Reservoir Modeling PDF
Document Details
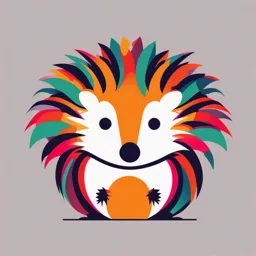
Uploaded by IndividualizedNihonium
University of Mosul
Tags
Summary
This document provides an overview of reservoir modeling, from its early stages to advanced techniques employing machine learning and artificial intelligence. It discusses the different types of models, their applications, and the challenges involved in building and using reservoir models.
Full Transcript
Reservoir Modelling 1- Introduction A reservoir model is a digital representation of a subsurface reservoir using geological, geophysical, and engineering data. Simulation refers to the process of running numerical models on these representations to predict fluid flow behavior within the reser...
Reservoir Modelling 1- Introduction A reservoir model is a digital representation of a subsurface reservoir using geological, geophysical, and engineering data. Simulation refers to the process of running numerical models on these representations to predict fluid flow behavior within the reservoir. Reservoir simulation is performed to infer fluid flow behavior from a mathematical model. The integration of these two processes allows engineers to optimize production strategies, estimate reserves accurately, and make informed decisions regarding field development plans. The goal of reservoir modeling and fluid simulation is increased hydrocarbon production with an increased rate of return. The 3D quantification is performed in a geo-cellular model that consists of reservoir geometry, lithology, porosity, permeability and initial fluid saturation (Fig.1). Integration of information from seismic data, cores, wireline logs and outcrops provide the quantification of the static reservoir model of the reservoir. Static reservoir model provides a representation of the structure, thickness, lithology, porosity, initial fluids in the reservoir. A dynamic reservoir model is a representation of the changes in fluid flow in the reservoir model that needs to be validated with reservoir performance data-pressure changes, production and injection rates (Fig.2). Building a reservoir model includes the construction of a structural and stratigraphic model and determining the spatial distributions of facies and various petrophysical properties in the model. Therefore, when we build models of oil and gas resources in the subsurface we should never ignore the fact that the fluid resources are contained within rock formations. Constructing a good reservoir model requires multi- specialties analyses and integration of geological, geophysical, petrophysical, and reservoir engineering data using scientific and statistical inferences. So building a model of an oil and gas reservoir is complex because of the variety of data types involved as the many different steps required. Reservoir modelling is also a challenge. The challenge is to integrate measurements that are of different Scale, Uncertainty, Resolution, and Environment or the SURE Challenge (Fig.3). In addition to the complex fluids present in the reservoir. 1 2- Evolution of Reservoir Modeling Techniques Over the Years: Reservoir modeling techniques have evolved significantly over the years, with the introduction of new technologies and advancements in data processing and analysis. The following is a general overview of the evolution of reservoir modeling techniques. 1- Early Reservoir Models: The earliest reservoir models were basic and relied on simple geological data to estimate reservoir properties and fluid flow. They were limited in their accuracy and did not take into account complex geological features and heterogeneities. These models were mainly used for exploration purposes and to estimate the size of the reservoir. 2- 2D Reservoir Models: In the 1980s, 2D reservoir models were introduced, which allowed for a more accurate representation of geological features and heterogeneities. These models were able to simulate fluid flow in more complex reservoirs, such as those with faults and fractures. However, they still had limitations in their ability to capture the full complexity of the reservoir. 3- 3D Reservoir Models: In the 1990s, 3D reservoir models became more widely used, allowing for a more accurate representation of the reservoir and its properties. These models were able to simulate fluid flow in complex reservoirs and were used to optimize production strategies. They provided a more detailed understanding of the reservoir's structure and properties, enabling operators to make better decisions about drilling and production. 4- Integrated Reservoir Models: In the 2000s, integrated reservoir models were introduced, which combined geological, geophysical, and engineering data to create a more comprehensive model of the reservoir. These models allowed operators to simulate fluid flow and production in real-time, enabling them to adjust production strategies based on changing conditions. They also allowed for better prediction of future reservoir behavior and provided a more complete understanding of the reservoir. 5- Advanced Reservoir Models: In recent years, advanced reservoir modeling techniques have been developed, including machine learning and artificial intelligence-based models. These models are able to analyze large amounts of data and make predictions about future reservoir behavior. They can identify patterns and trends that may not be immediately apparent to human operators, allowing for more informed decisions about production strategies. These models are particularly useful in unconventional reservoirs, where traditional modeling techniques may not be sufficient. 2 3- The Uses of Reservoir Modelling: 1- Evaluation of rock volumes and the original hydrocarbons in place. 2- Representation of geological and petrophysical descriptions of the reservoir for input to reservoir simulation. 3- Increase profitability through better reservoir management, including development plans for new fields and depletion strategies for mature fields. 4- Prediction of the fluid volume (oil, gas, and water), decline analysis, secondary or tertiary recovery option injection strategies, and well and completion designs. 5- Observation of fluid movement contacts and pressures. 6- Analysis of fault seal and transmissibility in addition to calculating the displacement of the fault vertically and laterally. 7- Assessment of wells number and types that required to produce the reservoir economically (e.g. vertical, slant, horizontal, multilateral, etc.) and locations. 4- Reservoir Modeling According to the Stages the Reservoir Life Cycle: 4-1 Exploration Stage: The exploration stage requires delineation of the reservoir limits and assessment of its economic feasibility. Enhance depositional environment and conceptual model understanding. Refine stratigraphic model. Assess fault partition. Identify new prospects. Use the model as a data/information store. 4-2 Development Stage: The development stage requires a somewhat more accurate assessment of the reservoir extent and better appraisal of the economic viability of the reservoir. Build more-detailed structural and stratigraphic model. Plan and design wells, including well path. Computing the production profiles (oil, gas, and water). Estimating the oil and gas technical reserves. Assess intermediate-scale reservoir heterogeneities and connectivity. 4-3 Production Stage: Assess small-scale heterogeneities, including flow units modeling. Use for reservoir management. 3 Matching of the past production history (fluid rates, GOR, pressures, etc). Optimize production in the field. Perform enhanced oil recovery (EOR) Types of Reservoir Models 1-Structural Model: The structural framework for all reservoir models is defined by a combination of structural inputs (faults and surfaces from seismic to impart gross geometry) and stratigraphic inputs (to define internal layering). Building the structural model is the first step in the modelling workflow. The objective is to construct a consistent reservoir framework that can be gridded for 3D geological or dynamic simulation. The framework should capture the large-scale heterogeneities affecting flow in the reservoir, such as faults or unconformities. Large-scale tectonic settings have significant impacts on the characteristics of subsurface formations. In reservoir characterization, structural elements, such as sequence boundaries, unconformities, major tectonic folding, and large faults, typically control reservoir construction. What is a common task in structural interpretation of seismic data? A common task in structural interpretation of seismic data is to analyze and derive major sequence boundaries and other critical entities that may control hydrocarbon storage. For reservoir modeling, these large-scale geological properties should be built into the structural and stratigraphic framework because of their control on hydrocarbon trap volume and large-scale reservoir zonations. 1-1 Seismic Interpretation: Structural analysis is largely based on the interpretation of 2D and 3D seismic interpretation. The seismic interpreter is looking for potential hydrocarbon traps in the data; these may be structural or stratigraphic (Fig.23) and may exhibit a Direct Hydrocarbon Indicator (DHI) (Fig.22). The quality and extent of the data set will determine what can be achieved. Seismic data are measured in two-way travel time; the time it takes for an acoustic wave to travel to a reflector and return to the receiver. A seismic reflection is a measure of the contrast in elasticity between two different rocks, also known as the reflection coefficient. 4 The reflection coefficient can be estimated from the velocity (Vp) and bulk density (𝜌b) of the rocks using values from the sonic and density logs. A direct expression of a seismic interpretation will tend to be a conservative representation of the fault architecture, because it will directly reflect the resolution of the data. Facets of such data are: Fault networks tend to be incomplete, e.g. faults may be missing in areas of poor seismic quality Faults may not be joined (under-linked) due to seismic noise in some areas. Horizon interpretations may stop short of faults due to seismic noise around the fault zone. Horizon interpretations may be extended down fault planes. There are a number of uncertainties in seismic interpretation; this is often a function of data quality or due to interpretation error. The main sources of uncertainties in seismic interpretation as follows: 1- Seismic well tie: The reflection recorded on the seismic section may not tie the same event in a well; poor seismic resolution can result in a 10-20 m mismatch. 2- Seismic pick: The reflector that is being traced may lose coherency or continuity introducing another uncertainty. 3- Imaging: The seismic response weakens with depth or below high-velocity layers as the acoustic energy is depleted, resulting in poorer amplitude response and lower resolution. 4- Depth conversion: This process leads to greatest systematic uncertainty in seismic interpretation. A description of the variability or tolerance can be quantified for future uncertainty workflows: 1- Depth Conversion: Velocity analysis is a very important part of the process leading to the seismic framework. The analysis involves finding velocity trends and velocity functions that yield a proper velocity model for depth conversion (Fig.24).There are two main sources of velocity information: well data and seismic imaging. 5 There are many methods to convert seismic times to depths (Fig.25). Depth conversion methods can be separated into two broad categories: direct time-depth conversion, and velocity modeling for depth conversion. Whichever method is selected, an accurate and reliable depth conversion is one that will 1) tie the existing wells, and 2) accurately predict depths at new well locations. 1-2 Fault Modeling: The structural framework based on input data such as fault sticks, gridded surfaces and line data. - Fault modeling: is the process of mapping faults in 3D within the reservoir. The purpose of this step is to define the shape of each of the faults that should be modeled (Fig.27). This is done by generating “key pillars”. - Pillar gridding: process, where a set of pillars will be inserted in the entire project area (Fig.27). The result of the pillar gridding process is a “skeleton grid”, defined by all of the faults and all of the pillars. - Layering: The final step is to insert the horizons into the faulted 3D grid. 1-2-1 Fault Modelling Workflow Preparation and assigning the input data to the faults: Fault modelling is performed using a variety of fault input data types. Generate the fault network: In the network, Hanging Wall and Foot Wall are defined for the faults (Fig.28). The fault network can be automatically generated or created by the user (digitized). Generate fault surfaces: The fault surfaces can be gridded using any data source that describes the position of the fault planes in space (Fault sticks, Fault surfaces, and Fault line and/or polygon data). Generate horizon lines: These lines are manually/ automatically generated by resampling the intersection using an influence distance and an extrapolation distance (Fig.29). Adjust horizons to the faults: The final step in the fault-modelling process is to ensure that the horizons match the fault model. 6 1-3 Horizon Modeling: Horizon modeling leads directly to the stratigraphic modeling (Fig.31). Horizons in a reservoir model are used to capture the large-scale geological relationship represented by the seismic interpretation; they can represent the base of a sequence, an erosional event or a discontinuous surface. 2- Stratigraphic Model: 2-1 Introduction A stratigraphic model is the model framework that incorporates the stratigraphic surfaces and zonations. A stratigraphic model may or may not have faults. Both structural and stratigraphic models are also called a geological or reservoir model framework. A reservoir framework can be constructed from geological interpretations of stratigraphic surfaces (Fig.33). Geological surfaces are interpreted using sequence stratigraphic analysis and are then used to construct the framework. For subsurface formations, one cannot interpret surfaces as easily as for outcrops because of limited and indirect data. The stratigraphic levels in the reservoir framework is represented by seismically interpreted horizons or events and geologically significant surfaces identified in well data: where the levels are identified in both data sets, then the mapped seismic horizons are constrained by the well picks 2-3 Well-to-Well Correlation: The product of well correlation provides a fundamental constraint on the reservoir framework. Well correlation should be done using TVD (true vertical depth) will stretch/squeeze the thickness, especially of highly deviated wells (fig. 36). 2-4 Stratigraphic Surfaces: The surfaces were used to construct the framework with conformable stratigraphic zonations (Fig.37). The choice of horizon type is the decision to use a lithostratigraphic well correlation or to employ sequence stratigraphic principles. 2-5 How Many Zones: A zone in a grid-based modelling context is a modelling unit or sub-grid (Fig.38). In conventional map-based modelling, the zones are introduced to keep a certain 7 level of vertical resolution. 3D geocellular modelling builds a grid within each zone, thus simplifying the reservoir model. 2-5-1 Multi-Zone Grid or Single-Zone Grids: Models can be built as single-zone grids (SZG) or multi zone grids (MZG) depending on what is required by the modelling team: speed, accuracy, simplicity. 1- Reasons for Using MZG: Everything is stored within one zone container. Operations can be performed on all zones at the same time or separetly. Fault modelling will easier, as the grid construction is performed for the full reservoir. With SZG, it is possible to generate faults that are inconsistent from one zone to another 2- Reasons for Using SZG: If only one or a few zones are of interest for a particular study, it is much faster to work with SZG than a huge multi-zone, both for visualization and calculations. You can easily choose independent well data sets for each zone for conditioning. For instance, if well tracks are good in some zones whereas poor in other zones, you can make flexible choices. With MSG, you have to use the same XY grid resolution on all your zones. This means that if a zone needs a particularly refined XY grid, all other zones will have to build with that resolution. Using SZG makes it possible for several people to work with different zones at the same time. 2-6 Geocellular Model The structural and stratigraphic models provide the skeleton of the reservoir framework and comprise the largely deterministic element. The geocellular model, a generic term often used to describe any 3D model, provides the fine-scale internal architecture of the reservoir that will ultimately be populated with facies and petrophysical properties. The definition of the internal architecture should not be done without reference to the conceptual depositional model and the dynamic properties of the reservoir. 8