Materials e Methods (Part 5) - RNA-Sequencing Methods & Technique [Corrected 2].docx
Document Details
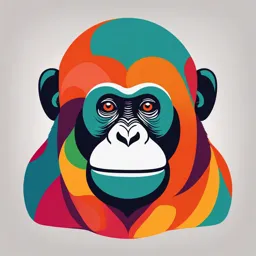
Uploaded by DiligentPolynomial
Open University
Tags
Full Transcript
**MATERIALS AND METHODS** **[5 RNA-Sequencing: Methods & Technique]** RNA-sequencing (RNA-Seq) is a remarkable technique researchers use in genomics and molecular biology to obtain a complete profiling of the entire transcriptome. The methodology applied is based on the identification and quantifi...
**MATERIALS AND METHODS** **[5 RNA-Sequencing: Methods & Technique]** RNA-sequencing (RNA-Seq) is a remarkable technique researchers use in genomics and molecular biology to obtain a complete profiling of the entire transcriptome. The methodology applied is based on the identification and quantification of the various types of existing RNAs, such as mRNA, non-coding RNA and microRNA, as well as the detection of the mechanism of activation and inhibition of genes and how their expression is regulated. In addition, the methodology allows for differential analyses between the different conditions, comparing the transcripts in order to identify the resulting pathways and biological processes. **[5.1 Sequencing Workflow and Pre-Analysis:]** The first step of the RNA-Sequencing workflow consists of extracting the RNA from samples and converting it into a library of cDNA fragments. Subsequently, the fragments are sequenced to generate millions of short reads, identifying only the determined RNA sequences. This first step prepares the data for subsequent analyses, such as the measurement of the gene expression levels and the identification of transcripts. *5.1.1 RNA Extraction and Library Preparation* It is possible to distinguish different phases in the RNA extraction and library preparation procedure. The protocol used in our laboratory analysis is the \"TruSeq Stranded Total RNA (Low Sample)\"^1^ : 1. **RNA Extraction**: This phase consists of extracting total RNA from the biological samples. Depending on the objective of the analysis, it is possible to identify messenger RNAs, non-coding RNAs and other types of RNAs. After the extraction process, the quality of the RNA is evaluated using a Bioanalyzer with a specific RNA chip. 2. **Selection and Enrichment of RNA**: Depending on the research activity, it is necessary to select specific subpopulations of RNA, such as mRNA, which constitutes the perfect example of study for protein-coding genes. The mRNA selection process requires the use of magnetic beads coated with poly-T oligo-nucleotides, which are nothing more than short sequences of T-thymines. These sequences are complementary to the adenine tail (polyA) present at the 3\' end of the mRNA, to which they are then mixed to allow binding by complementarity. The separation occurs consecutively thanks to the application of a magnetic field, which attracts the magnetic beads linked to the mRNA to be extracted and subsequently through an elution process involving the application of a solution that allows the purified mRNA to be recovered. This step is necessary as it removes the cytoplasmic ribosomal RNA. 3. **Conversion to cDNA**: In general, the transcriptome (RNA) is relatively unstable and it cannot be sequenced directly. Therefore, it must first be converted into complementary DNA (cDNA) using various enzymes. In particular, the first enzyme is *Reverse Transcriptase*, which converts messenger RNA (mRNA) into a single strand of complementary DNA (cDNA). This enzyme uses random primers (short sequences of random nucleotides) to initiate DNA synthesis. Random primers perform their function by binding to random sites along the RNA strand, indicating a starting point for the action of reverse transcriptase and ensuring broad coverage of the RNA regions to increase the representativeness of the cDNA produced. The second step involves the *Ribonuclease H enzyme*, which degrades the original RNA strand, as it is necessary to clean and filter the residual RNA from the remaining hybrids, leaving only the cDNA strand. The third and final step involves *DNA polymerase*, which is necessary to synthesize the second and complementary cDNA strand. 4. **Preparation of the Library**: The library is prepared by processing the cDNA, which is fragmented into smaller parts. Sequence-specific adapters (adenine bases) are added to the ends of each fragment. This is necessary to trigger the ligation of adapters, i.e., binding of the cDNA to the sequencing platform and amplification. 5. **Purification Quality Assessment**: PCR purifies and amplifies the fragments to create the final cDNA library. PCR amplifies only fragments with adapters on both ends, using \"cocktail primers\" that bind exactly to these ends to increase the amount of genetic material. To obtain high-quality data, an excellent cluster density is necessary, which involves an accurate quantification of the DNA library template. 6. **Sequencing**: The final step is loading of the library onto the sequencing platform, which, in our case, is the "Illumina Next-Seq 500" system. The cDNA fragments are sequenced to produce reads that represent the transcripts present in the original sample. *5.1.2 Illumina NGS Process Workflow* The main steps of Illumina NGS sequencing are the same for both DNA and RNA, and are shown below^2^: A. **Library Preparation**. The library is prepared by random fragmentation of the DNA or cDNA sample, followed by adapter ligation at the fragments\' 5\' and 3\' ends (Fig. 1 A), as described in the previous paragraph. B. **Cluster Generation**. The cluster generation phase takes place inside the Flow-Cell, a small cartridge containing several channels called \"lanes\" through which the DNA flows. The presence of the \"lanes\" allows the loading of several samples to be sequenced simultaneously, guaranteeing the parallelization of the sequencing process. After loading the DNA library inside the Flow-Cell, the DNA fragments bind to the complementary oligonucleotides fixed on the cell surface, corresponding to the fragments\' adapters. Bridge Amplification follows this initial ligation process and consists of a particular amplification that takes place *in situ*, similar to PCR (Polymerase Chain Reaction), directly on the surface of the flow cell. In this phase, each DNA fragment folds, creating a \"bridge\" that can bind to a further complementary oligo-nucleotide. This process is repeated multiple times in order to create several thousand copies of each fragment for each single cluster, which are physically separated from each other. A high number of identical copies of the original fragment in each cluster is essential to obtain a sequencing signal that is precise and clear, especially in the read phase (Fig. 1B). C. **Sequencing**. Sequencing occurs inside the flow-cell through the Sequencing by Synthesis (SBS) process specific to Illumina technology. In particular, each DNA cluster is sequenced by adding specific nucleotides that are chemically modified and labelled with a different fluorochrome. The identification of each added base occurs thanks to the light associated with a specific wavelength emitted by each fluorochrome. A \"terminator\" is added to each nucleotide in succession to temporarily prevent the addition of further nucleotides and to allow sequential base-by-base reading. This terminator is then eliminated in each incorporation cycle to allow DNA synthesis in such a way as to allow the capture of the emitted fluorescence signal, which is detected and recorded by an imaging system. These signals are then translated into base sequences (A, T, C, G) representative of the sample\'s genomic sequence (Fig. 1C). D. **Data Analysis**. For the purposes of data analysis, the reads of the identified sequences are aligned to a reference genome (Fig. 1 D). After alignment, the data are imported into tools or software in order to implement a pipeline for analysis.  **[5.2 RNA-Sequencing Pre-Processing phase:]** The pre-analysis steps involve quality control checks to ensure the integrity and usability of the data, including the removal of low-quality reads and the alignment of sequences to a reference genome. *5.2.1 Multiplexing and Demultiplexing* Over the years, the amount of data has increased, particularly regarding NGS experiments. This increase requires sequencing of a larger number of samples and a larger number of libraries in the shortest possible time. In particular, Multiplexing is a technique that allows grouping of different DNA or RNA libraries to be sequenced in a single run (Fig. 2). With multiplexed libraries, unique index sequences are added to each DNA fragment during library preparation to identify and sort each read before final data analysis. The principal advantage is a considerable reduction in analysis times. On the other hand, this time gain results in an added level of complexity to the sequenced reads. Consequently, this involves the need to identify and order the sequenced reads computationally through the Demultiplexing process before proceeding with the final data analysis^2^. Demultiplexing is a crucial step in Next-Generation Sequencing (NGS) analysis, inherent to the data pre-processing phase. In fact, the grouping and parallel processing of multiple samples in a single run (Multiplexing) involves sorting the sequencing reads in the respective samples. This process is possible thanks to the unique index sequences added to the samples during library preparation. The high number of samples and sequences present characterizes the complexity of Demultiplexing, as it requires the use of complex computational techniques and methods to precisely identify and assign millions of reads to the correct samples. To this purpose, the \"bcl2fastq\" tool, developed by Illumina, is often used. Bcl2fastq transforms Binary Base Call (BCL) files, which are the raw output of Illumina sequencing devices, to the more accessible FASTQ format^3^. FASTQ files, which include both nucleotide sequences and quality ratings, permit downstream bioinformatics analyses. During the conversion, \"bcl2fastq\" also demultiplexes, using the index information to assign each read to the correct sample. The software \"bcl2fastq\" commonly works by setting the relative commands into the bash terminal^4^. The program needs input folders holding the BCL files as well as the sample sheet, which contains information on the index sequences used in each sample. In addition, users provide an output path for the demultiplexed FASTQ files. The application offers numerous customization options for the conversion process, such as adjusting the permissible number of mismatches in index sequences or handling compressed BCL files, making it a versatile tool in the NGS data processing pipeline. *5.2.2 FASTQ file quality control* After the Demultiplexing phase, evaluating the quality of the generated FASTQ Files is an essential and advisable step. This is possible through FastQC, an informatic tool widely used in the field of genomics and bioinformatics, which allows the evaluation of the quality of NGS experiments^5^. Many formats are accepted as input, including BAM, SAM, and FASTQ Files, which are also obtained from different experiments and sequencing platforms. The advantage of this program lies in the possibility of accurately and immediately identifying troubles present in the sequence data and implementing a preliminary evaluation before applying a more detailed analysis. A further positive aspect is the modular structure, where different outputs from parameter analyses structured in modules are collected. These include, for example, evaluations of sequence quality scores, assessment of GC content, evaluation of sequence duplication levels and overrepresented sequences. FastQC illustrates the results with the use of graphs and summary tables, which offer a generic and concise overview of the data and allow the user to easily identify and access files and sections characterized by poor quality. In addition, these results are provided by reports in HTML format. Unlike similar tools used in the field, FastQC is flexible and permits quality analyses interactively or offline. This tool allows automation of the processing procedures. The implementation of FastQC into Java is an aspect that should not to be underestimated, as it gives rise to vast compatibility among the various operating systems. *5.2.3 Alignment of the RNA-Seq Data* During this stage, we matched the paired-end reads obtained from the RNA-Seq experiment with the reference genome using alignment. We utilized the reference genome HG38 (GRCh38.p12), which is a comprehensive digital repository of nucleic acid sequences. HG38 was methodically compiled by researchers and scientists to serve as a representative model of the whole set of genes found in the human species (Homo sapiens). It is possible to access the data using the dedicated servers of UCSC Genome Browser and Ensembl. Aligning high-throughput sequencing (HTS) generated datasets of big reads to a reference genome is a crucial step in the processing of RNA-Seq data^6^. The sequenced reads consist of microscopic fragments of 150 base pairs, which is far less than the typical size of human genes (24 kilobase pairs). Due to factors, such as the potential existence of deletions, insertions, mismatches, and sequencing mistakes, the alignment of these reads with various genomic regions might be misleading. Therefore, we performed the alignment using STAR (Spliced Transcript Alignment to a Reference), a specialized sequence aligner that is tailored to align non-contiguous sequences directly to the reference genome^6^. STAR outperforms other aligners in terms of mapping speed, sensitivity and alignment correctness. It is possible to distinguish two types of approaches in the Illumina Next-Generation Sequencing: \- **Single-Read Sequencing**, also known as single-end sequencing, is a method permitting sequencing of the DNA from just one end of each DNA fragment. \- **Paired-End Sequencing** (PE) allows sequencing of both ends of the DNA fragment. Typically, PE sequencing results in a greater quantity of SNV calls after read-pair alignment. Although some techniques, including short RNA sequencing, are more suitable for single-read sequencing, currently, the majority of researchers chooses the paired-end strategy. Alignment algorithms may efficiently map readings across repeated portions using the known distance between each paired read. This results in better alignment of reads, especially in repetitive, difficult-to-sequence regions of the genome. The STAR method comprises two distinct phases: the seed search phase and the clustering/stitching/scoring phase. 1. **Seed search**: The primary concept behind the STAR seed discovery phase is the systematic search for a Maximal Mappable Prefix ([MMP]{.math.inline}). The [MMP]{.math.inline} of a read sequence [*R*]{.math.inline} at position [*i*]{.math.inline}, with respect to a reference genome sequence [*G*]{.math.inline}, is defined as the longest substring [(*R*~*i*~,*R*~*i* + 1~,...,*R*~*i* + *MML* − 1~)]{.math.inline} that matches one or more substrings of [*G*]{.math.inline}, where [MML]{.math.inline} is the maximum length that may be mapped. Initially, the method identifies [MMP]{.math.inline} beginning from the first base of the read. When a splice junction is present, the read cannot be mapped to the genome continuously. As a result, the first seed is mapped to a donor splice site. Subsequently, the [MMP]{.math.inline} search is reiterated for the unaligned segment of the sequence, which, in this particular scenario, will be aligned with an acceptor splice site. Splice junctions are identified in a single alignment process without any prior knowledge of their locations or characteristics, and without the necessity for a preliminary alignment pass required by junction database methods. The [MMP]{.math.inline} search is conducted in both the forward and backward directions of the read sequence^6^. 2. **Clustering, Stitching and Scoring**: During the second step of the algorithm, STAR constructs alignments of the complete read sequence by connecting all the seeds aligned to the genome in the initial phase. Initially, the seeds are grouped together based on their closeness to a certain set of "anchor" seeds. The size of genomic windows dictates the upper limit for the intron size in the spliced alignments. This technique allows for unlimited number of mismatches, but only permits one insertion or deletion (gap). It is important to highlight that the seeds from the mates of paired-end RNA-seq reads are clustered and stitched together at the same time. Each paired-end read is treated as a single sequence, which allows for the possibility of a chromosomal gap or overlap between the inner ends of the mates. The primary method for utilizing the paired-end information is by acknowledging its ability to represent the nature of the paired-end accurate reads, namely the fact that the mates are fragments (ends) of the same sequence. By employing this strategy, the algorithm sensitivity is enhanced, as a single accurate anchor from either mate is sufficient to precisely align the whole read^6^. In the stitching phase, a local alignment scoring method is used to guide the process. This scheme incorporates user-defined scores, or penalties, for matches, mismatches, insertions, deletions, and splice junction gaps. This permits a quantitative evaluation of the alignment quality and rankings. The stitched combination with the greatest score is selected as the optimal alignment of a read. For multi-mapping readings, alignments having scores within a specific range chosen by the user and lower than the highest score are provided^6^. The STAR command aligns sequencing reads to a reference genome in the context of RNA-Seq data processing. Usually, in a Bash environment, the input files holding the RNA-Seq reads, the reference genome directory and the path to the STAR program must be provided. A fundamental STAR command may read like this: \ [**STAR** **−** **−** **genomeDir** **/path/to/genomeDir** **−** **−** **readFilesIn** **/path/to/read1.fastq** **/path/to/read2.fastq** **−** **−** **runThreadN** **NumberOfThreads**]{.math.display}\ The indexed reference genome directory is specified by \--genomeDir; the paths to the paired-end read files (in the case of single-end reads, only one file is specified) are followed by \--readFilesIn; and the alignment process can be accelerated by setting the number of threads for parallel computing with \--runThreadN. Counting the reads mapping the exon of each identified gene, the \--quantMod GeneCounts option in the STAR program yields gene-level expression quantification.  Although a STAR run for RNA-Seq data produces several files, the aligned read sequences are included in the files Aligned.out.sam, Aligned.out.bam, or Aligned.out.tab. These files depend on later investigations, including the determination of splice variants or the measurement of the gene expression levels. The Log.final.out file is especially significant, as it sheds light on the alignment and sequencing data quality and it offers summary statistics of the alignment process, including the proportion of uniquely mapped reads. **[5.3 RNA-Sequencing Post-Processing phase:]** Following initial processing, the emphasis switches to employing \"Differential Expression Analysis\" using DESeq2 and \"Gene Set Enrichment Analysis\" (GSEA) to explore pathways of biological significance. *5.3.1 Differential Expression Analysis with DESeq2* In bioinformatics, differential expression analysis using DESeq2 is the predominant algorithm for detecting variations in gene expression levels across samples under different conditions. This includes analyses specifically intended to elaborate data obtained from sequencing studies, including RNA-seq, as indicated in the Bioconductor software package DESeq2. DESeq2 works by statistically comparing gene expression variations between experimental groups. Raw counts are the first type of data implemented in the process, and they indicate the number of reads mapped to every gene in every sample. These data must be specifically prepared to guarantee the correct representation of the gene expression levels. The DESeq2 algorithm takes into account the heterogeneity of gene expression data. This involves the application of a negative binomial distribution to model the read-counts before carrying out subsequent operations, such as enrichment analyses or differential expression. This method is very advantageous and effective as the nature of the data used as inputs is typically discrete, with a strong correlation between variance and mean, another innate characteristic of this type of data. Differential analysis is carried out using the variables representative of the experimental conditions examined, adjusting any variations in the size of the library between the samples to provide unbiased comparisons^7^. One peculiar aspect of the analysis using DESeq2 concerns the estimation of dimensional factors, which, as mentioned above, permits to compensate for the variations caused by the sequencing depth or the size of the library between samples. Indeed, the main aim is to guarantee that variations in gene expression are due exclusively (where possible) to biological differences rather than to batch-effects caused by technological or other discrepancies. In addition, DESeq2 allows setting of the testing hypotheses, based on changes in gene expression. In particular, the most commonly used tests, especially for most queries and experimental designs, are the Wald test and the Likelihood Ratio Test (LRT). Usually, pairwise comparisons are performed with the Wald test, while complex experimental designs with many components may benefit from LRT. The DESeq2 output provide p-values for every gene after statistical testing, which indicates the probability that the observed expression difference is the result of chance. False positives are less likely when these p-values are adjusted for multiple testing, often using the Benjamini-Hochberg method. Lists of differentially expressed genes are part of the DESeq2 outcomes, using the log2(Fold-Changes), which quantifies the size and impact of changes in gene expression. Visualization techniques like MA plots, volcano plots, and heatmaps may be used to get views on the overall structure of the data and specific patterns of gene expression^8^. *5.3.2 Gene Set Enrichment Analysis (GSEA)* Measuring the amounts of DNA, RNA, and proteins in biological samples has become a standard procedure. This results in a substantial volume of data allowing researchers to investigate new biological functions, correlations between genotypes and phenotypes, and processes of diseases^9,10^. The current difficulty is interpreting the outcomes in order to acquire knowledge of biological systems. To address these analytical difficulties, we may use the route enrichment analysis, which typically consists of three main phases^11^. Initially, a selection of genes that are of particular interest is established utilizing omics data. For example, RNA-seq data might provide a list of genes that are expressed differently in different conditions^12^. Furthermore, a statistical methodology permits the discovery of pathways that have a higher level of enrichment in the gene list, relative to what is caused by random chance. The gene list is evaluated for enrichment in all pathways included in a certain database. There are several pathway enrichment analysis methods that may be used, and the selection relies on the nature of the gene list, *i.e.* whether it is ranked (e.g., GSEA, Camera) or not (e.g., g:Profiler)^13^. **Gene Set Enrichment Analysis** (**GSEA**) is a computational method used for the interpretation of gene expression data. This robust analytical approach centers on a group of genes that is determined based on pre-existing biological knowledge, such as published data on biochemical pathways, co-expression analysis or differential expression analysis results. The GSEA method requires an expression data matrix (*D*) of *k* samples including *N* genes, a ranking mechanism or correlation, and a phenotype of interest *C*. It also needs an exponent *p* to define the weight of each step and an independently created gene set *S* consisting of *N~H~* genes. The program then generates the gene list *L*. First, the method arranges the *N* genes according to their correlation (*r~j~*) with the phenotype of interest, resulting in the set L= {*g*~1~,\...*,g~N~*}. Subsequently, for each place in the sorted list of genes, it calculates the proportion of genes in the gene set *S* that are present (\"hits\") or not present (\"misses\") at that position^11^.  The ES represents the highest amount by which the difference between *Phit* and *Pmiss* deviates from zero (*Phit- Pmiss*). If the genes included in S are highly concentrated at either the top or bottom of the list, the value of ES(S) are correspondingly high. Typically, the value of p is equal to 1. Therefore, the genes in S are assigned weights based on their correlation with C and then normalized by dividing the total of correlations across all genes in S. To assess the importance of the Enrichment Score (ES), the GSEA algorithm conducts 1000 permutations of the phenotypic labels and recalculates the ES for the gene set using the permuted data. This process generates a null distribution for the ES. The nominal p-value is determined with the use of the positive or negative segment of the null distribution corresponding to the sign of the observed effect size (ES). When evaluating a whole database of gene sets, the algorithm takes into consideration multiple hypothesis testing and modifies the calculated p-value accordingly. Initially, the enrichment score (ES) is calculated for every gene set in the collection. Next, the computation of ES(S,π) is performed for each S and 1000 predetermined permutations π of the phenotypic labels. The ES and ES(S,π) values are adjusted for each gene set to consider the size of the set, resulting in normalized enrichment scores NES(S) and NES(S,π). The false discovery rate (FDR) is calculated by adjusting the ratio of false positives to the total number of gene-sets, while maintaining a constant level of significance (often 0.05), for both positive (negative) NES(S) and NES(S,π)^11^. In the case in question, the GSEA relies on the sets of annotated genes obtained from the MSigDB, which is the predominant database of gene-sets^14^. Currently, it has over 10,000 annotated gene sets for humans and mice^15^, which include a diverse range of biological processes and diseases^14^. The Correlation adjusted Mean Rank (**Camera**) is a gene expression analysis method that takes into account the inter-gene correlation structure of the data. This method greatly improves the ability to detect gene sets that are differentially expressed, leading to improved power and accuracy in identifying differential biological pathway^16^. The workflow begins by choosing a particular gene-set or pathway, and then calculating the covariance matrix of the genes within that pathway. The covariance matrix undergoes an iterative process to consider the relationship between different genes. This process yields a new set of variables that are independent from one another. Subsequently, Variance Inflation Factors (VIFs) are computed for every gene inside the route. Variance inflation factors (VIFs) are used to address the possibility of multicollinearity between a certain gene and other genes in the pathway, excluding any possible bias o influence. Afterwards, the test statistics for global and gene-specific analyses are calculated using the rotated covariance matrix and the weights adjusted for VIF. The global test statistics quantifies the overall relevance of the pathway, while the gene-specific test statistics asses the significance of each gene inside the pathway. Ultimately, the significance of the test statistics is determined by a method based on permutations. For each permutation, the test statistics are computed after randomly rearranging the phenotypic labels of the samples. The p-value is determined by calculating the fraction of permutation test statistics that are equal to or more extreme than the observed test statistic. **[BIBLIOGRAPHY:]** 1\. Waern, K., Nagalakshmi, U. & Snyder, M. RNA Sequencing. in *Yeast Systems Biology: Methods and Protocols* (eds. Castrillo, J. I. & Oliver, S. G.) 125--132 (Humana Press, Totowa, NJ, 2011). doi:10.1007/978-1-61779-173-4\_8.2. Illumina Inc. Illumina sequencing introduction. *Illumina Seq. Introd.* 1--8 (2017) doi:http://www.illumina.com/content/dam/illumina-marketing/documents/products/illumina\_sequencing\_introduction.pdf.3. Illumina. Demultiplexing. *Demultiplexing (Illumina)* https://support-docs.illumina.com/SW/ClarityLIMS/ClarityAPI/Content/SW/ClarityLIMS/API/Demultiplexing\_swCL.htm (2013).4. Biowulf. bcl2fastq on Biowulf. *Biowulf - High Performance Computing at the NIH* https://hpc.nih.gov/apps/bcl2fastq.html.5. Andrews, S. Babraham Bioinformatics - FastQC A Quality Control tool for High Throughput Sequence Data. https://www.bioinformatics.babraham.ac.uk/projects/fastqc/ (2010).6. Dobin, A. *et al.* STAR: Ultrafast universal RNA-seq aligner. *Bioinformatics* **29**, 15--21 (2013).7. Love, M., Anders, S. & Huber, W. Analyzing RNA-seq data with DESeq2. *Bioconductor* **2**, 1--63 (2017).8. Love, M., Anders, S. & Huber, W. *Package ' DESeq2 '*. (2013).9. Lander, E. S. Initial impact of the sequencing of the human genome. *Nature* **470**, 187--197 (2011).10. Stephens, Z. D. *et al.* Big Data: Astronomical or Genomical? *PLoS Biol.* **13**, e1002195 (2015).11. Subramanian, A. *et al.* Gene set enrichment analysis: A knowledge-based approach for interpreting genome-wide expression profiles. *Proc. Natl. Acad. Sci.* **102**, 15545--15550 (2005).12. Anders, S. *et al.* Count-based differential expression analysis of RNA sequencing data using R and Bioconductor. *Nat. Protoc.* **8**, 1765--1786 (2013).13. Reimand, J. *et al.* Pathway enrichment analysis and visualization of omics data using g:Profiler, GSEA, Cytoscape and EnrichmentMap. *Nat. Protoc.* **14**, 482--517 (2019).14. Liberzon, A. *et al.* The Molecular Signatures Database (MSigDB) hallmark gene set collection. *Cell Syst.* **1**, 417--425 (2015).15. GSEA. https://www.gsea-msigdb.org/gsea/index.jsp.16. Wu, D. & Smyth, G. K. Camera: a competitive gene set test accounting for inter-gene correlation. *Nucleic Acids Res.* **40**, e133 (2012).