MBD Data Viz -- Session 3.pdf
Document Details
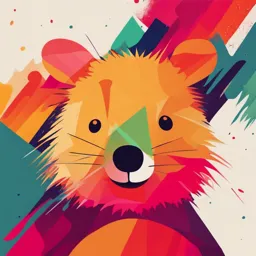
Uploaded by PerfectPanda
IE University
2024
Tags
Full Transcript
Data Visualization MBD Session 3 “There is magic in graphs. The profile of a curve reveals in a flash a whole situation – the life history of an epidemic, a panic, or an era of prosperity. The curve informs the mind, awakens the imagination, convinces.” –Henry D. Hubbard Professor Christina Stathopo...
Data Visualization MBD Session 3 “There is magic in graphs. The profile of a curve reveals in a flash a whole situation – the life history of an epidemic, a panic, or an era of prosperity. The curve informs the mind, awakens the imagination, convinces.” –Henry D. Hubbard Professor Christina Stathopoulos, February 2024 Book of the Day ColorWise by Kate Strachnyi Session 3 Agenda Design Review Color Theory Additional Design Tips Data à to à Viz Design Review Let’s test your understanding How to Improve a Graph What is wrong here? How to Improve a Graph What is wrong here? How to Improve a Graph What is wrong here? How to Improve a Graph What is wrong here? How to Improve a Graph What is wrong here? How to Improve a Graph What is wrong here? How to Improve a Graph What is wrong here? How to Improve a Graph What is wrong here? How to Improve a Graph What is wrong here? How to Improve a Graph What is wrong here? How to Improve a Graph What is wrong here? How to Improve a Graph Much better! Review Marks? Basic visual objects that represent data Channels? Visual variables that we can use to represent characteristics of these marks Pre-Attentive Attributes? Pattern recognition, attributes that our brain unconsciously focuses on Gestalt Principles? Making sense of things by seeing a whole, rather than the individual parts With a partner next to you Go to: - https://lookerstudio.google.com/gallery?category=visualization OR - https://public.tableau.com/app/discover/viz-of-the-day Select different data visualizations & discuss how they are (or are not) using channels, pre-attentive attributes and/or Gestalt Principles appropriately. ~5 Minutes Color Theory The Intentional Use of Color ”..remember, in data visualizations, don’t camouflage the visual and the message you want to get across.” Types of Colors Used in Data Viz Source: Steve Wexler, Jeffrey Shaffer & Andy Cotgreave. The Big Book of Dashboards (2017). Types of Colors Used in Data Viz Use Sequential for the most intuitive reading of a numeric, color scale. Types of Colors Used in Data Viz Use Diverging… If there is a meaningful middle point. To highlight varying extremes. To emphasize the difference between data points. Types of Colors Used in Data Viz Use Categorical for clear differentiation of categorical variables. Types of Colors Used in Data Viz Use Highlight to make your viewers focus on a specific piece. Types of Colors Used in Data Viz Flu Cases over Time Use Alert to warn your viewers of something, typically a negative connotation displayed with the color red. 100K 75K China 50K Germany South Africa 25K Brazil 0 DO – Use Light to Dark for Continuous Data DO – Use Contrasting Colors for Comparison DO – Use Contrasting Colors for Comparison DO – Use your Brand Colors DO – Use your Brand Colors DO – Use your Brand Colors, Create a Palette Brand Palette DO – Include a Color Key DO – Use Semantic Color Association (when possible) DO – Consider Color Psychology DO – Consider Color Psychology What does the color red mean to YOU? Danger = Western Happiness = Chinese Source: Six Degrees. https://www.six-degrees.com/pdf/International-Color-Symbolism-Chart.pdf DON’T – Use Too Many Colors DON’T – Forget Color Blindness Avoid… § § § § § § § § green + red green + brown blue + purple green + blue light green + yellow blue + grey green + grey green + black DON’T – Forget Color Blindness Solution? VIRIDIS color library Make plots that are… Elegant Better represent your data Easier to read for colorblindness Print well in greyscale https://cran.r-project.org/web/packages/viridis/vignettes/intro-to-viridis.html https://www.geeksforgeeks.org/matplotlib-pyplot-viridis-in-python/ DON’T – Use Colors too Hard to Distinguish Color Theory Summary DO § Keep in mind appropriate uses of color § § § § § § for sequential, diverging, categorical, highlight and alert purposes Use light to dark for continuous data Use contrasting colors for comparison Use your brand colors Include a color key Use semantic color association Consider color psychology DON’T § Use too many colors § Use colors too hard to distinguish § Forget color blindness The Power of Color & More “The way a data visualization is designed will convey a particular message. The same data can often be displayed in multiple ways to create different messages.” ~Andy Cotgreave Additional Design Tips Effective Data Visualization Take advantage of the way the mind works when building text or a visual Remember the Z pattern when designing a dashboard. 1 2 3 4 Legends Job Satisfaction 100 Females with salary up to 30,000E Females with salary over 30,000E 75 Males with salary up to 30,000E 50 Males with salary over 30,000E 25 0 Job Satisfaction Females with salary up to 30,000E 100 75 Females with salary over 30,000E 50 Males with salary up to 30,000E 25 Males with salary over 30,000E 0 Before 50 After 50 Legends Job Satisfaction 100 Remember to always consider the Data-Ink Ratio! 75 Males with salary up to 30,000E 50 Males with salary over 30,000E 25 Job Satisfaction Females with salary up to 30,000E Females with salary over 30,000E 0 Remove unnecessary borders Females with salary up to 30,000E 100 Females with salary over 30,000E 75 Males with salary up to 30,000E 50 Males with salary over 30,000E 25 0 Job Satisfaction Job Satisfaction Females with salary up to 30,000E 100 75 50 25 100 Females with salary over 30,000E 75 Males with salary up to 30,000E 50 Males with salary over 30,000E Females with salary up to 30,000E Males with salary over 30,000E Females with salary over 30,000E 25 Males with salary up to 30,000E 0 0 Before 50 After 50 Label directly where possible Before 50 After 50 4 –2 02 2Q An 1 a– 20 22 Jo Q1 rg e –2 02 2Q An 2 a20 22 Q2 Jo rg e An a Jo rg e –2 02 1Q –2 02 1Q 4 Grouping Avoid repeated labels by grouping wherever possible (quarter, year, using legends) Jorge Ana Q4 Q1 2021 Q2 2022 Orientation Flip your bar chart & use longer axis labels Washington, DC Raleigh-Durham, NC San Francisco, CA Miami-Dade, FL Myrtle Beach, SC Hilton Head, SC Miami-Dade, FL San Francisco, CA Raleigh-Durham, NC Washington, DC Hilton Head, SC Jan 2021 Feb 2021 Mar 2021 Apr 2021 May 2021 Jun 2021 Myrtle Beach, SC Always maintain consistency between dimensions & colors Consistency Operations HR 75 Sales Operations HR 75 HR 50 Operations Sales 25 0 HR 50 Operations Sales 25 0 2021 2022 2021 2022 Sales Thresholds < 6 lines < 10 bars 2-5 slices Filter or group more Split into separate charts Filter or group more Add an ‘Other’ category Filter or group more Split into separate charts Data à to à Viz The Right Graph for your Data Taxonomy Intro How to choose the best graph for: Comparing categories Showing hierarchies Analyzing temporal changes Making connections & relationships Mapping geospatial data Comparison – Bar Chart Variables: 1 categorical 1 quantitative Excuses for Being Late to Class I had no clean pants to wear I thought it was Saturday Great for… ü Comparing across categories ü Highlighting differences ü Showing trends & outliers ü Revealing highs and lows I forgot to set my alarm I thought it was still nighttime I got stuck in traffic 0 2 4 6 8 10 Comparison – Stacked Bar Chart Variables: 2 categorical (color, position) 1 quantitative Great for… ü Highlighting differences ü Showing how the total differs over time, and how each piece contributes to the total Careful… ü Bad for showing growth of each variable within (other than the baseline / first variable) (value annotations can help) Comparison – Grouped/Clustered Bar Chart Variables: 2 categorical (color, position) 1 quantitative Great for… ü Highlighting differences ü Precise understanding of how each section / category compares Careful… ü No way to observe what each category represents of total Comparison – Stacked Cumulative Bar Chart Variables: 2 categorical (color, position) 1 quantitative Great for… ü Highlighting how the distribution changes over time ü Showing how each piece contributes to the total Careful… ü Bad for showing growth of each variable within (other than the two baselines at each end) ü No way to understand how totals differ over time Comparison – Area Chart (same concept as Stacked Bar Chart!) Variables: 2 categorical 1 quantitative Great for… ü Highlighting differences ü Showing how the total differs over time, and how each piece contributes to the total Careful… ü Bad for showing growth of each variable within (other than the baseline / first variable) (value annotations can help) Hierarchies – Pie Chart Variables: 1 categorical (color) 1 quantitative-ratio (angle) FOUNDATION OF ANY RELATIONSHIP LIKING THE SAME THING Great for… ü Comparing across few categories, each with significantly varying values Careful… ü Bad for many categories ü Bad for several categories with similar values (value annotations can help) HATING THE SAME THING Hierarchies – Donut Chart Variables: 1 categorical (color) 1 quantitative-ratio (angle) (skip the Pie Chart!) COUNTRIES BY AREA Great for… ü Comparing information across categories in a simplistic way Careful… ü Bad for many categories ü Bad for several categories with similar values (value annotations can help) TIP: Always use a separator (in my case here, white borders) Hierarchies – Pie Chart | Donut Chart Why so much hate? à Less accurate à Harder to interpret. People are much better at comparing length or height, rather than areas. Thinner pieces become unreadable. Difficult to understand the difference between pie slices with similar values. Hierarchies – Circle Packing Diagram Variables: Multiple categorical (color, position) 1 quantitative-ratio (area) Great for… ü Showing distribution and partto-whole in very large datasets Careful… ü Don’t over-do, it’s typically used more for ‘show’ or the wow factor ü Uses space less efficiently than a treemap Hierarchies – Treemap Variables: Multiple categorical (color, position) 1 quantitative-ratio (area) Great for… ü Showing distribution and partto-whole in very large datasets ü Uses space more efficiently than a circle packing diagram Careful… ü Don’t over-do it - too many categories, especially small ones, can quickly get overwhelming ü Category names can easily get cut off and thus become unreadable Lunch Breakfast Time Series – Line Chart Variables: 1 categorical (color) 1 quantitative-interval 1 quantitative-ratio Great for… ü Viewing trends, usually over time (can also be over time intervals and other ordinal data) Careful… ü Use too many lines and it becomes a ‘spaghetti chart’ TIP: Combine a line graph with a bar chart, they go well together if used correctly! Time Series – Line Chart (vs a Target) Variables: 1 categorical (color) 1 quantitative-interval 1 quantitative-ratio Great for… ü Viewing trends, usually over time (can also be over time intervals and other ordinal data) Careful… ü Use too many lines and it becomes a ‘spaghetti chart’ Source: Roshaan Khan TIP: Combine a line graph with a bar chart, they go well together if used correctly! Temporal – Spark Lines Variables: 2 quantitative-interval (can show multiple) 1 quantitative-ratio (can show multiple) Great for… ü Quickly being able to see seasonal trends or economic cycles ü Quick to read and draw overall conclusions Careful… ü Can’t see the difference between trends that are only slightly different Relationships – Scatterplot Variables: 2 quantitative Great for… ü Investigating a relationship between different variables ü Showing correlations ü Often used in regression analysis TIP: Customize points to TIP: Add a trend line to show highlight where you want the correlation user to focus Relationships – Bubble Plot Variables: 2-3 quantitative 1-2 categorical Great for… ü Showing large amounts of information at once TIP: Use color as an extra dimension to visualize an additional category TIP: Overlay on a map when using geographically-related data Relationships – Heat Map Variables: 1 quantitative Multiple categorical (x and y axis) Great for… ü Using color to highlight the progress of data points, usually over time TIP: Use a ‘highlight table’ that includes the precise figures when needed Source: Roshaan Khan Mapping – (Geospatial) Discrete Choropleth Map Variables: Geo data Great for… ü Visualizing data connected to country names, postal codes, states, etc Careful… ü You may need to take population / density into account TIP: Continuous scales look visually appealing but harder to read, stick to bucketed discrete groups (although loses some detail) Source: Claus O Wilke. https://clauswilke.com/dataviz/geospatial-data.html, Original Data Source: 2015 Five-Year American Community Mapping – (Geospatial) Discrete Choropleth Map Variables: Geo data Great for… ü Visualizing data connected to country names, postal codes, states, etc Careful… ü You may need to take population / density into account ü Large or small geo sizes can distort Source: Claus O Wilke. https://clauswilke.com/dataviz/geospatial-data.html, Original Data Source: 2015 Five-Year American Community Mapping – (Geospatial) Cartogram Heatmap Variables: Geo data Great for… ü Visualizing data connected to country names, postal codes, states, etc Careful… ü Does not correct for population or consider size, treats all geos equally Source: Claus O Wilke. https://clauswilke.com/dataviz/geospatial-data.html, Original Data Source: 2015 Five-Year American Community Mapping – (Geospatial) Individual-Plot Cartogram Variables: Geo data Great for… ü Visualizing data connected to country names, postal codes, states, etc Careful… ü Does not consider population or size, treats all geos equally Source: Claus O Wilke. https://clauswilke.com/dataviz/geospatial-data.html, Original Data Source: US Bureau of Labor Statistics Mapping – (Geospatial) Bubble Map Variables: Geo data Great for… ü Visualizing data connected to country names, postal codes, states, etc ü Considering 3 variables (location, size, category) Careful… ü Can become overwhelming or unreadable in densely populated areas This legend and coloring could be improved. It’s a lot to remember, isn’t it? https://www.data-to-viz.com/ https://www.python-graph-gallery.com/ https://r-graph-gallery.com Individual Assignment Perform exploratory analysis on the data of your choosing to create data visualizations for each of the core situations: (1) comparing categories, (2) showing hierarchies, (3) analyzing temporal changes, (4) making connections & relationships and (5) mapping geospatial data. Must be completed in Looker Studio. Make note of why that is the best visualization for the data and any relevant design elements that you used (e.g. Pre-attentive attributes, Gestalt, etc.). DUE: March 5. It must be uploaded to the designated assignment block by midnight on the day before Session 10. NOTE: You can use one, large dataset that can cover every purpose OR multiple datasets aligned with different visualizations. Reminder! Data Viz leader presentations next class Group Assignment Create ONE slide about a data visualization leader past or present. Be prepared to explain the work that person has done in the field & highlight one interesting thing you learned from them or a powerful data visualization piece of theirs. Delivery: Very quick presentation, ~3minutes. DUE: To be presented in Session 4. It must be uploaded to the designated assignment block by midnight on the day before Session 4. Only one person from your group needs to upload it. The world is one big data problem. Feel free to stay in touch! Any questions? What are you going to do about it?