use these blindly. The d statistic has a natural interpretation in and of itself: it redescribes the di↵erent in means as the number of standard deviations that separates those means. So it’s generally a good idea to think about what that means in practical terms. In some contexts a “small” e↵ect could be of big practical importance. In other situations a “large” e↵ect may not be all that interesting. d-value rough interpretation about 0.2 “small” e↵ect about 0.5 “moderate” e↵ect about 0.8 “large” e↵ect....................................................................................................... an estimate of Cohen’s d as part of the output. However, if you’re using t.test() then you’ll need to use the cohensD() function (also in the lsr package) to do the calculations. 13.8.1 Cohen’s d from one sample The simplest situation to consider is the one corresponding to a one-sample t-test. In this case, the one sample mean X̄ and one (hypothesised) population mean µo to compare it to. Not only that, there’s really only one sensible way to estimate the population standard deviation: we just use our usual estimate ˆ. Therefore, we end up with the following as the only way to calculate d, X̄ ´ µ0 d“ ˆ When writing the cohensD() function, I’ve made some attempt to make it work in a similar way to t.test(). As a consequence, cohensD() can calculate your e↵ect size regardless of which type of t-test you performed. If what you want is a measure of Cohen’s d to accompany a one-sample t-test, there’s only two arguments that you need to care about. These are: x. A numeric vector containing the sample data. mu. The mean against which the mean of x is compared (default value is mu = 0). We don’t need to specify what method to use, because there’s only one version of d that makes sense in this context. So, in order to compute an e↵ect size for the data from Dr Zeppo’s class (Section 13.2), we’d type something like this: > cohensD( x = grades, # data are stored in the grades vector + mu = 67.5 # compare students to a mean of 67.5 + ) 0.5041691 and, just so that you can see that there’s nothing fancy going on, the command below shows you how to calculate it if there weren’t no fancypants cohensD() function available: > ( mean(grades) - 67.5 ) / sd(grades) 0.5041691 Yep, same number. Overall, then, the psychology students in Dr Zeppo’s class are achieving grades (mean = 72.3%) that are about.5 standard deviations higher than the level that you’d expect (67.5%) if they were performing at the same level as other students. Judged against Cohen’s rough guide, this is a moderate e↵ect size. - 413 - 13.8.2 Cohen’s d from a Student t test The majority of discussions of Cohen’s d focus on a situation that is analogous to Student’s inde- pendent samples t test, and it’s in this context that the story becomes messier, since there are several di↵erent versions of d that you might want to use in this situation, and you can use the method argument to the cohensD() function to pick the one you want. To understand why there are multiple versions of d, it helps to take the time to write down a formula that corresponds to the true population e↵ect size. It’s pretty straightforward, µ1 ´ µ2 “ where, as usual, µ1 and µ2 are the population means corresponding to group 1 and group 2 respectively, and is the standard deviation (the same for both populations). The obvious way to estimate is to do exactly the same thing that we did in the t-test itself: use the sample means as the top line, and a pooled standard deviation estimate for the bottom line: X̄1 ´ X̄2 d“ ˆp where ˆp is the exact same pooled standard deviation measure that appears in the t-test. This is the most commonly used version of Cohen’s d when applied to the outcome of a Student t-test ,and is sometimes referred to as Hedges’ g statistic (Hedges, 1981). It corresponds to method = "pooled" in the cohensD() function, and it’s the default. However, there are other possibilities, which I’ll briefly describe. Firstly, you may have reason to want to use only one of the two groups as the basis for calculating the standard deviation. This approach (often called Glass’ ) only makes most sense when you have good reason to treat one of the two groups as a purer reflection of “natural variation” than the other. This can happen if, for instance, one of the two groups is a control group. If that’s what you want, then use method = "x.sd" or method = "y.sd" when using cohensD(). Secondly, recall that in the usual calculation of the pooled standard deviation we divide by N ´ 2 to correct for the bias in the sample variance; in one version of Cohen’s d this correction is omitted. Instead, we divide by N. This version (method = "raw") makes sense primarily when you’re trying to calculate the e↵ect size in the sample; rather than estimating an e↵ect size in the population. Finally, there is a version based on Hedges and Olkin (1985), who point out there is a small bias in the usual (pooled) estimation for Cohen’s d. Thus they introduce a small correction (method = "corrected"), by multiplying the usual value of d by pN ´ 3q{pN ´ 2.25q. In any case, ignoring all those variations that you could make use of if you wanted, let’s have a look at how to calculate the default version. In particular, suppose we look at the data from Dr Harpo’s class (the harpo data frame). The command that we want to use is very similar to the relevant t.test() command, but also specifies a method > cohensD( formula = grade ~ tutor, # outcome ~ group + data = harpo, # data frame + method = "pooled" # which version to calculate? + ) 0.7395614 This is the version of Cohen’s d that gets reported by the independentSamplesTTest() function whenever it runs a Student t-test. 13.8.3 Cohen’s d from a Welch test Suppose the situation you’re in is more like the Welch test: you still have two independent samples, - 414 - but you no longer believe that the corresponding populations have equal variances. When this happens, we have to redefine what we mean by the population e↵ect size. I’ll refer to this new measure as 1 , so as to keep it distinct from the measure which we defined previously. What Cohen (1988) suggests is that we could define our new population e↵ect size by averaging the two population variances. What this means is that we get: 1 µ1 ´ µ2 “ 1 where c 1 ` 22 1 2 “ 2 This seems quite reasonable, but notice that none of the measures that we’ve discussed so far are at- tempting to estimate this new quantity. It might just be my own ignorance of the topic, but I’m only aware of one version of Cohen’s d that actually estimates the unequal-variance e↵ect size 1 rather than the equal-variance e↵ect size. All we do to calculate d for this version (method = "unequal") is substitute the sample means X̄1 and X̄2 and the corrected sample standard deviations ˆ1 and ˆ2 into the equation for 1. This gives us the following equation for d, X̄1 ´ X̄2 d“ c ˆ12 ` ˆ22 2 as our estimate of the e↵ect size. There’s nothing particularly difficult about calculating this version in R, since all we have to do is change the method argument: > cohensD( formula = grade ~ tutor, + data = harpo, + method = "unequal" + ) 0.7244995 his is the version of Cohen’s d that gets reported by the independentSamplesTTest() function whenever it runs a Welch t-test. 13.8.4 Cohen’s d from a paired-samples test Finally, what should we do for a paired samples t-test? In this case, the answer depends on what it is you’re trying to do. If you want to measure your e↵ect sizes relative to the distribution of di↵erence scores, the measure of d that you calculate is just (method = "paired") D̄ d“ ˆD where ˆD is the estimate of the standard deviation of the di↵erences. The calculation here is pretty straightforward > cohensD( x = chico$grade_test2, + y = chico$grade_test1, + method = "paired" + ) 1.447952 This is the version of Cohen’s d that gets reported by the pairedSamplesTTest() function. The only wrinkle is figuring out whether this is the measure you want or not. To the extent that you care about - 415 - the practical consequences of your research, you often want to measure the e↵ect size relative to the original variables, not the di↵erence scores (e.g., the 1% improvement in Dr Chico’s class is pretty small when measured against the amount of between-student variation in grades), in which case you use the same versions of Cohen’s d that you would use for a Student or Welch test. For instance, when we do that for Dr Chico’s class, > cohensD( x = chico$grade_test2, + y = chico$grade_test1, + method = "pooled" + ) 0.2157646 what we see is that the overall e↵ect size is quite small, when assessed on the scale of the original variables. 13.9 Checking the normality of a sample All of the tests that we have discussed so far in this chapter have assumed that the data are normally distributed. This assumption is often quite reasonable, because the central limit theorem (Section 10.3.3) does tend to ensure that many real world quantities are normally distributed: any time that you suspect that your variable is actually an average of lots of di↵erent things, there’s a pretty good chance that it will be normally distributed; or at least close enough to normal that you can get away with using t-tests. However, life doesn’t come with guarantees; and besides, there are lots of ways in which you can end up with variables that are highly non-normal. For example, any time you think that your variable is actually the minimum of lots of di↵erent things, there’s a very good chance it will end up quite skewed. In psychology, response time (RT) data is a good example of this. If you suppose that there are lots of things that could trigger a response from a human participant, then the actual response will occur the first time one of these trigger events occurs.16 This means that RT data are systematically non-normal. Okay, so if normality is assumed by all the tests, and is mostly but not always satisfied (at least approximately) by real world data, how can we check the normality of a sample? In this section I discuss two methods: QQ plots, and the Shapiro-Wilk test. 13.9.1 QQ plots One way to check whether a sample violates the normality assumption is to draw a “quantile-quantile” plot (QQ plot). This allows you to visually check whether you’re seeing any systematic violations. In a QQ plot, each observation is plotted as a single dot. The x co-ordinate is the theoretical quantile that the observation should fall in, if the data were normally distributed (with mean and variance estimated from the sample) and on the y co-ordinate is the actual quantile of the data within the sample. If the data are normal, the dots should form a straight line. For instance, lets see what happens if we generate data by sampling from a normal distribution, and then drawing a QQ plot using the R function qqnorm(). The qqnorm() function has a few arguments, but the only one we really need to care about here is y, a vector specifying the data whose normality we’re interested in checking. Here’s the R commands: > normal.data hist( x = normal.data ) # draw a histogram of these numbers > qqnorm( y = normal.data ) # draw the QQ plot 16 This is a massive oversimplification. - 416 - Normally Distributed Data Normal Q−Q Plot 2 20 Sample Quantiles 1 15 Frequency 0 10 −1 5 −2 0 −2 −1 0 1 2 −2 −1 0 1 2 Value Theoretical Quantiles (a) (b) Figure 13.11: Histogram (panel a) and normal QQ plot (panel b) of normal.data, a normally distributed sample with 100 observations. The Shapiro-Wilk statistic associated with these data is W “.99, indi- cating that no significant departures from normality were detected (p “.73)........................................................................................................ And the results are shown in Figure 13.11. As you can see, these data form a pretty straight line; which is no surprise given that we sampled them from a normal distribution! In contrast, have a look at the two data sets shown in Figure 13.12. The top panels show the histogram and a QQ plot for a data set that is highly skewed: the QQ plot curves upwards. The lower panels show the same plots for a heavy tailed (i.e., high kurtosis) data set: in this case, the QQ plot flattens in the middle and curves sharply at either end. 13.9.2 Shapiro-Wilk tests Although QQ plots provide a nice way to informally check the normality of your data, sometimes you’ll want to do something a bit more formal. And when that moment comes, the Shapiro-Wilk test (Shapiro & Wilk, 1965) is probably what you’re looking for.17 As you’d expect, the null hypothesis being tested is that a set of N observations is normally distributed. The test statistic that it calculates is conventionally denoted as W , and it’s calculated as follows. First, we sort the observations in order of increasing size, and let X1 be the smallest value in the sample, X2 be the second smallest and so on. Then the value of W is given by ´∞ ¯2 N i“1 a i X i W “ ∞N i“1 pXi ´ X̄q 2 where X̄ is the mean of the observations, and the ai values are... mumble, mumble... something complicated that is a bit beyond the scope of an introductory text. 17 Either that, or the Kolmogorov-Smirnov test, which is probably more traditional than the Shapiro-Wilk, though most things I’ve read seem to suggest Shapiro-Wilk is the better test of normality; although Kolomogorov-Smirnov is a general purpose test of distributional equivalence, so it can be adapted to handle other kinds of distribution tests; in R it’s implemented via the ks.test() function. - 417 - Skewed Data Normal Q−Q Plot 10 20 30 40 50 60 2.0 Sample Quantiles Frequency 1.5 1.0 0.5 0.0 0 0.0 0.5 1.0 1.5 2.0 2.5 −2 −1 0 1 2 Value Theoretical Quantiles (a) (b) Heavy−Tailed Data Normal Q−Q Plot 40 6 Sample Quantiles 4 30 Frequency 2 20 0 −6 −4 −2 10 0 −6 −4 −2 0 2 4 6 8 −2 −1 0 1 2 Value Theoretical Quantiles (c) (d) Figure 13.12: In the top row, a histogram (panel a) and normal QQ plot (panel b) of the 100 observations in a skewed.data set. The skewness of the data here is 1.94, and is reflected in a QQ plot that curves upwards. As a consequence, the Shapiro-Wilk statistic is W “.80, reflecting a significant departure from normality (p †.001). The bottom row shows the same plots for a heavy tailed data set, again consisting of 100 observations. In this case, the heavy tails in the data produce a high kurtosis (2.80), and cause the QQ plot to flatten in the middle, and curve away sharply on either side. The resulting Shapiro-Wilk statistic is W “.93, again reflecting significant non-normality (p †.001)........................................................................................................ - 418 - Sampling distribution of W (for normally distributed data) N = 10 N = 20 N = 50 0.75 0.80 0.85 0.90 0.95 1.00 Value of W Figure 13.13: Sampling distribution of the Shapiro-Wilk W statistic, under the null hypothesis that the data are normally distributed, for samples of size 10, 20 and 50. Note that small values of W indicate departure from normality........................................................................................................ Because it’s a little hard to explain the maths behind the W statistic, a better idea is to give a broad brush description of how it behaves. Unlike most of the test statistics that we’ll encounter in this book, it’s actually small values of W that indicated departure from normality. The W statistic has a maximum value of 1, which arises when the data look “perfectly normal”. The smaller the value of W , the less normal the data are. However, the sampling distribution for W – which is not one of the standard ones that I discussed in Chapter 9 and is in fact a complete pain in the arse to work with – does depend on the sample size N. To give you a feel for what these sampling distributions look like, I’ve plotted three of them in Figure 13.13. Notice that, as the sample size starts to get large, the sampling distribution becomes very tightly clumped up near W “ 1, and as a consequence, for larger samples W doesn’t have to be very much smaller than 1 in order for the test to be significant. To run the test in R, we use the shapiro.test() function. It has only a single argument x, which is a numeric vector containing the data whose normality needs to be tested. For example, when we apply this function to our normal.data, we get the following: > shapiro.test( x = normal.data ) Shapiro-Wilk normality test data: normal.data W = 0.9903, p-value = 0.6862 So, not surprisingly, we have no evidence that these data depart from normality. When reporting the results for a Shapiro-Wilk test, you should (as usual) make sure to include the test statistic W and the p value, though given that the sampling distribution depends so heavily on N it would probably be a politeness to include N as well. - 419 - 13.10 Testing non-normal data with Wilcoxon tests Okay, suppose your data turn out to be pretty substantially non-normal, but you still want to run something like a t-test? This situation occurs a lot in real life: for the AFL winning margins data, for instance, the Shapiro-Wilk test made it very clear that the normality assumption is violated. This is the situation where you want to use Wilcoxon tests. Like the t-test, the Wilcoxon test comes in two forms, one-sample and two-sample, and they’re used in more or less the exact same situations as the corresponding t-tests. Unlike the t-test, the Wilcoxon test doesn’t assume normality, which is nice. In fact, they don’t make any assumptions about what kind of distribution is involved: in statistical jargon, this makes them nonparametric tests. While avoiding the normality assumption is nice, there’s a drawback: the Wilcoxon test is usually less powerful than the t-test (i.e., higher Type II error rate). I won’t discuss the Wilcoxon tests in as much detail as the t-tests, but I’ll give you a brief overview. 13.10.1 Two sample Wilcoxon test I’ll start by describing the two sample Wilcoxon test (also known as the Mann-Whitney test), since it’s actually simpler than the one sample version. Suppose we’re looking at the scores of 10 people on some test. Since my imagination has now failed me completely, let’s pretend it’s a “test of awesomeness”, and there are two groups of people, “A” and “B”. I’m curious to know which group is more awesome. The data are included in the file awesome.Rdata, and like many of the data sets I’ve been using, it contains only a single data frame, in this case called awesome. Here’s the data: > load("awesome.Rdata") > print( awesome ) scores group 1 6.4 A 2 10.7 A 3 11.9 A 4 7.3 A 5 10.0 A 6 14.5 B 7 10.4 B 8 12.9 B 9 11.7 B 10 13.0 B As long as there are no ties (i.e., people with the exact same awesomeness score), then the test that we want to do is surprisingly simple. All we have to do is construct a table that compares every observation in group A against every observation in group B. Whenever the group A datum is larger, we place a check mark in the table: group B 14.5 10.4 12.4 11.7 13.0 6.4..... 10.7. X... group A 11.9. X. X. 7.3..... 10.0..... - 420 - We then count up the number of checkmarks. This is our test statistic, W.18 The actual sampling distribution for W is somewhat complicated, and I’ll skip the details. For our purposes, it’s sufficient to note that the interpretation of W is qualitatively the same as the interpretation of t or z. That is, if we want a two-sided test, then we reject the null hypothesis when W is very large or very small; but if we have a directional (i.e., one-sided) hypothesis, then we only use one or the other. The structure of the wilcox.test() function should feel very familiar to you by now. When you have your data organised in terms of an outcome variable and a grouping variable, then you use the formula and data arguments, so your command looks like this: > wilcox.test( formula = scores ~ group, data = awesome) Wilcoxon rank sum test data: scores by group W = 3, p-value = 0.05556 alternative hypothesis: true location shift is not equal to 0 Just like we saw with the t.test() function, there is an alternative argument that you can use to switch between two-sided tests and one-sided tests, plus a few other arguments that we don’t need to worry too much about at an introductory level. Similarly, the wilcox.test() function allows you to use the x and y arguments when you have your data stored separately for each group. For instance, suppose we use the data from the awesome2.Rdata file: > load( "awesome2.Rdata" ) > score.A 6.4 10.7 11.9 7.3 10.0 > score.B 14.5 10.4 12.9 11.7 13.0 When your data are organised like this, then you would use a command like this: > wilcox.test( x = score.A, y = score.B ) The output that R produces is pretty much the same as last time. 13.10.2 One sample Wilcoxon test What about the one sample Wilcoxon test (or equivalently, the paired samples Wilcoxon test)? Suppose I’m interested in finding out whether taking a statistics class has any e↵ect on the happiness of students. Here’s my data: > load( "happy.Rdata" ) > print( happiness ) before after change 1 30 6 -24 2 43 29 -14 3 21 11 -10 4 24 31 7 5 23 17 -6 6 40 2 -38 18 Actually, there are two di↵erent versions of the test statistic; they di↵er from each other by a constant value. The version that I’ve described is the one that R calculates. - 421 - 7 29 31 2 8 56 21 -35 9 38 8 -30 10 16 21 5 What I’ve measured here is the happiness of each student before taking the class and after taking the class; the change score is the di↵erence between the two. Just like we saw with the t-test, there’s no fundamental di↵erence between doing a paired-samples test using before and after, versus doing a one- sample test using the change scores. As before, the simplest way to think about the test is to construct a tabulation. The way to do it this time is to take those change scores that are positive valued, and tabulate them against all the complete sample. What you end up with is a table that looks like this: all di↵erences -24 -14 -10 7 -6 -38 2 -35 -30 5 7... X X. X.. X positive di↵erences 2...... X... 5...... X.. X Counting up the tick marks this time, we get a test statistic of V “ 7. As before, if our test is two sided, then we reject the null hypothesis when V is very large or very small. As far of running it in R goes, it’s pretty much what you’d expect. For the one-sample version, the command you would use is > wilcox.test( x = happiness$change, + mu = 0 + ) and the output that you get is Wilcoxon signed rank test data: happiness$change V = 7, p-value = 0.03711 alternative hypothesis: true location is not equal to 0 As this shows, we have a significant e↵ect. Evidently, taking a statistics class does have an e↵ect on your happiness. Switching to a paired samples version of the test won’t give us di↵erent answers, of course; but here’s the command to do it: > wilcox.test( x = happiness$after, + y = happiness$before, + paired = TRUE + ) 13.11 Summary A one sample t-test is used to compare a single sample mean against a hypothesised value for the population mean. (Section 13.2) An independent samples t-test is used to compare the means of two groups, and tests the null hypothesis that they have the same mean. It comes in two forms: the Student test (Section 13.3) assumes that the groups have the same standard deviation, the Welch test (Section 13.4) does not. - 422 - A paired samples t-test is used when you have two scores from each person, and you want to test the null hypothesis that the two scores have the same mean. It is equivalent to taking the di↵erence between the two scores for each person, and then running a one sample t-test on the di↵erence scores. (Section 13.5) E↵ect size calculations for the di↵erence between means can be calculated via the Cohen’s d statistic. (Section 13.8). You can check the normality of a sample using QQ plots and the Shapiro-Wilk test. (Section 13.9) If your data are non-normal, you can use Wilcoxon tests instead of t-tests. (Section 13.10) - 423 - - 424 - 14. Comparing several means (one-way ANOVA) This chapter introduces one of the most widely used tools in statistics, known as “the analysis of variance”, which is usually referred to as ANOVA. The basic technique was developed by Sir Ronald Fisher in the early 20th century, and it is to him that we owe the rather unfortunate terminology. The term ANOVA is a little misleading, in two respects. Firstly, although the name of the technique refers to variances, ANOVA is concerned with investigating di↵erences in means. Secondly, there are several di↵erent things out there that are all referred to as ANOVAs, some of which have only a very tenuous connection to one another. Later on in the book we’ll encounter a range of di↵erent ANOVA methods that apply in quite di↵erent situations, but for the purposes of this chapter we’ll only consider the simplest form of ANOVA, in which we have several di↵erent groups of observations, and we’re interested in finding out whether those groups di↵er in terms of some outcome variable of interest. This is the question that is addressed by a one-way ANOVA. The structure of this chapter is as follows: In Section 14.1 I’ll introduce a fictitious data set that we’ll use as a running example throughout the chapter. After introducing the data, I’ll describe the mechanics of how a one-way ANOVA actually works (Section 14.2) and then focus on how you can run one in R (Section 14.3). These two sections are the core of the chapter. The remainder of the chapter discusses a range of important topics that inevitably arise when running an ANOVA, namely how to calculate e↵ect sizes (Section 14.4), post hoc tests and corrections for multiple comparisons (Section 14.5) and the assumptions that ANOVA relies upon (Section 14.6). We’ll also talk about how to check those assumptions and some of the things you can do if the assumptions are violated (Sections 14.7 to 14.10). At the end of the chapter we’ll talk a little about the relationship between ANOVA and other statistical tools (Section 14.11). 14.1 An illustrative data set Suppose you’ve become involved in a clinical trial in which you are testing a new antidepressant drug called Joyzepam. In order to construct a fair test of the drug’s e↵ectiveness, the study involves three separate drugs to be administered. One is a placebo, and the other is an existing antidepressant / anti-anxiety drug called Anxifree. A collection of 18 participants with moderate to severe depression are recruited for your initial testing. Because the drugs are sometimes administered in conjunction with psychological therapy, your study includes 9 people undergoing cognitive behavioural therapy (CBT) and 9 who are not. Participants are randomly assigned (doubly blinded, of course) a treatment, such that there are 3 CBT people and 3 no-therapy people assigned to each of the 3 drugs. A psychologist assesses the mood of each person after a 3 month run with each drug: and the overall improvement in each person’s mood is assessed on a scale ranging from ´5 to `5. - 425 - With that as the study design, let’s now look at what we’ve got in the data file: > load( "clinicaltrial.Rdata" ) # load data > who( expand = TRUE ) # inspect the workspace -- Name -- -- Class -- -- Size -- clin.trial data.frame 18 x 3 $drug factor 18 $therapy factor 18 $mood.gain numeric 18 So we have a single data frame called clin.trial, containing three variables; drug, therapy and mood.gain. Next, let’s print the data frame to get a sense of what the data actually look like. > print( clin.trial ) drug therapy mood.gain 1 placebo no.therapy 0.5 2 placebo no.therapy 0.3 3 placebo no.therapy 0.1 4 anxifree no.therapy 0.6 5 anxifree no.therapy 0.4 6 anxifree no.therapy 0.2 7 joyzepam no.therapy 1.4 8 joyzepam no.therapy 1.7 9 joyzepam no.therapy 1.3 10 placebo CBT 0.6 11 placebo CBT 0.9 12 placebo CBT 0.3 13 anxifree CBT 1.1 14 anxifree CBT 0.8 15 anxifree CBT 1.2 16 joyzepam CBT 1.8 17 joyzepam CBT 1.3 18 joyzepam CBT 1.4 For the purposes of this chapter, what we’re really interested in is the e↵ect of drug on mood.gain. The first thing to do is calculate some descriptive statistics and draw some graphs. In Chapter 5 we discussed a variety of di↵erent functions that can be used for this purpose. For instance, we can use the xtabs() function to see how many people we have in each group: > xtabs( ~drug, clin.trial ) drug placebo anxifree joyzepam 6 6 6 Similarly, we can use the aggregate() function to calculate means and standard deviations for the mood.gain variable broken down by which drug was administered: > aggregate( mood.gain ~ drug, clin.trial, mean ) drug mood.gain 1 placebo 0.4500000 2 anxifree 0.7166667 3 joyzepam 1.4833333 > aggregate( mood.gain ~ drug, clin.trial, sd ) drug mood.gain - 426 - 1.5 Mood Gain 1.0 0.5 placebo anxifree joyzepam Drug Administered Figure 14.1: Average mood gain as a function of drug administered. Error bars depict 95% confidence intervals associated with each of the group means........................................................................................................ 1 placebo 0.2810694 2 anxifree 0.3920034 3 joyzepam 0.2136976 Finally, we can use plotmeans() from the gplots package to produce a pretty picture. > library(gplots) > plotmeans( formula = mood.gain ~ drug, # plot mood.gain by drug + data = clin.trial, # the data frame + xlab = "Drug Administered", # x-axis label + ylab = "Mood Gain", # y-axis label + n.label = FALSE # don’t display sample size + ) The results are shown in Figure 14.1, which plots the average mood gain for all three conditions; error bars show 95% confidence intervals. As the plot makes clear, there is a larger improvement in mood for participants in the Joyzepam group than for either the Anxifree group or the placebo group. The Anxifree group shows a larger mood gain than the control group, but the di↵erence isn’t as large. The question that we want to answer is: are these di↵erence “real”, or are they just due to chance? 14.2 How ANOVA works In order to answer the question posed by our clinical trial data, we’re going to run a one-way ANOVA. As usual, I’m going to start by showing you how to do it the hard way, building the statistical tool from - 427 - the ground up and showing you how you could do it in R if you didn’t have access to any of the cool built-in ANOVA functions. And, as always, I hope you’ll read it carefully, try to do it the long way once or twice to make sure you really understand how ANOVA works, and then – once you’ve grasped the concept – never ever do it this way again. The experimental design that I described in the previous section strongly suggests that we’re interested in comparing the average mood change for the three di↵erent drugs. In that sense, we’re talking about an analysis similar to the t-test (Chapter 13), but involving more than two groups. If we let µP denote the population mean for the mood change induced by the placebo, and let µA and µJ denote the corresponding means for our two drugs, Anxifree and Joyzepam, then the (somewhat pessimistic) null hypothesis that we want to test is that all three population means are identical: that is, neither of the two drugs is any more e↵ective than a placebo. Mathematically, we write this null hypothesis like this: H0 : it is true that µP “ µA “ µJ As a consequence, our alternative hypothesis is that at least one of the three di↵erent treatments is di↵erent from the others. It’s a little trickier to write this mathematically, because (as we’ll discuss) there are quite a few di↵erent ways in which the null hypothesis can be false. So for now we’ll just write the alternative hypothesis like this: H1 : it is not true that µP “ µA “ µJ This null hypothesis is a lot trickier to test than any of the ones we’ve seen previously. How shall we do it? A sensible guess would be to “do an ANOVA”, since that’s the title of the chapter, but it’s not particularly clear why an “analysis of variances” will help us learn anything useful about the means. In fact, this is one of the biggest conceptual difficulties that people have when first encountering ANOVA. To see how this works, I find it most helpful to start by talking about variances. In fact, what I’m going to do is start by playing some mathematical games with the formula that describes the variance. That is, we’ll start out by playing around with variances, and it will turn out that this gives us a useful tool for investigating means. 14.2.1 Two formulas for the variance of Y Firstly, let’s start by introducing some notation. We’ll use G to refer to the total number of groups. For our data set, there are three drugs, so there are G “ 3 groups. Next, we’ll use N to refer to the total sample size: there are a total of N “ 18 people in our data set. Similarly, let’s use Nk to denote the number of people in the k-th group. In our fake clinical trial, the sample size is Nk “ 6 for all three groups.1 Finally, we’ll use Y to denote the outcome variable: in our case, Y refers to mood change. Specifically, we’ll use Yik to refer to the mood change experienced by the i-th member of the k-th group. Similarly, we’ll use Ȳ to be the average mood change, taken across all 18 people in the experiment, and Ȳk to refer to the average mood change experienced by the 6 people in group k. Excellent. Now that we’ve got our notation sorted out, we can start writing down formulas. To start with, let’s recall the formula for the variance that we used in Section 5.2, way back in those kinder days when we were just doing descriptive statistics. The sample variance of Y is defined as follows: G N 1 ÿ ÿk ` ˘2 VarpY q “ Yik ´ Ȳ N k“1 i“1 1 When all groups have the same number of observations, the experimental design is said to be “balanced”. Balance isn’t such a big deal for one-way ANOVA, which is the topic of this chapter. It becomes more important when you start doing more complicated ANOVAs. - 428 - This formula looks pretty much identical to the formula for the variance in Section 5.2. The only di↵erence is that this time around I’ve got two summations here: I’m summing over groups (i.e., values for k) and over the people within the groups (i.e., values for i). This is purely a cosmetic detail: if I’d instead used the notation Yp to refer to the value of the outcome variable for person p in the sample, then I’d only have a single summation. The only reason that we have a double summation here is that I’ve classified people into groups, and then assigned numbers to people within groups. A concrete example might be useful here. Let’s consider this table, in which we have a total of N “ 5 people sorted into G “ 2 groups. Arbitrarily, let’s say that the “cool” people are group 1, and the “uncool” people are group 2, and it turns out that we have three cool people (N1 “ 3) and two uncool people (N2 “ 2). name person group group num. index in group grumpiness p k i Yik or Yp Ann 1 cool 1 1 20 Ben 2 cool 1 2 55 Cat 3 cool 1 3 21 Dan 4 uncool 2 1 91 Egg 5 uncool 2 2 22 Notice that I’ve constructed two di↵erent labelling schemes here. We have a “person” variable p, so it would be perfectly sensible to refer to Yp as the grumpiness of the p-th person in the sample. For instance, the table shows that Dan is the four so we’d say p “ 4. So, when talking about the grumpiness Y of this “Dan” person, whoever he might be, we could refer to his grumpiness by saying that Yp “ 91, for person p “ 4 that is. However, that’s not the only way we could refer to Dan. As an alternative we could note that Dan belongs to the “uncool” group (k “ 2), and is in fact the first person listed in the uncool group (i “ 1). So it’s equally valid to refer to Dan’s grumpiness by saying that Yik “ 91, where k “ 2 and i “ 1. In other words, each person p corresponds to a unique ik combination, and so the formula that I gave above is actually identical to our original formula for the variance, which would be 1 ÿ` N ˘2 VarpY q “ Yp ´ Ȳ N p“1 In both formulas, all we’re doing is summing over all of the observations in the sample. Most of the time we would just use the simpler Yp notation: the equation using Yp is clearly the simpler of the two. However, when doing an ANOVA it’s important to keep track of which participants belong in which groups, and we need to use the Yik notation to do this. 14.2.2 From variances to sums of squares Okay, now that we’ve got a good grasp on how the variance is calculated, let’s define something called the total sum of squares, which is denoted SStot. This is very simple: instead of averaging the squared deviations, which is what we do when calculating the variance, we just add them up. So the formula for the total sum of squares is almost identical to the formula for the variance: ÿ Nk G ÿ ` ˘2 SStot “ Yik ´ Ȳ k“1 i“1 When we talk about analysing variances in the context of ANOVA, what we’re really doing is working with the total sums of squares rather than the actual variance. One very nice thing about the total sum of squares is that we can break it up into two di↵erent kinds of variation. Firstly, we can talk about the - 429 - Between−group variation Within−group variation (i.e., differences among group means) (i.e., deviations from group means) group 1 group 2 group 3 group 1 group 2 group 3 (a) (b) Figure 14.2: Graphical illustration of “between groups” variation (panel a) and “within groups” variation (panel b). On the left, the arrows show the di↵erences in the group means; on the right, the arrows highlight the variability within each group........................................................................................................ within-group sum of squares, in which we look to see how di↵erent each individual person is from their own group mean: ÿG ÿNk ` ˘2 SSw “ Yik ´ Ȳk k“1 i“1 where Ȳk is a group mean. In our example, Ȳk would be the average mood change experienced by those people given the k-th drug. So, instead of comparing individuals to the average of all people in the experiment, we’re only comparing them to those people in the the same group. As a consequence, you’d expect the value of SSw to be smaller than the total sum of squares, because it’s completely ignoring any group di↵erences – that is, the fact that the drugs (if they work) will have di↵erent e↵ects on people’s moods. Next, we can define a third notion of variation which captures only the di↵erences between groups. We do this by looking at the di↵erences between the group means Ȳk and grand mean Ȳ. In order to quantify the extent of this variation, what we do is calculate the between-group sum of squares: ÿ Nk G ÿ ` ˘2 SSb “ Ȳk ´ Ȳ k“1 i“1 ÿ G ` ˘2 “ Nk Ȳk ´ Ȳ k“1 It’s not too difficult to show that the total variation among people in the experiment SStot is actually the sum of the di↵erences between the groups SSb and the variation inside the groups SSw. That is: SSw ` SSb “ SStot Yay. Okay, so what have we found out? We’ve discovered that the total variability associated with the outcome variable (SStot ) can be mathematically carved up into the sum of “the variation due to the di↵erences in the sample means for the di↵erent groups” (SSb ) plus “all the rest of the variation” (SSw ). How does that help me find out whether the groups have di↵erent population means? Um. Wait. Hold on a second... now that I think about it, this is exactly what we were looking for. If the null hypothesis - 430 - is true, then you’d expect all the sample means to be pretty similar to each other, right? And that would imply that you’d expect SSb to be really small, or at least you’d expect it to be a lot smaller than the “the variation associated with everything else”, SSw. Hm. I detect a hypothesis test coming on... 14.2.3 From sums of squares to the F -test As we saw in the last section, the qualitative idea behind ANOVA is to compare the two sums of squares values SSb and SSw to each other: if the between-group variation is SSb is large relative to the within-group variation SSw then we have reason to suspect that the population means for the di↵erent groups aren’t identical to each other. In order to convert this into a workable hypothesis test, there’s a little bit of “fiddling around” needed. What I’ll do is first show you what we do to calculate our test statistic – which is called an F ratio – and then try to give you a feel for why we do it this way. In order to convert our SS values into an F -ratio, the first thing we need to calculate is the degrees of freedom associated with the SSb and SSw values. As usual, the degrees of freedom corresponds to the number of unique “data points” that contribute to a particular calculation, minus the number of “constraints” that they need to satisfy. For the within-groups variability, what we’re calculating is the variation of the individual observations (N data points) around the group means (G constraints). In contrast, for the between groups variability, we’re interested in the variation of the group means (G data points) around the grand mean (1 constraint). Therefore, the degrees of freedom here are: dfb “ G´1 dfw “ N ´G Okay, that seems simple enough. What we do next is convert our summed squares value into a “mean squares” value, which we do by dividing by the degrees of freedom: SSb MSb “ dfb SSw MSw “ dfw Finally, we calculate the F -ratio by dividing the between-groups MS by the within-groups MS: MSb F “ MSw At a very general level, the intuition behind the F statistic is straightforward: bigger values of F means that the between-groups variation is large, relative to the within-groups variation. As a consequence, the larger the value of F , the more evidence we have against the null hypothesis. But how large does F have to be in order to actually reject H0 ? In order to understand this, you need a slightly deeper understanding of what ANOVA is and what the mean squares values actually are. The next section discusses that in a bit of detail, but for readers that aren’t interested in the details of what the test is actually measuring, I’ll cut to the chase. In order to complete our hypothesis test, we need to know the sampling distribution for F if the null hypothesis is true. Not surprisingly, the sampling distribution for the F statistic under the null hypothesis is an F distribution. If you recall back to our discussion of the F distribution in Chapter 9, the F distribution has two parameters, corresponding to the two degrees of freedom involved: the first one df1 is the between groups degrees of freedom dfb , and the second one df2 is the within groups degrees of freedom dfw. A summary of all the key quantities involved in a one-way ANOVA, including the formulas showing - 431 - Table 14.1: All of the key quantities involved in an ANOVA, organised into a “standard” ANOVA table. The formulas for all quantities (except the p-value, which has a very ugly formula and would be nightmarishly hard to calculate without a computer) are shown. df sum of squares mean squares F -statistic p-value ÿ G SSb MSb between groups dfb “ G ´ 1 SSb “ Nk pȲk ´ Ȳ q2 MSb “ F “ [complicated] k“1 dfb MSw G N ÿ ÿk SSw within groups dfw “ N ´ G SSw “ pYik ´ Ȳk q2 MSw “ - - k“1 i“1 dfw............................................................................................................... how they are calculated, is shown in Table 14.1. 14.2.4 The model for the data and the meaning of F At a fundamental level, ANOVA is a competition between two di↵erent statistical models, H0 and H1. When I described the null and alternative hypotheses at the start of the section, I was a little imprecise about what these models actually are. I’ll remedy that now, though you probably won’t like me for doing so. If you recall, our null hypothesis was that all of the group means are identical to one another. If so, then a natural way to think about the outcome variable Yik is to describe individual scores in terms of a single population mean µ, plus the deviation from that population mean. This deviation is usually denoted ✏ik and is traditionally called the error or residual associated with that observation. Be careful though: just like we saw with the word “significant”, the word “error” has a technical meaning in statistics that isn’t quite the same as its everyday English definition. In everyday language, “error” implies a mistake of some kind; in statistics, it doesn’t (or at least, not necessarily). With that in mind, the word “residual” is a better term than the word “error”. In statistics, both words mean “leftover variability”: that is, “stu↵” that the model can’t explain. In any case, here’s what the null hypothesis looks like when we write it as a statistical model: Yik “ µ ` ✏ik where we make the assumption (discussed later) that the residual values ✏ik are normally distributed, with mean 0 and a standard deviation that is the same for all groups. To use the notation that we introduced in Chapter 9 we would write this assumption like this: 2 ✏ik „ Normalp0, q What about the alternative hypothesis, H1 ? The only di↵erence between the null hypothesis and the alternative hypothesis is that we allow each group to have a di↵erent population mean. So, if we let µk denote the population mean for the k-th group in our experiment, then the statistical model corresponding to H1 is: Yik “ µk ` ✏ik where, once again, we assume that the error terms are normally distributed with mean 0 and standard deviation. That is, the alternative hypothesis also assumes that ✏ „ Normalp0, 2 q Okay, now that we’ve described the statistical models underpinning H0 and H1 in more detail, it’s now pretty straightforward to say what the mean square values are measuring, and what this means for the interpretation of F. I won’t bore you with the proof of this, but it turns out that the within-groups - 432 - mean square, MSw , can be viewed as an estimator (in the technical sense: Chapter 10) of the error variance 2. The between-groups mean square MSb is also an estimator; but what it estimates is the error variance plus a quantity that depends on the true di↵erences among the group means. If we call this quantity Q, then we can see that the F -statistic is basically2 Q̂ ` ˆ 2 F “ ˆ2 where the true value Q “ 0 if the null hypothesis is true, and Q ° 0 if the alternative hypothesis is true (e.g. Hays, 1994, ch. 10). Therefore, at a bare minimum the F value must be larger than 1 to have any chance of rejecting the null hypothesis. Note that this doesn’t mean that it’s impossible to get an F -value less than 1. What it means is that, if the null hypothesis is true the sampling distribution of the F ratio has a mean of 1,3 and so we need to see F -values larger than 1 in order to safely reject the null. To be a bit more precise about the sampling distribution, notice that if the null hypothesis is true, both MSb and MSw are estimators of the variance of the residuals ✏ik. If those residuals are normally distributed, then you might suspect that the estimate of the variance of ✏ik is chi-square distributed... because (as discussed in Section 9.6) that’s what a chi-square distribution is: it’s what you get when you square a bunch of normally-distributed things and add them up. And since the F distribution is (again, by definition) what you get when you take the ratio between two things that are 2 distributed... we have our sampling distribution. Obviously, I’m glossing over a whole lot of stu↵ when I say this, but in broad terms, this really is where our sampling distribution comes from. 14.2.5 A worked example The previous discussion was fairly abstract, and a little on the technical side, so I think that at this point it might be useful to see a worked example. For that, let’s go back to the clinical trial data that I introduced at the start of the chapter. The descriptive statistics that we calculated at the beginning tell us our group means: an average mood gain of 0.45 for the placebo, 0.72 for Anxifree, and 1.48 for Joyzepam. With that in mind, let’s party like it’s 1899 4 and start doing some pencil and paper calculations. I’ll only do this for the first 5 observations, because it’s not bloody 1899 and I’m very lazy. Let’s start by calculating SSw , the within-group sums of squares. First, let’s draw up a nice table to help us with our calculations... group outcome k Yik placebo 0.5 placebo 0.3 placebo 0.1 anxifree 0.6 anxifree 0.4 At this stage, the only thing I’ve included in the table is the raw data itself: that is, the grouping variable (i.e., drug) and outcome variable (i.e. mood.gain) for each person. Note that the outcome variable here corresponds to the Yik value in our equation previously. The next step in the calculation is to write down, for each person in the study, the corresponding group mean; that is, Ȳk. This is slightly repetitive, but not particularly difficult since we already calculated those group means when doing our descriptive 2 In a later versions I’m intending to expand on this. But because I’m writing in a rush, and am already over my deadlines, I’ll just briefly note that if you read ahead to Chapter 16 and look at how the “treatment e↵ect” at level k of a factor is defined in terms of the ↵k values (see Section 16.2), it turns out that Q refers to a weighted mean of the squared ∞ treatment e↵ects, Q “ p G k“1 Nk ↵k q{pG ´ 1q. 2 3 Or, if we want to be sticklers for accuracy, 1 ` 2 df2 ´2. 4 Or, to be precise, party like “it’s 1899 and we’ve got no friends and nothing better to do with our time than do some calculations that wouldn’t have made any sense in 1899 because ANOVA didn’t exist until about the 1920s”. - 433 - statistics: group outcome group mean k Yik Ȳk placebo 0.5 0.45 placebo 0.3 0.45 placebo 0.1 0.45 anxifree 0.6 0.72 anxifree 0.4 0.72 Now that we’ve written those down, we need to calculate – again for every person – the deviation from the corresponding group mean. That is, we want to subtract Yik ´ Ȳk. After we’ve done that, we need to square everything. When we do that, here’s what we get:. group outcome group mean dev. from group mean squared deviation k Yik Ȳk Yik ´ Ȳk pYik ´ Ȳk q2 placebo 0.5 0.45 0.05 0.0025 placebo 0.3 0.45 -0.15 0.0225 placebo 0.1 0.45 -0.35 0.1225 anxifree 0.6 0.72 -0.12 0.0136 anxifree 0.4 0.72 -0.32 0.1003 The last step is equally straightforward. In order to calculate the within-group sum of squares, we just add up the squared deviations across all observations: SSw “ 0.0025 ` 0.0225 ` 0.1225 ` 0.0136 ` 0.1003 “ 0.2614 Of course, if we actually wanted to get the right answer, we’d need to do this for all 18 observations in the data set, not just the first five. We could continue with the pencil and paper calculations if we wanted to, but it’s pretty tedious. Alternatively, it’s not too hard to get R to do it. Here’s how: > outcome group gp.means gp.means dev.from.gp.means squared.devs Y print(Y, digits = 2) group outcome gp.means dev.from.gp.means squared.devs 1 placebo 0.5 0.45 0.050 0.0025 2 placebo 0.3 0.45 -0.150 0.0225 - 434 - 3 placebo 0.1 0.45 -0.350 0.1225 4 anxifree 0.6 0.72 -0.117 0.0136 5 anxifree 0.4 0.72 -0.317 0.1003 BLAH BLAH BLAH 16 joyzepam 1.8 1.48 0.317 0.1003 17 joyzepam 1.3 1.48 -0.183 0.0336 18 joyzepam 1.4 1.48 -0.083 0.0069 If you compare this output to the contents of the table I’ve been constructing by hand, you can see that R has done exactly the same calculations that I was doing, and much faster too. So, if we want to finish the calculations of the within-group sum of squares in R, we just ask for the sum() of the squared.devs variable: > SSw print( SSw ) 1.39 Obviously, this isn’t the same as what I calculated, because R used all 18 observations. But if I’d typed sum( squared.devs[1:5] ) instead, it would have given the same answer that I got earlier. Okay. Now that we’ve calculated the within groups variation, SSw , it’s time to turn our attention to the between-group sum of squares, SSb. The calculations for this case are very similar. The main di↵erence is that, instead of calculating the di↵erences between an observation Yik and a group mean Ȳk for all of the observations, we calculate the di↵erences between the group means Ȳk and the grand mean Ȳ (in this case 0.88) for all of the groups... group group mean grand mean deviation squared deviations k Ȳk Ȳ Ȳk ´ Ȳ pȲk ´ Ȳ q2 placebo 0.45 0.88 -0.43 0.18 anxifree 0.72 0.88 -0.16 0.03 joyzepam 1.48 0.88 0.60 0.36 However, for the between group calculations we need to multiply each of these squared deviations by Nk , the number of observations in the group. We do this because every observation in the group (all Nk of them) is associated with a between group di↵erence. So if there are six people in the placebo group, and the placebo group mean di↵ers from the grand mean by 0.19, then the total between group variation associated with these six people is 6 ˆ 0.16 “ 1.14. So we have to extend our little table of calculations... group... squared deviations sample size weighted squared dev k... pȲk ´ Ȳ q2 Nk Nk pȲk ´ Ȳ q2 placebo... 0.18 6 1.11 anxifree... 0.03 6 0.16 joyzepam... 0.36 6 2.18 And so now our between group sum of squares is obtained by summing these “weighted squared devia- tions” over all three groups in the study: SSb “ 1.11 ` 0.16 ` 2.18 “ 3.45 As you can see, the between group calculations are a lot shorter, so you probably wouldn’t usually want to bother using R as your calculator. However, if you did decide to do so, here’s one way you could do it: - 435 - > gp.means grand.mean dev.from.grand.mean squared.devs gp.sizes wt.squared.devs Y print(Y, digits = 2) gp.means grand.mean dev.from.grand.mean squared.devs gp.sizes wt.squared.devs placebo 0.45 0.88 -0.43 0.188 6 1.13 anxifree 0.72 0.88 -0.17 0.028 6 0.17 joyzepam 1.48 0.88 0.60 0.360 6 2.16 Clearly, these are basically the same numbers that we got before. There are a few tiny di↵erences, but that’s only because the hand-calculated versions have some small errors caused by the fact that I rounded all my numbers to 2 decimal places at each step in the calculations, whereas R only does it at the end (obviously, Rs version is more accurate). Anyway, here’s the R command showing the final step: > SSb print( SSb ) 3.453333 which is (ignoring the slight di↵erences due to rounding error) the same answer that I got when doing things by hand. Now that we’ve calculated our sums of squares values, SSb and SSw , the rest of the ANOVA is pretty painless. The next step is to calculate the degrees of freedom. Since we have G “ 3 groups and N “ 18 observations in total, our degrees of freedom can be calculated by simple subtraction: dfb “ G´1 “ 2 dfw “ N ´G “ 15 Next, since we’ve now calculated the values for the sums of squares and the degrees of freedom, for both the within-groups variability and the between-groups variability, we can obtain the mean square values by dividing one by the other: SSb 3.45 MSb “ “ “ 1.73 dfb 2 SSw 1.39 MSw “ “ “ 0.09 dfw 15 We’re almost done. The mean square values can be used to calculate the F -value, which is the test statistic that we’re interested in. We do this by dividing the between-groups MS value by the and within-groups MS value. MSb 1.73 F “ “ “ 18.6 MSw 0.09 Woohooo! This is terribly exciting, yes? Now that we have our test statistic, the last step is to find out whether the test itself gives us a significant result. As discussed in Chapter 11, what we really ought to do - 436 - is choose an ↵ level (i.e., acceptable Type I error rate) ahead of time, construct our rejection region, etc etc. But in practice it’s just easier to directly calculate the p-value. Back in the “old days”, what we’d do is open up a statistics textbook or something and flick to the back section which would actually have a huge lookup table... that’s how we’d “compute” our p-value, because it’s too much e↵ort to do it any other way. However, since we have access to R, I’ll use the pf() function to do it instead. Now, remember that I explained earlier that the F -test is always one sided? And that we only reject the null hypothesis for very large F -values? That means we’re only interested in the upper tail of the F -distribution. The command that you’d use here would be this... > pf( 18.6, df1 = 2, df2 = 15, lower.tail = FALSE) 8.6727e-05 Therefore, our p-value comes to 0.0000867, or 8.67 ˆ 10´5 in scientific notation. So, unless we’re being extremely conservative about our Type I error rate, we’re pretty much guaranteed to reject the null hypothesis. At this point, we’re basically done. Having completed our calculations, it’s traditional to organise all these numbers into an ANOVA table like the one in Table 14.1. For our clinical trial data, the ANOVA table would look like this: df sum of squares mean squares F -statistic p-value between groups 2 3.45 1.73 18.6 8.67 ˆ 10´5 within groups 15 1.39 0.09 - - These days, you’ll probably never have much reason to want to construct one of these tables yourself, but you will find that almost all statistical software (R included) tends to organise the output of an ANOVA into a table like this, so it’s a good idea to get used to reading them. However, although the software will output a full ANOVA table, there’s almost never a good reason to include the whole table in your write up. A pretty standard way of reporting this result would be to write something like this: One-way ANOVA showed a significant e↵ect of drug on mood gain (F p2, 15q “ 18.6, p †.001). Sigh. So much work for one short sentence. 14.3 Running an ANOVA in R I’m pretty sure I know what you’re thinking after reading the last section, especially if you followed my advice and tried typing all the commands in yourself.... doing the ANOVA calculations yourself sucks. There’s quite a lot of calculations that we needed to do along the way, and it would be tedious to have to do this over and over again every time you wanted to do an ANOVA. One possible solution to the problem would be to take all these calculations and turn them into some R functions yourself. You’d still have to do a lot of typing, but at least you’d only have to do it the one time: once you’ve created the functions, you can reuse them over and over again. However, writing your own functions is a lot of work, so this is kind of a last resort. Besides, it’s much better if someone else does all the work for you... - 437 - 14.3.1 Using the function to specify your ANOVA To make life easier for you, R provides a function called aov(), which – obviously – is an acronym of “Analysis Of Variance”.5 If you type ?aov and have a look at the help documentation, you’ll see that there are several arguments to the aov() function, but the only two that we’re interested in are formula and data. As we’ve seen in a few places previously, the formula argument is what you use to specify the outcome variable and the grouping variable, and the data argument is what you use to specify the data frame that stores these variables. In other words, to do the same ANOVA that I laboriously calculated in the previous section, I’d use a command like this: > aov( formula = mood.gain ~ drug, data = clin.trial ) Actually, that’s not quite the whole story, as you’ll see as soon as you look at the output from this command, which I’ve hidden for the moment in order to avoid confusing you. Before we go into specifics, I should point out that either of these commands will do the same thing: > aov( clin.trial$mood.gain ~ clin.trial$drug ) > aov( mood.gain ~ drug, clin.trial ) In the first command, I didn’t specify a data set, and instead relied on the $ operator to tell R how to find the variables. In the second command, I dropped the argument names, which is okay in this case because formula is the first argument to the aov() function, and data is the second one. Regardless of how I specify the ANOVA, I can assign the output of the aov() function to a variable, like this for example: > my.anova class( my.anova ) "aov" "lm"... we discover that my.anova actually has two classes! The first class tells us that it’s an aov (analysis of variance) object, but the second tells us that it’s also an lm (linear model) object. Later on, we’ll see that this reflects a pretty deep statistical relationship between ANOVA and regression (Chapter 15) and it means that any function that exists in R for dealing with regressions can also be applied to aov objects, which is neat; but I’m getting ahead of myself. For now, I want to note that what we’ve created is an aov object, and to also make the point that aov objects are actually rather complicated beasts. I won’t be trying to explain everything about them, since it’s way beyond the scope of an introductory statistics subject, but to give you a tiny hint of some of the stu↵ that R stores inside an aov object, let’s ask it to print out the names() of all the stored quantities... 5 Actually, it also provides a function called anova(), but that works a bit di↵erently, so let’s just ignore it for now. - 438 - > names( my.anova ) "coefficients" "residuals" "effects" "rank" "fitted.values" "assign" "qr" "df.residual" "contrasts" "xlevels" "call" "terms" "model" As we go through the rest of the book, I hope that a few of these will become a little more obvious to you, but right now that’s going to look pretty damned opaque. That’s okay. You don’t need to know any of the details about it right now, and most of it you don’t need at all... what you do need to understand is that the aov() function does a lot of calculations for you, not just the basic ones that I outlined in the previous sections. What this means is that it’s generally a good idea to create a variable like my.anova that stores the output of the aov() function... because later on, you can use my.anova as an input to lots of other functions: those other functions can pull out bits and pieces from the aov object, and calculate various other things that you might need. Right then. The simplest thing you can do with an aov object is to print() it out. When we do that, it shows us a few of the key quantities of interest: > print( my.anova ) Call: aov(formula = mood.gain ~ drug, data = clin.trial) Terms: drug Residuals Sum of Squares 3.4533 1.3917 Deg. of Freedom 2 15 Residual standard error: 0.30459 Estimated effects may be unbalanced Specificially, it prints out a reminder of the command that you used when you called aov() in the first place, shows you the sums of squares values, the degrees of freedom, and a couple of other quantities that we’re not really interested in right now. Notice, however, that R doesn’t use the names “between-group” and “within-group”. Instead, it tries to assign more meaningful names: in our particular example, the between groups variance corresponds to the e↵ect that the drug has on the outcome variable; and the within groups variance is corresponds to the “leftover” variability, so it calls that the residuals. If we compare these numbers to the numbers that I calculated by hand in Section 14.2.5, you can see that they’re identical... the between groups sums of squares is SSb “ 3.45, the within groups sums of squares is SSw “ 1.39, and the degrees of freedom are 2 and 15 repectively. 14.3.3 Running the hypothesis tests for the ANOVA Okay, so we’ve verified that my.anova seems to be storing a bunch of the numbers that we’re looking for, but the print() function didn’t quite give us the output that we really wanted. Where’s the F -value? The p-value? These are the most important numbers in our hypothesis test, but the print() function doesn’t provide them. To get those numbers, we need to use a di↵erent function. Instead of asking R to print() out the aov object, we should have asked for a summary() of it.6 When we do that... > summary( my.anova ) Df Sum Sq Mean Sq F value Pr(>F) 6 It’s worth noting that you can get the same result by using the command anova( my.anova ). - 439 - drug 2 3.45 1.727 18.6 8.6e-05 *** Residuals 15 1.39 0.093 --- Signif. codes: 0 *** 0.001 ** 0.01 * 0.05. 0.1 1... we get all of the key numbers that we calculated earlier. We get the sums of squares, the degrees of freedom, the mean squares, the F -statistic, and the p-value itself. These are all identical to the numbers that we calculated ourselves when doing it the long and tedious way, and it’s even organised into the same kind of ANOVA table that I showed in Table 14.1, and then filled out by hand in Section 14.2.5. The only things that are even slightly di↵erent is that some of the row and column names are a bit di↵erent. 14.4 E↵ect size There’s a few di↵erent ways you could measure the e↵ect size in an ANOVA, but the most commonly used measures are ⌘ 2 (eta squared) and partial ⌘ 2. For a one way analysis of variance they’re identical to each other, so for the moment I’ll just explain ⌘ 2. The definition of ⌘ 2 is actually really simple: SSb ⌘2 “ SStot That’s all it is. So when I look at the ANOVA table above, I see that SSb “ 3.45 and SStot “ 3.45`1.39 “ 4.84. Thus we get an ⌘ 2 value of 3.45 ⌘2 “ “ 0.71 4.84 The interpretation of ⌘ 2 is equally straightforward: it refers to the proportion of the variability in the outcome variable (mood.gain) that can be explained in terms of the predictor (drug). A value of ⌘ 2 “ 0 means that there is no relationship at all between the two, whereas a value of ⌘ 2 “ 1 means that the relationship is perfect. Better yet, the ⌘ 2 value is very closely related to a squared correlation (i.e., r2 ). So, if you’re trying to figure out whether a particular value of ⌘ 2 is big or small, it’s sometimes useful to remember that c SSb ⌘“ SStot can be interpreted as if it referred to the magnitude ? of a Pearson correlation. So in our drugs example, the ⌘ 2 value of.71 corresponds to an ⌘ value of.71 “.84. If we think about this as being equivalent to a correlation of about.84, we’d conclude that the relationship between drug and mood.gain is strong. The core packages in R don’t include any functions for calculating ⌘ 2. However, it’s pretty straight- forward to calculate it directly from the numbers in the ANOVA table. In fact, since I’ve already got the SSw and SSb variables lying around from my earlier calculations, I can do this: > SStot eta.squared print( eta.squared ) 0.71276 However, since it can be tedious to do this the long way (especially when we start running more compli- cated ANOVAs, such as those in Chapter 16) I’ve included an etaSquared() function in the lsr package which will do it for you. For now, the only argument you need to care about is x, which should be the aov object corresponding to your ANOVA. When we do this, what we get as output is this: - 440 - > etaSquared( x = my.anova ) eta.sq eta.sq.part drug 0.7127623 0.7127623 The output here shows two di↵erent numbers. The first one corresponds to the ⌘ 2 statistic, precisely as described above. The second one refers to “partial ⌘ 2 ”, which is a somewhat di↵erent measure of e↵ect size that I’ll describe later. For the simple ANOVA that we’ve just run, they’re the same number. But this won’t always be true once we start running more complicated ANOVAs.7 14.5 Multiple comparisons and post hoc tests Any time you run an ANOVA with more than two groups, and you end up with a significant e↵ect, the first thing you’ll probably want to ask is which groups are actually di↵erent from one another. In our drugs example, our null hypothesis was that all three drugs (placebo, Anxifree and Joyzepam) have the exact same e↵ect on mood. But if you think about it, the null hypothesis is actually claiming three di↵erent things all at once here. Specifically, it claims that: Your competitor’s drug (Anxifree) is no better than a placebo (i.e., µA “ µP ) Your drug (Joyzepam) is no better than a placebo (i.e., µJ “ µP ) Anxifree and Joyzepam are equally e↵ective (i.e., µJ “ µA ) If any one of those three claims is false, then the null hypothesis is also false. So, now that we’ve rejected our null hypothesis, we’re thinking that at least one of those things isn’t true. But which ones? All three of these propositions are of interest: you certainly want to know if your new drug Joyzepam is better than a placebo, and it would be nice to know how well it stacks up against an existing commercial alternative (i.e., Anxifree). It would even be useful to check the performance of Anxifree against the placebo: even if Anxifree has already been extensively tested against placebos by other researchers, it can still be very useful to check that your study is producing similar results to earlier work. When we characterise the null hypothesis in terms of these three distinct propositions, it becomes clear that there are eight possible “states of the world” that we need to distinguish between: possibility: is µP “ µA ? is µP “ µJ ? is µA “ µJ ? which hypothesis? 1 X X X null 2 X X alternative 3 X X alternative 4 X alternative 5 X X alternative 6 X alternative 7 X alternative 8 alternative 7 A potentially important footnote – I wrote the etaSquared() function for the lsr package as a teaching exercise, but like all the other functions in the lsr package it hasn’t been exhaustively tested. As of this writing – lsr package version 0.5 – there is at least one known bug in the code. In some cases at least, it doesn’t work (and can give very silly answers) when you set the weights on the observations to something other than uniform. That doesn’t matter at all for this book, since those kinds of analyses are well beyond the scope, but I haven’t had a chance to revisit the package in a long time; it would probably be wise to be very cautious with the use of this function in any context other than very simple introductory analyses. Thanks to Emil Kirkegaard for finding the bug! (Oh, and while I’m here, there’s an interesting blog post by Daniel Lakens suggesting that eta-squared itself is perhaps not the best measure of e↵ect size in real world data analysis: http://daniellakens.blogspot.com.au/2015/06/why-you-should-use-omega-squared.html - 441 - By rejecting the null hypothesis, we’ve decided that we don’t believe that #1 is the true state of the world. The next question to ask is, which of the other seven possibilities do we think is right? When faced with this situation, its usually helps to look at the data. For instance, if we look at the plots in Figure 14.1, it’s tempting to conclude that Joyzepam is better than the placebo and better than Anxifree, but there’s no real di↵erence between Anxifree and the placebo. However, if we want to get a clearer answer about this, it might help to run some tests. 14.5.1 Running “pairwise” t-tests How might we go about solving our problem? Given that we’ve got three separate pairs of means (placebo versus Anxifree, placebo versus Joyzepam, and Anxifree versus Joyzepam) to compare, what we could do is run three separate t-tests and see what happens. There’s a couple of ways that we could do this. One method would be to construct new variables corresponding the groups you want to compare (e.g., anxifree, placebo and joyzepam), and then run a t-test on these new variables: > t.test( anxifree, placebo, var.equal = TRUE ) # Student t-test or, you could use the subset argument in the t.test() function to select only those observations corre- sponding to one of the two groups we’re interested in: > t.test( formula = mood.gain ~ drug, + data = clin.trial, + subset = drug %in% c("placebo","anxifree"), + var.equal = TRUE + ) See Chapter 7 if you’ve forgotten how the %in% operator works. Regardless of which version we do, R will print out the results of the t-test, though I haven’t included that output here. If we go on to do this for all possible pairs of variables, we can look to see which (if any) pairs of groups are significantly di↵erent to each other. This “lots of t-tests idea” isn’t a bad strategy, though as we’ll see later on there are some problems with it. However, for the moment our bigger problem is that it’s a pain to have to type in such a long command over and over again: for instance, if your experiment has 10 groups, then you have to run 45 t-tests. That’s way too much typing. To help keep the typing to a minimum, R provides a function called pairwise.t.test() that automat- ically runs all of the t-tests for you. There are three arguments that you need to specify, the outcome variable x, the group variable g, and the p.adjust.method argument, which “adjusts” the p-value in one way or another. I’ll explain p-value adjustment in a moment, but for now we can just set p.adjust.method = "none" since we’re not doing any adjustments. For our example, here’s what we do: > pairwise.t.test( x = clin.trial$mood.gain, # outcome variable + g = clin.trial$drug, # grouping variable + p.adjust.method = "none" # which correction to use? + ) Pairwise comparisons using t tests with pooled SD data: clin.trial$mood.gain and clin.trial$drug placebo anxifree anxifree 0.15021 - joyzepam 3e-05 0.00056 P value adjustment method: none - 442 - One thing that bugs me slightly about the pairwise.t.test() function is that you can’t just give it an aov object, and have it produce this output. After all, I went to all that trouble earlier of getting R to create the my.anova variable and – as we saw in Section 14.3.2 – R has actually stored enough information inside it that I should just be able to get it to run all the pairwise tests using my.anova as an input. To that end, I’ve included a posthocPairwiseT() function in the lsr package that lets you do this. The idea behind this function is that you can just input the aov object itself,8 and then get the pairwise tests as an output. As of the current writing, posthocPairwiseT() is actually just a simple way of calling pairwise.t.test() function, but you should be aware that I intend to make some changes to it later on. Here’s an example: > posthocPairwiseT( x = my.anova, p.adjust.method = "none" ) Pairwise comparisons using t tests with pooled SD data: mood.gain and drug placebo anxifree anxifree 0.15021 - joyzepam 3e-05 0.00056 P value adjustment method: none In later versions, I plan to add more functionality (e.g., adjusted confidence intervals), but for now I think it’s at least kind of useful. To see why, let’s suppose you’ve run your ANOVA and stored the results in my.anova, and you’re happy using the Holm correction (the default method in pairwise.t.test(), which I’ll explain this in a moment). In that case, all you have to do is type this: > posthocPairwiseT( my.anova ) and R will output the test results. Much more convenient, I think. 14.5.2 Corrections for multiple testing In the previous section I hinted that there’s a problem with just running lots and lots of t-tests. The concern is that when running these analyses, what we’re doing is going on a “fishing expedition”: we’re running lots and lots of tests without much theoretical guidance, in the hope that some of them come up significant. This kind of theory-free search for group di↵erences is referred to as post hoc analysis (“post hoc” being Latin for “after this”).9 It’s okay to run post hoc analyses, but a lot of care is required. For instance, the analysis that I ran in the previous section is actually pretty dangerous: each individual t-test is designed to have a 5% Type I error rate (i.e., ↵ “.05), and I ran three of these tests. Imagine what would have happened if my ANOVA involved 10 di↵erent groups, and I had decided to run 45 “post hoc” t-tests to try to find out which ones were significantly di↵erent from each other, you’d expect 2 or 3 of them to come up significant by chance alone. As we saw in Chapter 11, the central organising principle behind null hypothesis testing is that we seek to control our Type I error rate, but now that I’m running lots of t-tests at once, in order to determine the source of my ANOVA results, my actual Type I error rate across this whole family of tests has gotten completely out of control. 8 I should point out that there are other functions in R for running multiple comparisons, and at least one of them works this way: the TukeyHSD() function takes an aov object as its input, and outputs Tukey’s “honestly significant di↵erence” tests. I talk about Tukey’s HSD in Chapter 16. 9 If you do have some theoretical basis for wanting to investigate some comparisons but not others, it’s a di↵erent story. In those circumstances you’re not really ru