Molecular Genetics - Reading PDF
Document Details
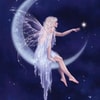
Uploaded by AppealingAmazonite
null
Tags
Summary
This document provides a discussion of molecular genetics, focusing on genome-wide association studies and their application to understand the genetic components of common adult diseases. It details the scientific rationale behind GWAS, discusses the results and discoveries, and touches upon limitations and future directions.
Full Transcript
Molecular Genetics - Reading 31 October 2023 13:28 Source Notes 10 Years of GWAS Discovery: Biology, Function, and Translation Introduction (Visscher et al., 2017) Genome-wide association studies (GWASs) have facilitated a remarkable range of discoveries in population and complex-trait genetics, the...
Molecular Genetics - Reading 31 October 2023 13:28 Source Notes 10 Years of GWAS Discovery: Biology, Function, and Translation Introduction (Visscher et al., 2017) Genome-wide association studies (GWASs) have facilitated a remarkable range of discoveries in population and complex-trait genetics, the biology of diseases, and translation toward new therapeutics. This review provides a background for GWASs, summarizes its scope and layout, and revisits the scientific rationale for GWASs. It then reviews general conclusions that can be drawn from GWAS discoveries across a wide range of traits and highlights morespecific results of discoveries and methods on the path from GWAS to biology. Finally, it discusses the limitations of current experimental designs and possible ways to overcome them, and provides a prediction on the future of GWASs for human traits. Background ○ Five years ago, a review of the first 5 years of GWAS discoveries was published, which sought to set the record straight on t he discoveries made by GWASs. ○ There is now much more acceptance of the experimental design because the empirical results have been robust and overwhelming. Scope and Framework ○ The scope of the review is novel discoveries on the genetics and resulting biology of common adult diseases (auto -immune, metabolic, and psychiatric disease in particular) and their risk factors and the wider implications of those discoveries. ○ The focus is on associations between complex traits and SNPs, but the review notes that there have been many reported associations between traits and copy-number variants (CNVs) and that there are known mechanisms by which CNVs can be associated with disease. ○ Results from other genome-wide surveys, including exome and whole-genome sequencing (WGS) studies, are not reviewed. GWAS Rationale and Scientific Basis GWASs are used to detect associations between genetic variants and traits in samples from populations. ○ The primary goal of GWASs is to better understand the biology of disease. ○ GWASs rely on linkage disequilibrium (LD), the correlation structure that exists among DNA variants in the current human geno me. ○ The statistical power to detect associations between DNA variants and a trait depends on the experimental sample size, the di stribution of effect sizes of (unknown) causal genetic variants, the frequency of those variants, and the LD between observed genotyped DNA variants and the unknown causal variants. ○ The potential of a GWAS to succeed for a particular trait or disease depends on: ▪ How many loci affecting the trait segregate in the population ▪ The joint distribution of effect size and allele frequency at those loci ▪ The experimental sample size ▪ The panel of genome-wide variants that are used in the GWAS ▪ How heterogeneous the trait or disease being studied is Common vs. Rare Variants ○ Most genetic variants that have been surveyed through GWASs are common in the population, in that they have a minor allele fr equency (MAF) typically larger than 1%. ○ Rare variants are defined to have MAF < 1%. ○ GWASs are not powerful enough to detect associations due to rare causal variants. ○ Statistical imputation of unobserved variants can recover some of the information lost because of imperfect LD between observ ed genotypes and unobserved causal variants. ○ WGS can identify associations due to rare variants, but only when the effect sizes of the polymorphisms (mutations) are very large. Power of Detection ○ The minimum sample size required for detecting an association depends on the genotype method (SNP array plus imputation or WG S), allele frequency, and effect size. ○ For rare variants with an effect size of 1 phenotypic standard deviation unit, a sample size of more than one million is requ ired. ○ For case-control studies of disease, the effects sizes of b ¼ 0.01, 0.1, and 1 phenotypic standard deviation correspond approximately to odds ratios of 1.02, 1.2, and 4, respectively, if we assume that both allele frequency and population prevalence are 0.01 or lower. Power can be increased for: ○ Highly ascertained cases ○ Enrichment of extreme cases ○ Family-based studies with multiple cases of a rare disease ○ Combining alleles of similar impact (e.g., via burden tests across a gene) Challenges: ○ Prior knowledge about function or frequency is required for determining which alleles in a gene should be included in the bur den count. General Results Complex Traits Are Highly Polygenic: ○ GWAS results have been reported for hundreds of complex traits. ○ Strong associations between genetic variants and complex traits have been identified, typically requiring large sample sizes. ○ Many loci contribute to genetic variation in almost all complex traits studied, with each individual having a unique combinat ion of alleles affecting the trait. ○ Larger sample sizes have led to the discovery of more associated genetic variants over the years. Pleiotropy Is Pervasive: ○ Pleiotropy means that a single gene affects two or more characters ○ Complex traits are associated with variants at hundreds to thousands of loci in the genome. ○ Evidence suggests widespread pleiotropy, where the same genetic variants can affect multiple traits and diseases. ○ The concept of "one gene, one function, one trait" is challenged, and it's important to consider interactions between differe nt genetic variants in understanding complex traits. New Analysis Methodology Underpinning New Discovery: ○ GWAS data have led to the development of new analysis methods for various purposes, including modelling population structure, detecting novel variants and gene loci, estimating genetic correlations, and inferring causality. ○ Improved algorithms for imputation of unobserved genotypes and human leukocyte antigen (HLA) genes have enhanced GWAS data an alysis. Common Variants Together Tag a Substantial Proportion of Additive Genetic Variance: ○ Single nucleotide polymorphism is a genomic variant at a single base position in the DNA ○ GWASs have helped estimate "SNP heritability," which quantifies how much of the total additive genetic variation is tagged by genotyped and imputed SNPs. ○ SNP heritability indicates that a significant portion of genetic variation is explained by common genotyped and imputed SNPs through linkage disequilibrium (LD). ○ The contribution of rare and low-frequency variants to genetic variation remains to be explicitly estimated. The Utility of GWAS-Derived Genetic Predictors: ○ Genetic predictors (polygenic risk scores) have been developed based on GWAS data, which can be used for predicting an indivi dual's risk of disease. ○ Polygenic predictions are more informative for separating groups based on risk than for individual risk prediction. ○ Genetic predictors can also be used to detect new trait associations and study genotype-environment interactions. The Public Availability of Data Has Enabled Novel Research and Discoveries: ○ Sharing of genetic data within the gene-mapping community has played a significant role in gene-mapping success. ○ Availability of GWAS summary statistics in the public domain has increased in recent years, enabling novel discoveries, SNP -based heritability estimation, quantification of pleiotropy, and more accurate prediction scores. ○ The UK Biobank is releasing genome-wide genotypes and rich phenotypic data for 500,000 people to the international research community. From GWAS to Biology PSYC0036 Genes and Behaviour Page 1 Challenges of interpreting GWAS findings: ○ GWASs do not yield a particular gene target or mechanism. ○ The sheer number of associated variants means that follow-up functional studies are not appropriate or achievable. ○ Efforts to understand the biological mechanisms have been thwarted by limitations in the capacity to perform large -scale evaluation of functional impact. The advent of sequence-based omics analyses: ○ Sequence-based omics analyses have allowed functional analyses of risk variants to be pursued on the same genome scale as GWASs. ○ Maps of regulatory annotations and connections in disease-relevant tissues have been crucial to interpretation of the non-coding variants that account for the majority of GWAS-identified risk alleles. Integrating data from GWASs and eQTL studies: ○ New analytical methods integrate data from GWASs and expression quantitative trait locus (eQTL) studies to identify associati ons between transcripts and complex traits. ○ These methods are useful for prioritizing genes from known GWAS loci for functional follow -up, detecting novel gene-trait associations, and inferring the directions of associations. ○ Analytical results indicate that only about one-third of the associated genes are the nearest genes, which is informative for the design of finemapping experiments. Translational advances: ○ One of the ultimate objectives of genetic research is to drive translational advances that enable more effective prevention a nd/or treatment of disease. ○ A growing number of examples highlight the diverse routes by which human genetics can inform translational medicine. Three Exemplars of GWAS success Types 2 Diabetes (T2D) Variant and Gene Discovery ▪ Over 100 common variant signals identified in GWAS. ▪ Most genetic variation influencing T2D appears to reside at common variant sites. ▪ Common risk alleles are replicated across major ethnic groups, but more ethnic-specific alleles are being identified. ▪ Some ethnic-specific alleles have a relatively large phenotypic impact. Gene-Gene and Gene-Environment Interactions ▪ Efforts to identify compelling evidence for gene-gene and gene-environment interactions have been largely unsuccessful. From GWAS to Biology ▪ Regulatory Information □ Variants strongly associated with T2D are preferentially located at active enhancers in pancreatic islets and to a lesser extent in fat, muscle, and liver. □ Tissue-specific genomic enrichment patterns align with physiological data indicating primary effects on insulin secretion. ▪ Advances in Understanding □ Cis-expression mapping has highlighted specific genes mediating T2D signals. □ Identification of causal transcripts at GWAS loci from known biology, involvement in related monogenic conditions, or data from animal models. Coding Variants ▪ Accumulation of data on coding variants highlighted instances where non-coding GWAS signals can be reassigned to causal coding variants. Translation ▪ Examples of How Genetics Informs Translational Medicine (1) Loss-of-function mutations in SLC30A8 are protective for T2D, leading to ZnT-8 antagonist development. (2) Genetic variants used as instruments to clarify the roles of vitamin D intake, early nutrition, circulating lipid levels, andchronic inflammation in T2D development. (3) Identification of genetic variants associated with individual variation in response to therapeutic agents refines understanding and leads to therapeutic optimization. (4) Integration of -omic measurements, clinical phenotypes, and GWAS data highlights molecules associated with T2D progression and provides tools for stratification and prognostication. Auto-immune Disease Variant and Gene Discovery ▪ GWASs conducted for major immune-mediated diseases in the last 5 years with large sample sizes. ▪ Hundreds of associated loci identified for immune-mediated diseases. ▪ Statistical approaches for cross-disease studies have been productive in identifying new genes and understanding disease relationships. ▪ Transethnic studies have shown substantial genetic overlap between ethnically remote populations. From GWAS to Biology ▪ GWAS results have contributed to deeper biological understanding of immune-mediated diseases. ▪ New loci have implicated genes related to methylation variation, bacteria-sensing, host microbiome, and NFKB pathway. ▪ Evidence of pleiotropy includes variants with different directions of association in different diseases. ▪ Genetics can predict potential toxicities for therapeutic targeting. Translation ▪ GWAS results have initiated medication repositioning. ▪ Medications targeting components of the IL-23 pathway (IL-12p40, IL-17, IL-23p19) are mainstay treatments for psoriasis, psoriatic arthritis, and are effective in AS and IBD. ▪ The association between PADI4 and RA led to the development of PAD inhibitors in RA. ▪ Drug-development programs target ERAP1 and ERAP2 for AS, psoriasis, IBD, Behcet disease, and Birdshot retinopathy. ▪ Bioinformatic analysis of GWAS results has identified potential new therapies for RA, such as CDK4 and CDK6 inhibitors. Schizophrenia Variant and Gene Discovery ▪ Over 100 risk loci discovered in the last 5 years. ▪ Enrichment of genes with de novo mutations in schizophrenia, autism, and intellectual disability. ▪ Identified loci relevant to major hypotheses of schizophrenia aetiology, including DRD2 and genes related to glutamatergic neurotransmission. ▪ Common variants contribute to risk in a highly polygenic manner. ▪ Evidence of pleiotropy with other psychiatric disorders. ▪ Genetic architecture may differ between psychiatric disorders, with higher rates of rare, de novo penetrant CNVs and single-nucleotide variants in autism. From GWAS to Biology ▪ Bioinformatic analyses have been crucial for functional follow-up in psychiatric disorders. ▪ Schizophrenia risk loci are over-represented in regulatory regions active in the brain. ▪ Enrichment in genes related to synaptic and neuronal pathways. ▪ Integration with eQTL datasets implicates synaptic genes and genes involved in neurodevelopment. ▪ 3D contact analysis supports interactions between risk variants and promoters in relevant genes. Fine-mapping ▪ Accomplished for the strongest association within the MHC region. ▪ Investigation of common structural haplotypes of complement factor 4 genes C4A and C4B correlated with schizophrenia risk. ▪ Decreased numbers of synapses suggested as a primary abnormality in schizophrenia. Translation ▪ No new molecular targets for schizophrenia identified since the first antipsychotic drugs. ▪ High-potency single-target drug development has been the focus. ▪ GWAS results indicate polygenicity and the potential for a multi-target approach. ▪ Gene-set enrichment of schizophrenia risk alleles suggests potential repurposing opportunities. ▪ Single-target medications may be suitable for specific genetic subgroups, but genetic subtypes are not yet part of the clinical trial paradigm. Discussion PSYC0036 Genes and Behaviour Page 2 Discussion The present ○ GWASs have led to diverse discoveries in human genetics. ○ Most traits and diseases studied exhibit a large mutational target in the genome. ○ Widespread pleiotropy implies that many genetic variants affect multiple traits. ○ The proportion of all segregating genetic variants that are 'functional' remains elusive. ○ Examples of routes from GWAS to biology and translation were provided. ○ Rapid translation of genetic findings toward clinical application has been observed. Sample Size and Number of Risk Loci ▪ The relationship between sample size and the number of risk loci detected varies between traits. ▪ No trait has shown evidence of a plateau in the number of risk loci discovered. ▪ Future discoveries may saturate associated pathways first, followed by genes and variants. ▪ Multiple risk variants are expected to be detected within known loci as sample sizes increase. GWAS and Molecular Traits ▪ GWASs have been applied to molecular traits such as gene expression, DNA methylation, and metabolites. ▪ Molecular phenotypes, like complex traits, result from a combination of genetic factors and environmental exposures. ▪ Genetic loci can be mapped by GWASs, challenging the discovery of causal pathways. Transition to Whole Genome Sequencing (WGS) ▪ The price difference between SNP arrays and WGS remains substantial. ▪ Hundreds of thousands of genomes are being sequenced as part of major initiatives. ▪ Direct comparisons between sequencing and array studies will be possible in the next 5 years. Detection of Structural Variants ▪ Precision of detection of structural variants is currently less than that of SNP detection. ▪ New technologies enabling long-range haplotyping can improve the detection of structural variants. ▪ Genome-wide technologies for structural variants are needed to advance this area. Fine-Mapping ▪ Fine-mapping aims to identify causal variants responsible for GWAS signals. ▪ Statistical fine-mapping power will improve with large sample sizes and WGS data. ▪ Additional information, such as prior knowledge of variant function, could aid in reducing the set of statistical candidates. Population Diversity ▪ Most GWASs have been conducted on individuals of European descent. ▪ Common variants are expected to be shared across ethnicities. ▪ Utilizing mixed and admixed ethnicity can aid in fine-mapping causal variants. ▪ New methods are emerging to deal with diverse population data. The future ○ Keeping the current GWAS experimental strategy of SNP arrays and imputation would lead to the following: (1) Discovery of more variants and genes associated with traits. (2) Better understanding and accounting for genetic variation. (3) Improved genetic predictors. (4) Enhanced ability to evaluate disease heterogeneity and genetically informed diagnoses. ○ In fields like psychiatry, GWASs have contributed quantitative data for re-evaluating relationships among distinct disorders. ○ Challenges in the future will involve identifying loci with smaller effect sizes, especially for rare variants. ○ Upscaling technology and experimental perturbations, such as genome-wide CRISPR, may address the challenges of small effect sizes. ○ GWAS by whole-genome sequencing (WGS) is expected to gradually replace SNP arrays, particularly for quantitative traits and common diseases. ○ Phenotypically informative sample sizes genotyped on SNP chips remain a powerful strategy for maximizing discovery. ○ The emphasis in research should shift from gene discovery to translation into biological understanding and patient -focused outcomes. Conclusion ▪ Over the past decade, GWASs have led to a wide range of discoveries in human genetics. ▪ They have detected associations between common DNA variants and human disease, enhancing the understanding of the genetic architecture of complex traits. ▪ GWASs have contributed to the discovery of variants, genes, and biological pathways related to specific diseases and disorders. ▪ Disease epidemiology and candidate therapeutics have benefited from these discoveries. ▪ The future holds promise with whole-genome surveys of genetic variation and detailed phenotypic data, likely leading to fundamental discoveries in human genetics. ▪ Genomic personalized or precision medicine is expected to become widespread in the next 10 years, influencing risk stratification, new treatments, and preventive strategies based on GWAS results. The future of genomics for developmentalists (Plomin & Michael, 2013) Introduction Why this paper was written ○ For behavioral researchers and clinicians interested in development, rather than for experts in genomics. ○ To discuss a few issues that will shape the future of developmental research and, eventually, translate to the clinic. The future of genomics ○ The breathtaking momentum of current advances will sweep the field into the next decade. ○ Some important advances will be completely new and unanticipated. What Is Inherited Is DNA Sequence Variation: Everything Else Is a Phenotype What is inherited ○ Only DNA sequence variation is inherited. ○ All else is a phenotype, which is the result of interactions between DNA sequence variation and the environment. Epigenetic inheritance ○ Epigenetic inheritance is often discussed as an exception to the rule that only DNA sequence variation is inherited. ○ However, the extent of transmitted methylation is limited and individual differences in DNA methylation appear to be largely environmental in origin. ○ Epigenetics may be an especially good biomarker of early developmental adversity. Direction of effects ○ Correlations between DNA sequence variation and behavior are ultimately causal from genes to behavior. ○ Other correlations between behavior and biology, including all the -omics and the brain, are questionable concerning the direction of effects. Predictive ability ○ The ability of DNA sequence variation to predict problems long before they appear, even prenatally, opens up the possibility of interventions to prevent disorders. Personalized genomics ○ The ultimate hope is for personalized genomics, individualized gene-based diagnoses, and treatment programs The Future Includes Quantitative Genetics Quantitative genetics in the future ○ Quantitative genetics is genomic, meaning that it appraises the net effect on traits of genes throughout the genome regardless of the types of genes, the number of genes, the frequency of their alleles, the size of their effects, or the complications of their interact ions with other genes or environments. ○ Quantitative genetics is as much about the environment as it is about genetics. Because heritability is always substantially less than 100%, quantitative genetic research provides the best available evidence for the importance of the environment, while controlling f or the environment. Why quantitative genetics will continue to be important ○ Quantitative genetics will continue to be an important part of the future of developmental research for three reasons: ○ It is genomic. ○ It is as much about the environment as it is about genetics. We are a long way from identifying all of the genes responsible for the heritability of any complex trait. PSYC0036 Genes and Behaviour Page 3 ○ We are a long way from identifying all of the genes responsible for the heritability of any complex trait. Examples of how quantitative genetics is being used in developmental research today ○ Longitudinal studies have revealed genetic change as well as continuity in development. ○ Multivariate studies have found a surprising degree of genetic overlap as well as specificity between traits. ○ Studies of gene–environment interplay have demonstrated the importance of gene–environment correlation as well as interaction. ○ Twin studies are being used to investigate individual differences at all the -omic levels between genes and behavior (van Dongen, Slagboom, Draisma, Martin, & Boomsma, 2012). ○ Developmental studies are increasingly using genetically sensitive designs such as the twin method to identify causal environ mental effects free of genetic mediation. ○ Epigenomic studies are using discordant monozygotic (MZ) twins to identify biomarkers of nonshared environmental influences t hat make genetically identical co-twins different. Quantitative Genetics Using DNA Alone Quantitative genetics using DNA alone ○ DNA-based quantitative genetic methods, such as GCTA, can be used to estimate the net effect of genetic influence on traits using DNA alone in unrelated individuals. ○ GCTA requires genotyping hundreds of thousands of SNPs for many thousands of individuals. ○ GCTA has been used to study a wide range of traits, including common medical diseases, psychopathology, cognitive abilities, personality, and economic and political preferences. ○ GCTA estimates of genetic influence are generally only about half the heritabilities estimated by twin studies. ▪ This is likely due to a combination of factors, including the fact that GCTA only detects the additive effects of common SNPs and is limited to detecting genetic effects that can be detected by the SNPs that happen to be incorporated in the DNA microarrays used in GWA studies. ○ Bivariate GCTA can be used to study the covariance between two traits. This has been used to confirm important twin study fin dings about cognitive development, such as the extensive overlap (pleiotropy) of genetic effects on diverse cognitive abilities. The promise of GCTA ○ GCTA provides a new approach that brings the potential of quantitative genetic analysis to any large sample of unrelated indi viduals for whom genome-wide genotypes are available. ○ The promise of GCTA will be more fully realized as it is used to go beyond simply confirming the heritability of traits to in vestigate developmental, multivariate, and gene–environment questions. The future of genomics research for developmentalists ○ Nothing will advance the future of genomics research for developmentalists more than identifying the specific G, C, T, and A sequence variation responsible for heritability. Finding the Missing Heritability Finding the missing heritability ○ GCTA predicts that additive effects of common variants on commercially available DNA arrays can account for about half of the heritability. The implications for future attempts to identify the DNA variants responsible for heritability ○ GWA has been successful in identifying hundreds of genes for hundreds of common diseases and quantitative traits. ○ The largest effect sizes for the additive effects of common SNPs on current DNA arrays are astonishingly small in the populat ion. ○ GWA research implies that if the largest effects are so small, the smallest effects are likely to be infinitesimal. ○ Incredibly large samples are needed to detect such small effects. The largest effects are very small ○ The largest effect sizes for quantitative traits are closer to 0.1%, comparable to a correlation of.03. ○ The largest effect sizes for behavioral traits are also tiny. ▪ For example, the largest study to date for a behavioral trait has recently been reported for the complex trait of total years of education, with the largest association accounting for only 0.02% of the variance. Implications for psychiatric disorders ○ Associations for psychiatric disorders are smaller than those found for medical disorders, although even here the largest eff ect sizes involve odds ratios of less than 1.2. Implications for future research ○ GWA research implies that if the largest effects are so small, the smallest effects are likely to be infinitesimal. ○ Incredibly large samples are needed to detect such small effects. Missing heritability from GWA research using common SNPs on current DNA arrays Aggregating the effects of individual SNPs ▪ Even though the effect sizes of individual SNPs are very small, their effects can be aggregated to estimate the size of the missing heritability gap. ▪ Selecting DNA variants using less stringent criteria generally explains more variance in independent samples. □ For example, for weight, 32 replicated SNPs explain about 2% of the variance, but thousands of SNPs explain about 5% of the variance. Implications for different traits ▪ For height, 180 SNPs account for 10% of the variance, which increases to 13% when more SNPs are added. ▪ For total years of education, the GWA meta-analysis with more than 100,000 individuals explained about 1% of the variance using 3,500 SNPs and 2.5% of the variance using all 2.5 million SNPs. ▪ For childhood IQ, 180 SNPs explained about 1% of the variance. ▪ For medical disorders, where GWA consortia have been large enough to detect many reliable associations, specific SNPs in total account for up to about 8% of the liability. ▪ For coronary artery disease, 150 SNPs accounted for about 5% of the total variance of liability. ▪ For schizophrenia, significant SNPs accounted for about 1% of the liability, and all SNPs accounted for almost 6% of the liability. ▪ For bipolar disorder, SNPs account for 1% to 3% of the liability. The future of GWA studies ▪ As larger samples are amassed, the common SNPs on current DNA arrays will account for more of the missing heritability. The biggest question in genomic sciences ▪ The biggest question now in the genomic sciences is where the rest of the missing heritability can be found. Beyond common SNPs on current DNA arrays Beyond common SNPs on current DNA arrays ▪ Less common DNA variants, including less common SNPs, may be responsible for some of the missing heritability. ▪ Whole-exome sequencing has revealed that we each have several dozen non-inherited (de novo) gene-disrupting mutations, some of which have been shown to contribute to sporadic cases of neurodevelopmental disorders. ▪ Rare, large CNVs involving hundreds of thousands or even millions of base pairs have been shown to be risk factors for several common diseases. ▪ More than 10,000 smaller common CNVs have been identified with a frequency of at least 5% in the population. ▪ Repeat sequence variants consist of two, three, or four base pairs that are repeated up to 100 times, creating multiple alleles, and have been found at as many as 50,000 loci throughout the genome. Methods for detecting rare variants ▪ Gene-based analyses can aggregate multiple DNA variants in a gene, including rare variants. ▪ Grouping genes in terms of pathway networks of related functions can help identify nonadditive gene–gene interactions. ▪ Aggregating rare variants can help identify deleterious mutations. Worries and hopes ▪ It is worrying that GWA research relies on additive effects, and that nonadditive gene–gene or gene–environment interactions may be difficult to identify. ▪ There is hope that quantitative genetic research is correct in its conclusion that most genetic variance is additive. ▪ There is also a need for careful behavioral measurement, for including assessments of the environment, and for a developmental perspective. Polygenic Scores PSYC0036 Genes and Behaviour Page 4 Polygenic scores ○ Polygenic scores are aggregate genotypic scores consisting of hundreds or thousands of DNA variants. ○ Polygenic scores can predict children’s genetic risk and resilience. ○ Polygenic scores will replace studies of a few SNPs in a candidate gene. Definition and calculation of polygenic scores ○ Polygenic scores are calculated by summing the genotype scores for each DNA variant, weighted by the strength of their associ ation with the phenotype. ○ Polygenic scores are typically additive, but non-additivity at a locus (dominance) or between loci (epistasis) can also be incorporated. Implications of polygenic scores for developmental research ○ Polygenic scores can be used to investigate developmental, multivariate, and gene–environment issues as genetic predictors. ○ Polygenic scores draw attention to the positive end of the normal distribution of polygenic scores, which has been called pos itive genetics. Custom DNA arrays ○ Custom DNA arrays will become available that will assess hundreds of thousands of DNA variants relevant to particular traits at a cost less than the current cost of genotyping a few candidate genes. Whole-genome sequencing ○ Whole-genome sequencing has the huge advantage that, once a genome is sequenced, there is no more genotyping to be done. ○ A tipping point will come in the next few years as the plummeting cost of whole-genome sequencing approaches the cost of DNA arrays. Pleiotropic polygenic scores Pleiotropy and polygenicity ○ Pleiotropy is the manifold effects of genes; that is, each gene affects many traits. ○ Pleiotropy might drive polygenicity: if each gene affects many traits then many traits will be influenced by many genes. ○ Substantial pleiotropy has been found for cognitive and learning abilities and disabilities, childhood psychopathology, and a dult psychosis. ○ GWA studies have confirmed the genetic overlap between schizophrenia and bipolar disorder. ○ Extensive overlap of DNA variants has also been found for other medical disorders, especially autoimmune diseases. Multivariate GWA studies ○ Multivariate GWA studies focus on genetic associations in common across traits. ○ A multivariate GWA analysis of five major psychiatric disorders found three SNPs that were associated with all five disorders. ○ A more truly multivariate GWA analysis would focus on the covariance per se between traits rather than the variance of each t rait. Implications for developmental research ○ Pleiotropic polygenic scores could be created that predict what is in common across broad domains such as psychopathology and cognitive abilities and disabilities. ○ Trait-specific polygenic scores that remove the considerable genetic variance spread across traits are also likely to provide clean er targets for research on typical and atypical development. The End of Genotyping: Whole-Genome Sequencing The cost of whole-genome sequencing is dropping quickly and is predicted to become competitive with the cost of DNA arrays. Sequencing the entire genome yields all DNA sequence variation, meaning that no more genotyping is required. Parents have begun to pay to have their children's DNA sequenced, and it is likely that in some countries, all children will have their DNA sequenced. Future of Genomics for Developmentalists ○ Developmental clinicians can use polygenic scores derived from DNA sequence data to provide diagnostic information, suggest p ersonalized interventions, and enable early prediction. ○ Developmental researchers can use polygenic scores to predict genetic propensities, trace how those genetic dispositions develop, and understand links between the genome, epigenome, transcriptome, proteome, neurome, and behavior. ○ Genomics will serve as a common denominator integrating all the life sciences. The next 10 years of behavioural genomic research Introduction (Plomin, 2022) The fusion of quantitative and molecular genetics ○ Quantitative genetics focuses on complex traits such as behavioral traits that were presumed to be influenced by many genes o f small effect. ○ Molecular genetics focuses on single-gene disorders. ○ The two fields converged as advances in molecular genetics made it possible to go beyond single-gene effects to investigate complex traits influenced by many genes. Genome-wide association (GWA) ○ GWA studies genotype thousands of DNA variants across the genome to identify associations with traits of interest. ○ GWA studies have revealed that complex traits are extremely polygenic, with thousands of SNPs contributing to heritability. ○ GCTA and LD score regression are two methods for estimating heritability and genetic correlations using GWA summary statistic s. Polygenic scores ○ Polygenic scores are weighted sums of SNP genotypes, with weights derived from GWA summary statistics. ○ Polygenic scores can be used to predict risk for complex traits, even when individual SNPs have small effects. Future research directions ○ The genetic architecture of psychopathology ▪ GCTA and LD score regression have revealed substantial genetic correlations between different psychiatric disorders, suggesting that they share a common genetic architecture. ▪ Future research will focus on identifying the specific genes and pathways that contribute to this shared genetic architecture. ○ Causal modelling of gene-environment interplay ▪ Gene-environment interactions are complex and difficult to study using traditional methods. ▪ Future research will use polygenic scores and other tools from behavioral genomics to develop causal models of gene-environment interplay. ○ The use of DNA as an early warning system ▪ Polygenic scores can be used to identify individuals at high risk for developing complex traits. ▪ Future research will explore the use of polygenic scores to develop early warning systems for complex traits, such as psychiatric disorders. The genetic architecture of psychopathology The inadequacies of current nosology (i.e., the branch of medical science dealing with the classification of diseases) ○ Current psychiatric diagnoses are based on symptoms, which have been shown to be unreliable and highly comorbid. ○ Attempts to reclassify disorders on the basis of presumed causes such as neural processes have been unsuccessful. ○ Genetics is a unique causal factor in psychopathology, and can be used to reveal its underlying genetic architecture. The genetic architecture of psychopathology ○ Genetic and genomic research has revealed that the genetic architecture of psychopathology is very different from current symptom-based diagnoses. ○ Future research in the next 10 years can reveal the genetic architecture of psychopathology by: ▪ Conducting large-scale genome-wide association studies (GWAS) of psychopathological traits. ▪ Using polygenic scores to identify genetic variants that contribute to risk for multiple psychopathological disorders. ▪ Developing new statistical and computational methods to analyze genetic data and identify the underlying genetic architecture of psychopathology. Genetic correlations between disorders GENETIC CORRELATIONS BETWEEN DISORDERS: ○ Genetic research has shown that current diagnostic categories are inadequate. ○ Anxiety and depression have a genetic correlation near 1.0, indicating they do not differ genetically. ○ Schizophrenia and bipolar disorder share many associated SNPs. Behavioral genomic analysis can estimate genetic correlations between disorders without assessing them in the same individual s. PSYC0036 Genes and Behaviour Page 5 ○ Behavioral genomic analysis can estimate genetic correlations between disorders without assessing them in the same individual s. ○ The highest genetic correlation (0.87) was found between major depressive disorder (MDD) and anxiety disorders (ANX). ○ Other correlations include schizophrenia (SCZ) and bipolar disorder (BIP) at 0.68, ADHD and autism (AUT) at 0.38, anorexia ne rvosa (AN) and obsessive-compulsive disorder (OCD) at 0.46, and post-traumatic stress disorder (PTSD) with MDD (0.75), ANX (0.58), and ADHD (0.78). GENETIC FACTORS CLUSTERS: ○ Four clusters emerged: internalizing (MDD and ANX), psychotic (SCZ and BIP), developmental (ADHD and AUT), and compulsive (AN and OCD). ○ PTSD loaded on both internalizing and developmental factors. ○ There is a positive manifold among all 11 disorders, reflecting a transdiagnostic factor called "p." DISORDERS AS DIMENSIONS: ○ Common disorders are dimensions, not genetically distinct disorders. ○ Polygenic scores from case-control studies show continuous genetic risk for psychopathology. ○ Disorders correlate genetically with quantitative traits. ○ A personality perspective suggests considering problems as extremes of normal personality dimensions, which are normally dist ributed. REVEALING THE GENETIC ARCHITECTURE: ○ Research needs to move beyond the limitations of current diagnostic nosology. ○ A hierarchical model with a "p" factor on top is emerging. ○ Building the genetic architecture from dimensional measures is crucial. ○ The normal distribution of polygenic scores will enable research on both ends of the distribution. FUTURE DIRECTIONS: ○ Over the next decade, behavioral genomic research will reveal the genetic architecture of psychopathology. ○ The predictive structure of childhood psychopathology in adulthood will be explored. ○ The clinical utility of knowing the genetic structure is still uncertain, but the scientific value is evident. Causal modelling of GE interplay CAUSAL MODELLING OF GE INTERPLAY: ○ In the next decade, behavioral genomic research will focus on causal modelling of the interplay between genes and environment (GE). ○ Traditional quantitative genetic methods offered limited insights into GE interplay, such as interactions (GxE) and correlati ons (rGE). ○ Twin and adoption studies provided initial insights into GxE and rGE. ○ The first stage emphasized the importance of rGE, showing substantial genetic influence on environmental measures and mediati on of environmental measures by genetics. ○ The second stage introduced candidate gene research but suffered from low power due to the small effects of single DNA varian ts. ○ The third stage, behavioral genomics, uses genome-wide association (GWA) genotyping data for large samples but faces challenges in replicating GxE and rGE findings. ○ The focus of this section is on methods enabling new possibilities for causal modeling of GE interplay. MENDELIAN RANDOMIZATION: ○ Mendelian randomization is a method that uses genetics to identify causal effects of modifiable environmental factors on outc omes. ○ It leverages the random allocation of genotypes from parents to offspring. ○ It can be used to isolate the causal effect of an exposure if certain assumptions are met. ○ Although it has been applied to single-gene effects, its application to complex psychopathology is more challenging due to polygenic effects and rGE. BACK TO FAMILIES: ○ GWA analyses in unrelated individuals include rGE effects as well as between-family effects like ethnicity and socioeconomic status. ○ Within-family correlations help control for between-family effects. ○ Within-sibship analyses eliminate passive rGE but not evocative or active rGE. ○ Trios consisting of two parents and a child can help tease apart rGE. ○ Parental polygenic scores can predict their children's traits independently of the children's polygenic scores. ○ These effects are called "indirect effects" or "genetic nurture," but they primarily control for passive rGE. ○ These analyses do not specify which environmental factors are responsible for the effects. ○ Measured environments can be incorporated to investigate evocative and active rGE. CAUSAL MODELLING OF GE INTERPLAY USING POLYGENIC SCORES: ○ Causal modeling of GE interplay will be an important focus in the coming years. ○ The challenge is to disentangle direct genetic effects from rGE. ○ Strategies similar to the candidate gene era will be used, but with polygenic scores instead of candidate genes. ○ Emphasis on replication and systematic multivariate studies will be crucial. Using polygenic scores as an early warning system Using polygenic scores as an early warning system ○ Polygenic scores can be used to predict psychopathology in adulthood, even in childhood. ○ Polygenic scores could be used as an early warning system to identify children at high risk for developing psychopathology. ○ The goal is to develop polygenic scores that predict more than 10% of the variance in psychopathology. Reaching the 10% target ○ Polygenic scores already predict more than 10% of the variance for quantitative traits such as intelligence, educational atta inment, and educational achievement. ○ There is plenty of headroom for increasing the predictive power of polygenic scores for psychopathology. ○ The ultimate ceiling for polygenic score prediction is twin heritability, but the current ceiling is SNP heritability. ○ The missing heritability gap can be narrowed through larger GWA case-control studies and by using dimensional measures and longitudinal assessments. ○ Another type of missing heritability is the gap between SNP heritability and estimates of heritability using family and twin designs. ○ Narrowing this missing heritability gap will require different technologies such as whole -genome sequencing. Implications and applications Implications and applications of polygenic scores ○ Polygenic scores are transforming research in developmental psychology by democratizing genomics and making it possible to us e genomic data in any moderately sized sample of unrelated individuals. ○ Polygenic scores have the potential to transform clinical work from symptoms to causes, from treatment to prediction and prev ention, from onesize-fits-all interventions to individually tailored interventions, and from qualitative diagnoses to quantitative dimensions. ○ Polygenic scores will likely be incorporated into newborn screening and prenatal screening in the future. ○ Developmental researchers and clinicians may soon be able to add polygenic scores to their research at no cost other than ana lytic costs. ○ More predictive polygenic scores can be created by moving beyond Eurocentric samples to more diverse samples. Conclusion ○ The outpouring of opportunities created by behavioural genomics is unparalleled in the behavioural sciences. ○ Understanding the genetic architecture of psychopathology, investigating genetic and environmental causal paths, and using po lygenic scores as an early warning system will also eventually transform clinical practice. Common disorders are quantitative traits Introduction (Plomin et al., 2009) Plomin et al. (2009) argue that genome-wide association research is bringing together the two worlds of genetics, Mendelian and biometric, and that this has implications for how we think about common disorders. They suggest that, in the future, we will see common disorders as the extremes of quantitative traits, rather than as distinct entities. This has far-reaching implications for research, clinical practice, and our understanding of human behavior. The two worlds of genetics Mendelians looked for Mendelian patterns of inheritance in qualitative traits. Biometricians argued that Mendel's laws could not apply to quantitative traits because such traits showed no simple pattern of inheritance. The disagreement between the Mendelians and biometricians ○ The disagreement was resolved when biometricians realized that Mendel's laws of inheritance of single genes would also apply to complex traits that are influenced by several genes, each of which is inherited according to Mendel's laws. This resolution was formalized in R. A. Fisher's 1918 paper, 'The correlation between relatives on the supposition of Mendeli an inheritance'. PSYC0036 Genes and Behaviour Page 6 ○ This resolution was formalized in R. A. Fisher's 1918 paper, 'The correlation between relatives on the supposition of Mendeli an inheritance'. Quantitative genetics versus molecular genetics ○ Quantitative geneticists investigated the genetics of naturally occurring phenotypic variation by using methods that estimate d the cumulative effect of genetic influence. ○ Molecular geneticists studied how genes work rather than particular phenotypes: they focused on rare naturally occurring mono genic effects or experimentally induced ones. Coming together in genome-wide association research ○ GWA research finds associations of small effect size with odds ratios of less than two, which implies that many such genes ar e needed to account for the heritability of the disorders. Qualitative disorders as quantitative traits ○ If GWA studies indicate that multiple genes affect common disorders, this implies that their genetic liability is distributed quantitatively rather than qualitatively. Thinking quantitatively Multiple DNA variants are associated with common disorders. This leads to a shift in thinking about disorders in quantitative terms. DNA variants can be aggregated into composites representing polygenic liability underlying common disorders. Identification of Quantitative Traits: ○ Common disorders can be viewed as extremes of quantitative traits. ○ Some disorders have obvious associated quantitative traits (e.g., BMI for obesity). ○ For many disorders, the relevant quantitative traits are unclear. ○ Disorders like alcoholism, arthritis, autism, cancers, dementia, diabetes, and heart disease lack clearly defined associated quantitative traits. Shifting Vocabulary: ○ A shift in vocabulary is needed to promote thinking quantitatively. ○ Replace 'disorders' with 'dimensions.' ○ Replace genetic 'risk' with genetic 'variability.' Statistical Understanding: ○ Familiarity with the statistics of quantitative traits is essential. ○ Use linear regression instead of logistic regression. ○ Focus on variance rather than mean differences. ○ Consider covariance instead of comorbidity. Normal Distribution of Polygenic Liability: ○ The polygenic liability for common disorders follows a normal distribution. ○ The challenge is to determine how quantitative traits reflect this liability. Broad Analysis Levels: ○ Quantitative traits can occur at any level of analysis, not limited to symptoms of the diagnosed disorder. Identifying quantitative mechanisms Multiple genes associated with a disorder lead to understanding the mechanisms by which each gene affects the disorder. Quantitative traits are recognized at various levels of analysis, including gene expression profiles, other '-omic' levels, physiology, and brain structure/function. Examples of Quantitative Approaches: ○ Type 2 diabetes (T2D) has embraced a quantitative approach with significant results. ○ Studies have focused on quantitative traits related to T2D, such as fasting glucose, c-reactive protein, and glucose tolerance. ○ These studies refine the definition of T2D. Examples from Crohn's Disease (CD): ○ Crohn's disease studies illustrate how quantitative traits related to the disease can emerge from genome-wide association (GWA) studies. ○ GWA studies identify genes associated with CD susceptibility. ○ The search for mechanisms behind these genes leads to quantitative traits, including inflammatory response, bacterial surviva l, and chronic inflammation. ○ Autophagy is implicated as a previously unexpected quantitative mechanism in CD pathogenesis. Potential for Other Disorders: ○ Other disorders under GWA scrutiny may also lead to the identification of quantitative traits. ○ GWA studies reveal that various quantitative mechanisms underlie disorders. ○ Sets of variants associated with each mechanism may relate to disease subtypes. Weighting Disease Genes and Pleiotropy: ○ GWA studies suggest using weighted sets of variants to reflect polygenic liability and predict clinically useful features. ○ Evidence indicates unexpected pleiotropic overlap among genetic variants affecting different human diseases. ○ Genetic links exist between subtypes of the same disorder and apparently disparate conditions. ○ The concept of the human 'diseasome' synthesizes all human genetic disorders and disease genes, revealing these connections. ○ As molecular databases of genome-wide assays mature, these links and mechanisms will become clearer. Future Implications: ○ The practice of weighting disease genes may extend beyond narrow classifications of qualitative diseases to a full quantitati ve understanding of the multivariate diseasome PSYC0036 Genes and Behaviour Page 7 The practical use of polygenic risk scores Polygenic risk scores are sets of multiple DNA variants associated with a disorder. They are used to predict the population-wide genetic risk for common disorders, such as breast cancer, atherosclerosis, coronary heart disease, and others. They can also be used in research without clinical sensitivity and specificity thresholds. Research applications of polygenic risk scores can lead to quantitative traits, as they are themselves normally distributed quantitative traits. Sensitive Genotypic Selection: ○ Polygenic risk scores can be used for selecting individuals at high polygenic risk based on genotype rather than phenotype. ○ This is particularly useful in intensive and expensive research, such as neuroimaging, where large sample sizes are required due to the small effect sizes of individual DNA variants. ○ More subtle and sensitive quantitative trait measures will be needed to investigate the multivariate traits reflecting polyge nic risk. Thinking Positively: ○ Thinking quantitatively about polygenic liability encourages a positive direction in research. ○ Instead of just focusing on vulnerabilities in individuals with high polygenic risk scores, it suggests investigating the res ilience of individuals with low polygenic risk scores. ○ This approach could lead to mechanisms promoting healthy outcomes and interventions for public health. Studying the Full Range of Variation: ○ Polygenic risk scores encourage research on the full range of normal quantitative trait variation. ○ Studying populations rather than probands may better reflect polygenic risk scores. ○ Multivariate research based on polygenic risk scores might reveal networks of related quantitative traits. ○ Even when DNA variants have differing functions, their joint association with a disorder indicates functional overlap. ○ Research on the full range of normal variation has implications for genome-wide association (GWA) studies. Implications for GWA studies Quantitative traits reflecting polygenic liability have practical implications for Genome-Wide Association (GWA) research. Enhancing Statistical Power: ○ GWA studies for common disorders can benefit from comparing the low and high extremes of quantitative traits or studying the entire distribution. ○ This approach is more powerful than dichotomizing the distribution into cases and controls, especially as disorders become mo re common. ○ A quantitative approach is advantageous as it avoids contamination of the control group by individuals who nearly reach the d iagnostic thresholds for the disorder. Benefit for Rare Disorders: ○ Identifying quantitative dimensions of complex traits is valuable for dissecting rare disorders. ○ Population cohort studies with extensive data on multiple quantitative phenotypes and their changes over time are helpful for exploring how disorders develop and relate to each other in etiological terms. ○ These studies complement the prevalence of case-control GWA studies. Emphasis on Population Cohort Studies: ○ The increasing emphasis on population cohort studies opens up opportunities for new phenotyping. ○ Population cohorts with relevant quantitative phenotypes serve as ideal sampling frames for new data collection. ○ Advances in technology, such as web-based testing and digitalization of patient records, allow the accumulation of massive amounts of quantitative information on population cohorts. ○ This will lead to a holistic understanding of the origins of human disease, contributing to the field of phenomics. Perspectives Common disorders result from multiple genes affecting them, leading to a continuous distribution of polygenic liability following a normal curve. Common disorders are the quantitative extremes of continuous genetic risk distributions. The hypothesis is that polygenic risk scores will not only be associated with differences between cases and controls but alsowith individual differences throughout the entire range of variation in relevant traits. Challenges in Identifying Relevant Quantitative Traits: ○ The challenge is identifying the relevant quantitative traits for most disorders. ○ Research on polygenic risk scores captures the diffuse pleiotropic effects of genes and considers the full range of normal va riation in population cohort studies. ○ Sensitivity measures beyond case-control discrimination are often needed to assess individual differences throughout the normal distribution. Clinical Implications: ○ Polygenic risk scores are already used to predict individuals at high polygenic risk. ○ Current effect sizes of associations, even when aggregated, do not yet reach the sensitivity and specificity levels required for clinical utility. ○ A broader clinical repercussion is the shift toward thinking quantitatively about the full range of normal variation, includi ng the previously neglected "other end" of the normal distribution of polygenic risk. Impact on Diagnosis and Interventions: ○ Qualitative diagnoses may give way to quantitative dimensions due to research on polygenic risk. ○ This trend is visible in the field of mental disorders, where new diagnostic procedures include dimensional approaches. ○ Thinking quantitatively leads to a public health model that evaluates the population's risk quantitatively and focuses on pre vention rather than just treating cases. ○ Improved prediction for effective prevention will happen by studying complex traits as multivariate continuous dimensions, mo ving beyond clinical diagnoses and definitions. Conclusions Quantitative dimensions will become more important than qualitative disorders in polygenic liability research. Extreme values of quantitative traits are important medically and socially. Diagnostic constructs are historically based on symptoms rather than aetiology, and reifying them has no scientific advantage. PSYC0036 Genes and Behaviour Page 8 Diagnostic constructs are historically based on symptoms rather than aetiology, and reifying them has no scientific advantage. From the perspective of polygenic liability, common disorders do not exist, only the extremes of quantitative traits. PSYC0036 Genes and Behaviour Page 9