Biostatistics Summary PDF
Document Details
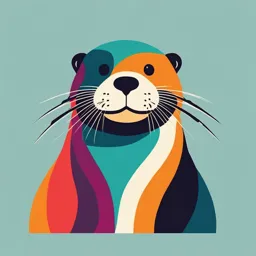
Uploaded by ComfortingAestheticism
University of Debrecen Faculty of Medicine
2024
Tibor G. Szántó
Tags
Summary
This document summarizes key concepts in biostatistics, including probability, conditional probability, and descriptive statistics. It covers various distributions, such as binomial, Poisson, and normal distributions, with medical applications. Formulas and notations are also included within the document.
Full Transcript
Biostatistics: Summary Tibor G. Szántó 08/11/2024 Events, probability, conditional probability. Highlights: Some of the basic ideas and concepts of probability (axioms of Kolomogorov) were presented. The probability is a number between 0 and 1 that measures the likelihood...
Biostatistics: Summary Tibor G. Szántó 08/11/2024 Events, probability, conditional probability. Highlights: Some of the basic ideas and concepts of probability (axioms of Kolomogorov) were presented. The probability is a number between 0 and 1 that measures the likelihood of the occurrence of events. Defined and illustrated the calculation of marginal, joint, and conditional probabilities. The meaning and calculation of independent, mutually exclusive, and complementary events. Medical implications of conditional probability: specificity, sensitivity, predictive value positive, and negative predictive value as applied to a screening test or disease symptom. Notations: - The probability and the relative frequency cannot be greater than 1. In contrast, the (absolute) frequency can be. gyakorisága of “tail” 1 0.9 k 0.8 frequency - Relative frequency (k/n) ≠ probability (p), but =p 0.7 lim 0.6 n → n 0.5 "Fej" relatív 0.4 Relative 0.3 0.2 0.1 - Addition rule: p(A+B) = p(A)+p(B)–p(AB) 0 0 100 200 Number of300flips dobások száma 400 500 p(A+B) = p(A) + p(B) Only true for mutually exclusive events! - Mutually exclusive events are not independent! (if one of them occurs, the other will definitely NOT occur because they have no intersection. So, the occurrence of one DOES have an effect on the occurrence of the other) - Independent events: p(AB)=p(A)p(B) but p(AB)=0 for mutually exclusive events - The sum of the probabilities of two mutually exclusive events is not necessarily equal to one. 2 Events, probability, conditional probability. Formulas: Graphs, tables: Venn diagrams 𝑛 𝑛 Marginalization 𝑝 𝐴 = 𝑝 𝐴𝐵𝑖 = 𝑝 𝐴 𝐵𝑖 × 𝑝 𝐵𝑖 A B A B A B A B 𝑖=1 𝑖=1 A B A B Ā independent events Contingency table, e.g., Disease Test result Yes (D+) No (D-) Total 𝑇𝑃 𝑃 𝑇 + 𝐷 + = Positive (T+) TP FP (T+)=TP+FP 𝑇𝑃 + 𝐹𝑁 Negative (T-) FN TN (T-)=FN+TN 𝑇𝑁 𝑃 𝑇 − 𝐷 − = 𝑇𝑁 + 𝐹𝑃 Total (D+)=TP+FN (D-)=FP+TN TN+FP+FN+TP 𝑇𝑃 𝑃 𝐷 + 𝑇 + = 𝑇𝑃 + 𝐹𝑃 𝑇𝑁 𝑃 𝐷 − 𝑇 − = 𝑇𝑁 + 𝐹𝑁 3 Events, probability, conditional probability. True or false? 1. When rolling a fair die there are 6 outcomes of the experiment, and each outcome has a probability of 1/6. 2. The probability of getting a head in coin flipping is ½. 3. The relative frequency of getting a head in coin flipping always ½. 4. Conditional probability can be larger than one in the case of events depending on each other. 5. The specificity of a diagnostic test is the conditional probability of a negative test result given the absence of the disease. 4 Descriptive statistics. Highlights: Various descriptive statistical procedures are explained; e.g., the organization of data by means of the ordered array, the frequency distribution, the relative frequency distribution, and the histogram. The concepts of central tendency and spread are described, along with methods for computing their more common measures: the mean, median, mode, range, variance, and standard deviation. The concepts of skewness and exploratory data analysis such as the box-and-whisker plot. Software (e.g., Excel) and statistical mode of calculators are useful tools for calculating descriptive measures and constructing various distributions from data sets. Notations: - The mean is distorted by outliers and skewed data. - The mean and the median are the same only if the distribution is symmetrical. - If the number of elements is odd, the median is the middle value; if the number of elements is even, the median is the average of the two middle values in the ordered array. - The number of modes is not necessarily one. If all the values are different in the sample, there is no mode. - Standard deviation (SD, s) of samples is an unbiased estimation of the population SD (). xi the i-th element of the sample or in the population ( x − x) n 2 𝑥ҧ the mean of the sample (x − ) 2 i SDsample = i =1 and x = i μ the mean of the population n −1 N n the number of elements in the sample N the number of the elements in the population - C.V. is a useful statistic for comparing the variability of two or more variables measured on different scales. - Different methods can be used for determining Q1, and Q3!! 5 Descriptive statistics. Formulas: Graphs, tables: Histogram Sturges’ rule (the number 𝑘 = 1 + 3,3219 log10 𝑁 of class intervals, k) Box-and-whisker plot maximum (sample) Q3 (population) x = (x − ) i N 2 median } interquartile range Q1 minimum 6 Descriptive statistics. True or false? 1. The coefficient of variation (CV) expresses the standard deviation as a percentage of the mean. 2. The coefficient of variation (CV) cannot be greater than 100%. 3. The mode of a set of values is the value which occurs the least frequently. 4. The difference between the mean and the mode is equal to the median. 5. The value found with the largest frequency in a sample is the mean of the sample. 7 Distributions: binomial, Poisson, normal, and standard normal distribution. Highlights: The concepts of discrete and continuous random variables; probability distributions and cumulative distribution function. In particular, two discrete probability distributions, the binomial and the Poisson, and one continuous probability distribution, the normal, are examined in considerable detail. These distributions allow us to make probability statements about certain random variables that have medical relevance, since many variables in medicine have exactly two outcomes or can be modeled by normal distribution. Notations: - A variable assigned to a random event is called a random variable. - The probability distribution of a discrete random variable is a table, graph, or formula used to specify all possible values of a discrete random variable along with their respective probabilities. - Cumulative probability distribution (cumulative distribution function – CDF) gives the probability that a random variable is less than or equal to a specified value. - Continuous random variables have probability density function (PDF), as well. - Properties of the binomial distribution: 1. Each observation falls into one of just two categories. 2. The n observations are all independent. 3. The probability of a given outcome is constant over all trials. 4. The number of trials n is fixed. - Poisson distribution is preferred if n is large (the experiment is carried out a large number of times or the population from which the sample is taken is large) and/or p (or q) is small. - Normal distributions are always symmetrical; the mean, the median, and the mode are all equal. - Standard normal distribution has a mean of 0 and a standard deviation of 1. 8 Distributions: binomial, Poisson, normal, and standard normal distribution. Formulas: Graphs, functions: = pk xk CDF (discrete random variable) k n n = pi ( x − ) = pi xi2 − 2 2 2 i =1 i =1 𝑛! stepwise function 𝑃 x=𝑘 = 𝑝𝑘 𝑞 𝑛−𝑘 𝑘! 𝑛 − 𝑘 ! Mean and SD of the μ=n×p 𝑆𝐷 = n×p×q binomial distribution l𝑘 −l 𝑝𝑘 = 𝑃 x = 𝑘 = 𝑒 𝑘! Mean and SD of the μ=l 𝑆𝐷 = l f(x) Poisson distribution 9 Distributions: binomial, Poisson, normal, and standard normal distribution. Graphs, functions: PDF (continuous random variable) Example: P (60 ≤ x ≤ 80) Probability density 80 function P ( 60 x 80 ) = f ( x ) dx 60 a b random variable CDF (continuous random variable) F(x) 0.5 Sigmoidal curve 0 μ 10 Distributions: binomial, Poisson, normal, and standard normal distribution. True or false? 1. The value assumed by the cumulative distribution function (c.d.f., also known as cumulative probability distribution) of a random variable at x gives the probability that the random variable is smaller than or equal to x. 2. A continuous random variable does not have a cumulative distribution function. 3. The binomial distribution is a good approximation of the age distribution of individuals in a large population. 4. All normal distributions have a standard deviation of 1. 5. If the mean of a normal distribution is larger than 1, the probability that the random variable assumes a value smaller than zero is less than 0.5. 11 Sampling. Highlights: A parameter is a numerical characteristic of interest from the target population. It has a DEFINITIVE value (even if we do not know this value) A sample is selected to collect information about this (population) parameter Goals of sampling: 1. estimate the value of the parameter (point estimate, confidence interval), 2. compare the estimated parameters for two subpopulations using statistical tests Central limit theorem: when n is large, the distribution of the sample means (𝒙𝒊 ) will be a normal distribution with a mean of , and with a standard deviation of σ𝑥 = σ𝑥 / 𝑛 (=SEM). Notations: Parameters vs. statistics: Sample Population (statistics) (parameter) 𝑥ҧ s, SD(n-1) - An efficient estimator has a relatively small standard error, meaning that the variance of its sampling distribution is small - The most important attributes of the quality of an estimate: accuracy (unbiasedness, its expected value is equal to the estimated parameter) and precision (reproducibility, its standard deviation is small). - Representative sampling: each element of the population has equal probability of being sampled. In general, the larger the sample the more precise the estimate of the population parameter. ഥ is an unbiased estimator of . - 𝒙 - SD(n-1) of a sample gives an unbiased estimation of the population SD 𝝈𝒙 12 Sampling. Formulas: Graphs, functions: 𝑆𝐷 𝑛−1 Low accuracy Low accuracy 𝑆𝐸𝑀 = Low precision High precision 𝑛 It measures how precisely the sample mean 𝑥 as a statistic estimates the population mean µ High accuracy High accuracy Low precision High precision If n → ∞ 𝑥ҧ → 𝜇 𝑆𝐷 → 𝜎 𝑆𝐸𝑀 → 0 sample mean population small n large n 13 Sampling. True or false? 1. If a sample is large (n>30), it is by definition representative. 2. The fact whether a sample is representative is determined by its size. 3. If the standard errors of the mean (SEM) of two samples taken from the same population are compared, the SEM of the larger sample is smaller. 4. According to the central limit theorem, the distribution of any random variable can be approximated by the normal distribution. 14 Hypothesis testing. Highlights: Even if the original population was not normally distributed, at large sample sizes the sample mean will still be normally distributed and parametric statistical tests can be performed. General concepts of hypothesis testing are discussed. A number of specific hypothesis tests are described in detail and illustrated with appropriate examples. These include tests concerning population means (u- and t-test), the difference between two population means (unpaired t-test), paired comparisons (paired t-test), and the population variance (F-test). In addition we discussed the type I and type II errors, and the p-value of test statistics. Notations: - A general procedure for carrying out a hypothesis test consisting of the following steps is suggested 1. Decide to be used. 2. Statement of null and alternative hypotheses. 3. Calculation of test statistic from sample data. 4. Find the critical value from the appropriate statistical table (one- or two tailed test!) 5. Statistical decision: keep or reject H0 by comparing the calculated value (from sample) and the critical value (from table). 6. Conclusion. 7. Determination of p value. If p< , null hypothesis is rejected If p> , null hypothesis is not rejected 15 Hypothesis testing. Highlights: Even if the original population was not normally distributed, at large sample sizes the sample mean will still be normally distributed and parametric statistical tests can be performed. General concepts of hypothesis testing are discussed. A number of specific hypothesis tests are described in detail and illustrated with appropriate examples. These include tests concerning population means (u- and t-test), the difference between two population means (unpaired t-test), paired comparisons (paired t-test), and the population variance (F-test). In addition we discussed the type I and type II errors, and the p-value of test statistics. Notations: - u: , - t: ( is estimated by the SD of the sample) - Paired t: the same subjects may be measured before and after receiving some treatment. - F-test is always two-tailed related to an unpaired t-test. - Failure to reject the null hypothesis implies insufficient evidence for its rejection. - The value is the probability of the current data or data that is more extreme assuming H0 is true. - Statistical significance says very little about the importance of a relation. 16 Hypothesis testing. Formulas: Graphs, tables: Test statistics for … Let =0.05 𝑥ҧ − 𝜇 Two-tailed test u-test: 𝑢 = 𝜎 𝑛 𝑥ҧ − 𝜇 𝑥ҧ − 𝜇 One sample t-test: 𝑡𝑛−1 = = 𝑆𝐷 𝑆𝐸𝑀 𝑛 𝑑ҧ Paired t-test: 𝑡𝑛−1 = 𝑆𝐷𝑑 𝑛 𝑥ҧ1 − 𝑥ҧ 2 Unpaired t-test: 𝑡𝑛1 +𝑛2−2 = 1 1 𝑠𝑝2 + 𝑛1 𝑛2 𝑛1 − 1 𝑆𝐷12 + 𝑛2 − 1 𝑆𝐷22 pooled variance 𝑠𝑝2 = 𝑛1 + 𝑛2 − 2 𝑆𝐷12 F-test: 𝐹𝑛1−1,𝑛2 −1 = if SD1>SD2 𝑆𝐷22 17 Hypothesis testing. True or false? 1. The level of significance of a statistical test is equal to the probability of committing a type II error. 2. A two-sample t-test can be used to compare the SDs of two samples. 3. If the p value of a statistical test is smaller than the level of significance, the null hypothesis has to be rejected. 4. A statistically significant difference implies the importance of the observed difference (if the level of significance is smaller than 1%). 5. In the case of a two-sided statistical test, the rejection region consists of two parts on the two sides of the distribution of the test statistics. 18 Medical implications of conditional probability. Highlights: Test of independence using 2 test is discussed. The procedure consists of computing a statistic that measures the discrepancy between the observed and expected frequencies of occurrence of values in certain discrete categories. The 2×2 contingency table has special importance, since the investigation of two dichotomous random variables is wide-spread (e.g. positive/negative, yes/no, male/female). In addition, we discussed and illustrated other techniques for analyzing frequency data that can be presented in the form of a contingency table: odds ratio and relative risk. Finally, we discussed the basic concepts of survival analysis. Notations: - The null hypothesis of a chi-square test states that the two variables are independent of each other. - In the case of chi-square test the number of degrees of freedom is (number of columns – 1)×(number of rows – 1). - The reciprocal relationship between sensitivity and specificity is demonstrated by the ROC plot. - Positive and negative predictive values are dependent on the fraction of diseased persons. - The odds ratio approximates the relative risk well if the number of sick persons is low. - In case-control studies the OR is the appropriate statistic, the RR depends on the size of the diseased population in the sample. Medical implications of conditional probability. Formulas: Graphs, tables: Test statistics for chi-square test: ROC curve 1 2 𝑒𝑥𝑝𝑒𝑐𝑡𝑒𝑑 − 𝑜𝑏𝑠𝑒𝑟𝑣𝑒𝑑 2 = 0.8 𝑜𝑏𝑠𝑒𝑟𝑣𝑒𝑑 𝑓𝑜𝑟 𝑎𝑙𝑙 𝑐𝑒𝑙𝑙𝑠 sensitivity 0.6 0.4 𝑎/ 𝑎 + 𝑏 𝑎 𝑐+𝑑 𝑅𝑅 = = 𝑐/ 𝑐 + 𝑑 𝑐 𝑎+𝑏 0.2 0 0 0.2 0.4 0.6 0.8 1 𝑜𝑑𝑑𝑠𝑑𝑖𝑠𝑒𝑎𝑠𝑒𝑑 𝑎𝑑 𝑂𝑅 = = 1-specificity 𝑜𝑑𝑑𝑠ℎ𝑒𝑎𝑙𝑡ℎ𝑦 𝑏𝑐 Kaplan-Meier curve 1 rel. freq. of survivors Risk Disease 0.8 total factor yes no 0.6 yes a b a+b 0.4 no c d c+d 0.2 total a+c b+d N=a+b+c+d 0 0 5 10 15 20 25 20 median survival time survival time (months) Medical implications of conditional probability. True or false? 1. A diagnostic test whose positive predictive value is 100% correctly identifies 100% of diseased individuals. 2. It is possible to modify the threshold value of a diagnostic test in such a way that both sensitivity and specificity increase. 3. The probability that a patient survives beyond the median survival time is 50%. 4. Odds ratio is always greater than 1. 5. For a given diagnostic test, the negative predicitve value is always less than its positive predicitve value. 21 Medical implications of conditional probability. Analysis of discrete random variables 1 0.8 proportion surviving 0.6 0.4 0.2 0 0 50 100 150 time (months) Investigation of discrete random variables Conditional probability Medical applications: evaluation of diagnostic procedures (specificity, sensitivity, positive and negative predictive values) epidemiologic studies, evaluation of risk factors analysis of survival curves 1/18 Contingency table used for plotting the relationship between two discrete random variables values of the 1st discrete rand. var. Plot of two continuous random type 1 type 2 type 3 variables random variable 2 treatment treatment treatment ineffective number of number of number of Σ(ineffective) discrete rand. var. values of the 2nd individuals individuals individuals partially number of number of number of Σ(partially effective individuals individuals individuals effective) effective number of number of number of Σ(effective) individuals individuals individuals Σ(treatment Σ(treatment Σ(treatment all persons cell 1) 2) 3) random variable 1 marginal frequencies The table above is a 3×3 contingency table. Values of the discrete random variable are called category, class or level. 2/18 Test of independence using χ2 test (1) Why is the test of independence useful? E.g. if drug treatment and treatment outcome are independent, the drug does not work. 1. Sampling and generation of the contingency table Types of treatment A1 A2 A3 efficiency of treatment B1 (in- number of number of number of Σ(B1) =NB1 effective) individuals individuals individuals B2 number of number of number of (partially Σ(B2) =NB2 individuals individuals individuals effective) B3 number of number of number of Σ(B3) =NB3 (effective) individuals individuals individuals Total number of Σ(A1)=NA1 Σ(A2)=NA2 Σ(A3) =NA3 persons (N) Types of treatment E.g.: A1 A2 A3 B1 (in- efficiency of treatment 16 22 19 57 effective) B2 (partially 19 29 10 58 effective) B3 47 40 15 102 (effective) 82 91 44 217 3/18 Test of independence using χ2 test (2) Types of treatment Types of treatment A1 A2 A3 A1 A2 A3 B1 (in- number of number of number of efficiency of treatment Σ(B1) =NB1 B1: in- 16 22 19 efficiency of treatment effective) individuals individuals individuals 57 effective 21.5 23.9 11.6 B2 number of number of number of (partially Σ(B2) =NB2 B2: partially 19 29 10 individuals individuals individuals 58 effective) effective 21.9 24.3 11.8 B3 number of number of number of Σ(B3) =NB3 47 40 15 (effective) individuals individuals individuals B3: effective 102 38.5 42.8 20.7 Total number of Σ(A1)=NA1 Σ(A2)=NA2 Σ(A3) =NA3 persons (N) 82 91 44 217 2. Set up the null hypothesis: the two variables are independent of each other. 3. Calculate the expected frequencies , i.e. numbers, in each cell assuming that the two variables are independent of each other. The probabilities are approximated by the relative frequencies (e.g. NA1/N). Probability of the joint occurrence of A1 and B1. N A1 N B1 N N E A1, B1 = P ( A1 B1 ) N = P ( A1 ) P ( B1 ) N = N = A1 B1 N N N ( E A1, B1 = the expected number of persons in the red cell) N A1 N B1 82 ⋅ 57 N A 2 N B1 91 ⋅ 57 E A1, B1 = = =21.5 E A 2, B1 = = =23.9 … N 217 N 217 There will be 9 observed and expected frequency pairs in the table. 4/18 Test of independence using χ2 test (3) Types of treatment A1 A2 A3 B1: in- 16 22 19 efficiency of treatment 57 effective 21.5 23.9 11.6 B2: partially 19 29 10 58 effective 21.9 24.3 11.8 47 40 15 B3: effective 102 38.5 42.8 20.7 82 91 44 217 4. Calculate the χ2 statistics: (E ij − Oij ) 2 Eij – the expected number of individuals in a cell χ2 = for every cell Eij Oij – the observed number of individuals in a cell ( 21.5 − 16) ( 23.9 − 22) (11.6 − 19) 2 2 2 χ = 2 for every cell 21.5 + 23.9 + 11.6 +... = 11.5 5/18 Test of independence using χ2 test (4) 5. Degrees of freedom of the test: d. f. = ( c − 1)( r − 1) , where c and r are the number of columns and rows, respectively. 6. Look up the critical value corresponding to the given level of significance from the table of the χ2 test with the corresponding degrees of freedom. Tests of independence are one-sided. f(χ2) critical χ2=9.49 (d.f.=4, level of significance=5%) χ2 7. If χ2calculated> χ2critical, the null hypothesis is rejected, the two variables are not independent of each other. 6/18 What is the relationship between the χ2 test and the formula learnt previously for the independence of two events? Types of treatment Two events (A and B) are independent if A1 A2 A3 P ( A B) = P ( A) B1: in- 16 22 19 efficiency of treatment 57 effective 21.5 23.9 11.6 P ( AB ) P ( A B) = = P ( A) B2: partially 19 29 10 58 P (B) effective 21.9 24.3 11.8 47 40 15 B3: effective 102 38.5 42.8 20.7 P ( AB ) = P ( A ) P ( B ) 82 91 44 217 We use the same formula in the χ2 test for the calculation of the expected number of the joint occurrence of two events (A1, B1) assuming their independence : N A1 N B1 N N 82 ⋅ 57 P ( A1 ) P ( B1 ) N = N = A1 B1 = E A1, B1 = =21.5 ⇔ OA1, B1 = 16 N N N 217 What is the reason for the difference? We can decide between the two 1. random variation options using the χ2 test. 2. the null hypothesis is not true, therefore, the observed and expected frequencies differ because the expected frequencies were calculated using a wrong null hypothesis. 7/18 Conditions for using the χ2 test 1. It can be used for testing random variables with any kind of distribution. 2. Sample size should be large. Specific numerical requirements vary: N≥30 there cannot be any cell in which the expected frequency is less than 5. Other sources state a less stringent requirement: the number of cells in which the expected frequency is less than 5 cannot be larger than 1/5 (in other sources 50%,…) of all cells. What to do if the sample size is small? 1. Yates’s correction: (E ) 2 − Oij − 0.5 ij χYates 2 = for every cell Eij Reduces the value of the calculated χ2, increases the p value of the test (more extreme values are required to reach significance). Its applicability is disputed, it overcompensates according to many sources. 2. Fisher exact test 8/18 Test of independence using a 2×2 contingency table Special importance, since the investigation of two dichotomous random variables is wide-spread (dichotomous – assumes only two values, has only two classes, e.g. positive/negative, yes/no, male/female). values of rand. variable ‘B’ B1 B2 Total values of rand. A1 a b a+b variable ‘A’ A2 c d c+d Total: a+c b+d N=a+b+c+d The expected frequency cannot be less than 5 in any of the cells. The simplified formula of the χ2 test for a 2×2 contingency table: N ( ad − bc ) 2 χ2 = ( a + b )( c + d )( a + c )( b + d ) The degrees of freedom of the test is 1. 9/18 Epidemiologic investigations (1): types of observational studies – Case-control study (retrospective): I want to find the Observational study: the ratio of risk-exposed investigator does not modify the risk present ? healthy persons in the past studied parameter, i.e. people are risk absent by analyzing medical not forced to be exposed to the records, asking questions, etc. risk factor. risk present ? risk absent diseased past present I will investigate the ratio of – Prospective cohort study diseased persons in the two groups. I create two groups and wait diseased till the disease has time to no risk factor healthy develop, and then … ? Cohort: a group sharing some characteristics, e.g. risk factor diseased exposure, followed in time. risk factor healthy present ? future I retrospectively identify exposed and non-exposed people (e.g. in medical records) and investigate – Retrospective cohort study diseased their current disease condition no risk factor healthy ? diseased risk factor healthy ? past present risk exposed risk free – Cross-sectional study: a snapshot is taken in healthy healthy the present time diseased diseased present 10/18 Epidemiologic investigations (2): relative risk and the odds ratio Diseased Not diseased Total Risk factor present A B A+B Risk factor absent C D C+D Total A+C B+D Relative risk (RR): how many times the disease is more frequent in the group exposed to a risk factor than in the non- exposed, control group: A RelFreqrisk+ A + B AC+D A⋅ D RR = = = ≈ , if C 0 ҧ 𝑥−𝜇 ( x1 − x2 ) − ( 1 − 2 ) 𝑡𝑛−1 = 𝑆𝐷𝑛−1 / 𝑛 tn1 + n2 − 2 = sx1 − x2 (difference between sample means) - (difference between population means) (standard error of the difference between sample means) 2 2 1 1 (𝑛1 −1)𝑆𝐷𝑛−1,1 + (𝑛 2 − 1)𝑆𝐷𝑛−1,2 sx − x = s + 2 𝑠𝑝2 = p n1 n2 1 2 𝑛1 + 𝑛2 − 2 Critically important assumption: the 12 and 22 variances are equal. 19 Why do we need an F test? We need to check if the population variances are equal so that we can proceed with the t-test. 𝑯𝟎 : 𝝈𝟐𝟏 = 𝝈𝟐𝟐 𝑯𝑨 : 𝝈𝟐𝟏 ≠ 𝝈𝟐𝟐 ❑20 F-test When SDn-1,12 and SDn-1,22 are sample variances from independent samples of sizes n1 and n2 drawn from normal populations, the F statistic 2 𝑆𝐷𝑛−1,1 𝐹= 2 𝑆𝐷𝑛−1,2 has the F distribution with n1 − 1 and n2 − 1 degrees of freedom when H0: σ1 = σ2 is true. 21 F-test The F distributions are right-skewed and cannot take negative values. ◼ The peak of the F density curve is near 1 when the two population standard deviations are equal. ◼ Values of F far from 1 provide evidence against the hypothesis of equal standard deviations. 𝑆𝐷𝑛21−1 Always divide the larger variance by 𝐹= the smaller, that way F 1. 𝑆𝐷𝑛2 −1 2 𝐷𝑓𝑛𝑢𝑚𝑒𝑟𝑎𝑡𝑜𝑟 : 𝑛1 −1 𝐹 ℎ𝑎𝑠 𝐷𝑓𝑑𝑒𝑛𝑜𝑚 : 𝑛2 −1 ❑22 Calculation and critical value: 2 𝑆𝐷𝑛−1,1 142 𝐹𝑐𝑎𝑙𝑐 = 2 = 2 = 1.36 𝑆𝐷𝑛−1,2 12 ❑ Usually we carry out a two-tailed F-test at α=0.05 ❑ in a one-tailed table, we look for the page of α=0.025 ❑ Each row corresponds to a denominator df ❑ Each column corresponds to a numerator df ❑ Fcalc < F.975 = 2.45, so we do not reject the H0: 1 = 2 so we we meet the condition of equal population variances, ❑23 ❑ we can proceed with the t-test Proceed with the unpaired t-test 2 2 2 2 (𝑛1 −1)𝑆𝐷𝑛−1,1 + (𝑛 2 − 1)𝑆𝐷𝑛−1,2 25 − 1 14 + (20 − 1)12 𝑠𝑝2 = = = 173.02 𝑛1 + 𝑛2 − 2 25 + 20 − 2 𝑠𝑝 = 13.154 1 1 1 1 sX − X = s p + = 13.154 + = 3.945 1 2 n1 n2 25 20 ( X1 − X 2 ) − ( 1 − 2 ) (97 − 89) − 0 t= = = 2.027 s X1 − X 2 3.945 We usually assume 1= 2, so (1- 2) can be omitted. 24 The 2-sample t-test complete formula 𝑥ҧ1 − 𝑥ҧ2 𝑡𝑛1+𝑛2 −2 = 2 2 (𝑛1 −1)𝑆𝐷𝑛−1,1 + (𝑛2 − 1)𝑆𝐷𝑛−1,2 1 1 + 𝑛1 + 𝑛2 − 2 𝑛1 𝑛2 25 Decision: significance level =0.01 (1-tailed), d.f. = n1+n2-2 = 25+20-2 = 43 d.f. 0.4 0.3 =0.01 f (x) 0.2 Col 1 vs norm. distr. = 0.05 Col 1 vs t distr small df Col 1 vs t distr large_df 0.1 Do not reject H0 rejectthe Reject H00 hypothesis 0.0 -4 -3 -2 -1 0 1 2 3 4 tcalc=2.027 t =2.423 crit 1.895 6.92 At ⍺ = 0.01 (the closest to df 40) critical value is tcrit = 2.423 Conclusion: do not reject the null hypothesis. The mean monthly insurance premium paid by the drivers insured by company A is not higher than the mean monthly insurance premium paid by the drivers insured by company B. 26 Hypothesis testing: z-test and t-test. Tibor G Szántó 2024.X.18. Reminder: statistical design and analysis In practice, we rarely want to know the probability of a single measurement falling into a certain range The target of investigation is a population with a certain characteristic. A parameter is a numerical characteristic of interest from the target population. A sample is selected to collect information about this (population) parameter Two types of inference are of interest: 1. Estimate the value of the parameter (point estimate, confidence interval) 2. Compare the estimated parameters for two subpopulations using statistical tests Requirements for proper samples: - the sample must be representative. The sample is representative when we use random sampling, i.e. each element of the population has equal probability of being sampled - the sample must contain large number of elements so that the conclusion be reliable Statistics help us draw inferences or conclusions about population parameters. - after a sample is obtained, the value of the statistics (i.e. the sample mean) is known and fixed. - if another sample is selected the value of the statistic will probably be different. a statistic is a random variable that takes different values from one sample to another 2 Hypothesis testing: introduction. We are again concerned about drawing some conclusion about a population parameter using the information contained in a sample of observations. With statistical tests we claim that the mean of the population is equal to some postulated value μ0 which is called the null hypothesis (H0). The alternative hypothesis (HA) is a second statement that contradicts H0. We are testing both hypotheses at the same time Our result will allow us to either “reject H0” or “fail to reject H0” 3 Hypothesis testing: an example. Consider the distribution of serum cholesterol levels for adult males that is normally distributed with a known σ = 45 mg/100 ml. We hypothesize the population mean of serum cholesterol () is equal to 0=211 mg/100 ml. Test the following null and alternative hypotheses: H0: = µ0(=211 mg/100ml) - our initial guess was likely correct HA: µ0(=211 mg/100ml) - our initial guess was likely incorrect To test these hypotheses we must acquire data from the population - sample size: n = 25 ഥ = 224.5 mg/100 ml (estimate of ) - calculating sample mean: 𝒙 One does not have to be Einstein to see that 224.5 ≠ 211. So what is the rest of the lecture about? Why don’t we simply reject the null hypothesis and say that μ ≠ 211 mg/100ml? Because if we draw multiple samples from the same population, we will get many sample means, i.e., x fluctuates around µ ! So we must reformulate the question: How likely is it to get a sample mean of 𝐱ത=224.5 if the null hypothesis is true? 4 Hypothesis testing: switching to the standard normal distribution. Since 𝐱ത follows a normal distribution (CLT), we can use the standard normal distribution to 0.4 address this question 0=211 Standardization in general: 0.3 relevant x statistics − hypothesized mean of x z( x ) = standardization f (x) 0.2 standard deviation of x 0.1 In this particular case: σ𝑥 =9 0.0 X x − 0 x − 0 224.5 − 211 13.5 211 202 220 ഥ=224.5 𝒙 (x ) = zzcalc = = = = 1.5 x 45 9 0.4 =0 0.4 n 25 zcalc 0.3 0.3 f (x) 0.2 When is z=1.5 is likely to occur? 0.1 0.0 Option A: critical value approach, f (x) 0.2 Option B: exact P approach 0.1 σ𝑧 =1 0.0 -1 0 1 zcalc=1.5 Z(X) 5 Hypothesis testing: level of significance, . When is a z value likely to occur? This is not objective and is determined by the level of significance (α). It specifies the area in the rejection region that determines … … a region where values of the test statistics are likely to occur (with a probability of (1-α)) if the null hypothesis is true. … two regions where values of the test statistics are unlikely to occur (with a cumulative probability of (α)) if the null hypothesis is true. The two regions are symmetric around the origin. α=0.05 α/2=0.025 α/2=0.025 6 Hypothesis testing: critical values. -1.96 and 1.96 are the critical values corresponding to α=0.05 The probability of obtaining values between -1.96 and 1.96 is (1-α) = 0.95 for a standard normal distribution (acceptance area) The probability of obtaining values smaller than -1.96 and larger than 1.96 is cumulative 0.05 for a standard normal distribution (rejection area) α=0.05 α/2=0.025 α/2=0.025 REJECTION AREA ACCEPTANCE AREA REJECTION AREA 7 Hypothesis testing: decision making. The calculated value of the test statistics in the example (z = 1.5) is between the critical values of zcrit = -1.96 and zcrit = 1.96 So it is likely to occur if the null hypothesis is true We do not reject the null hypothesis: H0: μ=211 mg/100 ml might be true! α=0.05 α/2=0.025 α/2=0.025 reject the null hypothesis reject the null hypothesis zz==1.5 1.5 donull do not reject the not hypothesis reject the 0 hypothesis 8 Hypothesis testing: decision making. If in the example 𝒙 ഥ = 233.5, then the calculated value of z: x1 − 233.5 − 211 z1 = = = 2.5 SEM 9 … i.e., z1 is unlike to occur if the null hypothesis is true … we reject the null hypothesis and favor the alternative hypothesis: HA: μ ≠ 211 mg/100 ml might be true! 9 Hypothesis testing: exact P method. Calculate probability (P) that the random variable with z distribution will be as extreme or more extreme than zcalc Compare this P probability with If P< , null hypothesis is rejected If P> , null hypothesis is not rejected 0.45 =0.05 0.4 0.35 =0, =1 0.3 0.25 f(x) 0.2 P/2=0.0668 0.15 P/2=0.0668 0.1 0.05 0 0 -zcalc-1.96 =-1.5 zcalc1.96 =1.5 z 10 Hypothesis testing: error types. Two possible ways to commit an error: type I error: reject H0 when it is true (α), type II error: fail to reject H0 when it is false (β) The goal in hypothesis testing is to keep the probabilities of type I and II errors as small as possible. H0 true H0 false do NOT reject H0 Type II error reject H0 Type I error 11 Hypothesis testing: type I error → . Reject H0 when it is true. How can it be? Due to random sampling individuals with cholesterol levels higher than μ are included in the sample only, i.e., a very unlikely outcome of sampling happened The probability of committing type I error is α, i.e., the level of significance α=0.05 α/2=0.025 α/2=0.025 rejecting the 0 hypothesis although it is true do not reject z = the 2.5 0 hypothesis 12 1 Hypothesis testing: type II error → . Fail to reject H0 when it is false. How can that happen? E.g., a HA: μ=250 mg/100 ml is true, but due to random sampling individuals with cholesterol levels smaller than μ are included in the sample. Thus, although H0: μ=211 mg/100 ml is false we fail to reject it. The probability of committing type II error (β) is unknown, however, is usually larger than α. If we reduce α we increase β! 0.45 α=0.05 0.4 0.35 =0, =1 =?, 1 =1 0.3 0.25 f(x) 0.2 /2=0.025 0.15 (1-)=0.95 /2=0.025 0.1 0.05 0 -1.96 0 1.96 z x=1.5 β Fail to reject a 0 hypothesis although it is false 13 Hypothesis testing: one- and two-tailed analysis. In the problem we tested the following hypotheses: we hypothesize the population mean of serum cholesterol is 211 mg/100 ml, i.e.: Ho: = µ0(=211 mg/100ml) HA: µ0(=211 mg/100ml) How would the hypotheses change if we asked the following: Do overweight people have higher cholesterol level than 211 mg/ml? Research claim is “overweight have increased cholesterol level” → Search for evidence against H0 Alternative hypotheses and null hypotheses ◼ HA: µ 211 (two-tailed) H0: µ = 211 ◼ HA: µ > 211 (one-tailed to right) H0: µ ≤ 211 ◼ HA: µ < 211 (one-tailed to left) H0: µ ≥ 211 14 How does a one-tailed analysis differ from the two-tailed one? The question is different: ◼ two tailed: is cholesterol level ◼ One tailed: is cholesterol level different from 211 mg/ml? We do larger/smaller? We do care about being not care if larger or smaller larger or smaller Alternative hypotheses and null hypotheses are different ◼ Two tailed: ◼ One-tailed to right: HA: µ 211, H0: µ = 211 HA: µ > 211, H0: µ ≤ 211 ◼ One.tailed to the left HA: µ < 211, H0: µ ≥ 211 The standardization is the same: 𝑥ҧ − 𝜇0 𝑧𝑠𝑡𝑎𝑡 = 𝜎 𝑛 15 How does a one-tailed analysis differ from the two-tailed one? zcrit corresponding to is different one-tailed to right: 0.45 0.4 0.35 /2=0.025 =0, =1 0.3 0.25 /2=0.025 f(x) 0.2 (1-)=0.95 0.15 0.1 0.05 Zcrit=1.64 0 one-tailed to left: -1.96 0 1.96 z -zcrit=-1.96 +zcrit=1.96 =0.05 (1-)=0.95 − ❑ 16 zcrit=-1.64 of 22 16 Hypothesis testing: z test. When is known we use the following test statistic to make statistical inference: ҧ 0 𝑥−𝜇 𝑧= 𝜎 𝑛 But to determine , we need µ (see previous lecture): x = i ( x − ) 2 N How can then we determine , if µ is unknown and only hypothesized? Instead, we calculate SD(n-1) from the sample and use this to estimate σ SD( n −1) = (x − X ) i 2 n −1 In this case, we use a modification of z distribution called the t-distribution 17 z-distribution vs. t distribution. The t distributions become wider for smaller sample sizes (n), reflecting the lack of precision in estimating σ from SD(n-1) → for every n there is a t distribution (more precisely n-1=degree of freedom) When n is very large, SD(n-1) is a very good estimate of σ and the corresponding t distributions are very close to the normal distribution (i.e., as df increases, t becomes increasingly like z ) 𝑥ҧ − 𝜇0 𝑥ҧ − 𝜇0 z= 𝜎 𝑡𝑑𝑓 = 𝑆𝐷(𝑛−1) 𝑛 𝑛 18 Critical values for z and t at identical . =0.05 t9, 0.025=2.262 t9, 0.025=2.262 zcrit=1.64 tcrit=1.833 19 Illustrative example: diabetic weight. Research question: Are diabetics overweight? Measure “% of ideal body weight” in n=18 diabetics” Data points are (actual body weight) ÷ (ideal body weight) × 100% Data {107, 119, 99, 114, 120, 104, 88, 114, 124, 116, 101, 121, 152, 100, 125, 114, 95, 117} Calculate sample mean (𝑥)ҧ = 112.8 sample standard deviation SD(n-1) = 14.4 Conditions for one-sample t-test: Simple random sample Normally distributed population or large sample (large n) estimated with SD(n-1) 20 One-sample t-test: procedure (steps). (A) State the null and alternative hypotheses (B) Decide to be used (determines tcrit) (C) Calculate test statistic tcalc from the sample (D) Decision to keep or reject H0 by comparing tcalc (from sample) and tcrit (from table) A: Step A: one- or two-tailed? H0 and HA accordingly!!!! Alternative hypotheses and 0 hypotheses: HA: µ 100 (two-sided) H0: µ = 100 HA: µ > 100 (one-sided to right) H0: µ ≤ 100 HA: µ < 100 (one-sided to left) H0: µ ≥ 100 21 One-sample t-test: procedure (steps). B: Fixed level testing (e.g., =0.05 and critical value) d.f. 0.4 0.3 = 0.05 f (x) 0.2 Col 1 vs norm. distr. Col 1 vs t distr small df Col 1 vs t distr large_df 0.1 do not reject 0 hypothesis Reject the 0 hypothesis 0.0 -4 -3 -2 -1 0 1 2 3 4 tcrit 1.895 6.92 determines tcrit, comparison of tcrit and tcalc leads to decision about H0 22 One-sample t-test: procedure (steps). C: Determine „tcalc” Convert the sample mean (𝑥ҧ ) to a tcalc 𝑥ҧ − 𝜇0 112.8 − 100 𝑡𝑐𝑎𝑙𝑐 = = = 3.8 𝑆𝐷(𝑛−1) 14.4 𝑛 18 tcalc tells you how many standard errors the sample mean is from hypothesized population mean. 23 One-sample t-test: procedure (steps). D: decision making, fixed level testing (=0.05) tn-1=t17 0.4 0.3 = 0.05 f (x) 0.2 Col 1 vs norm. distr. Col 1 vs t distr small df Col 1 vs t distr large_df 0.1 do not reject 0 hypothesis Reject the 0 hypothesis 0.0 -4 -3 -2 -1 0 1 2 3 4 tcrit = 1.74 tcalc 1.895 = 3.76 6.92 24 Misbeliefs. ▪ Failure to reject the null hypothesis leads to its acceptance. (WRONG! Failure to reject the null hypothesis implies insufficient evidence for its rejection.) ▪ The a value is the probability that the null hypothesis is incorrect. (WRONG! The a value is the probability of the current data or data that is more extreme assuming H0 is true.) ▪ α = 0.05 is a standard with an objective basis. (WRONG! α = 0.05 is merely a convention that has taken on unwise mechanical use. There is no sharp distinction between “significant” and “insignificant” results, only increasingly strong evidence as the p value gets smaller. Surely p = 0.06 is loveable nearly as much as p = 0.05) ▪ Small a values indicate large effects. (WRONG! values tell you next to nothing about the size of an effect.) ▪ Data show a theory to be true or false. (WRONG! Data can at best serve to bolster or refute a theory or claim.) ▪ Statistical significance implies importance. (WRONG! Statistical significance says very little about the importance of a relation) 25 Lecture 6 ❑Estimation, Sampling ❑ Representative sample ❑ Statistics as variables – sampling distribution ❑ Parameters and Statistics ❑ Unbiased estimators ❑ Distribution of the sample mean – the Central limit theorem ❑ SEM (standard error of the mean) ❑Basics of Hypothesis testing Julianna Volkó, Dept. of Biophys. & Cell Biol. The normal distribution: an example Blood sugar level of hungry people is normally distributed with a mean of 4 (mM) and a standard deviation of 5 (mM). What is the probability (p) of finding a value greater than 10 (mM) in a random sample? 0.09 0.08 0.07 0.06 0.05 f(x) 0.04 0.03 0.02 m=4, s=5 0.01 0 4 10 x Estimation Blood sugar level of hungry people is normally distributed with a mean of 4 (mM) and a standard deviation of 5 (mM). Are first year medical students starving during the biostatistics lecture? Would need to know: how the blood sugar of students is distributed important parameters of this distribution (mean, variance) how these compare to the known values of starving people. You can measure all the students’ blood sugar… Is it practical? Or: try to make an estimate (but how?) Estimation, Sampling A sample is selected to collect information about, and thereby estimate, a parameter of interest of the population Population Sample A parameter is a numerical characteristic of interest from the target population. It has a DEFINITIVE value (even if we do not know this value) Goals of sampling: (1) estimate the parameter or (2) compare the parameter across populations (hypothesis testing) Appropriateness of the Sample - Representative sampling ◼ It is important that a sample provides an accurate representation of the population from which it is selected. ◼ Therefore it is crucial that a random sample be drawn. Every element of the population must have equal chances of making it into the sample.* ◼ In general, the larger the sample the more precise the estimate of the population parameter. * This does not mean that all values will have equal chances of getting into the sample, those that occur rarely will rarely make it Estimation, Sampling The most important characteristics of the quality of an estimate: ▪ accuracy or unbiasedness: an estimate is unbiased or accurate if its expected value is equal to the estimated parameter ▪ precision or reproducibility: an estimate is precise or reproducible if its standard deviation is small Low accuracy Low accuracy Low precision High precision High accuracy High accuracy Low precision High precision Statistics are random variables ◼ Statistics help us draw inferences or conclusions about population parameters. ◼ After a sample is obtained, the value of the statistics (i.e. the sample mean) is known and fixed. ◼ If another sample is selected the value of the statistic will probably be different: so a statistic is a random variable that takes different values from one sample to another. These values fluctuate around an expected value, which is a parameter that the statistic can estimate. Parameters vs. Statistics Population Sample Estimation (parameter for the sample, used as) Parameter Statistic Quantity Notation Notation Mean m (mu) 𝑿 (x-bar) Variance s2 x S2 (SD2) Standard s (sigma, σx ) x S (SD) Deviation Proportion p (probability) k/n Unbiased estimation of the population mean µ 𝑿 , the sample mean, is an unbiased m estimator of µ. That is, as the number of X i samples, m, increases towards infinity, the m= i =1 cumulated average of the 𝑿 values will m tend towards µ m→ m X i =1 i m µ m Central Limit Theorem (CLT) n sample mean x population i x= i =1 small n large n n As the sample size n increases ◼ the sample mean X will better estimate the population mean µ ◼ the σ𝑋 standard deviation of the distribution of X will f(x) decrease: σ𝑋 = σ𝑥 /√𝒏 When n is large (>20), the distribution of the sample mean 𝑿 around its expected value µ will be normal even if the population is not 𝒙 𝒙 𝒙 distributed normally Unbiased estimation of the population standard deviation σx SD(n) is a parameter, SD(n-1) is a statistic! ◼ Measures the variability within the sample SD( n ) = i ( x − X ) 2 ◼ ◼ A parameter characteristic of the sample Will likely be different each time the sample n is drawn from the population ◼ Underestimates population variability ◼ We usually do not care about this value… Estimates, from the sample data, the (x − X ) ◼ 2 variability of the population (σx) SD( n −1) = i ◼ It is a statistic n −1 ◼ Will likely be different each time the sample is drawn from the population, so its value fluctuates around σx sx = i ( x − m ) 2 ◼ We usually need this value as we do not wish to measure ALL elements of the population and then CALCULATE σx as a parameter N Unbiased estimation of the population standard deviation σx population sample ( x − x) n 2 calculation i SDsample = i =1 n −1 calculation estimation sx = (x − m)i 2 Population SD N 10 October 2023 Standard Deviation vs. Standard Error ◼ Standard deviation (σ) measures the variability in the population or sample. For the population, σx can be estimated from the sample by SD(n-1) SD( n −1) = i ( x − X ) 2 = ⎯⎯ →s X n −1 ◼ Standard error (of the mean) (SE(M)) is an estimate of the standard deviation of the sample mean (σ𝑥 = σ𝑥 / 𝑛). It uses SD(n-1) as the estimate of σx SD( n −1) sX SEM = = ⎯⎯ → =sX n n ◼ It measures how precisely the sample mean 𝐗 as a statistic estimates the population mean µ ◼ In the absence of information about the population σx, it can be used for testing hypotheses about 𝑋, the sample mean (see t-test) Hypothesis testing: example ◼ We hypothesize that the population mean of serum cholesterol is 211 mg/100 ml. How can we test this experimentally? ◼ ഥ, the sample mean, is a good estimator of m, Since 𝒙 a sample could be drawn to get information about m. ◼ Additional info: we happen to know that the standard deviation of this distribution is assumed to be s=45 mg/100ml and the variable is normally distributed. 0.4 m =211 (???) (s = 45) 0.3 f (x) 0.2 0.1 ഥ= ???? 𝒙 0.0 166 211 256 Hypothesis testing: the null hypothesis ◼ In statistics, a hypothesis is a statement about a population, usually claiming that a parameter assumes a particular numerical value or falls in a certain range of values. ◼ In this example we suppose that the mean of the population (m) is equal to some postulated value m0 (211) which is called the null hypothesis (H0: m=m0) ◼ The alternative hypothesis (Ha) is a second statement that contradicts H0. Ha and H0 together must constitute the complete outcome space. (P of Ha + P of H0 = 1) ◼ In a statistical test we determine if the data (=a sample drawn from the population) support the null hypothesis or not. ◼ We are testing both hypotheses at the same time Our result will allow us to either “reject H0” or “fail to reject H0” Hypothesis testing: example ◼ The variable (Serum cholesterol) is normally distributed with a known s=45 mg/100ml. ◼ We hypothesize the population mean of serum cholesterol is 211 mg/100 ml. ◼ We test the following null and alternative hypotheses: Ho: m = µ0(=211 mg/100ml) - our initial guess was likely correct Ha: m µ0(=211 mg/100ml) - our initial guess was likely incorrect (note: these two possibilities are complementary events) ◼ To test these hypotheses we have to acquire data from the population ◼ Sample size n=25 ◼ What do we calculate from the sample? → the sample mean because it is the estimator of the µ population mean ◼ Let us say that the sample mean X =224.5 mg/100 ml ◼ X=224.5 211= µ0. So ??? ◼ REMEMBER: X fluctuates around µ ! Hypothesis testing: Reformulating the question ◼ Thus, we cannot accept or reject the null hypothesis yet, since we know that the sample mean X is a random variable: ◼ expected value: µ ◼ Standard deviation: σ𝑥 = σ / 𝑛 where σ is the standard deviation of the population and n is the sample size (in this case n=25) ◼ So we must reformulate the question: How likely is it, that a sample with a mean of 𝐱ത=224.5 is randomly drawn if the null hypothesis is true? ◼ The sample is drawn from a normal distribution with µ=211 and s=45 ◼ The sample means drawn from this population have a µ=211 and σ𝑥 =9 0.4 0.4 m=211 m=211 0.3 ഥ=224.5 𝒙 0.3