Quantitative Methods in Empirical Economic Geography PDF
Document Details
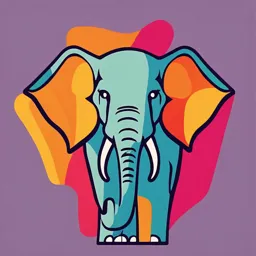
Uploaded by SleekFreeVerse
Leibniz Universität Hannover
Christian Hundt, Kerstin Nolte
Tags
Summary
This document provides lecture slides on quantitative methods in empirical economic geography, specifically focusing on social network analysis. The slides cover topics such as network analysis basics, data collection methods including primary and secondary data sources, and different types of networks. It also discusses descriptive analysis, including micro, meso, and macro levels. The slides conclude with a section on further reading resources.
Full Transcript
Quantitative Methods in Empirical Economic Geography Social Network Analysis Lecturer: Christian Hundt Slides: Christian Hundt und Kerstin Nolte Institute of Economic and Cultural Geography Leibniz University Hannover M2 – Methods in Empirical Economic Geography...
Quantitative Methods in Empirical Economic Geography Social Network Analysis Lecturer: Christian Hundt Slides: Christian Hundt und Kerstin Nolte Institute of Economic and Cultural Geography Leibniz University Hannover M2 – Methods in Empirical Economic Geography 1 Introduction M2 – Methods in Empirical Economic Geography 2 What is a network? ”Network is a general term for physical infrastructure or patterns of interaction that can be represented as a set of points connected by a set of linkages. The points are known as nodes or vertices, and can represent origins, destinations, and junctions. The linkages are known as links, arcs, or edges, Begriffe sind synonyme and represent connections of some kind among the points.” Kuby et al. (2009) Im Wesentlichen: Punkte und Verbindungen M2 – Methods in Empirical Economic Geography 3 Economic geography and networks ▪ What is the essential feature of a network? ▪ Connections among individuals (people, firms, countries…) →These connections play a key role in (more) recent debates in economic geography Der Kern: kann losgelöst sein von physischen Räumen M2 – Methods in Empirical Economic Geography 4 Economic geography and networks ▪ Importance of connections/ relations between individuals, firms – across space (?) →Social network analysis is an important method in economic geography, especially in the more recent literature Theoretischer Überbau: ▪ Relational economic geography (remember Bathelt & Glückler 2003) ▪ Evolutionary economic geography (Bathelt & Li, 2014; Boschma & Frenken, 2018) ▪ Clusters, Regional Innovation Systems, Knowledge Spillovers M2 – Methods in Empirical Economic Geography 5 Economic geography and networks ▪ What matters most for competitiveness of firms? ▪ Space or networks? the concept of ‘space of places’ expresses the idea that the location matters for learning and innovation (being in the right place is what counts), the concept of ‘space of flows’ focuses more on the idea that networks are important vehicles of knowledge transfer and diffusion (meaning that being part of a network is crucial). Ter Wal, A. L. J., & Boschma, R. A. (2009). M2 – Methods in Empirical Economic Geography 6 Economic geography and networks ▪ Networks and space ▪ Networks are located in space (think of Sillicon Valley) ▪ But it is not only about space: social networks matter ▪ Extra-cluster linkages are important to avoid lock-in clusters ▪… →Networks have their “own geography“ M2 – Methods in Empirical Economic Geography 7 Data Collection M2 – Methods in Empirical Economic Geography 8 Data collection ▪ So far, we have used attribute data in all our analyses ▪ Information on individual observations ▪ In SNA additional data need: information on connections between individuals → relational data M2 – Methods in Empirical Economic Geography 9 Data collection ▪ Data sources for relational data ▪ Primary data One data collection method: interviews including roster-recall methodology ▪ Secondary data E.g. Patent data base includes information on citation of patents M2 – Methods in Empirical Economic Geography 10 Primary data collection in network analysis ▪ Roster-recall methodology ▪ roster= Liste ▪ Aim: Collect full network data on a pre-defined population of actors → each of the actors in the population is provided with a full list of actors of the population Identifying the population may be a challenge Other methods do not start out with full list of actors, e.g. snowball system → but it risks missing isolated actors M2 – Methods in Empirical Economic Geography 11 Primary data collection in network analysis ▪ Roster-recall methodology ▪ (ideally) roster should include all actors as otherwise answers are biased ▪ For each of the pre-listed actors in the roster, the respondent has to indicate whether he/she had a relationship of a pre-defined type ▪ Recall: In addition, each respondents is asked to recall all other actors they had this type of relationship with and add them to the list Allows to enlarge pre-defined population M2 – Methods in Empirical Economic Geography 12 Primary data collection in network analysis Geht um die Beschreibung von Strukturen in Netzwerken ▪ Silly example roster-recall: Study group ▪ Roster for Person 1 Person 1 Person 2 Person 3 Person 4 Person 5 Recall any other person you studied with Never studied X 1 0 0 0 with studied with X 0 0 0 1 once studied with 2- X 0 0 0 0 Howard 4 times studied with >5 X 0 0 1 0 but X 0 1 0 0 John, Liza 10 M2 – Methods in Empirical Economic Geography 13 Types of networks ▪ Directed network Ausgerichtetes Beziehungsmuster ▪ Distinguishes sender/source and receiver/target ▪ (May) result in asymmetrical matrix: relationship must not be mutual (John considers Howard a friend, but Howard does not consider John a friend or: seeking advice) ▪ Undirected network Unausgerichtetes Netzwerk ▪ Results in symmetrical matrix: connections are always mutual (e.g. employer-employee relationship) M2 – Methods in Empirical Economic Geography 14 Example: directed network ▪ Directed ties (one direction) between Erica and Chris, Barbara and Erica… ▪ Reciprocal tie between Andrei and Hans Yang et al. (2017: p. 11). https://uk.sagepub.com/sites/default/files/upm-binaries/78651_Chapter_1.pdf M2 – Methods in Empirical Economic Geography 15 Example: undirected network ▪ Ties are always mutual Yang et al. (2017: p. 9). https://uk.sagepub.com/sites/default/files/upm-binaries/78651_Chapter_1.pdf M2 – Methods in Empirical Economic Geography 16 Types of networks ▪ Binary network ▪ No relationship/ relationship – 0/ 1 ▪ Valued network Intentsität der Netzwerkbeziehung wird gemessen ▪ No relationship vs different relational intensities ▪ Definition of relational intensity up to researcher M2 – Methods in Empirical Economic Geography 17 Saving data for network analysis ▪ Network data always consists of a “node list“ (=attribute data) and a dataset that defines the relationships among the actors, stored as one of the following ▪ Adjacency/ connectivity matrix See forthcoming lecture on spatial econometrics Rows and columns represent different vertices ▪ Edge list Two-column list of the two vertices that are connected M2 – Methods in Empirical Economic Geography 18 Saving data for network analysis ▪ Node list = attribute data ▪ Information on the unit of observations – ordinary data as for any other analysis ▪ Can be used in plotting the network, e.g. different colour for different gender, age groups, employment statuses Gender Age Employment status Person 1 M 43 Employed Person 2 F 35 Employed Person 3 F 22 In training Person 4 M 66 Retired Person 5 F 55 unemployed … M2 – Methods in Empirical Economic Geography 19 Defininig the relationship: Adjacency matrix Add actors from recall methodology Zeros on diagonal (but not always, e.g. trade example) Person 1 Person 2 Person 3 Person 4 Person 5 Howard John Liza Person 1 0 0 4 3 1 2 4 4 Person 2 0 Person 3 0 Person 4 0 Person 5 0 Howard 0 John 0 Liza 0 ▪ Here: enter information from questionnaire for person 1 (slide 13) ▪ Binary or valued? ▪ Directional or undirectional? M2 – Methods in Empirical Economic Geography 20 Defining the relationship: Edge list Sagt dasselbe aus wie die Tabelle auf der Folie davor, Nachbarschaftsmatrize auf S. 20 aber Empfehlung von Hundt Additional column for values Vertex 1 Vertex 2 Value Person 1 Person 3 4 Person 1 Person 4 3 Person 1 Person 5 1 Person 1 John 2 Person 1 Howard 4 Person 1 Liza 4 … … ▪ Here: enter information from questionnaire for person 1 (slide 13) ▪ Binary or valued? ▪ Directional or undirectional? M2 – Methods in Empirical Economic Geography 21 Plotting networks/ graphs ▪ Essential ideas ▪ Binary or valued network → thicker ties show stronger relationship ▪ Directed or undirected network → arrows for directed network ▪ Additional information from node list data → different colour for different groups of actors ▪ Many options on graph layout available in igraph package in R ▪ Tutorial M2 – Methods in Empirical Economic Geography 22 Descriptive analysis M2 – Methods in Empirical Economic Geography 23 Descriptive analysis: levels of analysis Level of analysis Level of analysis Key ideas Micro/ Local Distance between Node level nodes Centrality of actors: describing the position of actors in a network Meso Sub-Network level describing subgroups/ clusters in a network Famous level of analysis: the triad (any three nodes) Macro/ Global Network level describing the whole network M2 – Methods in Empirical Economic Geography 24 Descriptive analysis: levels of analysis http://www.fao.org/3/I8751EN/i8751en.pdf (p. 21) M2 – Methods in Empirical Economic Geography 25 The micro-level Distance between nodes Centrality of actors Zwei Kennzahlen der Mikroebene, die relativ beliebt sind M2 – Methods in Empirical Economic Geography 26 Distance between nodes ▪ Geodesic distance Geodäsie meint: ▪ the shortest path between two nodes (in terms of the minimum number of links) ▪ Example: many paths between 10 and 5 – but shortest path is direct ▪ Shortest path between 1 and 6? 3 options with 2 links M2 – Methods in Empirical Economic Geography 27 Centrality ▪ Idea ▪ one of the most frequently used ways to describe actors in a network ▪ measuring the centrality of individual actors ▪ High centrality: opportunity to influence & be influenced Zentralist als weitere Variable, die man nutzen kann ▪ How to do it? ▪Numerous measures with different ideas on what centrality is →R makes it simple for you to compare different measures M2 – Methods in Empirical Economic Geography 28 Centrality ▪ Measuring centrality: four main concepts 1. Degree 2. Closeness 3. Betweenness (brokerage) 4. Eigenvector ▪… →We need graph or adjacency matrix to calculate these measures of centrality Dafür Nachbarschaftsmatrize M2 – Methods in Empirical Economic Geography 29 Centrality Centrality (here D for Degree) of node i 1. Degree centrality 𝐶𝐷 𝑛𝑖 = 𝑑 𝑛𝑖 Number of links for node i ▪ Number of ties that involve a given node Example: undirected network Person 1 Person 2 Person 3 Person 4 Person 5 Degree centrality Person 1 0 0 1 1 1 3 Person 2 0 0 1 0 1 2 Person 3 1 1 0 0 1 3 Person 4 1 0 0 0 0 1 Person 5 1 1 1 0 0 3 ▪ Person 1: 𝐶𝐷 𝑛1 = 𝑑 𝑛1 = 3 Grad der Zentralität: Anzahl der Verbindungen M2 – Methods in Empirical Economic Geography 30 Centrality 1. Degree centrality ▪ For directed networks In-degrees and Out-degrees and total degrees Richtung wird hier berücksichtigt Degrees for I3 and W7? In- Out- Total degrees degrees degrees I3 0 0 0 W7 4 3 7 … M2 – Methods in Empirical Economic Geography 31 Centrality 2. Closeness centrality 1 Centrality (subscript C 𝐶𝑐 𝑛𝑖 = for Closeness) of node i σ𝑗 𝑔𝑖𝑗 Sum of geodesic distance between node i and all other nodes j ▪ Nodes with a high closeness score have the shortest distances to all other nodes. ▪ Index of expected time until arrival for given node When does actor learn about new information? M2 – Methods in Empirical Economic Geography 32 Centrality Warum Inversa bilden? Abzählen -> Inverse bilden -> Aufsummierung kleine Distanzen zeigen eine hohe Zentralität an Was ist mit Personen, die vorausgewählt waren, aber mit niemandem zusammenhängen? (I3 und S2) kann daran liegen, dass bspw. eine Liste veraltet ist 2. Closeness centrality kann man aus Forschung streichen In der ersten Forschungsphase kann man aber auch ▪ Inverse of sum of distances to all other nodes Fragezeichen setzten, falls sie wieder auftauchen ▪ 𝐶𝑐 𝑛𝑖 = σ 1 𝑔 (Inverse: short distances => high centrality) 𝑗 𝑖𝑗 http://www.analytictech.com/Essex/Lectures/centrality.pdf M2 – Methods in Empirical Economic Geography 33 Centrality 2. Closeness centrality 1 𝐶𝑐 𝑛𝐵 = 14 1 𝐶𝑐 𝑛𝐸 = 16 Man zählt alle Verbindung von B ausgehend: Direkt zu 5 zu D/F/ zwei Punkt zu J 3 Punkte -> Summe 14 (oder doch nicht?) Nur weil etwas zentral aussieht, muss es nicht zentral sein (siehe E) https://www.geeksforgeeks.org/closeness-centrality-centrality-measure/ M2 – Methods in Empirical Economic Geography 34 Centrality 3. Betweenness centrality ▪ How often does a node lie along the shortest path between two other nodes? ▪ Index of potential for gatekeeper, controlling the flow, liaising separate parts of the network ▪ Indicates power and access to diversity of what flows A = gatekeeper! Very central position according to betweenness centrality (all other connections pass through A) M2 – Methods in Empirical Economic Geography 35 Centrality 3. Betweenness centrality ▪ Computed as number of those paths that pass through ni 𝑔𝑗𝑘 (𝑛𝑖 ) Anzahl der Verbindungen, die durc h einen bestimmten Punkt/Person 𝐶𝐵 (𝑛𝑖 ) = σ𝑖,𝑗 laufen 𝑔𝑗𝑘 number of shortest paths from j to k Centrality (subscript B for Betweenness) of node i ▪ Example: If there are 11 shortest paths in the network 6 between j and k, and i is on 6 of them, then 𝐶𝐵 (𝑛𝑖 ) = 11 Auf wie viel der kürzesten Pfade liegt meine Person i? ▪ Video tutorial on “manual“ calculation: https://www.youtube.com/watch?v=l7_RNYay1qM&feature=youtu.be M2 – Methods in Empirical Economic Geography 36 Centrality 4. Eigenvector centrality ▪ Measure of being well-connected to the well-connected →Influence approach: a node is important if it is linked to other important nodes →Application: Google PageRank: Rank of a homepage depends on how often it is linked to at other homepages https://www.youtube.com/watch?v=DGVvm-j-NG4 M2 – Methods in Empirical Economic Geography 37 Centrality ▪ Page Rank ▪ developed by Larry Page (at Stanford), algorithm used by Google Search to rank web pages in their search results ▪ based on eigenvector centrality Wegen der Austauschbeziehung Links to pages with mit B profitiert C und wird deswegen größer und relevanter large eigenvectors are more important than links to pages with small eigenvectors E.g. C only has one link… https://en.wikipedia.org/wiki/PageRank#/media/File:PageRanks- Example.jpg M2 – Methods in Empirical Economic Geography 38 Centrality ▪ R‘s igraph package calculates all measures of centrality for you ▪ Do understand what kind of centrality makes sense for you ▪ Different measures of centrality are closely related but are likely to come to slightly different results – depending on their understanding of centrality ▪ Many of those measures are normalized (based on the number of observations) M2 – Methods in Empirical Economic Geography 39 The meso-level Subgroups in a network M2 – Methods in Empirical Economic Geography 40 Subgroups in a network ▪ Components ▪ Part of the network in which all actors are connected, directly or indirectly by at least one tie ▪ In R: per definition isolates do not count one component http://www.fao.org/3/I8751EN/i8751en.pdf (p 31) M2 – Methods in Empirical Economic Geography 41 Subgroups in a network ▪ Cliques ▪ „persons who interact with each other more regularly and intensely than others in the same setting“ (Salkind, 2008) ▪ Maximum sub-network with at least 3 nodes ▪ All actors are perfectly connected ▪ Nodes may belong to several cliques Alle sind durch eine Netzwerkverbindung miteinander verbunden Können auch 4er, 6er etc sein M2 – Methods in Empirical Economic Geography 42 Subgroups in a network ▪ How many cliques do you recognize? ▪ E.g. 1,2,3; 1,8,9; 3,7,9; 3,4,6; 2,3,6; 3,6,9; 4,6,10; 6,9,10 http://www.fao.org/3/I8751EN/i8751en.pdf (p 29) M2 – Methods in Empirical Economic Geography 43 Subgroups in a network ▪ Cluster coefficient → also called “transitivity“ ▪ A measure of a network of actors‘ tendency to “group together“ into pockets of dense connectivity. ▪ What exactly is transitivity? Whenever one node is connected to a second node and the second node is connected to a third node, the first node is also related to the third node → cliques are transitive M2 – Methods in Empirical Economic Geography 44 Subgroups in a network ▪ Cluster coefficient (transitivity) 𝑛𝑢𝑚𝑏𝑒𝑟 𝑜𝑓 𝑟𝑒𝑎𝑙𝑖𝑠𝑒𝑑 𝑐𝑜𝑛𝑛𝑒𝑐𝑡𝑖𝑜𝑛𝑠 𝑎𝑚𝑜𝑛𝑔 𝑛𝑒𝑖𝑔ℎ𝑏𝑜𝑢𝑟𝑠 𝐶= 𝑛𝑢𝑚𝑏𝑒𝑟 𝑜𝑓 𝑎𝑙𝑙 𝑝𝑜𝑠𝑠𝑖𝑏𝑙𝑒 𝑐𝑜𝑛𝑛𝑒𝑐𝑡𝑖𝑜𝑛𝑠 𝑎𝑚𝑜𝑛𝑔 𝑛𝑒𝑖𝑔ℎ𝑏𝑜𝑢𝑟𝑠 ▪ Here: from the perspective of the blue node C=1 C=1/3 C=0 Transivity: wie gut die Information ohne eigenes Dazutun durch das Netzwerk wandert Wie ist das Netzwerk außerhalb vom untersuchten Punkt vernetzt? -> bisschen wie gut die anderen miteinander vernetzt sind http://www.fao.org/3/I8751EN/i8751en.pdf (p 30) M2 – Methods in Empirical Economic Geography 45 The macro-level network properties M2 – Methods in Empirical Economic Geography 46 Network properties ▪ Network size Zahl der Netzwerkteilnehmer/-akteure ▪ The number of nodes in a network here: 10 nodes → network size = 10 M2 – Methods in Empirical Economic Geography 47 Network properties ▪ Network density ▪ 𝑁𝑒𝑡𝑤𝑜𝑟𝑘𝑑𝑒𝑛𝑠𝑖𝑡𝑦 = 𝑎𝑙𝑙 𝑎𝑐𝑡𝑢𝑎𝑙 𝑙𝑖𝑛𝑘𝑠 𝑎𝑙𝑙 𝑝𝑜𝑠𝑠𝑖𝑏𝑙𝑒 𝑙𝑖𝑛𝑘𝑠 ▪ Proportion of all links in the network to all possible links ▪ Density in cliques is always 1. Spezialfall Clique: immer 1 Beispiel Lerngruppe: Bei hoher Density erzielt sie im Durchschnitt ein besseres Ergebnis nice overview on terms in the Glossary M2 – Methods in Empirical Economic Geography 53 Further reading ▪ Blogs etc. ▪ https://www.youtube.com/watch?v=DfV-pjRTlLg ▪ http://pablobarbera.com/big-data-upf/html/02b-networks-descriptive-analysis.html ▪ http://www.analytictech.com/Essex/Lectures/centrality.pdf ▪ http://www.fao.org/3/I8751EN/i8751en.pdf --> nice overview on terms in the Glossary M2 – Methods in Empirical Economic Geography 53