MODULE-2.pptx
Document Details
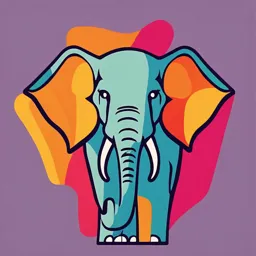
Uploaded by AttractiveDecagon238
Jain University
Tags
Related
Full Transcript
Revolutionising B.Tech Module 2: Potential outcomes of Causal Reasoning Course Name: Causal Reasoning [22TCSE313] Table of Content Aim Objectives Self Assessments Activities – Did You Know Summary Terminal Questions Aim The Aim of t...
Revolutionising B.Tech Module 2: Potential outcomes of Causal Reasoning Course Name: Causal Reasoning [22TCSE313] Table of Content Aim Objectives Self Assessments Activities – Did You Know Summary Terminal Questions Aim The Aim of the Potential outcomes framework in causal reasoning is to provide a systematic and rigorous approach to define, identify, and estimate causal effects. This framework, also known as the Rubin Causal Model (RCM), is central to understanding and conducting causal inference The potential outcomes framework in causal reasoning aims to: Define causal effects with precision. Provide a systematic approach for estimating these effects. Highlight and address the fundamental problem of causal inference. Incorporate and test necessary assumptions. Clarify the role of bias and confounding. Objective Support a variety of causal inference methods. Enable sensitivity analysis to ensure robust conclusions Potential outcomes Causal reasoning involves identifying relationships between causes and effects Potential outcomes in causal reasoning typically refer to the different results that could occur based on variations in the causal variable This framework is crucial in fields like statistics, economics, epidemiology, and social sciences. B r a d y Potential outcomes: intuition N e Inferring the effect of treatment/policy on some outcome a l 4 / Potential outcomes: intuition Inferring the effect of treatment/policy on some outcome Take pill Brady 4 / Potential outcomes: intuition Inferring the effect of treatment/policy on some outcome Take pill Don’t take pill Brady 4 / Potential outcomes: intuition Inferring the effect of treatment/policy on some outcome Take pill causal effect Don’t take pill Brady 4 / Potential outcomes: intuition Inferring the effect of treatment/policy on some outcome Take pill causal effect? Don’t take pill Brady 4 / Potential outcomes: intuition Inferring the effect of treatment/policy on some outcome Take pill no causal effect Don’t take pill Brady 4 / B r a d y Potential outcomes: notation N do(T = e a 1) l 5 / (1)Potential Outcomes Framework (Rubin Causal Model): This framework is often used to define causal effects. For each unit (e.g., individual, group), there are potential outcomes under different treatment conditions. Treated Outcome (Y1): The outcome if the unit receives the treatment. Control Outcome (Y0): The outcome if the unit does not receive the treatment. (2)Average Treatment Effect (ATE): ATE=E[Y1−Y0] This measures the average effect of a treatment or intervention across a population. It is the difference in outcomes between the treated and untreated groups. Conditional Average Treatment Effect (CATE): This is the average treatment effect for a population subgroup defined by certain characteristics. CATE(X)=E[Y1−Y0∣X]. Individual Treatment Effect (ITE): This is the difference in potential outcomes for a specific individual. ITEi​=Y1i​−Y0i​. This refers to the effect of a treatment on an individual. It varies from person to person and helps in personalized decision-making Counterfactuals: These involve considering what would happen if a different action or intervention were taken. For example, "What would the outcome be if the treatment was not given?“ To determine if getting a dog will make you happy Notation: Y(1): Your level of happiness if you get a dog. Y(0): Your level of happiness if you do not get a dog Possible Outcomes: 1.If you get a dog (Treatment = 1): 1. You observe your happiness level, which is Y(1). 2.If you do not get a dog (Treatment = 0): 1. You observe your happiness level, which is Y(0). Analysis: 1.Case 1: Happiness Increase with Dog 1. Suppose Y(1) = 8 (on a happiness scale of 1 to 10). 2. Suppose Y(0) = 5. 3. Causal Effect: Y(1) - Y(0) = 8 - 5 = 3. 4. In this case, the dog has a positive causal effect on your happiness, as having a dog increases your happiness by 3 points. Case 2: No Change in Happiness Suppose Y(1) = 7. Suppose Y(0) = 7. Causal Effect: Y(1) - Y(0) = 7 - 7 = 0. In this case, getting a dog has no causal effect on your happiness, as your happiness level would be the same regardless of having a dog or not. Case 3: Happiness Increase Without Dog Suppose Y(1) = 6. Suppose Y(0) = 8. Causal Effect: Y(1) - Y(0) = 6 - 8 = -2. In this case, not getting a dog actually makes you happier. The dog has a negative causal effect on your happiness, decreasing it by 2 points. Counterfactual: The key to understanding causality here is the counterfactual scenario—what your happiness level would be if you did not get the dog Necessity: If you would have become happy even without the dog (Y(0) = Y(1)), then the dog was not necessary for your happiness. Sufficiency: If getting the dog alone can explain the increase in your happiness (Y(1) > Y(0)), then the dog can be considered a sufficient cause for your happiness. Confounding Factors: Other factors (such as life events, social support, changes in environment) that might influence your happiness independently of getting a dog should be considered to isolate the dog's effect. Using the potential outcomes framework helps to quantify and understand whether getting a dog has a causal effect on your happiness by comparing your observed happiness with the dog (Y(1)) to the counterfactual scenario without the dog (Y(0)). By doing this, you can better assess the necessity and sufficiency of the dog in contributing to your happiness. The Fundamental Problem of Causal Inference The Fundamental Problem of Causal Inference (FPCI) refers to the difficulty of observing the causal effect of a treatment on an individual directly because we cannot observe both potential outcomes simultaneously. That is, we cannot observe what happens to an individual both when they receive the treatment and when they do not at the same time. This creates a challenge in determining the true causal effect. Counterfactuals: The causal effect is defined in terms of potential outcomes, which include both the outcome if the treatment is applied (Y(1)) and the outcome if the treatment is not applied (Y(0)). For any given individual, we can only observe one of these outcomes in reality, either Y(1) or Y(0), not both. The unobserved outcome is the counterfactual. Individual Causal Effect: The causal effect for an individual is the difference between their potential outcomes: Y(1) - Y(0). Since we can only observe one of these outcomes for each individual, we cannot directly calculate the individual causal effect. Examples Consider a scenario where you are trying to determine if a new drug causes a reduction in blood pressure. Y(1): Blood pressure level if the individual takes the new drug. Y(0): Blood pressure level if the individual does not take the new drug. For an individual who takes the drug, we observe Y(1), but we do not observe Y(0). Conversely, for an individual who does not take the drug, we observe Y(0), but not Y(1). Randomized Controlled Trials: Randomly assign individuals to either the treatment (new drug) group or the control (placebo) group. Compare the average blood pressure levels between the two groups to estimate the average treatment effect. Observational Study with Matching: Identify individuals who took the drug and find similar individuals (based on characteristics like age, weight, and baseline blood pressure) who did not take the drug. Compare the average outcomes between these matched groups to estimate the causal effect. The Fundamental Problem of Causal Inference highlights the intrinsic challenge of causal inference—observing both potential outcomes for the same individual is impossible. Researchers address this problem by focusing on average effects and employing various methodologies and assumptions to estimate causal relationships from observed data. Getting Around the Fundamental Problem The Fundamental Problem of Causal Inference (FPCI) posits a significant challenge in causal analysis: it is impossible to observe both potential outcomes for the same individual under different treatments simultaneously. This means that for any given person, we can only see what happens when they receive the treatment (Y(1)) or when they do not (Y(0)), but never both. To navigate this issue, researchers employ several strategies and methodologies One of the most robust approaches is the use of Randomized Controlled Trials (RCTs). In an RCT, subjects are randomly assigned to either the treatment or control group, ensuring that both groups are statistically equivalent and that any difference in outcomes can be attributed to the treatment itself. When randomization is not feasible, observational studies with methods like matching, instrumental variables, and regression analysis come into play. These methods attempt to control for confounding factors by comparing individuals with similar characteristics or using instruments that influence the treatment but not the outcome directly. Additionally, assumptions like ignorability, which assumes no unmeasured confounders, and the Stable Unit Treatment Value Assumption (SUTVA), which assumes that the treatment of one individual does not affect the outcome of another, are critical in making valid causal inferences. Through these techniques and assumptions, researchers can approximate the causal effects and mitigate the limitations imposed by the FPCI, thereby advancing our understanding of causal relationships. Average Treatment Effects and Missing Data Interpretation Average Treatment Effects (ATE) Definition: The Average Treatment Effect is the average difference in outcomes between units that receive the treatment and those that do not. It is expressed as: ATE=E[Y(1)−Y(0)] where: Y(1) is the potential outcome if the unit receives the treatment. Y(0) is the potential outcome if the unit does not receive the treatment. Interpretation of Missing Data In the context of causal inference, the challenge arises because for each individual, we only observe one of these potential outcomes (either Y(1) or Y(0). The other outcome is considered missing. Addressing this missing data issue is crucial for estimating the ATE accurately. Methods for Estimating ATE Randomized Controlled Trials (RCTs) Description: Randomization ensures that the treatment and control groups are statistically similar, allowing the difference in their average outcomes to estimate the ATE. Formula: ATE=E[Y∣T=1]−E[Y∣T=0] Interpretation: The average difference in outcomes between the treated and control groups. Observational Studies Matching Description: Match treated individuals with similar untreated individuals based on observed covariates. Formula: is the outcome of the matched control unit. Interpretation: The average difference in outcomes between treated units and their matched controls. Propensity Score Matching: Description: Use propensity scores to match treated and untreated individuals with similar probabilities of receiving the treatment. Interpretation: The average difference in outcomes between treated units and their matched controls based on similar propensity scores Regression Adjustment Description: Use regression models to adjust for differences in covariates between treated and untreated groups Interpretation: The difference in outcomes between treated and untreated units after adjusting for covariates Instrumental Variables (IV) Description: Use an instrument that affects treatment assignment but not the outcome directly. Handling Missing Data In any method, handling the missing potential outcomes (i.e., the unobserved counterfactuals) is crucial. Various imputation techniques and sensitivity analyses can be employed to ensure the robustness of the estimated ATE. Multiple Imputation Description: Generate multiple sets of plausible values for the missing data based on observed data patterns. Interpretation: The average of the imputed values provides an estimate of the missing outcomes, which can then be used to calculate the ATE Sensitivity Analysis Description: Assess how the estimated ATE changes under different assumptions about the missing data. Interpretation: Provides a range of possible ATE values, indicating the robustness of the causal inference. Example : Consider a study on the effect of a new educational program on student test scores. RCT Example: Treatment Group: Students who receive the new educational program. Control Group: Students who do not receive the new educational program. ATE: The difference in average test scores between the treatment and control groups Observational Study Example: Matching: Match students who participated in the program with similar students who did not based on covariates like prior test scores and socioeconomic status. Propensity Score Matching: Estimate propensity scores for each student and match based on these scores. Regression Adjustment: Use regression to adjust for differences in covariates between the two groups Instrumental Variables: Use the distance to the nearest school offering the program as an instrument to estimate the causal effect. Ignorability: Ignorability, also known as the assumption of no unmeasured confounders, is a key condition in causal inference. It states that treatment assignment is independent of the potential outcomes given observed covariates. Formally, it is expressed as: where: Y(0)and Y(1)are the potential outcomes. T is the treatment indicator (1 if treated, 0 if not). X represents the observed covariates. Importance: Ignorability ensures that the differences in outcomes between treated and untreated groups can be attributed to the treatment itself, rather than to other confounding factors Assumptions: 1.Conditional Independence: Given the observed covariates X, the treatment assignment T is independent of the potential outcomes Y(0) and Y(1). 2.Positivity: There is a positive probability of receiving each treatment level for all values of covariates X. Implications: If ignorability holds, causal effects can be estimated using observational data by controlling for the observed covariates X. Exchangeability Definition: Exchangeability refers to the idea that the distribution of potential outcomes is the same for treated and untreated groups, conditional on the observed covariates. It is a similar concept to ignorability but is often used in the context of randomized experiments. Formally, it implies Importance: Exchangeability ensures that the treated and untreated groups are comparable, making it possible to attribute differences in outcomes to the treatment effect rather than to pre-existing differences between the groups. Assumptions: Random Assignment: In a randomized controlled trial (RCT), random assignment ensures exchangeability by design. Conditional Exchangeability: In observational studies, exchangeability must be achieved by conditioning on observed covariates. Implications: If exchangeability holds, the causal effect can be estimated by comparing the outcomes between treated and untreated groups, either directly (in RCTs) or after adjusting for covariates (in observational studies). Summary Ignorability is the assumption that, conditional on observed covariates, treatment assignment is independent of potential outcomes. This allows for causal inference in observational studies by controlling for covariates. Exchangeability ensures that, conditional on observed covariates, the distribution of potential outcomes is the same for treated and untreated groups. This is often achieved through randomization in RCTs or conditioning on covariates in observational studies. Conditional Exchangeability and Unconfoundedness Conditional Exchangeability: Conditional exchangeability refers to the assumption that within levels of observed covariates, the potential outcomes are independent of the treatment assignment. This means that, after accounting for observed covariates, the treated and untreated groups are comparable in terms of their potential outcomes. Formally, it is expressed as: Importance: Conditional exchangeability allows researchers to use observational data to estimate causal effects by adjusting for observed covariates, making the treated and untreated groups comparable. Assumptions: Observed Covariates: All confounding variables that influence both the treatment assignment and the potential outcomes must be observed and included in X. Independence Given Covariates: Within each level of the observed covariates XXX, the potential outcomes are independent of the treatment assignment. Implications: If conditional exchangeability holds, the causal effect of the treatment can be estimated by comparing outcomes between treated and untreated groups, conditional on the covariates. Unconfoundedness Definition: Unconfoundedness, also known as the assumption of no unmeasured confounders, implies that the treatment assignment is independent of the potential outcomes given the observed covariates. This assumption is similar to conditional exchangeability and is critical for causal inference in observational studies. Formally, it is expressed as: Importance: Unconfoundedness ensures that any differences in outcomes between treated and untreated groups can be attributed to the treatment itself, rather than to other confounding factors. Assumptions: 1.Observed Covariates: All relevant confounders that affect both the treatment and the outcomes must be measured and included in the analysis. Conditional Independence: Given the observed covariates XXX, the treatment assignment TTT is independent of the potential outcomes Y(0)and Y(1). Implications: If unconfoundedness holds, causal effects can be estimated from observational data using techniques like regression adjustment, matching, or propensity score methods, as these methods rely on the assumption that there are no unmeasured confounders Summary Conditional Exchangeability: This assumption means that within levels of observed covariates, the treated and untreated groups are comparable regarding their potential outcomes. It allows for causal inference by ensuring that differences in outcomes can be attributed to the treatment rather than to other factors. Unconfoundedness: This assumption states that treatment assignment is independent of the potential outcomes given observed covariates. It ensures that observed covariates capture all relevant confounding variables, allowing for accurate estimation of causal effects from observational data. Positivity/Overlap and Extrapolation Positivity/Overlap: Positivity, also known as overlap, is an assumption in causal inference that ensures every unit in the population has a positive probability of receiving each treatment level, given the observed covariates. Importance: Positivity is crucial because it ensures that there are enough comparable units across different treatment groups for every combination of covariates. This comparability is essential for estimating causal effects without bias. Assumptions: 1.Non-Zero Probability: Every individual has a non-zero probability of receiving each treatment level. No Deterministic Treatment Assignment: There should be no combination of covariates that determine. Implications: When positivity holds, we can estimate causal effects across the entire range of observed covariates. Violation of this assumption (e.g., if certain covariate combinations only occur in one treatment group) can lead to biased estimates because there is no basis for comparison between treated and untreated groups for those covariate combinations terministically predicts the treatment assignment Extrapolation Definition: Extrapolation refers to the practice of extending conclusions beyond the range of the observed data. In causal inference, extrapolation involves making inferences about causal effects outside the support of the observed covariate distribution. Importance: Extrapolation is often necessary in practice when researchers are interested in the causal effects for populations or contexts that differ from the original study. However, it can introduce significant uncertainty and potential bias if the conditions and covariate distributions in the new context are substantially different from those in the original data. Challenges: 1.Lack of Data: Extrapolating beyond the observed range means relying on assumptions about relationships that have not been directly observed. 1.Increased Uncertainty: Predictions made outside the range of the data are less reliable because they depend on the assumption that observed relationships hold in unobserved regions. 2.Risk of Bias: Extrapolating can lead to biased estimates if the underlying relationships change outside the observed range. Implications: Extrapolation should be approached with caution. Researchers should clearly state their assumptions and consider the potential for increased uncertainty and bias. Robustness checks, sensitivity analyses, and validation with external data can help mitigate these risks. Summary Positivity/Overlap: Ensures that all units have a positive probability of receiving each treatment level, given their covariates. This assumption is essential for making unbiased causal inferences, as it guarantees comparability across treatment groups. Extrapolation: Involves extending causal inferences beyond the observed data range. While often necessary, it introduces significant uncertainty and potential bias. Careful consideration of assumptions and validation with external data are important when extrapolating. No interference, Consistency, and SUTVA No Interference Definition: The assumption of no interference, also known as the assumption of no spillover effects or the stable unit treatment value assumption (SUTVA), posits that the treatment assigned to one unit does not affect the outcomes of other units. Each unit's potential outcome is only influenced by its own treatment assignment. Importance: This assumption is crucial for isolating the causal effect of a treatment on an individual unit without contamination from other units' treatments. Implications: Isolated Effects: Ensures that causal effects can be attributed solely to the treatment received by each individual unit. Validity of Inferences: Violation of this assumption can lead to biased estimates, as outcomes could be influenced by the treatment status of other units. Example: In a clinical trial, no interference means that the treatment given to one patient does not affect the health outcomes of another patient. Consistency Definition: The consistency assumption states that the potential outcome under the observed treatment is equal to the actual observed outcome. Formally, if a unit receives treatment T=t then the observed outcome Y is equal to the potential outcome Y(t). Importance: Consistency ensures that the treatment effect being measured corresponds accurately to the treatment received. Implications: Accurate Attribution: Ensures that the observed outcomes are correctly attributed to the treatments received. Reliable Estimates: Violation of this assumption can lead to incorrect estimates of the causal effect because the observed outcomes do not reflect the intended treatment effects. Example: In a drug trial, if a patient is given a specific dosage of a drug, the observed health outcome should match the potential outcome for that dosage. Stable Unit Treatment Value Assumption (SUTVA) SUTVA encompasses two main components: 1.No Interference: The treatment assigned to one unit does not affect the outcomes of other units. 2.Consistency: The observed outcome for each unit corresponds to the potential outcome under the actual treatment received by that unit. Importance: SUTVA is a foundational assumption in causal inference, ensuring that each unit's potential outcomes are not influenced by other units' treatments and that observed outcomes reflect the intended treatments. Implications: Isolated and Accurate Effects: Ensures that causal effects can be accurately estimated without interference or misattribution. Validity of Estimates: Violations of SUTVA can lead to biased and unreliable causal estimates. Example: In an educational intervention study, SUTVA means that the outcome (e.g., test scores) of one student is not affected by the treatment received by another student, and each student's observed outcome matches the potential outcome under the given treatment Summary No Interference: Assumes that the treatment of one unit does not affect the outcomes of other units, ensuring isolated treatment effects. Consistency: Assumes that the observed outcome for a unit under a specific treatment is equal to the potential outcome for that treatment, ensuring accurate attribution. SUTVA: Combines no interference and consistency, ensuring that causal effects are isolated and accurately attributed to the treatments received. This foundational assumption is critical for the validity of causal inferences Activities Surprise Quiz in Class. Summary Outcomes: Terminal Questions Reference Links Thank you