Lecture 5: Morphological Classification of Galaxies PDF
Document Details
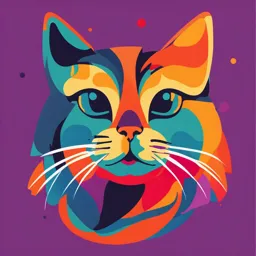
Uploaded by SelfSufficiencySpring8107
Universidad de La Laguna
Tags
Summary
This document provides an overview of different methods for classifying galaxies, focusing on both visual and model-based approaches. Key aspects such as the Hubble sequence, its limitations, more advanced methods like the CAS classification and machine learning techniques, as well as photometric decompositions, are discussed. The document also touches on various specific parameters and methods.
Full Transcript
Morphological Classi cation of Galaxies More Readings: Schneider - Chapter 3 Astronomy - Chap...
Morphological Classi cation of Galaxies More Readings: Schneider - Chapter 3 Astronomy - Chapter 26 ASTROFÍSICA EXTRAGALÁCTICA - Master Universitario en Astrofísica 2024-2025 fi Three main types of galaxy classi cations VISUAL MODEL INDEPENDENT MODEL DEPENDENT Morphological Classi cation CAS classi cation Photometric Concentration Hubble Sequence Asymmetry Decompositions Smoothness Galaxy Zoo Machine learning 2D algorithms and classi cation ( e.g., GALFIT, IMFIT) Citizen Science fi fi fi fi Three main types of galaxy classi cations VISUAL Morphological Classi cation Hubble Sequence Galaxy Zoo and Citizen Science fi fi MORPHOLOGICAL CLASSIFICATION E. Hubble, 1936 “The Realm of Nebulae” MORPHOLOGICAL CLASSIFICATION Principles of the classification: Galaxy light concentration Shape of the isophotes ε = 10 x (1-q) = 10 x (1-b/a) Relative prominence of the central bulge with respect to the disc Presence of a central stellar bar Tightly wound arms (low pitch angle) vs. loosely wound arms (large pitch angle) Pitch angle, the angle between arm and tangent of circle at radius R Early-type galaxies: Late-type galaxies: Ellipticals and Spirals Lenticulars SINGS Team - 2013 Hubble Sequence Main Drawbacks Subjective. It depends on the classifier. Superficial. It does not depend on physical properties. Inclination. It depends on the galaxy orientation. Wavelength. It depends on the wavelength range. Incomplete. It does not include Giant vs. Dwarf galaxies Hubble Sequence Main Drawbacks Subjective. It depends on the classifier. Superficial. It does not depend on physical properties. Inclination. It depends on the galaxy orientation. Wavelength. It depends on the wavelength range. Incomplete. It does not include Giant vs. Dwarf galaxies Hubble Sequence Main Drawbacks Subjective. It depends on the classifier. Superficial. It does not depend on physical properties. Inclination. It depends on the galaxy orientation. Wavelength. It depends on the wavelength range. Incomplete. It does not include Giant vs. Dwarf galaxies Hubble Sequence Main Drawbacks Subjective. It depends on the classifier. Superficial. It does not depend on physical properties. Inclination. It depends on the galaxy orientation. Wavelength. It depends on the wavelength range. Incomplete. It does not include Giant vs. Dwarf galaxies Hubble Sequence Main Drawbacks Subjective. It depends on the classifier. Superficial. It does not depend on physical properties. Inclination. It depends on the galaxy orientation. Wavelength. It depends on the wavelength range. Incomplete. It does not include Giant vs. Dwarf galaxies Hubble Sequence Main Drawbacks Subjective. It depends on the classifier. Superficial. It does not depend on physical properties. Inclination. It depends on the galaxy orientation. Wavelength. It depends on the wavelength range. Incomplete. It does not include Giant vs. Dwarf galaxies Hubble Sequence Main Drawbacks Subjective. It depends on the classifier. Superficial. It does not depend on physical properties. Inclination. It depends on the galaxy orientation. Wavelength. It depends on the wavelength range. Incomplete. It does not include Giant vs. Dwarf galaxies M101 NGC891 Credit: CFHT/NOAO/AURA/NSF/NASA/ESA/STScI Credit: Adam Block, Mt. Lemmon SkyCenter, U. Arizona Hubble Sequence Main Drawbacks Subjective. It depends on the classifier. Inclination. It depends on the galaxy orientation. Superficial. It does not depend on physical properties. Wavelength. It depends on the wavelength range. Incomplete. It does not include Giant vs. Dwarf galaxies Hubble Sequence Main Drawbacks Subjective. It depends on the classi er. Super cial. It does not depend on physical properties. Inclination. It depends on the galaxy orientation. Wavelength. It depends on the wavelength range. Incomplete. It does not include Giant vs. Dwarf galaxies Buta 2011, arXiv:1102.0550 fi fi Hubble Sequence “Good News” Galaxy structures reflect different formation and evolution processes. Some important physical trends along the Hubble Sequence E S0 Sa Sb Sc Sdm/Irr Pressure Support Rotational support Passive Star-forming Red colors Blue colors Hot gas Cold gas De Vaucouleurs Classi cation system de Vaucouleurs 1959, de Vaucouleurs 1964, Reference Catalogue of Bright Galaxies Mixed morphological types Mixed barred and normal SA, SB, SAB Sm type- between spiral and irregular Ring shaped vs S-shaped fi Classi cation numerical system OTHER CLASSIFICATIONS van den Bergh (1960) introduced a system of brightness classes for spirals. The nomenclature goes from type I to V, with I being spirals with very defined arms. Notice that it has to do with the luminosity and shape of the spiral arms, not with the total luminosity of the galaxy. fi A revised system The devil is in the details Comprehensive de Vaucouleurs Revised Hubble-Sandage (CVRHS) Buta 2015 More detailed classification of structures (r) - Inner ring (s) - Inner spiral (l) - Inner lens (bl) - barlens (R) - Outer ring (R’) - Outer pseudoring (L) - Outer lens Deep Photometry Martínez-Delgado 2020,The Realm of the Low-Surface-Brightness Universe How can we classify millions of galaxies? Classify a galaxy takes ~30 second. Classify a million galaxies would take us about a year without stop to eat or to sleep Galaxy Zoo Decision Tree Willett et al. 2013 Probabilistic Morphology Galloway et al. 2015 Three main types of galaxy classi cations VISUAL MODEL INDEPENDENT MODEL DEPENDENT Morphological Classi cation CAS classi cation Photometric Concentration Hubble Sequence Asymmetry Decompositions Smoothness Galaxy Zoo Machine learning 2D algorithms and classi cation ( e.g., GALFIT, IMFIT) Citizen Science fi fi fi fi Three main types of galaxy classi cations MODEL INDEPENDENT CAS classi cation Concentration Asymmetry Smoothness Machine learning classi cation fi fi fi CAS. Concentration, Asymmetry, Smoothness Abraham et al. 1994, 1996; Conselice 2003; Lotz et al. 2004 Asymmetry Smoothness/Clumpyness Concentration Conselice 2003 Combining parameters to classify galaxies Low High Low High Conselice 2003 High Low Typical CAS parameter for different morphologies Other common parameters Gini Parameter. Measures the relative distribution of flux in galaxy pixels G=0 Uniform Distribution; G=1 All flux in one pixel f: pixel flux n: number of pixels M20. Measures the concentration of light, but not necessarily in the galaxy center Xc and Yc are the galaxy center Using Gini-M20 to identify galaxy types and mergers Concentration Asymmetry Smoothness Gini M20 Lotz et al. (2004) Machine Learning applied to Galaxy Morphology Support Vector Machines Huertas-Company et al. (2008) More advanced techniques now in the market (Dieleman et al. 2015) Deep Learning applied to Galaxy Morphology Barchi et al. (2020) Deep Learning applied to Galaxy Morphology Three main types of galaxy classi cations VISUAL MODEL INDEPENDENT MODEL DEPENDENT Morphological Classi cation CAS classi cation Photometric Concentration Hubble Sequence Asymmetry Decompositions Smoothness Galaxy Zoo Machine learning 2D algorithms and classi cation ( e.g., GALFIT, IMFIT) Citizen Science fi fi fi fi Three main types of galaxy classi cations MODEL DEPENDENT Photometric Decompositions 2D algorithms ( e.g., GALFIT, IMFIT) fi Photometric Decomposition These are parametric techniques that use functional forms to describe the surface brightness distribution of the different stellar structures composing the galaxies. Surface Brightness Pro les Measure I(r) along isophotes I stands for intensity in e.g. F/pc2 isophotes denote constant brightness I(r) is an azimuthal average Often plotted in log scale µ(r) ~ -2.5 log I(r) magnitude scale Azimutal Angle Inner part blurred out due to both Point Spread Function (PSF) and Seeing seeing caused by atmospheric turbulence PSF caused by the telescope model the PSF to obtain true surface brightness profile The radial variation of the surface brightness is modelled with a set of empirical profiles μ r fi The Sérsic Pro le Graham & Driver, 1995 This profile is generally used to model elliptical galaxies and bulges. However, its flexibility makes it useful for other components as well. Empirically derived by Sérsic (1963) or expressed as magnitudes where I(r) = surface brightness as function of radius r Ie = effective surface brightness Re = effective radius n = Sérsic index For n = 4 de Vaucouleurs profile (ellipticals) For n = 1 exponential disc (discs) bn = function to assure Re contain half of the light. Approximation bn = 2n - 0.327 (Capaccioli 1989) fi The Sérsic Pro le Computing the luminosity of the component using the profile Using the Sérsic function instead of I(R) where is the incomplete gamma function and The total luminosity can be computed using the complete gamma function fi The Sérsic Pro le Graham & Driver, 1995 fi The Exponential Pro le This profile was proposed by Freeman (1970) to describe stellar discs The Sérsic profile can be reduced to an exponential assuming n=1. In general we use the simple expression I(R) = I0 exp(−r/h) or expressed as magnitudes 2.5 R μ(R) = μ0 + ln(10) h where I0 is the central intensity and h is the disc scale length. The total luminosity can be computed using the integral before given 2 L = 2 π I0 h fi Breaks in exponential pro les The surface-brightness profile of a galaxy disc is usually described with an exponential profile. However, nowadays it is commonly accepted that galaxy discs can be classified into three general categories (Erwin et al. 2005; Pohlen & Trujillo 2006): Type I profiles that follow a single exponential profile along the whole optical extent of the galaxies Type II profiles that present a double exponential law with a down-bending beyond the so-called break radius Type III profiles that exhibit an up-bending in the outer parts of the discs. Pohlen & Trujillo 2006 Méndez-Abreu et al. 2017 fi The Ferrers Pro le This represents the projected surface density of a three-dimensional Ferrers ellipsoid (Ferrers 1877, see also Aguerri et al. 2009) and it is generally used to describe the surface brightness of the bar component. where I0,bar is the central intensity of the bar abar is the bar radius nbar describe the shape of the profile fi PHOTOMETRIC DECOMPOSITIONS At the beginning they were used in 1D Freeman 1970, Kormendy 1977, Boroson 1981, Trujillo 2001 Aguerri et al. 2005 I(R) = Ibulge + Idisk + Ibar Now most photometric decompositions are done in 2D Several codes have been developed GALFIT (Peng et al. 2002) BUDDA (de Souza et al 2004) GASP2D (Méndez-Abreu et al. 2008) IMFIT (Erwin 2015) B/T = Lbulge/(Ltotal) D/T = Ldisc/(Ltotal) Bar/T = Lbar/(Ltotal) B/T + D/T + Bar/T = 1 Méndez-Abreu et al. 2017 or even in 3D! New attempts to perform spectra-photometric decompositions of galaxies using integral field spectroscopy BUDDI (Johnston et al. 2017) C2D (Méndez-Abreu et al. 2019a, Méndez-Abreu et al. 2019b, Méndez-Abreu et al. 2021)