Forecasting Lesson 1.2 PDF
Document Details
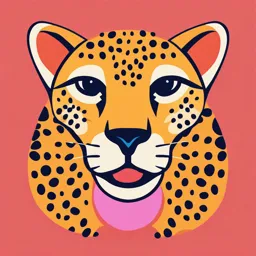
Uploaded by AwedSatyr
Visayas State University
Tags
Summary
This document explains forecasting methods including time series, qualitative, and causal methods. It includes examples and calculations to illustrate the concepts. The document appears to be part of a course or lecture notes on business operations management, focusing on forecasting techniques for businesses.
Full Transcript
Forecasting Lesson 1.2 Introduction Forecast - a prediction that will occur in the future. - Aids decision-makers in making effective and efficient decisions. Time-frame Forecasting Existence of patterns Components Number of variables Time-frame Short...
Forecasting Lesson 1.2 Introduction Forecast - a prediction that will occur in the future. - Aids decision-makers in making effective and efficient decisions. Time-frame Forecasting Existence of patterns Components Number of variables Time-frame Short-range forecasts- immediate future and are concerned with daily operations of the firm Ex. Daily demand and resource requirements Medium-range forecasts- starting from 1 to 2 months to 1 year Ex. Yearly production plan Long-range forecasts- encompasses period longer than 1 or 2 years. Long range- to plan for The farther into the new products, changing future one seeks to markets, build new predict, the more facilities or secure difficult forecasting long-term financing. becomes. Random variation Seasonal pattern The following are the 7 steps in Forecasting: 1.Determine the use of the forecast - It is important that we know the use of our forecasts because it can be used in driving decisions in the firm’s operations. 2. Select the items to be forecasted 3. Determine the time horizon of the forecast - The time horizon, as discussed previously can be short, medium or long term. 4. Select the forecasting model(s) - It includes a variety of statistical models like moving averages, econometrics, and regression analysis. 5. Gather the data needed to make the forecast 6. Make the forecast 7. Validate and implement the results - Forecasts should be reviewed regularly at the highest levels to make sure that the model, assumptions and data are valid. Basic types: Time series- uses historical data Regression (causal)- develops a Forecasting mathematical relationship between the Methods forecasted item and the factors that cause it to behave the way it does Qualitative methods- uses judgment, expertise and opinion to make forecasts QUALITATIVE METHODS ▪ Delphi Method ------ interactive group process consisting of obtaining information from a group of respondents through questionnaires and surveys ▪ Jury of Executive Opinion ------obtains opinions of a small group of high-level managers in combination with statistical models 9/19/2024 Delphi Method Jury of Executive Opinion- Marketing, Sales, HR, Finance, Operations, Production department, who discuss and provide their opinions and experience to the board of directors as high level executes are well acquainted with the strengths and weaknesses of the firm and what changes need to be done. ▪ Sales Force Composite ------ allows each salesperson to estimate the sales for his/her region and then compiles the data at a district or national level ▪ Consumer Market Survey ------- solicits input from customers or potential customers regarding their future purchasing plans Grassroots Approach The 'grassroots' is a method which base on the concept of asking the people who are close to the eventual consumer, such as sales person. TIME SERIES TECHNIQUES 1. Moving average consists of computing an average of the most recent n data values for the time series and using this average for the forecast of the next period. Example 1 Month Actual Three-Month Shed Moving Average Sales January 10 February 12 March 13 April 16 (10+12+13)/3 = 11 2/3 May 19 (12+13+16)/3 = 13 2/3 June 23 (13+16+19)/3 = 16 July 26 (16+19+23)/3 = 19 1/3 To accompany Quantitative Analysis for Management, 9e 5-35 by Render/Stair/Hanna Wallace Garden Supply’s Three-Month Moving Average Example 2 2. Weighted Moving Average Weighted moving averages use weights to put more emphasis on recent periods. To accompany Quantitative Analysis for Management, 9e 5-39 by Render/Stair/Hanna Calculating Weighted Moving Average 3. Naive Estimating technique in which the last period's actuals are used as this period's forecast, without adjusting them or attempting to establish causal factors. It is used only for comparison with the forecasts generated by the better (sophisticated) techniques. Period Month Demand Forecast 1 Jan 120 2 Feb 103 120 3 March 105 103 4 April 84 105 5 May 114 84 6 June 90 114 7 July 90 4. Exponential Smoothing -is a type of moving average technique that involves little record keeping of past data New forecast: Ft+1= previous forecast + α(previous actual –previous forecast) a. b. 9/19/2024 To accompany Quantitative Analysis for Management, 9e 5-44 by Render/Stair/Hanna Example 1. Port of Baltimore Exponential Smoothing To accompany Quantitative Analysis for Management, 9e 5-45 by Render/Stair/Hanna Port of Baltimore Exponential Smoothing Example Qtr Actual Tonnage Rounded Forecast using α =0.50 Unloaded 1 180 175 2 168 178 =175.00+0.50(180-175) 3 159 173 =177.50+0.50(168-177.50) 4 175 166 =172.75+0.50(159-172.75) 5 190 170 =165.88+0.50(175-165.88) 6 205 180 =170.44+0.50(190-170.44) 7 180 193 =180.22+0.50(205-180.22) 8 182 186 =192.61+0.50(180-192.61) 9 ? 184 =186.30+0.50(182-186.30) Smoothing constant In picking a value for the smoothing constant, the objective is to obtain the most accurate forecast. Several values of the smoothing constant may be tried, and the one with the lowest MAD could be selected. Example 2 Computations… A seasonal index is a measure used in time series analysis to quantify the seasonal variation in data. It helps to understand how a particular season (e.g., month, quarter) compares to the average season over a cycle. Here’s a detailed explanation: What is a Seasonal Index? Definition: A seasonal index indicates how much a particular period (such as a month or quarter) deviates from the average period. It is typically expressed as a percentage or a decimal. Purpose: It helps in identifying and quantifying seasonal patterns in data, which is crucial for accurate forecasting and analysis. CAUSAL METHOD Linear Trend -most often thought of as a causal method of forecasting in which a mathematical relationship is developed between demand and some other factor that affects demand behavior. Demand Estimation ❑ Simple Regression Explaining “y” in terms of “x”. also called as two-variable linear regression model. 56 Linear trend line: For the 13th month forecast: Demand Estimation and Forecasting ❑ Multiple Regression Extension of the linear regression. More amenable to ceteris paribus analysis because it allows us to explicitly control for many factors which simultaneously affect the dependent variable. 61 Demand Estimation and Forecasting ❑ Multiple Regression 62 Demand Estimation and Forecasting ❑ Multiple Regression Sample: A distributor of dessert pies wants to evaluate factors thought to influence sales. Dependent variable: Pie sales (units per week) Independent variables: Price (in US$) Advertising ($100’s) Data are collected for 15 weeks. 63 Demand Estimation and Forecasting Pie Sales Model 64 Demand Estimation and Forecasting ❑ Multiple Regression Output 65 A value of 1 means a perfect positive relationship and a value of zero means no relationship at all. Demand Estimation and Forecasting ❑ Multiple Regression Equation Where, Sales = number of b1 = -24.975: sales will b2 = 74.131: sales will pies per week decrease, on average, increase, on average, by Price= in US $ by 24.975 pies per week 74.131 pies per week for Advertising= in for each $1 increase in each $1 increase in $100’s selling price, net of the advertising, net of the effects of changes due effects of changes due to advertising to advertising 67 Forecasting at Disney Disney chairman receives a daily report from his main theme parks in Orlando, Florida, the report contains only two numbers: the forecast of yesterday’s attendance at the parks (Magic Kingdom, Epcot, Fort Wilderness, Hollywood Studios (formerly MGM Studios), Animal Kingdom, Typhoon Lagoon, and Blizzard Beach) and the actual attendance. An error close to zero (using MAPE as the measure) is expected. The chairman takes his forecasts very seriously. The forecasting team at Disney World doesn’t just do a daily prediction, however, and the chairman is not its only customer. It also provides daily, weekly, monthly, annual, and 5-year forecasts to the labor management, maintenance, operations, finance, and park scheduling departments. It uses judgmental models, econometric models, moving average models, and regression analysis. FORECAST ACCURACY Measures of Forecast Accuracy Mean Absolute Deviation (MAD) one of the most popular and simplest-to-use measures of forecast error sum of the absolute values of the individual forecast errors and dividing by the numbers of errors (n): Mean squared error (MSE), The average of the squared errors: 100 400 400 900 400 100 900 100 100 Sum of errors: 3400/9 MSE: 377.78 Using MSE… Compare it with benchmarks. Compare it with other forecasting tools. COMPARISON 1 COMPARISON 2 MAPE 7.03% 6.84% 4.22% 3.90% COMPARISON 3 COMPARISON 4 Forecasting MAD MSE MAPE method Naïve 10 50 60% Exponential 20 70 40% Smoothing Weighted 30 90 50% moving average