Classical Demand Theory PDF
Document Details
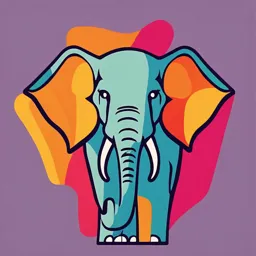
Uploaded by ComprehensiveFern
Tags
Summary
This chapter explores classical demand theory, focusing on preference-based approaches. It includes sections on preference relations, utility functions, expenditure minimization and duality theory.
Full Transcript
# Chapter 3: Classical Demand Theory ## 3.A Introduction In this chapter, we study the classical, preference-based approach to consumer demand. We begin in Section 3.B by introducing the consumer's preference relation and some of its basic properties. We assume throughout that this preference re...
# Chapter 3: Classical Demand Theory ## 3.A Introduction In this chapter, we study the classical, preference-based approach to consumer demand. We begin in Section 3.B by introducing the consumer's preference relation and some of its basic properties. We assume throughout that this preference relation is rational, offering a complete and transitive ranking of the consumer's possible consumption choices. We also discuss two properties, monotonicity (or its weaker version, local nonsatiation) and convexity, that are used extensively in the analysis that follows. Section 3.C considers a technical issue: the existence and continuity properties of utility functions that represent the consumer's preferences. We show that not all preference relations are representable by a utility function, and we then formulate an assumption on preferences, known as continuity, that is sufficient to guarantee the existence of a (continuous) utility function. In Section 3.D, we begin our study of the consumer's decision problem by assuming that there are $L$ commodities whose prices she takes as fixed and independent of her actions (the price-taking assumption). The consumer's problem is framed as one of utility maximization subject to the constraints embodied in the Walrasian budget set. We focus our study on two objects of central interest: the consumer's optimal choice, embodied in the Walrasian (or market or ordinary) demand correspondence, and the consumer's optimal utility value, captured by the indirect utility function. Section 3.E introduces the consumer's expenditure minimization problem, which bears a close relation to the consumer's goal of utility maximization. In parallel to our study of the demand correspondence and value function of the utility maximization problem, we study the equivalent objects for expenditure minimization. They are known, respectively, as the Hicksian (or compensated) demand correspondence and the expenditure function. We also provide an initial formal examination of the relationship between the expenditure minimization and utility maximization problems. In Section 3.F, we pause for an introduction to the mathematical underpinnings of duality theory. This material offers important insights into the structure of preference-based demand theory. Section 3.F may be skipped without loss of continuity in a first reading of the chapter. Nevertheless, we recommend the study of its material. Section 3.G continues our analysis of the utility maximization and expenditure minimization problems by establishing some of the most important results of demand theory. These results develop the fundamental connections between the demand and value functions of the two problems. In Section 3.H, we complete the study of the implications of the preference-based theory of consumer demand by asking how and when we can recover the consumer's underlying preferences from her demand behavior, an issue traditionally known as the integrability problem. In addition to their other uses, the results presented in this section tell us that the properties of consumer demand identified in Sections 3.D to 3.G as necessary implications of preference-maximizing behavior are also sufficient in the sense that any demand behavior satisfying these properties can be rationalized as preference-maximizing behavior. The results in Sections 3.D to 3.H also allow us to compare the implications of the preference-based approach to consumer demand with the choice-based theory studied in Section 2.F. Although the differences turn out to be slight, the two approaches are not equivalent; the choice-based demand theory founded on the weak axiom of revealed preference imposes fewer restrictions on demand than does the preference-based theory studied in this chapter. The extra condition added by the assumption of rational preferences turns out to be the symmetry of the Slutsky matrix. As a result, we conclude that satisfaction of the weak axiom does not ensure the existence of a rationalizing preference relation for consumer demand. Although our analysis in Sections 3.B to 3.H focuses entirely on the positive (i.e., descriptive) implications of the preference-based approach, one of the most important benefits of the latter is that it provides a framework for normative, or welfare, analysis. In Section 3.1, we take a first look at this subject by studying the effects of a price change on the consumer's welfare. In this connection, we discuss the use of the traditional concept of Marshallian surplus as a measure of consumer welfare. We conclude in Section 3.J by returning to the choice-based approach to consumer demand. We ask whether there is some strengthening of the weak axiom that leads to a choice-based theory of consumer demand equivalent to the preference-based approach. As an answer, we introduce the strong axiom of revealed preference and show that it leads to demand behavior that is consistent with the existence of underlying preferences. Appendix A discusses some technical issues related to the continuity and differentiability of Walrasian demand. For further reading, see the thorough treatment of classical demand theory offered by Deaton and Muellbauer (1980). ## 3.B Preference Relations: Basic Properties In the classical approach to consumer demand, the analysis of consumer behavior begins by specifying the consumer's preferences over the commodity bundles in the consumption set X = $R^L$. The consumer's preferences are captured by a preference relation (an "at-least-as-good-as" relation) defined on X that we take to be rational in the sense introduced in Section 1.B; that is, is complete and transitive. For convenience, we repeat the formal statement of this assumption from Definition 1.B.1.1 **Definition 3.B.1:** The preference relation ≥ on X is rational if it possesses the following two properties: **(i) Completeness.** For all x, y ∈ X, we have x ≥ y or y ≥ x (or both). **(ii) Transitivity.** For all x, y, z ∈ X, if x ≥ y and y ≥ z, then x ≥ z. In the discussion that follows, we also use two other types of assumptions about preferences: desirability assumptions and convexity assumptions. **(i) Desirability assumptions.** It is often reasonable to assume that larger amounts of commodities are preferred to smaller ones. This feature of preferences is captured in the assumption of monotonicity. For Definition 3.B.2, we assume that the consumption of larger amounts of goods is always feasible in principle; that is, if x ∈ X and y ≥ x, then y∈ X. **Definition 3.B.2:** The preference relation ≥ on X is **monotone** if x ≥ x and y >> x implies y ≻ x. It is **strongly monotone** if y ≥ x and y ≠ x imply that y ≻ x. The assumption that preferences are monotone is satisfied as long as commodities are "goods" rather than "bads". Even if some commodity is a bad, however, we may still be able to view preferences as monotone because it is often possible to redefine a consumption activity in a way that satisfies the assumption. For example, if one commodity is garbage, we can instead define the individual's consumption over the "absence of garbage". Note that if ≥ is monotone, we may have indifference with respect to an increase in the amount of some but not all commodities. In contrast, strong montonicity says that if y is larger than x for some commodity and is no less for any other, then y is strictly preferred to x. For much of the theory, however, a weaker desirability assumption than monotonicity, known as **local nonsatiation**, actually suffices. **Definition 3.B.3:** The preference relation ≥ on X is **locally nonsatiated** if for every x∈ X and every ε > 0, there is y ∈X such that ||y - x|| ≤ ε and y ≻ x. The test for locally nonsatiated preferences is depicted in Figure 3.B.1 for the case in which X = $R^L$. It says that for any consumption bundle x ∈ $R^L$ and any arbitrarily small distance away from x, denoted by ε > 0, there is another bundle y ∈ $R^L$ within this distance from x that is preferred to x. Note that the bundle y may even have less of every commodity than x, as shown in the figure. Nonetheless, when X = $R^L$ local nonsatiation rules out the extreme situation in which all commodities are bads, since in that case no consumption at all (the point x = 0) would be a satiation point. **(ii) Convexity assumptions.** A second significant assumption, that of **convexity** of ≥, concerns the trade-offs that the consumer is willing to make among different goods. **Definition 3.B.4:** The preference relation ≥ on X is **convex** if for every x∈ X, the upper contour set {y∈ X: y ≥ x} is convex; that is, if y ≥ x and z ≥ x, then ay + (1 - α)z ≥ x for any α ∈ [0, 1]. **Definition 3.B.5:** The preference relation ≥ on X is **strictly convex** if for every x, we have that y ≥ x, z ≥ x, and y ≠ z implies ay + (1 - α)z ≻ x for all α ∈ (0, 1). In applications (particularly those of an econometric nature), it is common to focus on preferences for which it is possible to deduce the consumer's entire preference relation from a single indifference set. Two examples are the classes of homothetic and quasilinear preferences. **Definition 3.B.6:** A monotone preference relation ≥ on X = $R^L$ is **homothetic** if all indifference sets are related by proportional expansion along rays; that is, if x ~ y, then ax ~ ay for any a ≥ 0. **Definition 3.B.7:** The preference relation ≥ on X = (-∞, ∞) × $R^{L-1}$ is **quasilinear** with respect to commodity 1 (called, in this case, the numeraire commodity) if **(i) All the indifference sets are parallel displacements of each other along the axis of commodity 1.** That is, if x ~ y, then (x + αe₁) ~ (y + αe₁) for e₁ = ( 1, 0, ..., 0) and any α ∈ R. **(ii) Good 1 is desirable.** That is, x + αe₁ ≻ x for all x and α > 0. ## 3.C Preference and Utility For analytical purposes, it is very helpful if we can summarize the consumer's preferences by means of a utility function because mathematical programming techniques can then be used to solve the consumer's problem. In this section, we study when this can be done. Unfortunately, with the assumptions made so far, a rational preference relation need not be representable by a utility function. We begin with an example illustrating this fact and then introduce a weak, economically natural assumption (called continuity) that guarantees the existence of a utility representation. **Example 3.C.1:** The Lexicographic Preference Relation. For simplicity, assume that X = $R^L$. Define x ≥ y if either “$x_1 > y_1$” or “$x_1 = y_1$ and $x_2 ≥ y_2$." This is known as the lexicographic preference relation. The name derives from the way a dictionary is organized; that is, commodity 1 has the highest priority in determining the preference ordering, just as the first letter of a word does in the ordering of a dictionary. When the level of the first commodity in two commodity bundles is the same, the amount of the second commodity in the two bundles determines the consumer's preferences. In Exercise 3.C.1, you are asked to verify that the lexicographic ordering is complete, transitive, strongly monotone, and strictly convex. Nevertheless, it can be shown that no utility function exists that represents this preference ordering. This is intuitive. With this preference ordering, no two distinct bundles are indifferent; indifference sets are singletons. Therefore, we have two dimensions of distinct indifference sets. Yet, each of these indifference sets must be assigned, in an order-preserving way, a different utility number from the one-dimensional real line. In fact, a somewhat subtle argument is actually required to establish this claim rigorously. It is given, for the more advanced reader, in the following paragraph. Suppose there is a utility function u(·). For every $x_1$, we can pick a rational number $r(x_1)$ such that $u(x_1, 2) > r(x_1) > u(x_1, 1)$. Note that because of the lexicographic character of preferences, $x_1 > x'_1$, implies $r(x_1) > r(x'_1)$ [since $r(x_1) > u(x_1, 1) > u(x_1, 2) > r(x'_1)]$. Therefore, $r(\cdot)$ provides a one-to-one function from the set of real numbers (which is uncountable) to the set of rational numbers (which is countable). This is a mathematical impossibility. Therefore, we conclude that there can be no utility function representing these preferences… The assumption that is needed to ensure the existence of a utility function is that the preference relation be continuous. **Definition 3.C.1:** The preference relation ≥ on X is **continuous** if it is preserved under limits. That is, for any sequence of pairs ${(x^n, y^n)}_{n = 1}^\infty$ with $x^n ≥ y^n$ for all n, x = lim$_{n→∞} x^n$, and y = lim$_{n→∞} y^n$, we have x ≥ y. ## 3.D The Utility Maximization Problem We now turn to the study of the consumer's decision problem. We assume throughout that the consumer has a rational, continuous, and locally nonsatiated preference relation, and we take u(x) to be a continuous utility function representing these preferences. For the sake of concreteness, we also assume throughout the remainder of the chapter that the consumption set is X = $R^L$. The consumer’s problem of choosing her most preferred consumption bundle, given prices p>> 0 and wealth level w > 0, can now be stated as the following utility maximization problem (UMP): $\max_{x \geq 0} u(x)$ $s.t. p \cdot x \leq w$. In the UMP, the consumer chooses a consumption bundle in the Walrasian budget set $B_{p, w}= {x ∈ R^L: p \cdot x ≤ w}$ to maximize her utility level. We begin with the results stated in Proposition 3.D.1 **Proposition 3.D.1:** If p >> 0 and u() is continuous, then the utility maximization problem has a solution. - If u(·) is continuously differentiable, an optimal consumption bundle x* ∈ x(p, w) can be characterized in a very useful manner by means of first-order conditions. The Kuhn Tucker (necessary) conditions (sec Section M.K of the Mathematical Appendix) say that if x* ∈ x(p,w) is a solution to the UMP, then there exists a Lagrange multiplier λ ≥ 0 such that for all l = 1, ..., L: $∇u(x^*) ≤ λp$, with equality if $x_l > 0$. Equivalently, if we let Vu(x) = [du(x)/dx1, …, du(x)/dxL] denote the gradient vector of u(·) at x, we can write (3.D.1) in matrix notation as $Vu(x^*) ≤ λp$ and $x^* \cdot [Vu(x^*) – λp] = 0$. Thus, if we are at an interior optimum (i.e., if x* >> 0), we must have Vu(x*) = λp. The expression on the left of (3.D.5) is the marginal rate of substitution of good for good k at x*, $MRS_{lk}(x*)$; it tells us the amount of good k that the consumer must be given to compensate her for a one-unit marginal reduction in her consumption of good . . In the case where L = 2, the slope of the consumer’s indifference set at x* is precisely $MRS_{12}(x*)$. Condition (3.D.5) tells us that at an interior optimum, the consumer’s marginal rate of substitution between any two goods must be equal to their price ratio, the marginal rate of exchange between them, as depicted in Figure 3.D.4(a). Were this not the case, the consumer could do better by marginally changing her consumption. For example, if [du(x*)/dx_]/[du(x*)/dxk] > (pklpk), then an increase in the consumption of good l of dx, combined with a decrease in good k’s consumption equal to ($p_l/p_k$) dx, would be feasible and would yield a utility change of [du(x*)/dx_]dx, - [du(x*)/dxk]($p_l/p_k$) dx, > 0. ## 3.E The Expenditure Minimization Problem In this section, we study the following expenditure minimization problem (EMP) for p >> 0 and u > u(0): Min p \cdot x x ≥ 0 s.t. u(x) ≥ u. Whereas the UMP computes the maximal level of utility that can be obtained given wealth w, the EMP computes the minimal level of wealth required to reach utility level u. The EMP is the “dual” problem to the UMP. It captures the same aim of efficient use of the consumer’s purchasing power while reversing the roles of objective function and constraint. Throughout this section, we assume that u(·) is a continuous utility function representing a locally nonsatiated preference relation defined on the consumption set $R^L$. The EMP is illustrated in Figure 3.E.1. The optimal consumption bundle x* is the least costly bundle that still allows the consumer to achieve the utility level u. Geometrically, it is the point in the set {x ∈ $R^L$: u(x) ≥ u} that lies on the lowest possible budget line associated with the price vector p. **Proposition 3.E.1:** Suppose that u(·) is a continuous utility function representing a locally nonsatiated preference relation defined on the consumption set X = $R^L$ and that the price vector is p >> 0. We have **(i) If x* is optimal in the UMP when wealth is w > 0, then x* is optimal in the EMP when the required utility level is u(x*). Moreover, the minimized expenditure level in this EMP is exactly w.** **(ii) If x* is optimal in the EMP when the required utility level is u > u(0), then x* is optimal in the UMP when wealth is p \cdot x*. Moreover, the maximized utility level in this UMP is exactly u.** **Proof:** **(i) Suppose that x* is not optimal in the EMP with required utility level u(x*). Then there exists an x' such that u(x') ≥ u(x*) and p \cdot x' < p \cdot x* < w. By local nonsatiation, we can find an x" very close to x' such that u(x") > u(x') and p \cdot x" < w. But this implies that x" ∈ $B_{p, w}$ and u(x") > u(x*), contradicting the optimality of x* in the UMP. Thus, x* must be optimal in the EMP when the required utility level is u(x*), and the minimized expenditure level is therefore p · x*. Finally, since x* solves the UMP when wealth is w, by Walras’ Law, we have p* · x* = w. **(ii) Since u > u(0), we must have x* ≠ 0. Hence, p · x* > 0. Suppose that x* is not optimal in the UMP when wealth is p · x*. Then there exists an x' such that u(x') > u(x*) and p \cdot x' < p · x*. Consider a bundle x" = αx' where α ∈ (0,1) (x" is a "scaled-down" version of x'). By continuity of u(), if α is close enough to 1, then we will have u(x") > u(x*) and p · x" < p · x*. But this contradicts the optimality of x* in the EMP. Thus, x* must be optimal in the UMP when wealth is p · x*, and the maximized utility level is therefore u(x*). In Proposition 3.E.3(ii), we will show that if x* solves the EMP when the required utility level is u, then u(x*) = u. ## 3.F Duality: A Mathematical Introduction This section constitutes a mathematical detour. It focuses on some aspects of the theory of convex sets and functions. Recall that a set K ∈ $R^L$ is convex if ax + (1 - α)z ∈ K whenever x, z ∈ K and a ∈ [0, 1]. Note that the intersection of two convex sets is a convex set, A half-space is a set of the form {x ∈ $R^L$: p · x ≥ c} for some p ∈ $R^L$, p≠ 0, called the normal vector to the half-space, and some c ∈ R. Its boundary {x ∈ $R^L$: p · x = c} is called a hyperplane. The term normal comes from the fact that whenever p · x = p · x' = c, we have p(x – x') = 0, and so p is orthogonal (i.e., perpendicular, or normal) to the hyperplane (see Figure 3.F.1). Note that both half-spaces and hyperplanes are convex sets. Suppose now that K < $R^L$ is a convex set that is also closed (i.e., it includes its boundary points), and consider any point x ∉ K outside of this set. A fundamental theorem of convexity theory, the separating hyperplane theorem, tells us that there is a half-space containing K and excluding x (see Section M.G of the Mathematical Appendix). That is, there is a p ∈ $R^L$ and a c ∈ R such that p · x < c < p · x for all x ∈ K. The basic idea behind duality theory is the fact that a closed, convex set can equivalently ("dually") be described as the intersection of the half-spaces that contain it; this is illustrated in Figure 3.F.2(a). Because any x ∉ K is excluded by some half-space that contains K, as we draw such half-spaces for more and more points x ∉ K, their intersection (the shaded area in the figure) becomes equal to K. More generally, if the set K is not convex, the intersection of the half-spaces that contain K is the smallest closed, convex set that contains K, known as the closed, convex hull of K. Figure 3.F.2(b) illustrates a case where the set K is nonconvex; in the figure, the closed convex hull of K is K. Given any closed (but not necessarily convex) set K < $R^L$ and a vector p ∈ $R^L$, we can define the support function of K. **Definition 3.F.1:** For any nonempty closed set K < $R^L$, the support function of K is defined for any p ∈ $R^L$ to be μκ(p) = Infimum {p · x:x∈K}. The infimum of a set of numbers, as used in Definition 3.F.1, is a generalized version of the set’s minimum value. In particular, it allows for situations in which no minimum exists because although points in the set can be found that come arbitrarily close to some lower bound value, no point in the set actually attains that value. For example, consider a strictly positive function $f(x)$ that approaches zero asymptotically as x increases. The minimum of this function does not exist, but its infimum is zero. The formulation also allows μκ(p) to take the value -∞ when points in K can be found that make the value of p · x unboundedly negative. When K is convex, the function μκ(·) provides an alternative ("dual") description of K because we can reconstruct K from knowledge of μκ(·). In particular, for every p, {x ∈ $R^L$: p · x≥ μκ(p)} is a half-space that contains K. In addition, as we discussed above, if x ∉ K, then p · x < μκ(p) for some p. Thus, the intersection of the half-spaces generated by all possible values of p is precisely K; that is, K = {x ∈ $R^L$: p · x≥ μκ(p) for every p}. By the same logic, if K is not convex, then {x ∈ $R^L$: p · x ≥ μκ(p) for every p} is the smallest closed, convex set containing K. The function μκ(·) is homogeneous of degree one. More interestingly, it is concave. To see this, consider p’ = ap + (1 – α)p’ for α ∈ [0,1]. To make things simple, suppose that the infimum is in fact attained, so that there is a z ∈ K such that μκ(p’) = p’ · z. Then, because μκ(p’) = ap · z + (1 - α)p’ · z ≥ αμκ(p) + (1 – α)μκ(p’). We conclude that μκ(·) is concave. The concavity of μκ(·) can also be seen geometrically. Figure 3.F.3 depicts the value of the function φx(p) = p · x, for various choices of x ∈ K, as a function of $p_2$ (with p₁ fixed at $p_1$). For each x, the function φx(·) is a linear function of $p_2$. Also shown in the figure is μκ(·). For each level of $p_2$, μκ(p₁, $p_2$) is equal to the minimum (technically, the infimum) of the various linear functions $φ_x(·)$ at p = ($p_1$, $p_2$); that is, μκ(p₁, $p_2$) = Min {φx(p₁, $p_2$): x ∈ K}. As can be seen in the figure, μκ(·) is therefore the lower envelope of all the φx(·). As K is for example when p₁ = $p_1$, φx(p₁, $p_2$) ≤ x(p₁, $p_2$) for all x ∈ K. As K is for example when p₁ = $p_1$, φx(p₁, $p_2$) ≤ x(p₁, $p_2$) for all x ∈ K. As K is for example when p₁ = $p_1$, φx(p₁, $p_2$) ≤ x(p₁, $p_2$) for all x ∈ K. As K is for example when p₁ = $p_1$, φx(p₁, $p_2$) ≤ x(p₁, $p_2$) for all x ∈ K. As K is for example when p₁ = $p_1$, φx(p₁, $p_2$) ≤ x(p₁, $p_2$) for all x ∈ K. As K is for example when p₁ = $p_1$, φx(p₁, $p_2$) ≤ x(p₁, $p_2$) for all x ∈ K. As K is for example when p₁ = $p_1$, φx(p₁, $p_2$) ≤ x(p₁, $p_2$) for all x ∈ K. As K is for example when p₁ = $p_1$, φx(p₁, $p_2$) ≤ x(p₁, $p_2$) for all x ∈ K. As K is for example when p₁ = $p_1$, φx(p₁, $p_2$) ≤ x(p₁, $p_2$) for all x ∈ K. As K is for example when p₁ = $p_1$, φx(p₁, $p_2$) ≤ x(p₁, $p_2$) for all x ∈ K. As K is for example when p₁ = $p_1$, φx(p₁, $p_2$) ≤ x(p₁, $p_2$) for all x ∈ K. As K is for example when p₁ = $p_1$, φx(p₁, $p_2$) ≤ x(p₁, $p_2$) for all x ∈ K. As K is for example when p₁ = $p_1$, φx(p₁, $p_2$) ≤ x(p₁, $p_2$) for all x ∈ K. As K is for example when p₁ = $p_1$, φx(p₁, $p_2$) ≤ x(p₁, $p_2$) for all x ∈ K. As K is for example when p₁ = $p_1$, φx(p₁, $p_2$) ≤ x(p₁, $p_2$) for all x ∈ K. As K is for example when p₁ = $p_1$, φx(p₁, $p_2$) ≤ x(p₁, $p_2$) for all x ∈ K. As K is for example when p₁ = $p_1$, φx(p₁, $p_2$) ≤ x(p₁, $p_2$) for all x ∈ K. As K is for example when p₁ = $p_1$, φx(p₁, $p_2$) ≤ x(p₁, $p_2$) for all x ∈ K. As K is for example when p₁ = $p_1$, φx(p₁, $p_2$) ≤ x(p₁, $p_2$) for all x ∈ K. As K is for example when p₁ = $p_1$, φx(p₁, $p_2$) ≤ x(p₁, $p_2$) for all x ∈ K. As K is for example when p₁ = $p_1$, φx(p₁, $p_2$) ≤ x(p₁, $p_2$) for all x ∈ K. As K is for example when p₁ = $p_1$, φx(p₁, $p_2$) ≤ x(p₁, $p_2$) for all x ∈ K. As K is for example when p₁ = $p_1$, φx(p₁, $p_2$) ≤ x(p₁, $p_2$) for all x ∈ K. As K is for example when p₁ = $p_1$, φx(p₁, $p_2$) ≤ x(p₁, $p_2$) for all x ∈ K. As K is for example when p₁ = $p_1$, φx(p₁, $p_2$) ≤ x(p₁, $p_2$) for all x ∈ K. As K is for example when p₁ = $p_1$, φx(p₁, $p_2$) ≤ x(p₁, $p_2$) for all x ∈ K. As K is for example when p₁ = $p_1$, φx(p₁, $p_2$) ≤ x(p₁, $p_2$) for all x ∈ K. As K is for example when p₁ = $p_1$, φx(p₁, $p_2$) ≤ x(p₁, $p_2$) for all x ∈ K. As K is for example when p₁ = $p_1$, φx(p₁, $p_2$) ≤ x(p₁, $p_2$) for all x ∈ K. As K is for example when p₁ = $p_1$, φx(p₁, $p_2$) ≤ x(p₁, $p_2$) for all x ∈ K. As K is for example when p₁ = $p_1$, φx(p₁, $p_2$) ≤ x(p₁, $p_2$) for all x ∈ K. As K is for example when p₁ = $p_1$, φx(p₁, $p_2$) ≤ x(p₁, $p_2$) for all x ∈ K. As K is for example when p₁ = $p_1$, φx(p₁, $p_2$) ≤ x(p₁, $p_2$) for all x ∈ K. As K is for example when p₁ = $p_1$, φx(p₁, $p_2$) ≤ x(p₁, $p_2$) for all x ∈ K. As K is for example when p₁ = $p_1$, φx(p₁, $p_2$) ≤ x(p₁, $p_2$) for all x ∈ K. As K is for example when p₁ = $p_1$, φx(p₁, $p_2$) ≤ x(p₁, $p_2$) for all x ∈ K. As K is for example when p₁ = $p_1$, φx(p₁, $p_2$) ≤ x(p₁, $p_2$) for all x ∈ K. As K is for example when p₁ = $p_1$, φx(p₁, $p_2$) ≤ x(p₁, $p_2$) for all x ∈ K. As K is for example when p₁ = $p_1$, φx(p₁, $p_2$) ≤ x(p₁, $p_2$) for all x ∈ K. As K is for example when p₁ = $p_1$, φx(p₁, $p_2$) ≤ x(p₁, $p_2$) for all x ∈ K. As K is for example when p₁ = $p_1$, φx(p₁, $p_2$) ≤ x(p₁, $p_2$) for all x ∈ K. As K is for example when p₁ = $p_1$, φx(p₁, $p_2$) ≤ x(p₁, $p_2$) for all x ∈ K. As K is for example when p₁ = $p_1$, φx(p₁, $p_2$) ≤ x(p₁, $p_2$) for all x ∈ K. As K is for example when p₁ = $p_1$, φx(p₁, $p_2$) ≤ x(p₁, $p_2$) for all x ∈ K. As K is for example when p₁ = $p_1$, φx(p₁, $p_2$) ≤ x(p₁, $p_2$) for all x ∈ K. As K is for example when p₁ = $p_1$, φx(p₁, $p_2$) ≤ x(p₁, $p_2$) for all x ∈ K. As K is for example when p₁ = $p_1$, φx(p₁, $p_2$) ≤ x(p₁, $p_2$) for all x ∈ K. As K is for example when p₁ = $p_1$, φx(p₁, $p_2$) ≤ x(p₁, $p_2$) for all x ∈ K. As K is for example when p₁ = $p_1$, φx(p₁, $p_2$) ≤ x(p₁, $p_2$) for all x ∈ K. As K is for example when p₁ = $p_1$, φx(p₁, $p_2$) ≤ x(p₁, $p_2$) for all x ∈ K. As K is for example when p₁ = $p_1$, φx(p₁, $p_2$) ≤ x(p₁, $p_2$) for all x ∈ K. As K is for example when p₁ = $p_1$, φx(p₁, $p_2$) ≤ x(p₁, $p_2$) for all x ∈ K. As K is for example when p₁ = $p_1$, φx(p₁, $p_2$) ≤ x(p₁, $p_2$) for all x ∈ K. As K is for example when p₁ = $p_1$, φx(p₁, $p_2$) ≤ x(p₁, $p_2$) for all x ∈ K. As K is for example when p₁ = $p_1$, φx(p₁, $p_2$) ≤ x(p₁, $p_2$) for all x ∈ K.