Measurement Tools for Precision and Accuracy in Epidemiological Studies PDF
Document Details
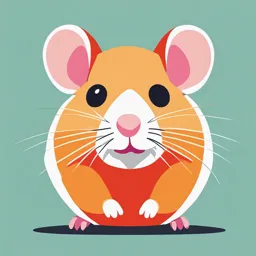
Uploaded by ObservantCalcite2069
Hodeidah University
Tags
Related
- PH146: Epidemiology Ethics in Research PDF
- ActivEpi Español Textbook - Epidemiología
- Formulating and Testing Hypotheses in Epidemiological Research PDF
- ENH 522 Research Methods PDF
- Epidemiology 1: Fundamentals of Epidemiology: Research Concepts and Designs PDF
- Error in Epidemiologic Research Lecture 1 PDF
Summary
This document discusses measurement tools and methodologies for ensuring precision and accuracy in epidemiological research, along with practical examples and types of errors that affect accuracy. The document explores key concepts like sensitivity, specificity, and likelihood ratios, essential for assessing diagnostic test performance.
Full Transcript
Measurement Tools for Precision and Accuracy in Epidemiological Studies In epidemiological research, ensuring both precision and accuracy of measurements is crucial for obtaining reliable and valid results. Precision refers to the consistency or repeatability of measurements, while accuracy descr...
Measurement Tools for Precision and Accuracy in Epidemiological Studies In epidemiological research, ensuring both precision and accuracy of measurements is crucial for obtaining reliable and valid results. Precision refers to the consistency or repeatability of measurements, while accuracy describes how close the measurements are to the true value. Here, we outline key tools and methodologies for assessing and improving these essential aspects of research, followed by an extended explanation of their concepts and applications. --- Key Tools for Measuring Precision and Accuracy 1. Standardized Measurement Protocols - Implementing standardized procedures ensures uniformity in data collection, reducing variability and enhancing precision. 2. Validated Instruments - Using tools validated in prior research guarantees accurate measurement of the intended variables, improving validity and reliability. 3. Training and Calibration of Data Collectors - Rigorous training for researchers and routine calibration of equipment ensure consistent and accurate measurements. 4. Pilot Testing - Conducting pilot studies helps identify potential issues in measurement tools or procedures, facilitating adjustments to enhance accuracy. 5. Blinding - Blinding both participants and data collectors minimizes bias and improves the accuracy of collected data. 6. Regular Quality Control Checks - Implementing ongoing checks detects errors early, maintaining the datas integrity. 7. Statistical Methods for Error Correction - Techniques like regression calibration and simulation-extrapolation help adjust for measurement errors. 8. Sensitivity and Specificity Tests - These diagnostic metrics assess a tests accuracy by evaluating its ability to identify true positives (sensitivity) and true negatives (specificity). 9. Comparison with Gold Standards - Assessing tools against a gold standard ensures alignment with the most reliable and accurate methods available. --- Understanding Accuracy and Precision Definitions - Accuracy: Reflects how close the measurements are to the true value. - Precision: Reflects the repeatability or consistency of measurements, regardless of their accuracy. Practical Example If a persons true weight is 70 kg: - Readings like 70.1, 69.9, 70.2 are both accurate and precise. - Readings like 80.1, 80.2, 80.3 are precise but not accurate. - Readings like 75, 80, 85 are neither accurate nor precise. --- Types of Errors Affecting Accuracy 1. Random Error - Unexpected variability in measurements that reduces precision. 2. Systematic Error (Bias) - A consistent deviation from the true value due to methodological flaws, significantly impacting accuracy. Reducing Errors - Random Errors: Can be minimized by increasing sample size and standardizing methods. - Systematic Errors: Require careful study design and calibration to avoid bias. --- Applications of Accuracy in Epidemiology Sensitivity and Specificity - Sensitivity: The ability to correctly identify true positives (e.g., detecting individuals with a disease). - Specificity: The ability to correctly identify true negatives (e.g., excluding individuals without a disease). Using a 2x2 Table to Assess Diagnostic Test Performance A 2x2 table is a fundamental tool for calculating sensitivity, specificity, and other diagnostic test metrics. It categorizes test outcomes as follows: | | Disease Present (+) | Disease Absent (-) | |---------------------|----------------------|---------------------| | Test Positive (+) | True Positive (TP) | False Positive (FP) | | Test Negative (-) | False Negative (FN) | True Negative (TN) | Key Metrics Derived from the 2x2 Table: 1. Sensitivity: Proportion of true positives among those with the disease. - Formula: Sensitivity = TP / (TP + FN) - Interpretation: High sensitivity ensures fewer missed cases. 2. Specificity: Proportion of true negatives among those without the disease. - Formula: Specificity = TN / (TN + FP) - Interpretation: High specificity ensures fewer false alarms. 3. Positive Predictive Value (PPV): Probability that a person with a positive test result truly has the disease. - Formula: PPV = TP / (TP + FP) - Interpretation: Reflects the tests reliability in confirming the disease. 4. Negative Predictive Value (NPV): Probability that a person with a negative test result truly does not have the disease. - Formula: NPV = TN / (TN + FN) - Interpretation: Reflects the tests reliability in ruling out the disease. 5. Accuracy: Overall correctness of the test. - Formula: Accuracy = (TP + TN) / (TP + TN + FP + FN) - Interpretation: Combines sensitivity and specificity into a single measure. 6. Likelihood Ratios: - Positive Likelihood Ratio (LR+): How much more likely a positive test result is in someone with the disease. - Formula: LR+ = Sensitivity / (1 - Specificity) - Negative Likelihood Ratio (LR-): How much less likely a negative test result is in someone with the disease. - Formula: LR- = (1 - Sensitivity) / Specificity Practical Implications: - High sensitivity is critical for screening tests to minimize missed cases. - High specificity is vital for confirmatory tests to avoid unnecessary interventions. - PPV and NPV depend on the disease prevalence; tests perform differently in high- vs. low-prevalence populations. Importance in Public Health - Ensuring accurate measurements prevents incorrect conclusions that might lead to ineffective health policies or treatments. - Accurate data forms the basis for reliable decision-making and policy formulation. --- Illustrating Accuracy vs. Precision: A Target Analogy - Accurate and Precise: Darts close to the bullseye and tightly grouped. - Precise but not Accurate: Darts tightly grouped but away from the bullseye. - Accurate but not Precise: Darts close to the bullseye but scattered. - Neither Accurate nor Precise: Darts scattered and far from the bullseye. --- Improving Accuracy and Precision in Studies 1. Reduce Systematic Errors - Use robust study designs and validated tools. - Train data collectors to minimize bias. 2. Enhance Data Collection Techniques - Standardize measurement protocols. - Employ pilot testing to refine methodologies. 3. Leverage Statistical Tools - Apply error correction methods like regression calibration to adjust for inaccuracies. 4. Ensure Comprehensive Quality Control - Conduct periodic checks and recalibrate instruments. --- Summary Accuracy and precision are pivotal in epidemiological research to ensure valid and consistent findings. - Accuracy focuses on closeness to the true value, ensuring the results represent reality. - Precision emphasizes the consistency of repeated measurements. Combining these two elements in study design and execution leads to reliable outcomes, enabling data-driven decisions and effective public health interventions.