Midterm Review STA 6176 Biostatistics PDF
Document Details
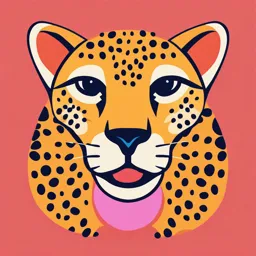
Uploaded by HeartfeltBigfoot7892
Florida International University
Wensong Wu
Tags
Summary
This document is a review for the STA 6176 Biostatistics midterm exam, covering topics such as the binomial model, chi-square tests for association and goodness-of-fit, and categorical data analysis. The material is presented by Wensong Wu.
Full Transcript
STA 6176 Biostatistics Midterm Review Instructor: Wensong Wu Wensong Wu Biostatistics Midterm Review Review by Topics I Counting Data I Topic 4: Binomial model, Binomial test (small sample) and Z test (large sample) for Binomial proportion. I To...
STA 6176 Biostatistics Midterm Review Instructor: Wensong Wu Wensong Wu Biostatistics Midterm Review Review by Topics I Counting Data I Topic 4: Binomial model, Binomial test (small sample) and Z test (large sample) for Binomial proportion. I Topic 5: Z test (large sample) and Fisher’s exact test (small sample) for two proportions, Hypergeometric model. I Topic 6: Poisson model, CI of Binomial proportion by Poisson approximation (large n, small π) I Topic 7: Multinomial model, χ2 Test for goodness-of-fit. I Categorical Data I Topic 8: χ2 Test for association in two-way contingency table. I Topic 9: χ2 Test for trend in 2 × k table. Wensong Wu Biostatistics Midterm Review Review by Types of Data I One categorical variable. I Two outcomes: Binomial model. I More than two outcomes: Multinomial model and goodness of fit. I Two categorical variables. I 2 × 2 table: Hypergeometric model and Fisher’s test. I r × c table: Association. I 2 × k table: Trend. Wensong Wu Biostatistics Midterm Review One Variable, Two Outcomes Success Failure Total Y n−Y N I Binomial model: Y ∼ Bin(n, π), where π =P(success). I Assumptions of Binomial model: Binary outcome, independent and identical trails. I Test for π: H0 : π = π0 , H1 : π , 6= π0 I Small sample (n ≤ 50): Binomial Exact test for π. I Moderately large sample (n ≤ 50 and nπ0 (1 − π0 ) ≥ 10): Z test for π with continuity correction. I Large sample (n ≤ 50 and nπ0 (1 − π0 ) ≥ 100): Z test for π. I Large n small π (n ≥ 20, π ≤ 0.1, and observed y ≥ 5): Poisson Approximated CI for π. Wensong Wu Biostatistics Midterm Review One Variable, More Than Two Outcomes Category 1 Category 2... Category k Total N1 N2... Nk N· I Multinomial model: (N1 , N2 ,..., Nk ) ∼ Multinomial(N· , π1 , π2 ,..., πk ). I χ2 test for goodness-of-fit: I H0 : π1 = π10 ,...., πk = πk0 , H1 : At least one is not equal. I Expected: E (Ni ) = nπi0. I DF=k − 1. I Require large sample. Wensong Wu Biostatistics Midterm Review Two Variables, 2 × 2 Table Success Failure Total Sample 1 n11 n12 n1· Sample 2 n21 n22 n2· Total n·1 n·2 I Two models: I Binomial model given row totals. I n11 ∼ Bin(n1· , π1 ), where π1 =P(Success) in Sample 1. I n21 ∼ Bin(n2· , π2 ), where π2 =P(Success) in Sample 2. I Two samples are independent. I Hypergeometric model given row totals and column total. I n11 ∼ Hypergeometric(n1· , n2· , n·1 ) I Test comparing two proportions: H0 : π1 = π2 , H1 : π1 , 6= π2 I Small sample: Fisher’s Exact Test. I Large sample (all cell counts ≥ 10 and n1· p1 (1 − p1 ) ≥ 10 and n2· p2 (1 − p2 ) ≥ 10): Z test for two proportions. I Large sample Z-score based CI for (π1 − π2 ). Wensong Wu Biostatistics Midterm Review Two Variables, r × c Table I χ2 test for association: I H0 : Row variable and column variable are independent (not associated), H1 : Row variable and column variable are dependent (associated). (ni· )(n·j ) I Expected: E (nij ) =. n·· I DF = (r − 1)(c − 1). I Require large sample. Wensong Wu Biostatistics Midterm Review STA 6176 Biostatistics Topic 8 Chi-Square Test for Association Instructor: Wensong Wu Wensong Wu Biostatistics Topic 8 Categorical Data Analysis I Starting from this topic, we will cover Chapter 7 Categorical Data in the textbook. I We study the relationship of two categorical variables. I Each variable may have two or more categories (levels). I Example: Smoking status vs cancer status, treatment groups vs health endpoints. I We count the number of occurrences under each pair of conditions and enter them in a table, called (two- way) contingency table. I This is a generalization of a 2 × 2 table. Wensong Wu Biostatistics Topic 8 Two-Way Contingency Table In general, we have a r × c contingency table. I r = the number of rows. I c = the number of columns. I i = index of row levels. i = 1, 2,..., r. I j = index of column levels. j = 1, 2,..., c. I nij = the number of occurrences in the ith row level and jth column level. j 1 2 C 1 n11 n1 2 n1c 2 n 21 n22 n2c r n,., n,2 n,.c ◄ □ ► ◄ ol ► ◄ - ► ◄ = ► ~ +)Cl_(" Wensong Wu Biostatistics Topic 8 Two-Way Contingency Table Example 7.1. Gastric freezing (F): A balloon was lowered into a subject’s stomach, and coolant at a temperature of −17 to −20C was introduced through tubing connected to the balloon. It was thought that a duodenal ulcer might heal. An elaborated sham procedure (control, S) simulates gastric freezing: The tube entering the patient’s mouth was cooled to the same temperature as in the actual procedure, but the coolant entering the stomach was at room temperature, so that no freezing took place. Random allocations to treatment and sham were balanced. At the termination of the study, patients were classified by the causes of endpoints as in the following contingency table. With With With No t Reaching Gro up Patients H emorrhage Operation Hospitali zati on Endpoint F (freeze) 69 9 17 9 34 S (sham) 68 9 14 7 38 ◄ □ ► ◄ ol ► ◄ - ► ◄ = ► ~ +)Cl_(" Wensong Wu Biostatistics Topic 8 Two-Way Contingency Table Example 7.1. With With With Not Reaching Gro up Patients Hemorrhage Operation H ospitali zation Endpoint F (fr eeze) 69 9 17 9 34 S (sham) 68 9 14 7 38 I r =?c =? nij =? I Research question: Any difference between the treatment and control? I Equivalently, any association between the treatments and the causes of endpoints? Wensong Wu Biostatistics Topic 8 Probability Model in Two-Way Contingency Table I A sample of units is taken from the population. I On each unit we observe the values of two categorical variables (the row variable and the column variable). I πij = the probability that the row variable takes on level i and the column variable takes on level j. Pc P r I j=1 πij = 1 P i=1 I πi· = j πij =P(Row=level i) P I π·j = i πij =P(Column=level j) I Think of nij as a random variable conditioning on the row total ni· , the column total n·j , and the grand total n·· I nij ∼ Bin(n·· , πij ), so E (nij ) = n·· πij , or πij = E (nij )/n··. I πi· = ni· /n·· , π·j = n·j /n·· Wensong Wu Biostatistics Topic 8 Chi-Square Test for Association I Hypotheses: I H0 : No association between the Row and Column variables. I H1: There is an association between the Row and Column variables. I Independence of two events A and B ⇐⇒ P(A ∩ B) = P(A)P(B). I No association between Row and Column variables ⇐⇒ P(Row=i and Column=j) = P(Row=i)P(Column=j) I Equivalent hypotheses in probabilities: I H0 : πij = (πi·)(π·j ) I 6 (πi· )(π·j ) H1 : πij = I If 2 × 2 table, H0 means no difference in probabilities of successes between two rows. I Small samples: Fisher’s Exact test. I Large samples: Z test for two proportions. Wensong Wu Biostatistics Topic 8 Chi-Square Test for Association I Under H0 of no association, πij = (πi· )(π·j ) implies E (nij ) ni· n·j = n·· n·· n·· I So the expected count of occurrence at ith row and jth column, given ni· , n·j , and n·· is (ni· )(n·j ) E (nij ) = n·· I Form test statistic similarly to the Goodness-of-Fit test: c X r 2 X (Observed − Expected)2 χ = Expected j=1 i=1 (ni· )(n·j ) 2 c X r ni − X n·· = ∼ χ2with df = (r − 1)(c − 1) (ni· )(n·j ) j=1 i=1 n·· Wensong Wu Biostatistics Topic 8 Chi-Square Test for Association Example. Any association between the treatments and the causes of endpoints? With With With Not Reaching Group Patients Hemorrhage Operation Hospitalization Endpoint F (freeze) 69 9 17 9 34 S (sham) 68 9 14 7 38 I Hypotheses: I Expected counts: I Test statistic: I DF= I P-value= I Conclusion: Wensong Wu Biostatistics Topic 8 STA 6176 Biostatistics Topic 7 Goodness-of-Fit Test Instructor: Wensong Wu Wensong Wu Biostatistics Topic 7 Goodness of Fit I Anyone could fit data with the most appropriate model in her mind. I How to examine the data to see if the applied model seems to fit the data? I This is a very general question. The hypothesis tests that are designed to answer it are all called the “goodness-of-fit tests”. I We will only focus on the count data in the multinomial model. Wensong Wu Biostatistics Topic 7 Multinomial Model I An extension of “Binomial” model. I Trials with k outcomes (k ≥ 2). I P(outcome i)=πi , i = 1, 2,..., k. I n independent and identical such trails. I Ni = the number of outcome i has a multinomial distribution. I Notation: Ni ∼ Multinomial(n, πi ) I Mean: E (Ni ) = nπ. Wensong Wu Biostatistics Topic 7 Multinomial Model Example I Denote A=dominant gene. a=recessive gene. I Two parents are Aa. The offsprings may be of genotype AA, Aa, or aa with the following probabilities. Genotype AA Aa aa Expected frequency ratio 1 2 1 Probability 1/4 1/2 1/4 I Consider a sample of 639 offsprings. Multinomial model: I N1 = the number of AA ∼ Multinomial(639, 1/4). I N2 = the number of Aa ∼ Multinomial(639, 1/2). I N3 = the number of aa ∼ Multinomial(639, 1/4). I Means (expected values) are I E (N1 ) = I E (N2 ) = I E (N3 ) = Wensong Wu Biostatistics Topic 7 Multinomial Model Example I Now in an experiment, we observe Genotype AA Aa aa Total Counts 159 321 159 639 I How do we measure the agreement between the data and the model? Wensong Wu Biostatistics Topic 7 Chi-Square Test for Goodness of Fit I Hypotheses: H0 : πi = πi0 , i = 1, 2,..., k. H1 : πi 6= πi0 , i = 1, 2,..., k. I Test Statistic: k k X (Observedi − Expectedi )2 X (Ni − nπi0 )2 χ2 = = Expectedi nπi0 i=1 i=1 I Sampling distribution under H0 : χ2 follows a Chi-Square distribution with degrees of freedom (k − 1). Wensong Wu Biostatistics Topic 7 Chi-Square Test for Goodness of Fit I Test Statistic: χ2 ∼ χk2 −1 I Reject H0 when χ2 statistic has a big value. Draw a graph! I P-value=Pr (Y ≥ χ2 ), where Y ∼ χ2k−1. Wensong Wu Biostatistics Topic 7 Chi-Square Test for Goodness of Fit I Back to the example, we put the counts (observed) and the means (expected) together Genotype AA Aa aa Total Observed 159 321 159 639 Expected 159.75 319.5 159.75 639 I Hypotheses: I Test Statistic: I DF= Wensong Wu Biostatistics Topic 7 Chi-Square Test for Goodness of Fit I Back to the example, we put the counts (observed) and the means (expected) together Genotype AA Aa aa Total Observed 159 321 159 639 Expected 159.75 319.5 159.75 639 I In R, Pr (Y ≤ x) is calculated by pchisq(x,df). I P-value= I Decision: I Interpretation: The data shows no evidence that the hypothesized probabilities 1/4, 1/2, 1/4 are wrong. Wensong Wu Biostatistics Topic 7 STA 6176 Biostatistics Topic 6 Poisson Model Instructor: Wensong Wu Wensong Wu Biostatistics Topic 6 Poisson Model Two major uses of Poisson Model: I Model the counts of events in space or time. I Assumption: The number of events occurring in one part of the continuum (of space or time) should be independent of that in another part of the continuum. I Example: The number of arrivals in an emergency room. I Approximate Binomial Model when n is large and π is small. I Assumption: n ≥ 20 and π ≤ 0.1. I Example: The number of a rare disease in a sample of people. We will focus on the second use. Wensong Wu Biostatistics Topic 6 Poisson Distribution I Let Y be a RV with the Poisson distribution with parameter λ. I Probability mass function: e −λ λk P(Y = k) = , k = 0, 1, 2,... k! Wensong Wu Biostatistics Topic 6 Poisson Parameter Estimate I Let Y be a RV with the Poisson distribution with parameter λ. I Mean and Variance of Y: E (Y ) = Var (Y ) = λ I An unbiased estimate of λ is Y. I When the observed y ≥ 5, an approximate 95% confidence interval of λ is: √ √ (( Y − 1)2 , ( Y + 1)2 ) Wensong Wu Biostatistics Topic 6 Poisson Approximation to Binomial I Poisson and Binomial distributions Bin(n, π) are very close when n is large and π is small. I In using the Poisson distribution to approximation the Binomial distribution, we equate the means: λ = nπ I The CI for λ is an approximate CI for nπ, so the CI for λ divided by n becomes an approximate CI for π. I Let X ∼ Bin(n, π). When n ≥ 20, π ≤ 0.1, and observed x ≥ 5, an approximate 95% confidence interval of π is √ √ ! ( X − 1)2 ( X + 1)2 , n n Wensong Wu Biostatistics Topic 6 Poisson Approximation to Binomial Example: Among 3584 black infants 43 were born with ABO hemolytic disease. Find a 95% confidence interval for the probability that a new born infant has this disease. I Let Y = the number of disease cases. Y ∼ Bin(n = 3584, π). I Can we use the large sample Normal approximation? I Find the CI using the large sample formula. Wensong Wu Biostatistics Topic 6 Poisson Approximation to Binomial Example: Among 3584 black infants 43 were born with ABO hemolytic disease. Find a 95% confidence interval for the probability that a new born infant has this disease. I Let Y = the number of disease cases. Y ∼ Bin(n = 3584, π). I Is it approximate to use Poisson approximation to Binomial? I Find the CI using the Poisson approximation formula. Wensong Wu Biostatistics Topic 6 STA 6176 Biostatistics Topic 5 Comparing Two Proportions Instructor: Wensong Wu Wensong Wu Biostatistics Topic 5 Two-Proportion Problem I Goal: To compare proportions of successes in two samples from two populations. I Data is summarized in a 2 × 2 table. Success Failure Row Total Sample 1 n11 n12 n1· Sample 2 n21 n22 n2· Column Total n·1 n·2 n·· I π1 =Proportion of success in population 1. I π2 =Proportion of success in population 2. Wensong Wu Biostatistics Topic 5 Two-Proportion Problem Example: In the nut allergy study, among 1366 children whose mothers consumed at least 5 servings of nuts per week, 17 children had nut allergy; and among 6842 mothers who consumed less than 5 servings of nuts per week, 129 children had nut allergy. Nut Allergy No Nut Allergy Row Total ≥ 5/week 17 1349 1366 < 5/week 129 6713 6842 Column Total 146 8062 8208 I Sample 1? Sample 2? I Population 1? Population 2? I “Success”? “Failure”? I π1 = I π2 = Wensong Wu Biostatistics Topic 5 Two-Proportion Problem Example: In the nut allergy study, among 1366 children whose mothers consumed at least 5 servings of nuts per week during pregnancy, 17 children had nut allergy; and among 6842 mothers who consumed less than 5 servings of nuts per week during pregnancy, 129 children had nut allergy. Nut Allergy No Nut Allergy Row Total ≥ 5/week 17 1349 1366 < 5/week 129 6713 6842 Column Total 146 8062 8208 I Sample 1? Sample 2? I Population 1? Population 2? I “Success”? “Failure”? I π1 =Prop. of allergy among all children whose moms ≥ 5/wk. I π2 =Prop. of allergy among all children whose moms < 5/wk. Wensong Wu Biostatistics Topic 5 Large Sample Inference I Target parameter is (π1 − π2 ). I We already know the distributions of the counts: I n11 ∼ Bin(n1· , π1 ), where π1 is estimated by p1 = n11 /n1· I n21 ∼ Bin(n2· , π2 ), where π2 is estimated by p2 = n21 /n2· I n11 and n21 are independent. I Naturally, (π1 − π2 ) is estimated by p1 − p2 , and we can calculate the mean and variance of this estimator (as an RV). I E (p1 − p2 ) = (π1 − π2 ) π1 (1 − π1 ) π2 (1 − π2 ) I Var (p1 − p2 ) = + n1· n2· I By CLT, when n1· and n2· are large, p1 − p2 is approximately Normal, and after standardization and approximation, (p1 − p2 ) − (π1 − π2 ) r ∼ N(0, 1) p1 (1 − p1 ) p2 (1 − p2 ) + n1· n2· Wensong Wu Biostatistics Topic 5 Large Sample Z Test I Conditions of large samples: I All cell counts n11 , n12 , n21 , n22 are all ≥ 10. I n1· p1 (1 − p1 ) ≥ 10 and n2· p2 (1 − p2 ) ≥ 10 I Consider testing H0 : π1 = π2 vs H1 : π1 6= π2 (two sided) (p1 − p2 ) I Test statistic: Z = r p1 (1 − p1 ) p2 (1 − p2 ) + n1· n2· I Sampling distribution under H0 : N(0,1). I α-level approach (same as all Z test): I Find standard normal quantile z1−α/2. I Calculate the value of the test statistic z. I Reject H0 if |z| ≥ z1−α/2. Wensong Wu Biostatistics Topic 5 Large Sample Z Test I Conditions of large samples: I All cell counts n11 , n12 , n21 , n22 are all ≥ 10. I n1· p1 (1 − p1 ) ≥ 10 and n2· p2 (1 − p2 ) ≥ 10 I Consider testing H0 : π1 = π2 vs H1 : π1 6= π2 (two sided) (p1 − p2 ) I Test statistic: Z = r p1 (1 − p1 ) p2 (1 − p2 ) + n1· n2· I Sampling distribution under H0 : N(0,1). I P-value approach (same as all Z test): I Calculate the value of the test statistic z. I P-value = Pr (|Z | ≥ |z|)), where Z ∼ N(0, 1). Wensong Wu Biostatistics Topic 5 Large Sample Z Test Example: In the nut allergy study, are the proportions of children’s nut allergy equal between the two categories of mothers’ nut consumption during pregnancy? Nut Allergy No Nut Allergy Row Total ≥ 5/week 17 1349 1366 < 5/week 129 6713 6842 Column Total 146 8062 8208 I Hypotheses: I Large sample? I Value of test statistic: I α-level approach: Wensong Wu Biostatistics Topic 5 Large Sample Z Test Example: In the nut allergy study, are the proportions of children’s nut allergy equal between the two categories of mothers’ nut consumption during pregnancy? Nut Allergy No Nut Allergy Row Total ≥ 5/week 17 1349 1366 < 5/week 129 6713 6842 Column Total 146 8062 8208 I Hypotheses: I Large sample? I Value of test statistic: I P-value approach: Wensong Wu Biostatistics Topic 5 Large Sample Z Test Example: Is the proportion of children’s nut allergy for those whose mother consumed ≥ 5/week nuts during pregnancy lower than that for those who consumed < 5/week nuts? Nut Allergy No Nut Allergy Row Total ≥ 5/week 17 1349 1366 < 5/week 129 6713 6842 Column Total 146 8062 8208 I Hypotheses: I Large sample? I Value of test statistic: I (One sided) P-value: Wensong Wu Biostatistics Topic 5 Large Sample Confidence Interval I Same conditions for large samples. I (1 − α) confidence interval of the difference between two proportions is: s p1 (1 − p1 ) p2 (1 − p2 ) (p1 − p2 ) ± z1−α/2 + n1· n2· I Example: In the nut allergy study, find the 95% confidence interval of the difference between the two proportions. I Interpret this interval. Which proportion is higher? Higher by how much? Wensong Wu Biostatistics Topic 5 Small Sample Test Comparing Proportions Example: Compare surgical mortality rate between emergency and non-emergency cases. Dead Alive Row Total Emergency 1 19 20 Other 7 369 376 Column Total 8 388 396 I Sample 1? Sample 2? Wensong Wu Biostatistics Topic 5 Small Sample Test Comparing Proportions Example: Compare surgical mortality rate between emergency and non-emergency cases. Dead Alive Row Total Emergency 1 19 20 Other 7 369 376 Column Total 8 388 396 I Sample 1? Sample 2? I Population 1? Population 2? Wensong Wu Biostatistics Topic 5 Small Sample Test Comparing Proportions Example: Compare surgical mortality rate between emergency and non-emergency cases. Dead Alive Row Total Emergency 1 19 20 Other 7 369 376 Column Total 8 388 396 I Sample 1? Sample 2? I Population 1? Population 2? I “Success”? “Failure”? Wensong Wu Biostatistics Topic 5 Small Sample Test Comparing Proportions Example: Compare surgical mortality rate between emergency and non-emergency cases. Dead Alive Row Total Emergency 1 19 20 Other 7 369 376 Column Total 8 388 396 I Sample 1? Sample 2? I Population 1? Population 2? I “Success”? “Failure”? I π1 = Wensong Wu Biostatistics Topic 5 Small Sample Test Comparing Proportions Example: Compare surgical mortality rate between emergency and non-emergency cases. Dead Alive Row Total Emergency 1 19 20 Other 7 369 376 Column Total 8 388 396 I Sample 1? Sample 2? I Population 1? Population 2? I “Success”? “Failure”? I π1 = I π2 = Wensong Wu Biostatistics Topic 5 Small Sample Test Comparing Proportions Example: Compare surgical mortality rate between emergency and non-emergency cases. Dead Alive Row Total Emergency 1 19 20 Other 7 369 376 Column Total 8 388 396 I Sample 1? Sample 2? I Population 1? Population 2? I “Success”? “Failure”? I π1 = I π2 = I Large sample? Wensong Wu Biostatistics Topic 5 Small Sample Test Comparing Proportions Example: Want to know if emergency cases have a higher surgical mortality rate. Dead Alive Row Total Emergency 1 19 20 Other 7 369 376 Column Total 8 388 396 I Hypotheses: Wensong Wu Biostatistics Topic 5 Small Sample Test Comparing Proportions Example: Want to know if emergency cases have a higher surgical mortality rate. Dead Alive Row Total Emergency 1 19 20 Other 7 369 376 Column Total 8 388 396 I Hypotheses: I Test statistic: Consider N11 = the number of successes in sample 1 (as a RV), conditioning on n1· , n2· (row totals), and n·1 (column total of sucesses). Wensong Wu Biostatistics Topic 5 Small Sample Test Comparing Proportions Example: Want to know if emergency cases have a higher surgical mortality rate. Dead Alive Row Total Emergency 1 19 20 Other 7 369 376 Column Total 8 388 396 I Hypotheses: I Test statistic: Consider N11 = the number of successes in sample 1 (as a RV), conditioning on n1· , n2· (row totals), and n·1 (column total of sucesses). I Sampling distribution under H0 : N11 has a hypergeometric distribution. Denote N11 ∼ hyper (n1· , n2· , n·1 ). Wensong Wu Biostatistics Topic 5 Small Sample Test Comparing Proportions Example: Want to know if emergency cases have a higher surgical mortality rate. Dead Alive Row Total Emergency 1 19 20 Other 7 369 376 Column Total 8 388 396 I Hypotheses: I Test statistic: Consider N11 = the number of successes in sample 1 (as a RV), conditioning on n1· , n2· (row totals), and n·1 (column total of sucesses). I Sampling distribution under H0 : N11 has a hypergeometric distribution. Denote N11 ∼ hyper (n1· , n2· , n·1 ). I P-value=Pr(Observe 1 or more deadly emergency surgery, conditional on 8 total death ) Wensong Wu Biostatistics Topic 5 Hypergeometric Distribution I A bowl contains n1· orange balls (sample 1) and n2· green balls (sample 2). I Select n·1 (total successes). I N11 is the number of orange balls among the selected. n1· n2· k n·1 − k I P(N11 = k) = n·· n·1 I In R, the pmf is calculated by dhyper. I Why is N11 not Binomial? Wensong Wu Biostatistics Topic 5 Fisher’s Exact Test I Hypotheses: H0 : π1 = π2 , H1 : π1 >, = 2) Wensong Wu Biostatistics Topic 4 Binomial Distribution I In R, calculate pmf by dbinom(k, n, π). Wensong Wu Biostatistics Topic 4 Binomial Distribution I In R, calculate pmf by dbinom(k, n, π). I P(Y = 0) = dbinom(0, 8, 0.5) = 0.00390625 Wensong Wu Biostatistics Topic 4 Binomial Distribution I In R, calculate pmf by dbinom(k, n, π). I P(Y = 0) = dbinom(0, 8, 0.5) = 0.00390625 I P(Y = 1) = dbinom(1, 8, 0.5) = 0.03125 Wensong Wu Biostatistics Topic 4 Binomial Distribution I In R, calculate pmf by dbinom(k, n, π). I P(Y = 0) = dbinom(0, 8, 0.5) = 0.00390625 I P(Y = 1) = dbinom(1, 8, 0.5) = 0.03125 I P(Y >= 2) = 1 − dbinom(0, 8, 0.5) − dbinom(1, 8, 0.5) = 0.9648438 Wensong Wu Biostatistics Topic 4 Binomial Mean and Variance I The mean and variance of Y ∼ Bin(n, π) are E (Y ) = nπ Var (Y ) = nπ(1 − π) I Example: What is the mean number of boys in a 8-child family? Variance? Standard deviation? Wensong Wu Biostatistics Topic 4 Point Estimator of Binomial Proportion I Usually in a Binomial model n is fixed and known, but π, the population proportion of success, is unknown. Wensong Wu Biostatistics Topic 4 Point Estimator of Binomial Proportion I Usually in a Binomial model n is fixed and known, but π, the population proportion of success, is unknown. I We collect data from a binomial experiment and want to make inference on π. Wensong Wu Biostatistics Topic 4 Point Estimator of Binomial Proportion I Usually in a Binomial model n is fixed and known, but π, the population proportion of success, is unknown. I We collect data from a binomial experiment and want to make inference on π. Y I E (Y ) = nπ, so E ( ) = π. n Wensong Wu Biostatistics Topic 4 Point Estimator of Binomial Proportion I Usually in a Binomial model n is fixed and known, but π, the population proportion of success, is unknown. I We collect data from a binomial experiment and want to make inference on π. Y I E (Y ) = nπ, so E ( ) = π. n Y I This means = Sample proportion of successes is an n unbiased estimator of π, the population proportion. Wensong Wu Biostatistics Topic 4 Point Estimator of Binomial Proportion I Usually in a Binomial model n is fixed and known, but π, the population proportion of success, is unknown. I We collect data from a binomial experiment and want to make inference on π. Y I E (Y ) = nπ, so E ( ) = π. n Y I This means = Sample proportion of successes is an n unbiased estimator of π, the population proportion. I Example: In the nut allergy study, 1366 mothers had at least 5 servings of nuts per week during pregnancy among 8205 mothers. What is an unbiased estimate of the proportion of mothers who had nuts at least 5 servings of nuts per week during pregnancy among all mothers? Wensong Wu Biostatistics Topic 4 Hypothesis Test for Binomial Proportion I Let’s construct a hypothesis test for π. Start with a two-sided alternative. I Hypotheses: H0 : π = π0 vs H1 : π 6= π0. I Test statistic: Y =the number of successes. I Sampling distribution of the test statistics assuming H0 is true: Y ∼ Bin(n, π0 ). Draw a graph! Notice the mean is nπ0. Wensong Wu Biostatistics Topic 4 Hypothesis Test for Binomial Proportion α-level approach: I Find the smallest c such that Pr (|Y − nπ0 | ≥ c) = Pr (Y ≤ nπ0 − c or Y ≥ nπ0 + c) ≤ α I Observe y from a data. Reject H0 if |y − nπ0 | ≥ c. Wensong Wu Biostatistics Topic 4 Hypothesis Test for Binomial Proportion P-value approach: I Observe y from a data. I Calculate c = |y − nπ0 |. I P-value = Pr (|Y − nπ0 | ≥ c) = Pr (Y ≤ nπ0 − c) + Pr (Y ≥ nπ0 + c) Wensong Wu Biostatistics Topic 4 Hypothesis Test for Binomial Proportion Example: In a 8-child family, there are 6 boys. Suppose this is a binomial experiment. Are the probabilities of having a boy and having a girl equal in this family? Use α = 0.10. I Denote π=probability of having a boy in this family. Wensong Wu Biostatistics Topic 4 Hypothesis Test for Binomial Proportion Example: In a 8-child family, there are 6 boys. Suppose this is a binomial experiment. Are the probabilities of having a boy and having a girl equal in this family? Use α = 0.10. I Denote π=probability of having a boy in this family. I Hypotheses: Wensong Wu Biostatistics Topic 4 Hypothesis Test for Binomial Proportion Example: In a 8-child family, there are 6 boys. Suppose this is a binomial experiment. Are the probabilities of having a boy and having a girl equal in this family? Use α = 0.10. I Denote π=probability of having a boy in this family. I Hypotheses: I Test statistic: Wensong Wu Biostatistics Topic 4 Hypothesis Test for Binomial Proportion Example: In a 8-child family, there are 6 boys. Suppose this is a binomial experiment. Are the probabilities of having a boy and having a girl equal in this family? Use α = 0.10. I Denote π=probability of having a boy in this family. I Hypotheses: I Test statistic: I Sampling distribution under H0 : Wensong Wu Biostatistics Topic 4 Hypothesis Test for Binomial Proportion Example: In a 8-child family, there are 6 boys. Suppose this is a binomial experiment. Are the probabilities of having a boy and having a girl equal in this family? Use α = 0.10. I Denote π=probability of having a boy in this family. I Hypotheses: I Test statistic: I Sampling distribution under H0 : I Observed value of test statistic: Wensong Wu Biostatistics Topic 4 Hypothesis Test for Binomial Proportion Example: In a 8-child family, there are 6 boys. Suppose this is a binomial experiment. Are the probabilities of having a boy and having a girl equal in this family? Use α = 0.10. I Denote π=probability of having a boy in this family. I Hypotheses: I Test statistic: I Sampling distribution under H0 : I Observed value of test statistic: I α-level approach: Wensong Wu Biostatistics Topic 4 Hypothesis Test for Binomial Proportion Example: In a 8-child family, there are 6 boys. Suppose this is a binomial experiment. Are the probabilities of having a boy and having a girl equal in this family? Use α = 0.10. I Denote π=probability of having a boy in this family. I Hypotheses: I Test statistic: I Sampling distribution under H0 : I Observed value of test statistic: I P-value approach: Wensong Wu Biostatistics Topic 4 Hypothesis Test for Binomial Proportion Example: In a 8-child family, there are 6 boys. Suppose this is a binomial experiment. Are the probabilities of having a boy and having a girl equal in this family? Use α = 0.10. I Denote π=probability of having a boy in this family. I Hypotheses: I Test statistic: I Sampling distribution under H0 : I Observed value of test statistic: I Conclusion and interpretation: Wensong Wu Biostatistics Topic 4 Hypothesis Test for Binomial Proportion Example: In a 8-child family, there are 6 boys. Suppose this is a binomial experiment. Is the probability of having a boy greater than having a girl in this family? Use α = 0.10. I Denote π=probability of having a boy in this family. I Hypotheses: I Test statistic: I Sampling distribution under H0 : I Observed value of test statistic: I One-sided p-value: Wensong Wu Biostatistics Topic 4 Binomial for Large n I What happens if n is large for Y ∼ Bin(n, π)? I Write Y = X1 + X2 +... + Xn , where Xi s are independent Bernoulli RV. Xi = 0 with prob π and Xi = 1 with prob 1 − π. I Then Y /n = (X1 + X2 +... + Xn )/n = X , or the sample mean of Xi ’s! I Central Limit Theorem: Y /n has an approximately Normal distribution when n is large, so is Y. I Y ∼ Bin(n, π) is approx. N(nπ, nπ(1 − π)) for large n. Y − nπ I Z=p ∼ N(0, 1) approx. for large n. (nπ(1 − π) Wensong Wu Biostatistics Topic 4 Large Sample Test for Binomial Proportion I Hypotheses: H0 : π = π0 vs H1 : π 6= π0. (Two-sided) Y − nπ0 I Test statistic: For large n, use Z = p (nπ0 (1 − π0 ) I Sampling distribution of the test statistics assuming H0 is true: Z ∼ N(0, 1) approx. Draw a graph! Wensong Wu Biostatistics Topic 4 Large Sample Test for Binomial Proportion α-level approach: I Find standard normal quantile z1−α/2. In R, qnorm(1-α/2). I Observe y and calculate z. Reject H0 if |z| ≥ z1−α/2. Wensong Wu Biostatistics Topic 4 Large Sample Test for Binomial Proportion P-value approach: I Observe y and and calculate z. I P-value = Pr (|Z | ≥ |z|)), where Z ∼ N(0, 1). In R, pnorm(z) calculates Pr (Z < z). Wensong Wu Biostatistics Topic 4 Continuity Correction When n is moderately large, Y may still be discrete and not very continuous, so a continuity correction of the test statistic is needed: Y − nπ0 − 1/2 1 p , if Y − nπ0 > (nπ0 (1 − π0 ) 2 Y − nπ0 1 Zc = p , if |Y − nπ0 | ≤ (nπ0 (1 − π0 ) 2 Y − nπ + 1/2 1 0 , if Y − nπ0 < − p (nπ0 (1 − π0 ) 2 How large n is considered large or moderately large? Criteria Sample Size Statistics Sampling Dist. n ≤ 50 Small Y Binomial nπ0 (1 − π0 ) ≥ 10 Moderately Large Zc Approx. Normal nπ0 (1 − π0 ) ≥ 100 Large Z Approx. Normal Wensong Wu Biostatistics Topic 4 Large Sample Test for Binomial Proportion Example: Among 1366 children whose mothers had more than 5 serving of nuts per week, 17 children had nut allergy. Is this probability different from the population prevalence of child’s nuts allergy equal to 1.8%? Use α = 0.05. I Denote π=probability of nuts allergy among children whose mothers had more than 5 serving of nuts per week. Wensong Wu Biostatistics Topic 4 Large Sample Test for Binomial Proportion Example: Among 1366 children whose mothers had more than 5 serving of nuts per week, 17 children had nut allergy. Is this probability different from the population prevalence of child’s nuts allergy equal to 1.8%? Use α = 0.05. I Denote π=probability of nuts allergy among children whose mothers had more than 5 serving of nuts per week. I Hypotheses: Wensong Wu Biostatistics Topic 4 Large Sample Test for Binomial Proportion Example: Among 1366 children whose mothers had more than 5 serving of nuts per week, 17 children had nut allergy. Is this probability different from the population prevalence of child’s nuts allergy equal to 1.8%? Use α = 0.05. I Denote π=probability of nuts allergy among children whose mothers had more than 5 serving of nuts per week. I Hypotheses: I Large sample? Wensong Wu Biostatistics Topic 4 Large Sample Test for Binomial Proportion Example: Among 1366 children whose mothers had more than 5 serving of nuts per week, 17 children had nut allergy. Is this probability different from the population prevalence of child’s nuts allergy equal to 1.8%? Use α = 0.05. I Denote π=probability of nuts allergy among children whose mothers had more than 5 serving of nuts per week. I Hypotheses: I Large sample? I Value of test statistic: Wensong Wu Biostatistics Topic 4 Large Sample Test for Binomial Proportion Example: Among 1366 children whose mothers had more than 5 serving of nuts per week, 17 children had nut allergy. Is this probability different from the population prevalence of child’s nuts allergy equal to 1.8%? Use α = 0.05. I Denote π=probability of nuts allergy among children whose mothers had more than 5 serving of nuts per week. I Hypotheses: I Large sample? I Value of test statistic: I α-level approach: Wensong Wu Biostatistics Topic 4 Large Sample Test for Binomial Proportion Example: Among 1366 children whose mothers had more than 5 serving of nuts per week, 17 children had nut allergy. Is this probability different from the population prevalence of child’s nuts allergy equal to 1.8%? Use α = 0.05. I Denote π=probability of nuts allergy among children whose mothers had more than 5 serving of nuts per week. I Hypotheses: I Large sample? I Value of test statistic: I P-value approach: Wensong Wu Biostatistics Topic 4 Large Sample Test for Binomial Proportion Example: Among 1366 children whose mothers had more than 5 serving of nuts per week, 17 children had nut allergy. Is this probability different from the population prevalence of child’s nuts allergy equal to 1.8%? Use α = 0.05. I Denote π=probability of nuts allergy among children whose mothers had more than 5 serving of nuts per week. I Hypotheses: I Large sample? I Value of test statistic: I Conclusion and interpretation: Wensong Wu Biostatistics Topic 4 Large Sample Confidence Interval for π Y Y − nπ p̂ − π p̂ − π I Let p̂ =. Z=p =q ≈q n (nπ(1 − π) π(1−π) p̂(1−p̂) n n Wensong Wu Biostatistics Topic 4 Large Sample Confidence Interval for π Y Y − nπ p̂ − π p̂ − π I Let p̂ =. Z=p =q ≈q n (nπ(1 − π) π(1−π) p̂(1−p̂) n n I For large sample, an approximate (1 − α) confidence interval of π is r p̂(1 − p̂) p̂ ± z1−α/2 n Wensong Wu Biostatistics Topic 4 Large Sample Confidence Interval for π Y Y − nπ p̂ − π p̂ − π I Let p̂ =. Z=p =q ≈q n (nπ(1 − π) π(1−π) p̂(1−p̂) n n I For large sample, an approximate (1 − α) confidence interval of π is r p̂(1 − p̂) p̂ ± z1−α/2 n Wensong Wu Biostatistics Topic 4 Large Sample Confidence Interval for π Y Y − nπ p̂ − π p̂ − π I Let p̂ =. Z=p =q ≈q n (nπ(1 − π) π(1−π) p̂(1−p̂) n n I For large sample, an approximate (1 − α) confidence interval of π is r p̂(1 − p̂) p̂ ± z1−α/2 n Example: Among 1366 children whose mothers had more than 5 serving of nuts per week, 17 children had nut allergy. Find and interpret a 95% confidence interval of the population proportion of nuts allergy among children whose mothers had more than 5 serving of nuts per week. Wensong Wu Biostatistics Topic 4 Summary of Binomial Model Topics covered: I Binomial experiment and Binomial random variable. I Binomial distribution, probabilities, mean, and variance. I Small sample test for π. I Large sample test for π. I Continuity correction for moderately large sample. I Large sample confidence interval of π Wensong Wu Biostatistics Topic 4 STA 6176 Biostatistics Topic 3: Review of Inferential Statistics Instructor: Wensong Wu Wensong Wu Biostatistics Topic 3 Sampling Distribution I Any sample statistics is a random variable. The value varies from one sample to another. I The probability distribution of a sample statistic (as a RV) is called Sampling Distribution. I We can apply probability theory to investigate the sampling distribution, and it will help us eventually infer the population parameter based on a sample. Example: Y1, Y2,... , Yn are iid from a population with a population mean µ. I µ is our target parameter. Sample mean Y = ( ni=1 Yi )/n is a point estimator of µ. P I I What is the sampling distribution of Y ? Wensong Wu Biostatistics Topic 3 Sampling Distribution Case A Case A: Let’s assume that I Population distribution is the Normal distribution. I Population variance σ2 is known. What is the sampling distribution of Y ? Wensong Wu Biostatistics Topic 3 Sampling Distribution Case A Case A: Let’s assume that I Population distribution is the Normal distribution. I Population variance σ2 is known. What is the sampling distribution of Y ? I E (Y ) = µ, the mean of Y is the population mean. Wensong Wu Biostatistics Topic 3 Sampling Distribution Case A Case A: Let’s assume that I Population distribution is the Normal distribution. I Population variance σ2 is known. What is the sampling distribution of Y ? I E (Y ) = µ, the mean of Y is the population mean. I This means Y is an unbiased estimator of µ. Wensong Wu Biostatistics Topic 3 Sampling Distribution Case A Case A: Let’s assume that I Population distribution is the Normal distribution. I Population variance σ2 is known. What is the sampling distribution of Y ? I E (Y ) = µ, the mean of Y is the population mean. I This means Y is an unbiased estimator of µ. I Var (Y ) = σ 2 /n, i.e. the var of Y is the pop var/sample size. Wensong Wu Biostatistics Topic 3 Sampling Distribution Case A Case A: Let’s assume that I Population distribution is the Normal distribution. I Population variance σ2 is known. What is the sampling distribution of Y ? I E (Y ) = µ, the mean of Y is the population mean. I This means Y is an unbiased estimator of µ. I Var (Y ) = σ 2 /n, i.e. the var of Y is the pop var/sample size. I This means variance of Y decreases in sample size. Wensong Wu Biostatistics Topic 3 Sampling Distribution Case A Case A: Let’s assume that I Population distribution is the Normal distribution. I Population variance σ2 is known. What is the sampling distribution of Y ? I E (Y ) = µ, the mean of Y is the population mean. I This means Y is an unbiased estimator of µ. I Var (Y ) = σ 2 /n, i.e. the var of Y is the pop var/sample size. I This means variance of Y decreases in sample size. I The distribution of Y is still Normal. Wensong Wu Biostatistics Topic 3 Sampling Distribution Case A Case A: Let’s assume that I Population distribution is the Normal distribution. I Population variance σ2 is known. What is the sampling distribution of Y ? I E (Y ) = µ, the mean of Y is the population mean. I This means Y is an unbiased estimator of µ. I Var (Y ) = σ 2 /n, i.e. the var of Y is the pop var/sample size. I This means variance of Y decreases in sample size. I The distribution of Y is still Normal. 2 I In short, Y ∼ N(µ, σn ). Wensong Wu Biostatistics Topic 3 Sampling Distribution Case A Case A: Let’s assume that I Population distribution is the Normal distribution. I Population variance σ2 is known. What is the sampling distribution of Y ? I E (Y ) = µ, the mean of Y is the population mean. I This means Y is an unbiased estimator of µ. I Var (Y ) = σ 2 /n, i.e. the var of Y is the pop var/sample size. I This means variance of Y decreases in sample size. I The distribution of Y is still Normal. 2 I In short, Y ∼ N(µ, σn ). I The standard deviation of the sampling distribution is called √ the Standard Error (SE). The SE of Y is σ/ n. Wensong Wu Biostatistics Topic 3 Sampling Distribution Case A Example: Let X =Your systolic blood pressure during the day. Assume X ∼ N(100, 6). Measure your systolic blood pressure n times and take the average. What is the sampling distribution of the average measurement when n = 2, 4, 9 times? What is the standard error? Wensong Wu Biostatistics Topic 3 Sampling Distribution Case A Example: Let X =Your systolic blood pressure during the day. Assume X ∼ N(100, 6). Measure your systolic blood pressure n times and take the average. What is the sampling distribution of the average measurement when n = 2, 4, 9 times? What is the standard error? 0:, 0 ····· n = 1 (D 0 --- n = 2 --n = 4 N 0 0 0 Wensong Wu Biostatistics Topic 3 Central Limit Theorem Case B I Case A assumes the Normal population. I Case B: Arbitrary population distribution. I Central Limit Theorem (CLT): When n is large enough (rule of thumb n ≥ 30), the sampling distribution of Y is approximately Normal, regardless of population. 1;JL.;:::::::::::: ~ ,~== ::::;..___~, ;~:~o~ 0.0 0.5 1.0 1.5 2.0 2.5 3.0 SampleMean Wensong Wu Biostatistics Topic 3 Central Limit Theorem Case B I Case A assumes the Normal population. I Case B: Arbitrary population distribution. I Central Limit Theorem (CLT): When n is large enough (rule of thumb n ≥ 30), the sampling distribution of Y is approximately Normal, regardless of population. 1;JL.;:::::::::::: ~ ,~== ::::;..___~, ;~:~o~ 0.0 0.5 1.0 1.5 2.0 2.5 3.0 SampleMean I We can make inference in Case A assuming Normal population, and the results in Case B will remain unchanged as long as the sample size is large! Wensong Wu Biostatistics Topic 3 Confidence Interval of µ 2 I Sampling distrubution of Y : Y ∼ N(µ, σn ) X −µ I Standardize: Z = √ ∼ N(0, 1) σ/ n I Let zq denote the qth quantile of a standard normal distribution, that is, Pr (Z < zq ) = q. I Example: z0.975 = 1.96. Always draw a graph! Wensong Wu Biostatistics Topic 3 Confidence Interval of µ 2 I Sampling distrubution of Y : Y ∼ N(µ, σn ) X −µ I Standardize: Z = √ ∼ N(0, 1) σ/ n I Let zq denote the qth quantile of a standard normal distribution, that is, Pr (Z < zq ) = q. I Example: z0.975 = 1.96. Always draw a graph! I With the “outside” area of α = 0.05, the middle area is 1 − 0.05 = Pr (−1.96 ≤ Z ≤ 1.96) Y −µ = Pr (−1.96 ≤ √ ≤ 1.96)...solve for µ σ/ n σ σ = Pr (Y − 1.96 √ ≤ µ ≤ Y + 1.96 √ ) n n Wensong Wu Biostatistics Topic 3 Confidence Interval of µ 2 I Sampling distrubution of Y : Y ∼ N(µ, σn ) X −µ I Standardize: Z = √ ∼ N(0, 1) σ/ n I Let zq denote the qth quantile of a standard normal distribution, that is, Pr (Z < zq ) = q. I Example: z0.975 = 1.96. Always draw a graph! I With the “outside” area of α = 0.05, the middle area is 1 − 0.05 = Pr (−1.96 ≤ Z ≤ 1.96) Y −µ = Pr (−1.96 ≤ √ ≤ 1.96)...solve for µ σ/ n σ σ = Pr (Y − 1.96 √ ≤ µ ≤ Y + 1.96 √ ) n n I In general, pick a small α, we can write σ σ 1 − α = Pr (Y − z1−α/2 √ ≤ µ ≤ Y + z1−α/2 √ ) n n Wensong Wu Biostatistics Topic 3 Confidence Interval of µ σ I (1 − α) confidence interval (CI) of µ is Y ± z1−α/2 √. n σ I When α = 0.05, it becomes the 95% CI of µ : Y ± 1.96 √ n I Alert: The probability calculation under the sampling distribution does not mean “the probability of µ”. Parameter µ is fixed, although unknown. So should not interpret the CI as “with 95% probability the population mean is between the lower limit (LL) and the upper limit (UL). I Interpret like this: We are 95% confident that the population mean is between LL and UL, where the “95% confidence” means if we keep sampling of size n and calculate CI for each sample, about 95% times the interval captures the true value of µ. Wensong Wu Biostatistics Topic 3 Confidence Interval of µ Interpretation Example: The calculated 95% confidence interval of your mean systolic blood pressure in mmHg based on three measurements is (100, 105). How do you interpret it? I Your systolic blood pressure is between 100 and 105. Wensong Wu Biostatistics Topic 3 Confidence Interval of µ Interpretation Example: The calculated 95% confidence interval of your mean systolic blood pressure in mmHg based on three measurements is (100, 105). How do you interpret it? I Your systolic blood pressure is between 100 and 105. I 95% of time your sys. blood pressure is between 100 and 105. Wensong Wu Biostatistics Topic 3 Confidence Interval of µ Interpretation Example: The calculated 95% confidence interval of your mean systolic blood pressure in mmHg based on three measurements is (100, 105). How do you interpret it? I Your systolic blood pressure is between 100 and 105. I 95% of time your sys. blood pressure is between 100 and 105. I The middle 95% of sys. blood pressure is btw 100 and 105. Wensong Wu Biostatistics Topic 3 Confidence Interval of µ Interpretation Example: The calculated 95% confidence interval of your mean systolic blood pressure in mmHg based on three measurements is (100, 105). How do you interpret it? I Your systolic blood pressure is between 100 and 105. I 95% of time your sys. blood pressure is between 100 and 105. I The middle 95% of sys. blood pressure is btw 100 and 105. I The median of your sys. blood pressure is btw 100 and 105. Wensong Wu Biostatistics Topic 3 Confidence Interval of µ Interpretation Example: The calculated 95% confidence interval of your mean systolic blood pressure in mmHg based on three measurements is (100, 105). How do you interpret it? I Your systolic blood pressure is between 100 and 105. I 95% of time your sys. blood pressure is between 100 and 105. I The middle 95% of sys. blood pressure is btw 100 and 105. I The median of your sys. blood pressure is btw 100 and 105. I The mean of the three measurements is between 100 and 105. Wensong Wu Biostatistics Topic 3 Confidence Interval of µ Interpretation Example: The calculated 95% confidence interval of your mean systolic blood pressure in mmHg based on three measurements is (100, 105). How do you interpret it? I Your systolic blood pressure is between 100 and 105. I 95% of time your sys. blood pressure is between 100 and 105. I The middle 95% of sys. blood pressure is btw 100 and 105. I The median of your sys. blood pressure is btw 100 and 105. I The mean of the three measurements is between 100 and 105. I The population mean sys. blood pressure is btw 100 and 105. Wensong Wu Biostatistics Topic 3 Confidence Interval of µ Interpretation Example: The calculated 95% confidence interval of your mean systolic blood pressure in mmHg based on three measurements is (100, 105). How do you interpret it? I Your systolic blood pressure is between 100 and 105. I 95% of time your sys. blood pressure is between 100 and 105. I The middle 95% of sys. blood pressure is btw 100 and 105. I The median of your sys. blood pressure is btw 100 and 105. I The mean of the three measurements is between 100 and 105. I The population mean sys. blood pressure is btw 100 and 105. I There’s a 95% probability that the population mean sys. blood pressure is between 100 and 105. Wensong Wu Biostatistics Topic 3 Confidence Interval of µ Interpretation Example: The calculated 95% confidence interval of your mean systolic blood pressure in mmHg based on three measurements is (100, 105). How do you interpret it? I Your systolic blood pressure is between 100 and 105. I 95% of time your sys. blood pressure is between 100 and 105. I The middle 95% of sys. blood pressure is btw 100 and 105. I The median of your sys. blood pressure is btw 100 and 105. I The mean of the three measurements is between 100 and 105. I The population mean sys. blood pressure is btw 100 and 105. I There’s a 95% probability that the population mean sys. blood pressure is between 100 and 105. I We are 95% confident that that the population mean sys. blood pressure is between 100 and 105. Wensong Wu Biostatistics Topic 3 Confidence Interval of µ Interpretation Example: The calculated 95% confidence interval of your mean systolic blood pressure in mmHg based on three measurements is (100, 105). How do you interpret it? I Your systolic blood pressure is between 100 and 105. I 95% of time your sys. blood pressure is between 100 and 105. I The middle 95% of sys. blood pressure is btw 100 and 105. I The median of your sys. blood pressure is btw 100 and 105. I The mean of the three measurements is between 100 and 105. I The population mean sys. blood pressure is btw 100 and 105. I There’s a 95% probability that the population mean sys. blood pressure is between 100 and 105. I We are 95% confident that that the population mean sys. blood pressure is between 100 and 105. I We are 95% confident that that the population mean systolic blood pressure is between 100 and 105 mmHg. Wensong Wu Biostatistics Topic 3 Confidence Interval of µ Interpretation Example: The calculated 95% confidence interval of your mean systolic blood pressure in mmHg based on three measurements is (100, 105). How do you interpret it? I We are 95% confident that that the population mean systolic blood pressure is between 100 and 105 mmHg. Wensong Wu Biostatistics Topic 3 Confidence Interval of µ Interpretation Example: The calculated 95% confidence interval of your mean systolic blood pressure in mmHg based on three measurements is (100, 105). How do you interpret it? I We are 95% confident that that the population mean systolic blood pressure is between 100 and 105 mmHg. I Can you claim the mean systolic blood pressure is above 95 mmHg? Wensong Wu Biostatistics Topic 3 Confidence Interval of µ Interpretation Example: The calculated 95% confidence interval of your mean systolic blood pressure in mmHg based on three measurements is (100, 105). How do you interpret it? I We are 95% confident that that the population mean systolic blood pressure is between 100 and 105 mmHg. I Can you claim the mean systolic blood pressure is above 95 mmHg? I Can you claim the mean systolic blood pressure is above 101 mmHg? Wensong Wu Biostatistics Topic 3 Hypothesis Test I Goal: Test if a statement about a population parameter is true based on a random sample - one kind of inferential statistics. Wensong Wu Biostatistics Topic 3 Hypothesis Test I Goal: Test if a statement about a population parameter is true based on a random sample - one kind of inferential statistics. I Example: An at-risk high blood pressure has a systolic blood pressure level higher than 120 mmHg. A random sample of four measurements has been taken on Jane to test if her blood pressure level is at risk of high blood pressure. Wensong Wu Biostatistics Topic 3 Hypothesis Test I Goal: Test if a statement about a population parameter is true based on a random sample - one kind of inferential statistics. I Example: An at-risk high blood pressure has a systolic blood pressure level higher than 120 mmHg. A random sample of four measurements has been taken on Jane to test if her blood pressure level is at risk of high blood pressure. I Step 1: Set up a pair of hypotheses. Wensong Wu Biostatistics Topic 3 Hypothesis Test I Goal: Test if a statement about a population parameter is true based on a random sample - one kind of inferential statistics. I Example: An at-risk high blood pressure has a systolic blood pressure level higher than 120 mmHg. A random sample of four measurements has been taken on Jane to test if her blood pressure level is at risk of high blood pressure. I Step 1: Set up a pair of hypotheses. I Null hypothesis H0 : µ = µ0, where µ0 is a fixed hypothesized value of population mean. Wensong Wu Biostatistics Topic 3 Hypothesis Test I Goal: Test if a statement about a population parameter is true based on a random sample - one kind of inferential statistics. I Example: An at-risk high blood pressure has a systolic blood pressure level higher than 120 mmHg. A random sample of four measurements has been taken on Jane to test if her blood pressure level is at risk of high blood pressure. I Step 1: Set up a pair of hypotheses. I Null hypothesis H0 : µ = µ0, where µ0 is a fixed hypothesized value of population mean. I Alternative/Research hypothesis, denoted by H1 or Ha. Wensong Wu Biostatistics Topic 3 Hypothesis Test I Goal: Test if a statement about a population parameter is true based on a random sample - one kind of inferential statistics. I Example: An at-risk high blood pressure has a systolic blood pressure level higher than 120 mmHg. A random sample of four measurements has been taken on Jane to test if her blood pressure level is at risk of high blood pressure. I Step 1: Set up a pair of hypotheses. I Null hypothesis H0 : µ = µ0, where µ0 is a fixed hypothesized value of population mean. I Alternative/Research hypothesis, denoted by H1 or Ha. I H1 : µ 6= µ0 (two-tailed / two-sided), or Wensong Wu Biostatistics Topic 3 Hypothesis Test I Goal: Test if a statement about a population parameter is true based on a random sample - one kind of inferential statistics. I Example: An at-risk high blood pressure has a systolic blood pressure level higher than 120 mmHg. A random sample of four measurements has been taken on Jane to test if her blood pressure level is at risk of high blood pressure. I Step 1: Set up a pair of hypotheses. I Null hypothesis H0 : µ = µ0, where µ0 is a fixed hypothesized value of population mean. I Alternative/Research hypothesis, denoted by H1 or Ha. I H1 : µ 6= µ0 (two-tailed / two-sided), or I H1 : µ µ0. I Focus on right-tailed for the moment. Step 2 Test statistic. Assuming H0 is true, Y −µ Y − µ0 H0 Z= √ = √ ∼ N(0, 1). σ/ n σ/ n Step 3 Draw a graph! Would like to reject H0 and support H1 if sample mean Y is large, so is T. But how large is large? Need a critical value to determine the rejection region... Wensong Wu Biostatistics Topic 3 Build up One Sample Z Test for µ y − µ0 Step 4 Given sample data calculate z = √. Make a conclusion: σ/ n I If z is in RR, reject H0 ⇒ Enough evidence to support H1. (Not enough simply saying enough evidence to reject H0.) I If z is not in RR, do not reject H0 ⇒ Not enough evidence for H1. Warning: It is wrong to claim “enough evidence for H0”. Wensong Wu Biostatistics Topic 3 One Sample Z Test for µ Example: An at-risk high blood pressure has a systolic blood pressure level higher than 120 mmHg. A random sample of four measurements has been taken on Jane to test if her blood pressure level is at risk of high blood pressure, and the results are y1 = 120, y2 = 117, y3 = 122, y4 = 123. The measurement is known to have a standard deviation of σ = 1. I Set up hypotheses. Wensong Wu Biostatistics Topic 3 One Sample Z Test for µ Example: An at-risk high blood pressure has a systolic blood pressure level higher than 120 mmHg. A random sample of four measurements has been taken on Jane to test if her blood pressure level is at risk of high blood pressure, and the results are y1 = 120, y2 = 117, y3 = 122, y4 = 123. The measurement is known to have a standard deviation of σ = 1. I Set up hypotheses. I Find the rejection region at α = 0.05. Wensong Wu Biostatistics Topic 3 One Sample Z Test for µ Example: An at-risk high blood pressure has a systolic blood pressure level higher than 120 mmHg. A random sample of four measurements has been taken on Jane to test if her blood pressure level is at risk of high blood pressure, and the results are y1 = 120, y2 = 117, y3 = 122, y4 = 123. The measurement is known to have a standard deviation of σ = 1. I Set up hypotheses. I Find the rejection region at α = 0.05. I The sample mean is calculated as y = 120.5. Find the value of the test statistic z. Wensong Wu Biostatistics Topic 3 One Sample Z Test for µ Example: An at-risk high blood pressure has a systolic blood pressure level higher than 120 mmHg. A random sample of four measurements has been taken on Jane to test if her blood pressure level is at risk of high blood pressure, and the results are y1 = 120, y2 = 117, y3 = 122, y4 = 123. The measurement is known to have a standard deviation of σ = 1. I Set up hypotheses. I Find the rejection region at α = 0.05. I The sample mean is calculated as y = 120.5. Find the value of the test statistic z. I Make a decision at α = 0.05, reject H0 or not? Wensong Wu Biostatistics Topic 3 One Sample Z Test for µ Example: An at-risk high blood pressure has a systolic blood pressure level higher than 120 mmHg. A random sample of four measurements has been taken on Jane to test if her blood pressure level is at risk of high blood pressure, and the results are y1 = 120, y2 = 117, y3 = 122, y4 = 123. The measurement is known to have a standard deviation of σ = 1. I Set up hypotheses. I Find the rejection region at α = 0.05. I The sample mean is calculated as y = 120.5. Find the value of the test statistic z. I Make a decision at α = 0.05, reject H0 or not? I Interpret your decision in the context of the problem. Wensong Wu Biostatistics Topic 3 One Sample Z Test for µ Example: An at-risk high blood pressure has a systolic blood pressure level higher than 120 mmHg. A random sample of four measurements has been taken on Jane to test if her blood pressure level is at risk of high blood pressure, and the results are y1 = 120, y2 = 117, y3 = 122, y4 = 123. The measurement is known to have a standard deviation of σ = 1. I Set up hypotheses. I Find the rejection region at α = 0.05. I The sample mean is calculated as y = 120.5. Find the value of the test statistic z. I Make a decision at α = 0.05, reject H0 or not? I Interpret your decision in the context of the problem. I Do not reject H0. At 0.5 level of significance, there is insufficient evidence that the mean systolic blood pressure is higher than 120 mmHg. Wensong Wu Biostatistics Topic 3 One Sample Z Test for µ Example: An at-risk high blood pressure has a systolic blood pressure level higher than 120 mmHg. A random sample of four measurements has been taken on Jane to test if her blood pressure level is at risk of high blood pressure, and the results are y1 = 120, y2 = 117, y3 = 122, y4 = 123. The measurement is known to have a standard deviation of σ = 1. I Do not reject H0. At 0.5 level of significance, there is insufficient evidence that the mean systolic blood pressure is higher than 120 mmHg. Wensong Wu Biostatistics Topic 3 One Sample Z Test for µ Example: An at-risk high blood pressure has a systolic blood pressure level higher than 120 mmHg. A random sample of four measurements has been taken on Jane to test if her blood pressure level is at risk of high blood pressure, and the results are y1 = 120, y2 = 117, y3 = 122, y4 = 123. The measurement is known to have a standard deviation of σ = 1. I Do not reject H0. At 0.5 level of significance, there is insufficient evidence that the mean systolic blood pressure is higher than 120 mmHg. I The sample mean y = 120.5 is higher than 120. Can you claim Jane is at risk of high blood pressure? Wensong Wu Biostatistics Topic 3 P-Value I Making decision based on rejection region has some drawbacks. Wensong Wu Biostatistics Topic 3 P-Value I Making decision based on rejection region has some drawbacks. I It depends on the direction of H1 and α. Wensong Wu Biostatistics Topic 3 P-Value I Making decision based on rejection region has some drawbacks. I It depends on the direction of H1 and α. I It does not provide a “degree of significance”. Wensong Wu Biostatistics Topic 3 P-Value I Making decision based on rejection region has some drawbacks. I It depends on the direction of H1 and α. I It does not provide a “degree of significance”. I P-value is an observed significance level. Interpretation: It is the probability of observing this sample data or more extreme towards H1 assuming H0 is true. So if it is small, we tend to reject H0. P-value is Wensong Wu Biostatistics Topic 3 P-Value I Making decision based on rejection region has some drawbacks. I It depends on the direction of H1 and α. I It does not provide a “degree of significance”. I P-value is an observed significance level. Interpretation: It is the probability of observing this sample data or more extreme towards H1 assuming H0 is true. So if it is small, we tend to reject H0. P-value is I NOT the prob. that the null hypothesis happened by chance. Wensong Wu Biostatistics Topic 3 P-Value I Making decision based on rejection region has some drawbacks. I It depends on the direction of H1 and α. I It does not provide a “degree of significance”. I P-value is an observed significance level. Interpretation: It is the probability of observing this sample data or more extreme towards H1 assuming H0 is true. So if it is small, we tend to reject H0. P-value is I NOT the prob. that the null hypothesis happened by chance. I NOT the prob. that your decision is wrong. Wensong Wu Biostatistics Topic 3 P-Value I Making decision based on rejection region has some drawbacks. I It depends on the direction of H1 and α. I It does not provide a “degree of significance”. I P-value is an observed significance level. Interpretation: It is the probability of observing this sample data or more extreme towards H1 assuming H0 is true. So if it is small, we tend to reject H0. P-value is I NOT the prob. that the null hypothesis happened by chance. I NOT the prob. that your decision is wrong. I NOT the prob the data is a product of random chance. Wensong Wu Biostatistics Topic 3 P-Value I P-value: It is the probability of observing this sample data or more extreme towards H1 assuming H0 is true. So if it is small, we tend to reject H0. Wensong Wu Biostatistics Topic 3 P-Value I P-value: It is the probability of observing this sample data or more extreme towards H1 assuming H0 is true. So if it is small, we tend to reject H0. I How to calculate it in the Z test? Tip: Draw a graph! Wensong Wu Biostatistics Topic 3 P-Value I P-value: It is the probability of observing this sample data or more extreme towards H1 assuming H0 is true. So if it is small, we tend to reject H0. I How to calculate it in the Z test? Tip: Draw a graph! I Make decision: Reject H0 whenever p-value < α. Wensong Wu Biostatistics Topic 3 One Sample Z Test for µ Example: An at-risk high blood pressure has a systolic blood pressure level higher than 120 mmHg. A random sample of four measurements has been taken on Jane to test if her blood pressure level is at risk of high blood pressure, and the results are y1 = 120, y2 = 117, y3 = 122, y4 = 123. The measurement is known to have a standard deviation of σ = 1. I The value of the test statistics was calculated as z = 1. Wensong Wu Biostatistics Topic 3 One Sample Z Test for µ Example: An at-risk high blood pressure has a systolic blood pressure level higher than 120 mmHg. A random sample of four measurements has been taken on Jane to test if her blood pressure level is at risk of high blood pressure, and the results are y1 = 120, y2 = 117, y3 = 122, y4 = 123. The measurement is known to have a standard deviation of σ = 1. I The value of the test statistics was calculated as z = 1. I Find the p-value. Interpret it. Wensong Wu Biostatistics Topic 3 One Sample Z Test for µ Example: An at-risk high blood pressure has a systolic blood pressure level higher than 120 mmHg. A random sample of four measurements has been taken on Jane to test if her blood pressure level is at risk of high blood pressure, and the results are y1 = 120, y2 = 117, y3 = 122, y4 = 123. The measurement is known to have a standard deviation of σ = 1. I The value of the test statistics was calculated as z = 1. I Find the p-value. Interpret it. I Make decision at α = 0.05. Wensong Wu Biostatistics Topic 3 One Sample Z Test for µ Example: An at-risk high blood pressure has a systolic blood pressure level higher than 120 mmHg. A random sample of four measurements has been taken on Jane to test if her blood pressure level is at risk of high blood pressure, and the results are y1 = 120, y2 = 117, y3 = 122, y4 = 123. The measurement is known to have a standard deviation of σ = 1. I The value of the test statistics was calculated as z = 1. I Find the p-value. Interpret it. I Make decision at α = 0.05. I Is the decision same as the one made by using RR? Wensong Wu Biostatistics Topic 3 Models We reviewed the Normal Model and the process of building up the inferential statistical methods for one population mean. When learning any statistical model, we will answer the following questions. I What type of data fits the model? What are the assumptions of the data? (The diagnosis phase). I What is the probability model behind the data? (Learn about the disease) I How to analyze the data using the model? How to implement the inferential statistical methods by formula and by software? What are the pros and cons of the methods? How to interpret the conclusion? (Learn how to treat the disease.) Wensong Wu Biostatistics Topic 3 STA 6176 Biostatistics Topic 2 Review of Basic Concepts Instructor: Wensong Wu Wensong Wu Biostatistics Topic 2 Basic Terminology I Population: The collection of all subjects considered in the research problem or question. I Sample: A subset of population. We collect data only on the sample of subjects. I Parameter: Numerical summary of a population. I e.g. population mean µ, population standard deviation σ I Usually unknown or difficult to know the true value. I Statistic: Numerical summary of a sample. I e.g. sample mean x̄, sample standard deviation s I Calculable from data I Usually serve as an estimator of a parameter. Wensong Wu Biostatistics Topic 2 Basic Terminology I Descriptive Statistics: Recognize patterns of sample. I Graphical tools, descriptive statistics I Help check conditions of statistical models and inferential methods. I Review in Chapter 3. I Inferential Statistics: Draw conclusions on population based on a sample. I Estimation of parameters. I Point estimates. I Confidence intervals. I Hypothesis testing. I Other inferences including prediction, classification,... I Probability: The mathematical tool that quantifies randomness caused by sampling. Wensong Wu Biostatistics Topic 2 Concepts in Probability: Random Variable A Random Variable (RV) is the chance process which generates the value of a variable in a sample. I Its value may vary from one subject to another with an underlying population distribution. I A variable can be categorical or numerical, but RV is usually reserved for numerical variables. I Categorical (or qualitative) variable: takes values on a set of categories, e.g. gender, blood type. I Numerical (or quantitative) variable: takes values on numbers. I Discrete RV: may take on only a countable number of distinct values, e.g. the number of boys in a family, the number of women who took oral contraceptives in a sample of 10, the amount of prize in a lottery... I Continuous RV: possible values form one or more intervals, e.g. blood pressure, age. Wensong Wu Biostatistics Topic 2 Concepts in Probability: Random Variable I Random Variable (RV) is the chance process which generates the value of a variable in a sample. I Usually denoted by X , Y , Z ,... I The realization of RV is called a (sample) data. I Usually denoted by x, y , z,.... Example: Let Y=the number of boys in a family. You plan to investigate n random selected families and denote the numbers of boys by Y1 , Y2 ,... , Yn. The recorded numbers of a boys from one sample are denoted by y1 , y2 ,P... , yn. From descriptive statistics: Sample mean of data: ȳ = ( inP =1 yi )/n n (yi − ȳ )2 Sample variance of data: s 2 = i=1. n−1 Q: Can we do the same to the RVs? Wensong Wu Biostatistics Topic 2 Concepts in Probability: Probability distribution Probability distribution of a RV describes how it varies by specifying possible values and corresponding probability. I For discrete RV: The probability distribution is described by the probability mass function (pmf). I It can be presented by a formula, a tabular form, or a graph. I Example: Y=the number of boys in a 8-child family. The pmf of Y is: p(y ) = Pr (Y = y ), y = 0, 1, 2,..., 8 0.3 Number of Boys Probability Number of Boys Probability 0.2 0 0.0040 6 0.1244 I 2 0.02 77 0.099 3 7 8 0.0 390 0.0064 1< o., 3 0. 1984 4 0.2787 5 0.2222 Total 1.0000 Numberof~ 3ln Fmmilie s wilhElghtC hildren □ ol Wensong Wu Biostatistics Topic 2 Concepts in Probability: Probability distribution Discrete RV: Probability mass function (pmf): p(y ) = Pr (Y = y ) Q: What is the total probability P I p(y )? I Expected Value (or Mean) of Y: X µ= yp(y ) I Variance of Y: X σ2 = (y − µ)2 p(y ) I They are the population mean and variance. Wensong Wu Biostatistics Topic 2 Concepts in Probability: Probability distribution Continuous RV: The probability distribution is described by the probability density function (pdf) f (y ) I It can be presented in the form of formula or graph. I The probabiity that the value of the RV Y falls in an interval (a, b), denoted by P(a < Y < b), is the area under the curve of pdf between a and b. I Q: What is the total area under pdf? I Q: What is P(Y = a) for any a? Wensong Wu Biostatistics Topic 2 Concepts in Probability: Probability distribution Continuous RV: Probability density function f (y ). I The expected value and variance of a continuous RV can be calculated using the pdf. Discrete Continuous R P µ: P yp(y ) yf (y )dy 2 2 (y − µ)2 f (y )dy R σ : (y − µ) p(y ) I Again, they are the population mean and variance. Wensong Wu Biostatistics Topic 2 Concepts in Probability: Probability distribution The most important continuous dist. is Normal Distribution. I N(µ, σ 2 ) denotes the normal distribution with a mean of µ and a standard deviation of σ. I N(0, 1) is called the standard normal distribution. I Standardization by Z-Score: If X ∼ N(µ, σ2) then Z = X σ−µ ∼ N(0, 1). I Important quantiles of standard normal Z : P(−1.96 < Z < 1.96) = 0.95. -1.96 1.96 Wensong Wu Biostatistics Topic 2 Concepts in Probability: Estimator I Observations are said to be statistically independent if the value of one observation does not influence the value of any other observations. I Estimator/Statistic as random variable! I It is a random variable with some probability distribution, because its value may vary from one sample to another. I For a parameter θ, an estimator θ̂ is unbiased if E (θ̂) = θ. I An estimate is a particular value of the estimator, computed from the sample data. It is considered fixed, given the data. Example: Suppose Y1 , Y2 ,... , Yn are independent and identically distributed Pn (iid) with a population mean µ. The sample mean Y = ( i=1 Yi )/n is an estimator of µ. Once data collected P from n an experiment, the sample mean has one value ȳ = ( i=1 yi )/n. Wensong Wu Biostatistics Topic 2 Concepts in Probability: Degrees of Freedom Given a set of N quantities and M(≤ N) independent constraints, the number of degrees of freedom (DF) associated with the N quantities is N − M. Example: In a family, there are X girls and Y boys. Consider the DF associated with X and Y. I If no constraint, DF =2, because X and Y are free to change. I If in an 8-child family, there is a linear constraint X + Y = 8. If X is free, then Y = 8 − X is determined. So we lose one degree of freedom, and the DF=2-1=1. Q: What is the DF of the sample variance RV? Pn 2 (Yi − Y¯ )2 S = i=1 n−1 Wensong Wu Biostatistics Topic 2 Sampling Distribution I The probability distribution of a sample statistic (as a RV) is called Sampling Distribution. I We can apply probability theory to investigate the sampling distribution, and it will help us eventually infer the population parameter based on a sample. Example: Y1, Y2,... , Yn are iid from a population with a population mean µ. I µ is our target parameter. Sample mean Y = ( ni=1 Yi )/n is a point estimator of µ. P I I What is the sampling distribution of Y ? Wensong Wu Biostatistics Topic 2 Sampling Distribution - Case A Case A: Let’s assume that I Population distribution is the Normal distribution. I Population variance σ2 is known. What is the sampling distribution of Y ? Wensong Wu Biostatistics Topic 2 Sampling Distribution - Case A Case A: Let’s assume that I Population distribution is the Normal distribution. I Population variance σ2 is known. What is the sampling distribution