LectureNote2_Q3_2024.pdf
Document Details
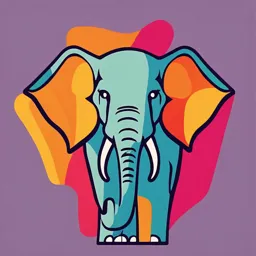
Uploaded by UnparalleledSugilite407
Western Sydney University
Tags
Full Transcript
Visual Perception and Visual Design Visualisation (COMP7016) Dr Zhonglin (Jolin) Qu [email protected] Dr Zhonglin (Jolin) Qu. Email: [email protected] 1 Staffs - Reminder Subject Coordinator & Lecturer: Ms Zhonglin (Jolin) Qu – Room: ER.G.1...
Visual Perception and Visual Design Visualisation (COMP7016) Dr Zhonglin (Jolin) Qu [email protected] Dr Zhonglin (Jolin) Qu. Email: [email protected] 1 Staffs - Reminder Subject Coordinator & Lecturer: Ms Zhonglin (Jolin) Qu – Room: ER.G.19, Building ER, Parramatta South Campus – Email: [email protected] (preferred contact method) – Consultation hours: Wednesday 10:00-11:00 am Tutors – Dr Zhonglin (Jolin) Qu, Email: [email protected] – Mr Vishal Patel , Email: [email protected] Dr Zhonglin (Jolin) Qu. Email: [email protected] 2 Last Lecture Why Visualisation? Big Data The Challenges in Visualisation Information Visualisation and Scientific Visualisation Good and Bad Visualisations Dr Zhonglin (Jolin) Qu. Email: [email protected] 3 Last Week Survey Visualisations Tell me some rumours you've heard about this subject or me.- Most frequent words in the corpus: subject (4); hard (3); easy (3); really (2); pass (2) What do you expect to learn from this subject?- Most frequent words in the corpus: visualisation (6); data (6); visualization (4); tableau (4); skills (4 ) Dr Zhonglin (Jolin) Qu. Email: [email protected] 4 Module Outline Perception and Cognition Human Vision Colour Gestalt Laws Visual Encoding Dr Zhonglin (Jolin) Qu. Email: [email protected] 5 PERCEPTION & COGNITION Dr Zhonglin (Jolin) Qu. Email: [email protected] 6 Perception and Cognition Perception – Identification and interpretation of sensory information – From the physical stimulus to recognizing information – Shaped by learning, memory, expectation – Processing of the signals coming in: what you “see” – E.g. hear someone speak: Perception Cognition – The processing of information, applying knowledge – How you understand and interpret what you see – E.g. understand the language and the words: Cognition Dr Zhonglin (Jolin) Qu. Email: [email protected] 7 Perception and Cognition (cont.) Perception Cognition Eye, optical nerve, visual Recognizing objects cortex Relations between objects Basic perception Conclusion drawing First processing (edges, Problem solving planes) Learning, … Not conscious Reflexes Dr Zhonglin (Jolin) Qu. Email: [email protected] 8 Perception: Bottom-up and Top-down Bottom-up processing – Low level: features → pattern → object – Detect edges, contours, colour, motion Top-down processing – Driven by goals, expectations – Uses prior knowledge, experience, filters what we “see” Dr Zhonglin (Jolin) Qu. Email: [email protected] 9 What are the Colours? RED GREEN BLUE BLACK PINK YELLOW BLACK ORANGE GREEN BLUE PINK BROWN PINK GREEN RED BLACK GREY WHITE BLUE BROWN GREEN PINK RED ORANGE Dr Zhonglin (Jolin) Qu. Email: [email protected] 10 Human Eyes Photoreceptor cells, e.g. Rods and Cones, are specialized types of neurons found in the retina that are capable of phototransduction. They convert light into signals that can stimulate biological processes ≈ 120 million Rods black/white very sensitive to ≈ 5-6 million Cones luminosity but turn Colour vision off in bright light Dense in the centre Sensitive to colour, but only in bright ≈ 1.5 million optic nerve light endings Source: https://en.wikipedia.org/wiki/Photoreceptor_cell Dr Zhonglin (Jolin) Qu. Email: [email protected] 11 Human Vision Source: http://www.rags-int-inc.com/PhotoTechStuff/CameraEye/ Dr Zhonglin (Jolin) Qu. Email: [email protected] 12 Human Vision (cont.) Highest bandwidth sense Fast and Parallel Pattern Recognition Pre-attentive Extends memory and cognitive capacity People think visually Dr Zhonglin (Jolin) Qu. Email: [email protected] 13 Human Vision (cont.) Vision is “constructed” top down from the input “What you see when you see a thing depends on what the thing is. What you see the thing as depends on what you know about what you are seeing.” Zenon Walter Pylyshyn Canadian Cognitive Scientist and Philosopher Dr Zhonglin (Jolin) Qu. Email: [email protected] 14 Human Vision vs Camera Human vision is not similar to a camera. It is more similar to a dynamic and ongoing construction project. Angle of View: each eye individually has anywhere from a 120-200° angle of view. The dual eye overlap region is around 130° Resolution & Detail: the human eye is able to resolve the equivalent of a 52 megapixel camera. Real-life is around up- to 16 million pixels Sensitivity and Dynamic Range: our eye's dynamic range depends on brightness and subject contrast. Under low- light conditions, our eyes become much more sensitive than camera Source: http://www.cambridgeincolour.com/tutorials/cameras-vs-human-eye.htm Dr Zhonglin (Jolin) Qu. Email: [email protected] 15 Human Visual System No general purpose vision What we see depends on our goals and expectations Relative judgments: strong Absolute judgments: weak Example Video: Ames Room https://www.youtube.com/watch?v=gJhyu6nlGt8 https://www.youtube.com/watch?v=NsZEWxubxUA Dr Zhonglin (Jolin) Qu. Email: [email protected] 16 COLOUR Dr Zhonglin (Jolin) Qu. Email: [email protected] 17 Visible Spectrum Dr Zhonglin (Jolin) Qu. Email: [email protected] 18 Dimension of Colour Hue: colour appearance parameter, includes red, green, blue, yellow, orange and violet Saturation: purity of colour Different Saturation, medium Value Value (Luminance, Brightness): – lightness or darkness of a colour – high saturation colours have medium values Different Values, low Saturation Dr Zhonglin (Jolin) Qu. Email: [email protected] 19 Additive and Subtractive Colour A monitor colour is additive colour – Contrast to subtractive colour model for inks on paper and canvas Most common way to specify colour on the computer is with RGB values – RGB = Red, Green, Blue – Each RGB value is set for 1 pixel Additive Colour: Add colours by Subtractive Colour: using adding light of certain wavelength pigments, inks by absorbing light Dr Zhonglin (Jolin) Qu. Email: [email protected] 20 Colour Vision Colour vision is irrelevant to much of normal vision! – does not help to perceive layout of objects – how they are moving – what shape they are Colour breaks camouflage Tells about material or item properties Dr Zhonglin (Jolin) Qu. Email: [email protected] 21 Can You See the Apples? Which image is better for identifying the apples, left or right? Dr Zhonglin (Jolin) Qu. Email: [email protected] 22 Using Colour in Visualisation Colour labelling (nominal information coding) – recommended: about 7±2, no more than 10 There are more than 10 colours for categories, but we can distinguish about 8 colours Dr Zhonglin (Jolin) Qu. Email: [email protected] 23 Quantitative Data Visualisation Use concrete value Saturation works but not as good Don‘t use hue! Be careful: rainbow colour map Very good for identifying 1-2 simultaneous, serial search for more [Ware 04]’s suggestion: red, green, yellow, blue, black, white, pink, cyan, grey, orange, Four data sets visualized with (a) rainbow, (b) grey-scale, (c) black- brown, purple. body radiation, and (d) isoluminant green–red colour maps Dr Zhonglin (Jolin) Qu. Email: [email protected] 24 Colour Maps (Engelke 2015) Rainbow Greyscale Blackbody GIST Earth Cool-Warm No perceptual Luminance variance. Good colour map Geographically Divergent colour map ordering. Simultaneous when no assumption inspired (white neutral point). Partial isoluminance. contrast issues. about data. (blue water, to white Sharp transitions. No simultaneous mountain tops). contrast issues. 25 Good Colour Guideline for Map http://colorbrewer2.org/ Dr Zhonglin (Jolin) Qu. Email: [email protected] 26 Good Visualizations Dr Zhonglin (Jolin) Qu. Email: [email protected] 27 Bad Visualizations Dr Zhonglin (Jolin) Qu. Email: [email protected] 28 Colour Blindness Affect 10% of males, 1% of females (probably due to Xchromosomal recessive inheritance) Most common: red-green blindness Reason: lack of medium or long wavelength receptors, or altered spectral sensitivity (most common: green shift) Dr Zhonglin (Jolin) Qu. Email: [email protected] 29 Luminance, Brightness, Lightness Luminance – measured amount of light (luminous intensity per area) Brightness – perceived amount of light Lightness – perceived reflectance of a surface – lightness of a colour Dr Zhonglin (Jolin) Qu. Email: [email protected] 30 Brightness Contrast The perceived brightness of an object is relative to it‘s background Very different on screen versus paper The inner squares have the same colour brightness Dr Zhonglin (Jolin) Qu. Email: [email protected] 31 Luminance Important for foreground and background to differ in brightness – Grayscale is probably not best way to encode data because of contrast issues Hello, here is some text. Can you read what it says? Hello, here is some text. Can you read what it says? Hello, here is some text. Can you read what it says? Hello, here is some text. Can you read what it says? Hello, here is some text. Can you read what it says? Hello, here is some text. Can you read what it says? Hello, here isDr Zhonglin some(Jolin) text. Can you read what it says? Qu. Email: [email protected] 32 Edge Enhancement Cornsweet effect Dr Zhonglin (Jolin) Qu. Email: [email protected] 33 Edge Enhancement Make objects more distinct Dr Zhonglin (Jolin) Qu. Email: [email protected] 34 Colour Blindness (cont.) Deuteranopia (no green receptors) Normal Colour Perception Protanopia (no red receptors) Dr Zhonglin (Jolin) Qu. Email: [email protected] 35 Colour Blindness Test Source: https://rgthinkcreative.files.wordpress.com/2011/10/red-green-color-blind-isihara-test-rgthinkcreative.jpg Dr Zhonglin (Jolin) Qu. Email: [email protected] 36 PREATTENTIVE PROCESSING Dr Zhonglin (Jolin) Qu. Email: [email protected] 37 Preattentive Processing How does human visual system analyse images? – very rapid ≈200-250 milliseconds (eye movements take 200 milliseconds) – very accurate – processed in parallel by low-level vision system Happens before focused attention → “preattentive” attention is very important for cognition Independent of the number of distractors! Opposite: sequential search (processed serially) Dr Zhonglin (Jolin) Qu. Email: [email protected] 38 How many 3’s? 1281768756138976546984506985604982826762 809858458224509856458945098450980943585 9091030209905959595772564675050678904567 8845789809821677654876364908560912949686 Dr Zhonglin (Jolin) Qu. Email: [email protected] 39 How many 3’s? 1281768756138976546984506985604982826762 809858458224509856458945098450980943585 9091030209905959595772564675050678904567 8845789809821677654876364908560912949686 Numbers differ only in shape, and are high-level symbols You have to literally scan them all and count the 3s The distinction of colour is immediate and pre-attentive You only have to scan and count the 3s This is why colour is an important visual attribute, especially for a categorial variable in visualisations Dr Zhonglin (Jolin) Qu. Email: [email protected] 40 Preattentive Processing - Tasks Target detection – Detect the presence or absence of a target Boundary detection – Detect a texture boundary between two or more groups of elements, where all of the elements in each group have a common visual property – Can the elements be grouped? Counting and estimation – Users count or estimate the number of elements with the same visual feature – How many elements of a certain type are present? Region Tracking – Track one or more elements with a unique visual feature as they move in time and space Dr Zhonglin (Jolin) Qu. Email: [email protected] 41 Difference in Hue Determine if red circle(s) is present in the picture? Difference in Hue can be done rapidly (preattentively) Surrounding objects called “distractors” Dr Zhonglin (Jolin) Qu. Email: [email protected] 42 Difference in Shape Determine if a blue square is present in the picture? Difference in Shape can be done preattentively Dr Zhonglin (Jolin) Qu. Email: [email protected] 43 Not Valid for Combinations Determine if a red circle is present in the picture? Cannot be done preattentively Must perform a sequential search Conjunction of features (shape and hue) causes it Distractor objects have both properties (red, circle) Dr Zhonglin (Jolin) Qu. Email: [email protected] 44 Boundary Detection Determine the groups of objects in the picture? Left image can be done preattentively since each group contains one unique feature Right image cannot since the two features are mixed (colour and shape) Dr Zhonglin (Jolin) Qu. Email: [email protected] 45 Potential Preattentive Features Length hue Width intensity Size flicker curvature direction of motion number binocular lustre terminators stereoscopic depth 3-D intersection depth cues closure lighting direction Dr Zhonglin (Jolin) Qu. Email: [email protected] 46 Some Preattentive Examples Source: Information Visualization:Perception for Design by Collin Ware Dr Zhonglin (Jolin) Qu. Email: [email protected] 47 Some Preattentive Examples (cont.) Source: Information Visualization:Perception for Design by Collin Ware Dr Zhonglin (Jolin) Qu. Email: [email protected] 48 Some Preattentive Examples (cont.) Dr Zhonglin (Jolin) Qu. Email: [email protected] 49 Source: Information Visualization:Perception for Design by Collin Ware Theories of Preattentive Processing Read the following article for more information http://www.csc2.ncsu.edu/faculty/healey/PP/i ndex.html Dr Zhonglin (Jolin) Qu. Email: [email protected] 50 Preattentive Processing in Visualisation Can be used to draw attention to areas of interest Can be used to express similarity/group memberships Visual features must be carefully designed Conjunctions must be avoided – Visual Cues: Practical Data Visualization, Peter R. Keller (Author), Mary M. Keller Dr Zhonglin (Jolin) Qu. Email: [email protected] 51 GESTALT PRINCIPLES “Gestalt” = “form”, German psychology in early 1900’s Attempt to understand pattern perception Founded Gestalt school of psychology Provided clear descriptions of many basic perceptual phenomena Gestalt Laws of Pattern Perception Perception as top-down process governed by holistic principles. Dr Zhonglin (Jolin) Qu. Email: [email protected] 52 The Visual Human-Data-Interface Data Visualisation Visual System Encoding/ Perception/ Design Cognition Image source: www.studyblue.com 53 Scales of Measurement (Stevens 1946) Categorical Quantitative Scale Nominal Ordinal Interval Ratio Distinct Distinct Ordered Meaningful Absolute zero feature categories categories distances Equality / Smaller than / Addition / Multiplication / Operations Inequality Larger than Subtraction Division Example: Participant Order of Scale rating (e.g. Response time in User study number participation 1 to 5) seconds 54 Gestalt Laws (Koffka 1935) Proximity Similarity Closure Continuity Symmetry Connectedness Past Experience Common fate [ ]{ }[ ] 55 Marks and Channels (Munzner 2014) Position Size Color Common scale: Length (1D): Hue: Unaligned scale: Area (2D): Saturation: Spatial: Volume (3D): Luminance: Shape Angle Curvature Motion 56 Gestalt Laws Proximity – Things close together are perceptually grouped together Dr Zhonglin (Jolin) Qu. Email: [email protected] 57 Gestalt Laws (cont.) Similarity – Similar elements get grouped together – Preattentive: colour, shape, size, value, orientation, texture – Modulate everything else: blurring, darkening, desaturating, etc. Dr Zhonglin (Jolin) Qu. Email: [email protected] 58 Gestalt Laws (cont.) Connectedness – Connecting different objects by lines or curve to unify them – Surround items with a outline, surface, volume Dr Zhonglin (Jolin) Qu. Email: [email protected] 59 Gestalt Laws (cont.) Continuity – More likely to construct visual entities out of smooth, continuous visual elements Dr Zhonglin (Jolin) Qu. Email: [email protected] 60 Gestalt Laws (cont.) Symmetry – Symmetrical patterns are perceived more as a whole Dr Zhonglin (Jolin) Qu. Email: [email protected] 61 Gestalt Laws (cont.) Closure – A closed contour is seen as an object Dr Zhonglin (Jolin) Qu. Email: [email protected] 62 Gestalt Laws (cont.) Relative Size – Smaller components of a pattern as perceived as objects Dr Zhonglin (Jolin) Qu. Email: [email protected] 63 Gestalt Laws (cont.) Figure & Ground – Figure is foreground, ground is behind Dr Zhonglin (Jolin) Qu. Email: [email protected] 64 VISUAL ENCODING Dr Zhonglin (Jolin) Qu. Email: [email protected] 65 Selective vs Associative Visual Variables (Bertin 1983) Selective: Enables to immediately isolate all correspondences belonging to the same category of that variable. Associative: Enables the immediate grouping of all correspondences differentiated by this variable. Image source: http://www.axismaps.com/guide/general/visual-variables/ 66 Effective Encoding of Data (Mackinlay 1986) Mackinlay, J. (1986). Automating the design of graphical presentations of relational information. Acm Transactions On Graphics (Tog), 5(2), 110-141. Images source: https://www.lucypark.kr/courses/2015-ba/visualization1.html 67 Separability/Integrality of Dimensions (Ware 2012) Separable Integral Group location Motion Motion Colour Size x-size Red-green Shape, size, Shape, size, Colour Colour Orientation y-size Yellow-blue orientation orientation 68 Relative vs Absolute Judgment (Cleveland 1984) Unaligned Unaligned Aligned Aligned No reference Reference No reference Reference 69 Encoding Multiple Attributes Position + Hue + Size + Shape Length + Position/Hue + Length/Hue + Length/Lum Fine Still fine Getting difficult Too much 70 Next Lecture Relational Data Visualisation – Part I Dr Zhonglin (Jolin) Qu. Email: [email protected] 71