c1276997636adb94bb9de6c8bb99f4b0.jpeg
Document Details
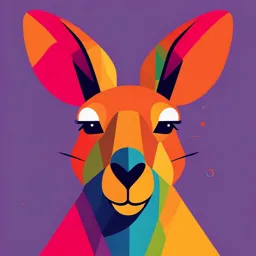
Uploaded by QuaintNarwhal5588
University of Perpetual Help System DALTA
Full Transcript
# Lecture 7: October 10, 2023 ## Quick Overview ### The Game Plan 1. Briefly discuss PS3 solutions. 2. Discuss non-parametric tests. 3. Start talking about experimental design. ### PS3 * Common issues: * The bias of $\hat{\sigma}^2$ when $\mu$ is unknown. * Confidence interval...
# Lecture 7: October 10, 2023 ## Quick Overview ### The Game Plan 1. Briefly discuss PS3 solutions. 2. Discuss non-parametric tests. 3. Start talking about experimental design. ### PS3 * Common issues: * The bias of $\hat{\sigma}^2$ when $\mu$ is unknown. * Confidence interval coverage. * Visualizations: always label your axes! ## Non-Parametric Statistical Tests ### What are they? * Statistical tests that make weaker assumptions than t-tests, ANOVAs, etc. * Do **not** assume that the data are normally distributed. * Do **not** assume that the variance is homogeneous across groups. ### Why use them? * Your data is severely non-normal. * Although t-tests and ANOVAs are robust to violations of normality, at large deviations from normality, non-parametric tests may be more appropriate. * Your data are inherently ranks/ordered (e.g. Likert scales). * You have outliers that exert a strong influence on the outcome of parametric tests. ### Examples * Sign test * Wilcoxon signed-rank test * Mann-Whitney U test * Kruskal-Wallis test * Friedman test ## The Sign Test ### Idea * We have a paired design. * $H_0:$ the median difference between pairs is zero. * For each pair, we record the sign of the difference. * Under $H_0$, we should see roughly 50% positive and 50% negative signs. * We can use a binomial test to see if the proportion of positive/negative signs differs significantly from 0.5. ### Example | Subject | Condition A | Condition B | Sign of Difference | | :-------: | :-----------: | :-----------: | :------------------: | | 1 | 10 | 12 | + | | 2 | 15 | 13 | - | | 3 | 11 | 14 | + | | 4 | 13 | 16 | + | | 5 | 12 | 11 | - | * Of the 5 subjects, 3 have a positive difference and 2 have a negative difference. * Is this enough evidence to reject the null hypothesis that the median difference is zero? * `binom.test(3, 5, p = 0.5)` * p-value = 1 * Do not reject the null hypothesis. * So we would conclude "There is no evidence that Condition A and Condition B are different." * **Note**: This is not necessarily the same as saying "Condition A and Condition B are the same." ## Wilcoxon Signed-Rank Test ### Idea * Like the sign test, but takes into account the magnitude of the differences. * $H_0:$ the median difference between pairs is zero. * For each pair: * We record the sign of the difference. * We rank the absolute value of the differences from smallest to largest. * We sum the ranks of the positive differences and the ranks of the negative differences. * Under $H_0$, the sums of the positive and negative ranks should be roughly equal. * We use a specialized table or software to determine if the difference between the sums of the ranks is large enough to reject $H_0$. ### Example | Subject | Condition A | Condition B | Difference | Absolute Difference | Rank | Signed Rank | | :-------: | :-----------: | :-----------: | :----------: | :-------------------: | :--: | :-----------: | | 1 | 10 | 12 | 2 | 2 | 1 | 1 | | 2 | 15 | 13 | -2 | 2 | 1 | -1 | | 3 | 11 | 14 | 3 | 3 | 3 | 3 | | 4 | 13 | 16 | 3 | 3 | 3 | 3 | | 5 | 12 | 11 | -1 | 1 | 5 | -5 | * Sum of positive ranks: 1 + 3 + 3 = 7 * Sum of negative ranks: (-1) + (-5) = -6 * Do these sums differ enough to reject the null hypothesis? * `wilcox.test(x, y, paired = TRUE)` * p-value = 0.625 * Do not reject the null hypothesis. * So we would conclude "There is no evidence that Condition A and Condition B are different." ### How to handle ties * Assign the average rank to the tied values. * In the previous example, subjects 1 and 2 both has absolute difference of 2, so they are assigned the average rank of 1.5. | Subject | Condition A | Condition B | Difference | Absolute Difference | Rank | Signed Rank | | :-------: | :-----------: | :-----------: | :----------: | :-------------------: | :--: | :-----------: | | 1 | 10 | 12 | 2 | 2 | 1.5 | 1.5 | | 2 | 15 | 13 | -2 | 2 | 1.5 | -1.5 | | 3 | 11 | 14 | 3 | 3 | 3 | 3 | | 4 | 13 | 16 | 3 | 3 | 3 | 3 | | 5 | 12 | 11 | -1 | 1 | 5 | -5 | * Sum of positive ranks: 1.5 + 3 + 3 = 7.5 * Sum of negative ranks: (-1.5) + (-5) = -6.5 * `wilcox.test(x, y, paired = TRUE)` * p-value = 0.625 * Do not reject the null hypothesis. * So we would conclude "There is no evidence that Condition A and Condition B are different." ## Mann-Whitney U Test ### Idea * An independent samples test that does not assume normality or homogeneity of variance. * $H_0:$ the two groups have the same distribution. * We rank all the data from smallest to largest, regardless of group membership. * We sum the ranks for each group. * If the groups have the same distribution, then the sums of the ranks should be roughly equal. * We use a specialized table or software to determine if the difference between the sums of the ranks is large enough to reject $H_0$. ### Example | Subject | Group | Value | Rank | | :-------: | :-----: | :-----: | :--: | | 1 | A | 10 | 1 | | 2 | A | 12 | 3 | | 3 | A | 14 | 5 | | 4 | B | 11 | 2 | | 5 | B | 13 | 4 | | 6 | B | 15 | 6 | * Sum of ranks for Group A: 1 + 3 + 5 = 9 * Sum of ranks for Group B: 2 + 4 + 6 = 12 * Do these sums differ enough to reject the null hypothesis? * `wilcox.test(x, y)` * p-value = 0.5476 * Do not reject the null hypothesis. * So we would conclude "There is no evidence that Group A and Group B are different." ## Kruskal-Wallis Test ### Idea * An extension of the Mann-Whitney U test to more than two groups. * $H_0:$ all groups have the same distribution. * We rank all the data from smallest to largest, regardless of group membership. * We sum the ranks for each group. * If the groups have the same distribution, then the sums of the ranks should be roughly equal. * We use a specialized table or software to determine if the difference between the sums of the ranks is large enough to reject $H_0$. * A significant Kruskal-Wallis test indicates that at least one group differs from the others. * **Post-hoc tests are needed to determine which groups differ from each other.** ## Friedman Test ### Idea * A non-parametric alternative to the repeated measures ANOVA. * $H_0:$ all conditions have the same distribution. * For each subject, we rank the data from smallest to largest across conditions. * We sum the ranks for each condition. * If all conditions have the same distribution, then the sums of the ranks should be roughly equal. * We use a specialized table or software to determine if the difference between the sums of the ranks is large enough to reject $H_0$. * A significant Friedman test indicates that at least one condition differs from the others. * **Post-hoc tests are needed to determine which conditions differ from each other.**