Module 8 Emerging Trends in IS Management PDF
Document Details
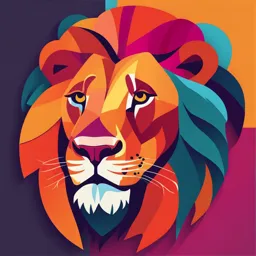
Uploaded by RealizableCarnelian2080
Isabela State University
Tags
Summary
This document discusses cloud computing, its impact on information systems management, and strategic considerations for adoption. It covers topics like definitions, characteristics, types of cloud deployments, and operational efficiency associated with cloud systems.
Full Transcript
Republic of the Philippines Isabela State University Echague, Isabela College of Computing Studies, Information and Communication Technology Module 8 Emerging Trends in IS Management Topics Covered...
Republic of the Philippines Isabela State University Echague, Isabela College of Computing Studies, Information and Communication Technology Module 8 Emerging Trends in IS Management Topics Covered Cloud computing and its impact on IS management Big data analytics and business intelligence Artificial Intelligence and machine learning applications in IS Cloud Computing and Its Impact on IS Management Objective To explore the concepts, benefits, challenges, and strategic implications of cloud computing in Information Systems (IS) management, emphasizing its transformative effects on organizational operations, scalability, security, and strategic decision-making. Introduction Welcome to today's lecture on cloud computing and its impact on IS management. Cloud computing has revolutionized how organizations store, manage, and process data and applications. By leveraging cloud services, businesses can achieve greater flexibility, scalability, and efficiency in IT operations. Today, we’ll delve into the fundamentals of cloud computing, its implications for IS management practices, and strategic considerations for adopting cloud technologies. Understanding a. Definition and Characteristics: Cloud Computing Cloud Computing: Refers to the delivery of computing services (e.g., storage, processing power, applications) over the internet, providing on-demand access to shared resources. Key Characteristics: Scalability, elasticity, pay-as-you-go pricing model, self-service provisioning, and resource pooling. b. Types of Cloud Deployments: i. Public Cloud: Services provided by third-party cloud service providers (CSPs) accessible to multiple organizations or the general public (e.g., AWS, Microsoft Azure, Google Cloud). Private Cloud: Dedicated cloud infrastructure operated solely for a single organization, offering enhanced control, security, and customization. Hybrid Cloud: Combination of public and private cloud environments, allowing data and applications to be shared between them based on workload requirements. Impact of Cloud a. Operational Efficiency: Computing on IS i. Scalability and Flexibility: Enables organizations to scale resources up Management or down based on demand, optimizing resource utilization and operational efficiency. Agility: Facilitates rapid deployment of new applications, updates, and services to meet business needs and respond to market changes effectively. Republic of the Philippines Isabela State University Echague, Isabela College of Computing Studies, Information and Communication Technology b. Cost Savings: i. CapEx to OpEx: Shifts IT expenses from capital expenditures (CapEx) to operational expenditures (OpEx), reducing upfront infrastructure costs and providing predictable billing. Resource Optimization: Minimizes costs associated with maintenance, upgrades, and hardware procurement by leveraging cloud provider’s infrastructure. Strategic a. Data Security and Privacy: Considerations i. Risk Management: Addresses concerns about data breaches, for IS compliance with regulatory requirements (e.g., GDPR, HIPAA), and Management implementing robust security measures. Encryption: Secures data in transit and at rest, protecting sensitive information from unauthorized access and cyber threats. b. Vendor Management: i. Service Level Agreements (SLAs): Defines performance metrics, availability, uptime guarantees, and responsibilities between organizations and cloud service providers. Vendor Lock-in: Evaluates risks and strategies for mitigating dependencies on specific cloud providers, ensuring flexibility and interoperability. Challenges in a. Data Integration and Interoperability: Cloud Computing i. Legacy Systems: Integrating existing IT infrastructure and legacy Adoption applications with cloud environments to ensure seamless data flow and interoperability. Inter-cloud Migration: Overcoming challenges associated with migrating data and applications between different cloud platforms and environments. b. Compliance and Regulatory Concerns: i. Jurisdictional Issues: Navigating international data residency requirements, cross-border data transfers, and ensuring compliance with regional privacy laws. Audits and Certifications: Obtaining certifications (e.g., SOC 2, ISO 27001) to demonstrate compliance with industry standards and regulatory frameworks. Future Trends a. Edge Computing: Integrating cloud computing capabilities closer to the and Innovations edge of the network, reducing latency and enabling real-time data processing for IoT devices and applications. Serverless Computing: Adopting serverless architectures (e.g., AWS Lambda, Azure Functions) for event-driven applications, optimizing resource utilization and reducing operational overhead. b. AI and Machine Learning (ML) Integration: i. AIaaS and MLaaS: Leveraging cloud-based AI and ML services for predictive analytics, data insights, and automation, enhancing business intelligence and decision-making capabilities. Republic of the Philippines Isabela State University Echague, Isabela College of Computing Studies, Information and Communication Technology Ethical AI: Addressing ethical considerations in AI development and deployment within cloud environments, including bias mitigation, transparency, and accountability. Conclusion Today, we have explored cloud computing and its impact on IS management, examining its transformative effects on operational efficiency, scalability, security, and strategic decision-making. By understanding the benefits, challenges, and strategic considerations of cloud adoption, organizations can harness the power of cloud technologies to drive innovation, optimize IT resources, and achieve sustainable growth in a digital economy. Discussion How can organizations overcome challenges related to data security and compliance when adopting cloud computing solutions? What role does cloud computing play in supporting digital transformation initiatives and enhancing organizational agility and competitiveness? Assignment Evaluate the strategic advantages and potential risks of migrating a specific organizational function or application to a public cloud environment. Analyze considerations related to cost savings, scalability, security, and regulatory compliance to propose recommendations for cloud adoption. Big Data Analytics and Business Intelligence Objective To explore the concepts, methodologies, benefits, challenges, and strategic implications of big data analytics and business intelligence (BI) in organizations, emphasizing their transformative role in decision-making, innovation, and competitive advantage. Introduction Welcome to today's lecture on big data analytics and business intelligence. In the digital age, organizations are inundated with vast amounts of data generated from various sources. Big data analytics and BI are instrumental in extracting actionable insights from this data to drive informed decision- making, optimize processes, and gain competitive advantages. Today, we’ll delve into the fundamentals of big data analytics, BI concepts, methodologies, and strategic considerations for leveraging data-driven insights in organizational contexts. Understanding a. Definition and Characteristics: Big Data Big Data: Refers to large volumes of structured, semi-structured, Analytics and unstructured data generated at high velocity and variety. Big Data Analytics: Involves processes, tools, and techniques to analyze and derive meaningful insights from big data sets. Republic of the Philippines Isabela State University Echague, Isabela College of Computing Studies, Information and Communication Technology b. Key Characteristics of Big Data: Volume: Large amounts of data that exceed traditional processing capabilities. Velocity: Speed at which data is generated, collected, and processed in real-time. Variety: Diversity of data types, including text, images, videos, sensor data, social media feeds, etc. Business a. Definition and Purpose: Intelligence (BI) Business Intelligence (BI): Refers to technologies, applications, Fundamentals and practices for collecting, integrating, analyzing, and presenting business data to facilitate decision-making. Key Components: Data warehousing, data mining, reporting, dashboards, and data visualization. b. Benefits of BI: Decision Support: Provides timely, accurate, and relevant insights to support strategic, tactical, and operational decision-making. Performance Monitoring: Tracks key performance indicators (KPIs) and metrics to measure organizational performance and identify trends. Operational Efficiency: Improves efficiency by automating reporting processes, reducing manual effort, and streamlining data access. Big Data a. Descriptive Analytics: Analytics Reporting and Dashboards: Summarizes historical data to Techniques provide insights into past performance and trends. Data Visualization: Uses charts, graphs, and interactive visualizations to communicate insights effectively. b. Predictive Analytics: Machine Learning (ML) Models: Applies statistical algorithms and ML techniques to predict future outcomes and trends based on historical data patterns. Forecasting: Uses predictive models to anticipate customer behavior, market trends, and operational demands. c. Prescriptive Analytics: Optimization Models: Recommends actions and strategies to optimize decision-making processes based on predictive insights and business objectives. Scenario Analysis: Simulates various scenarios to evaluate potential outcomes and make informed decisions. Strategic a. Competitive Advantage: Implications of Data-Driven Decision Making: Enables organizations to gain Big Data insights into customer preferences, market trends, and competitive Analytics and BI dynamics to drive innovation and strategic initiatives. Republic of the Philippines Isabela State University Echague, Isabela College of Computing Studies, Information and Communication Technology Agility and Flexibility: Adapts quickly to changing market conditions and customer demands through real-time analytics and responsive decision-making. b. Customer Experience and Personalization: 360-Degree View: Integrates customer data from multiple touchpoints to create personalized experiences and improve customer satisfaction and loyalty. Behavioral Analytics: Analyzes customer behavior and interactions to enhance marketing campaigns, product recommendations, and service offerings. Challenges and a. Data Quality and Integration: Considerations Data Cleansing: Ensures accuracy, completeness, and consistency of data across multiple sources and formats. Data Integration: Integrates disparate data sources to create a unified view for analysis and decision-making. b. Privacy and Security:** Ethical Use of Data: Ensures compliance with privacy regulations (e.g., GDPR, CCPA) and ethical standards in data collection, storage, and use. Data Security: Implements robust security measures to protect sensitive information from unauthorized access, breaches, and cyber threats. Future Trends in a. AI and Automation:** Big Data Cognitive Analytics: Integrates AI and natural language Analytics and BI processing (NLP) to derive insights from unstructured data sources (e.g., social media, customer reviews). Automated Insights: Uses AI-driven algorithms to automate data analysis, anomaly detection, and trend identification in real-time. b. Edge Computing:** Decentralized Data Processing: Moves data processing and analytics closer to the data source (e.g., IoT devices, sensors) to reduce latency and bandwidth usage. Conclusion Today, we have explored big data analytics and business intelligence, examining their concepts, methodologies, benefits, challenges, and strategic implications for organizations. By leveraging big data analytics and BI, organizations can harness the power of data-driven insights to optimize operations, enhance decision-making capabilities, and achieve sustainable growth in a competitive global market. Discussion How can organizations overcome challenges related to data quality and integration when implementing big data analytics solutions? What are the ethical considerations and best practices for ensuring responsible use of data in BI and analytics initiatives? Republic of the Philippines Isabela State University Echague, Isabela College of Computing Studies, Information and Communication Technology Assignment Conduct a case study analysis of a company that successfully implemented big data analytics and BI to achieve competitive advantage. Evaluate their strategies, challenges faced, and lessons learned to propose recommendations for other organizations looking to embark on similar initiatives. Artificial Intelligence and Machine Learning Applications in Information Systems Objective To explore the concepts, applications, benefits, challenges, and strategic implications of Artificial Intelligence (AI) and Machine Learning (ML) in Information Systems (IS), emphasizing their transformative role in decision-making, automation, and innovation. Introduction Welcome to today's lecture on Artificial Intelligence (AI) and Machine Learning (ML) applications in Information Systems (IS). AI and ML technologies are revolutionizing how organizations analyze data, automate processes, and derive actionable insights. Today, we’ll delve into the fundamentals of AI and ML, their applications across various IS domains, and strategic considerations for leveraging these technologies to drive organizational efficiency, innovation, and competitive advantage. Understanding a. Definition and Concepts: Artificial Artificial Intelligence (AI): Refers to the simulation of human Intelligence (AI) intelligence in machines that can perform tasks that typically and Machine require human intelligence, such as learning, reasoning, problem- Learning (ML) solving, and decision-making. Machine Learning (ML): Subset of AI that involves training algorithms to learn patterns and make predictions from data without being explicitly programmed. b. Types of Machine Learning: i. Supervised Learning: Trains models on labeled data to make predictions or decisions (e.g., classification, regression). Unsupervised Learning: Identifies patterns and structures in data without labeled outcomes (e.g., clustering, anomaly detection). Reinforcement Learning: Learns through trial and error by interacting with an environment to maximize rewards and achieve predefined goals. AI and ML a. Predictive Analytics: Applications in Customer Behavior Prediction: Uses historical data to forecast Information customer preferences, buying patterns, and churn rates. Systems (IS) Demand Forecasting: Predicts future demand for products or services based on historical sales data and market trends. b. Natural Language Processing (NLP): Text Analysis: Analyzes and extracts insights from unstructured textual data, such as customer reviews, social media posts, and emails. Republic of the Philippines Isabela State University Echague, Isabela College of Computing Studies, Information and Communication Technology Chatbots and Virtual Assistants: Uses NLP to understand and respond to natural language queries and automate customer support interactions. c. Image and Video Analysis: Computer Vision: Applies ML algorithms to analyze and interpret visual data, such as object recognition, image classification, and video surveillance. Medical Imaging: Assists in medical diagnostics through image analysis and pattern recognition in medical imaging data. Strategic a. Enhanced Decision Making: Implications of AI Data-Driven Insights: Provides real-time analytics and predictive and ML in IS insights to support strategic, tactical, and operational decision- making. Automation: Automates routine tasks and processes, reducing human error and operational costs while improving efficiency. b. Personalization and Customer Experience: Recommendation Systems: Recommends personalized products, services, and content based on individual preferences and behavior. Dynamic Pricing: Adjusts prices dynamically based on market demand, competitor pricing, and customer behavior analysis. Challenges and a. Data Quality and Bias: Considerations Data Preprocessing: Cleanses and preprocesses data to ensure accuracy, completeness, and consistency for reliable model training. Bias Mitigation: Addresses biases in training data and algorithms to ensure fairness and avoid discriminatory outcomes. b. Ethical and Regulatory Issues: Privacy Concerns: Ensures compliance with data protection regulations (e.g., GDPR, CCPA) and ethical guidelines in handling sensitive personal information. Transparency and Accountability: Enhances transparency in AI decision-making processes and accountability for AI-driven outcomes. Future Trends in a. Explainable AI (XAI): AI and ML Interpretable Models: Develops AI models that provide explanations and insights into decision-making processes for transparency and trust. Ethical AI: Integrates ethical considerations into AI design, deployment, and governance to promote responsible AI use. b. AI at the Edge: Edge Computing: Moves AI processing closer to data sources (e.g., IoT devices, sensors) to reduce latency, enhance data privacy, and enable real-time decision-making. Republic of the Philippines Isabela State University Echague, Isabela College of Computing Studies, Information and Communication Technology Conclusion Today, we have explored AI and ML applications in Information Systems (IS), examining their concepts, methodologies, benefits, challenges, and strategic implications for organizations. By leveraging AI and ML technologies, organizations can harness the power of data-driven insights, automation, and personalization to drive innovation, optimize operations, and achieve sustainable growth in a competitive digital landscape. Discussion How can organizations balance the benefits of AI and ML with ethical considerations and regulatory compliance in IS applications? What are the key factors to consider when integrating AI and ML technologies into existing IS infrastructure to maximize business value? Assignment Conduct a case study analysis of a company that successfully implemented AI or ML in an IS application (e.g., predictive analytics, NLP). Evaluate their strategies, challenges faced, and outcomes achieved to propose recommendations for future AI/ML initiatives.