Lecture Notes - Bioinformatics - Protein Structure - PDF
Document Details
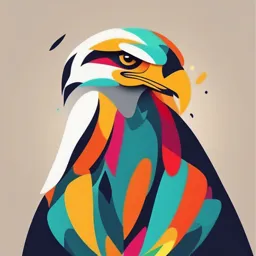
Uploaded by ConvincingOak
Imperial College London
Mike Sternberg
Tags
Related
- Computational Molecular Microbiology (MBIO 4700) Fall 2023 Lecture Notes PDF
- Computational Molecular Microbiology (MBIO 4700) Lecture Notes PDF
- Areas Related to Biochemistry PDF
- Computational Protein Structure Prediction PDF
- Protein Structure Prediction Lecture PDF
- Structural Bioinformatics Lecture 8 PDF
Summary
These lecture notes provide an overview of protein structure, covering topics such as protein backbone, chirality, amino acid residues, and thermodynamics of protein folding. The notes also touch upon hydrophobic interactions and electrostatic interactions.
Full Transcript
Bioinformatics -- Mike Sternberg Table of Contents {#table-of-contents.TOCHeading} ================= [Lecture 1 -- Protein Structure 1](#lecture-1-protein-structure) [Lecture 2 -- Protein Sequence Analysis 1](#lecture-1-protein-structure) [Lecture 3 -- Protein Structure Prediction 25](#lecture-3...
Bioinformatics -- Mike Sternberg Table of Contents {#table-of-contents.TOCHeading} ================= [Lecture 1 -- Protein Structure 1](#lecture-1-protein-structure) [Lecture 2 -- Protein Sequence Analysis 1](#lecture-1-protein-structure) [Lecture 3 -- Protein Structure Prediction 25](#lecture-3-protein-structure-prediction) [Lecture 4 -- Structural Bioinformatics 32](#section-4) ### Lecture 1 -- Protein Structure Hierarchy of Protein Structure: Primary -\> Secondary -\> Tertiary -\> Quaternary **Protein Backbone** 1.17: Protein Structure - Biology LibreTexts **Chirality of Amino Acid Residue** The Ca is **chiral** -- look down to the H to Ca and spell CO-R-N clockwise for the **L**-form.  **Amino Acid Residues** \- It should be noted that Glycine is much more **flexible** than Alanine for example, as it has a H rather than a side chain. \- Proline has less backbone flexibility compared to all other amino acids due to the covalent bond with amide nitrogen, thus, proline imports a **rigidity** to the chain and has less degrees of freedom for rotation. \- Cystine forms the only **covalent crosslink** -- the disulphide bridge, which is highly conserved. #### Protein Primary Structure Definition: a **polymer** of amino acid residues whose chemical formula termed the amino acid sequence. It consists of a fixed main-chain with variable side chains, and every amino acid residue has a **hand** (usually the L form) as there is generally 4 different chemical groups attached to the carbon alpha atom causing **chirality**. **Non-Bonded Interactions** \- Ionic Bond \- Van der Waals Interactions \- Hydrogen Bond **Thermodynamics of Protein Folding** \ [*ΔG*= *ΔH* − *TΔS*]{.math.display}\ Where: ∆G -- Free energy of folding ∆H -- Enthalpy (e.g. electrostatics and packing) T -- Temperature ∆S -- Entropy (systems favour disorder) **Packing and Hydrophobic Interactions** \- All atoms prefer to pack as touching hard speres, this is known as **van der Waals** interactions. - Groups of CH atoms often have little charge and are termed hydrophobic/non-polar. \- It is energetically favourable for hydrophobic groups to **pack together** to avoid contact with solvent, this hydrophobic effect is the main effect favouring the folded protein. **Hydrophobic Effect** Bulk water can adopt many **conformations** making hydrogen bonds, **disorder** is high here and so it is unfavourable for water to pack with non-polar residues as this prevents orientation of the water next to the non-polar residue. Adding non-polar residues **freezes** the number of **degrees of freedom** of the water and so is **entropically unfavourable**. **Electrostatic Interactions** **+ve** polar charge on H of main-chain NH group, and H on NH- groups of some sidechains. **-ve** polar charge on O of main-chain CO group, and O on CO groups of some sidechains. Favourable **+...- interaction** between partial charges is called a hydrogen bond, and between fully charged side chains are called salt bridges. **Energetics of Electrostatic Effects** The formation of electrostatic interactions is at best only **marginally favourable** in the folded protein. Unfolded chain, with two hydrogen bonds between protein and water -\> Folded chain, with 1 hydrogen bond intra-protein and 1 hydrogen bond between water. This has slightly more **net stability**, even though it is the same number of hydrogen bonds. One almost never finds **un-paired** main chain NH and CO groups or charged side chain atoms. This constrains the possible shapes that the chain can fold in and makes evolutionary change of a non-polar residue to charged residue unlikely. Proteins fold to avoid this and make **salt bridges** to avoid an **uncompensated** buried charge. **Entropic Effect** It is energetically **unfavourable** to restrict conformation of an unfolded chain. The unfolded chain can tumble between many conformations and has many degrees of freedom, while the folded chai is very restricted to local fluctuations and thus, has **less degrees of freedom** (less energetically favourable). #### Protein Secondary Structure Definition: **Local** conformation of the main chain (doesn't have to be repetitive). There are two common types of repetitive structures: **alpha helix** and **beta-strand sheet**, and also a characteristic non-repetitive local structure: **beta-turn**. **Alpha Helix** The alpha helix is the **most common** structural arrangement in the secondary structure of proteins. It is also the most extreme type of local structure, and it is the local structure that is **most easily predicted** from a sequence of amino acids. The alpha helix has a **right hand-helix conformation** in which every backbone N−H group hydrogen bonds to the backbone C=O group of the amino acid that is **four residues earlier** in the protein sequence. **B-Sheets** Beta sheets consist of beta strands (β-strands) connected laterally by at least two or three backbone **hydrogen bonds**, forming a generally twisted, **pleated** sheet. Because peptide chains have a **directionality** conferred by their N-terminus and C-terminus, β-strands too can be said to be **directional**. Adjacent β-strands can form hydrogen bonds in **antiparallel**, **parallel**, or mixed arrangements. In an **antiparallel** arrangement, the successive β-strands **alternate** directions so that the N-terminus of one strand is adjacent to the C-terminus of the next. This is the arrangement that produces the strongest **inter-strand stability** because it allows the **inter-strand hydrogen bonds** between carbonyls and amines to be **planar**, which is their preferred orientation. In a **parallel** arrangement, all of the N-termini of successive strands are oriented in the same direction; this orientation may be **slightly less stable** because it introduces **nonplanarity** in the inter-strand hydrogen bonding pattern. **B-Turns** These cause a **change in direction** of the polypeptide chain. There are four categories: Type I-IV. **Type I** is most common, because it most resembles an alpha helix. Type II beta turns, on the other hand, often occur in association with beta-sheet as part of beta-links. **Energetics** Alpha helices and beta sheets are **not** primarily stabilised by hydrogen bonds as electrostatic effects are not the driving force for protein folding. These periodic (regular repeating) structures are the **best way** to **bury** hydrophobic residues without burying the uncompensated partial charges of the main-chain NH and CO groups. **Dihedral Angles** A **Ramachandran plot** is a way to visualize energetically **allowed regions** for backbone dihedral angles **ψ against φ** of amino acid residues in protein structure. In a protein chain three dihedral angles are defined: **ω (omega)** is the angle in the chain Cα − C\' − N − Cα, **φ (phi)** is the angle in the chain C\' − N − Cα − C\' **ψ (psi)** is the angle in the chain N − Cα − C\' -- N The **side chain dihedral angles** are designated with χn (**chi**-n). They tend to cluster near 180°, 60°, and −60°, which are called the trans, gauche−, and gauche+ conformations. The stability of certain sidechain dihedral angles is affected by the values φ and ψ. These are called side chain **rotamers**, the allowed positions of the side chains. **Solvent Accessible Area** Solvent Accessible surface area (SASA) is the surface area of a biomolecule that is accessible to a solvent. Measurement of ASA is usually described in units of square angstroms. ASA is typically calculated using the \'rolling ball\' algorithm developed by Shrake & Rupley in 1973. This algorithm uses a sphere (of solvent) of a particular radius to \'probe\' the surface of the molecule. Relative solvent accessible area = Aobs/Ai Aobvs = total calculated solvent accessible area for a residue Ai = solvent area for residue type i If the atom is **buried**, then the **area = 0**. If the atom is highly **exposed**, then the **area = 1**. #### Tertiary Structure Definition: The **three-dimensional** structure of a single chain. The structure is revealed at near atomic resolution by X-ray crystallography, NMR and electron microscopy. The **core** contains **hydrophobic** residues, and charged atoms are nearly always stabilised by electrostatic interactions. The **surface** contains charged atoms interacting with water, and a substantial number of non-polar atoms despite the fact this is not favourable. This is because proteins have to interact with other proteins and so need sticky patches. NOTE: Proteins have **marginal stability** (10kcal/mole) (Proteins can't be too stable as we need to recycle them -- degrade them). #### Quaternary Structure Definition: **Arrangements** of different chains, generally symmetric. There are three **categories** of proteins: \- Transmembrane \- Globular (generally water soluble) \- Enzymes and antibodies \- Fibrous \- Elongated and generally not soluble (e.g. silk, muscle and collogen). **Fold/Topology** Definition: The **sequential arrangement** of chain sections, particularly alpha helices and beta strands. **NOTE**: Larger proteins fold into **domains**. **Protein Domains** Often a protein sequence is formed from parts known as domains, where each domain is a different **homologous family**. Domains are generally a **distinct structural unit**, and a **distinct evolutionary unit.** Domains can be classified into different **fold classes**: \- **α/α**: mainly packing of alpha helices \- **β/β**: mainly one or more beta sheets \- **α/**β: roughly alternate alpha and beta with beta-sheet tending to be parallel \- **α+β**: mixed alpha and beta \- **coil**: mainly small proteins (\35%). **SCOP2** This has a more **complex** hierarchy. **CATH** *Domain Classification* C -- fold **CLASS** A -- fold **ARCHITECTURE** (describes general features of several folds e.g. doubly would β-sheet.)\ T -- fold **TOPOLOGY** H -- **HOMOLOGOUS** superfamilies S -- **SEQUENCE** families (by ID) #### Structural Comparisons Identifying if all or part of one protein structure is similar to another is important when a new structure is solved to see if it is similar to a known fold, and if so, then it can be used to suggest evolution and function \--\> this is a central tool in classification systems. *Structural Similarity Searches* DALI, CATHEDRAL and FOLDSEEK have been used to create www based structural similarity search engines: - The DALI server is based at the EBI and is widely used. - CATHEDRAL is linked to CATH and UCL. - FOLDSEEK is new and very fast *FOLDSEEK* This is a major advance. It converts residue-residue interactions into linear sequence and uses sequence searching for speed. READ PAPER **EzMol** This is a graphics program designed for occasional users and works over the web (wizard driven via tabs). This produces images for publication. ### ### Lecture 2 -- Protein Sequence Analysis #### #### Primary DNA Sequence Databases \- Genbank (NCBI) \- ENA (EMBL) \- DDBJ Data is **exchanged** between these sights nightly, so they have the same core data. Initial DNA deposition translated into protein sequences: \- Genbank to **Genpept** \- EMBL to **TrEMBL** In parallel **Swissprot** is a high-quality source of **annotation** for some sequences. *UniProtKB* This is from Swissprot with high quality annotation. There are now **240M** entries, there has been a huge expansion in sequences. *Problems and Errors in Databases* \- Organisation of databases **changes** rapidly \- The names or proteins are very **variable** \- The errors are very **slow** to correct \- Sometimes will **not work** on a browser *Metagenomics* Work pioneered by Craig Ventor to obtain sequences in batch from **microorganisms** in exotic locations such as the middle of the ocean or the human gut to give an insight into **biodiversity**. However many sequences are of **poor quality** and are often **fragments**. **MGnify** database at EBI has 350,000 **amplicons** from 33,000 **metagenomes**. Detecting Evolutionary Relationships To detect evolutionary relationships, we need to **quantify** the **similarity** between the species. We can do this by pairwise protein sequence alignment. Orthologues and Paralogues There are two different events during evolution: gene duplication and speciation. Gene duplication is when a gene is duplicated within a genome and the two proteins and they are called paralogues. - This can result in a change of function, as only one copy is required to provide the original protein, so the second gene/protein can evolve a new function. - Speciation is when a new species is created. As a result the two species have a single copy of the same gene, and the two proteins are called orthologues. - Both species only have a single copy, so their function is less likely to change. #### Pairwise Protein Sequence Alignment *Requirements* A **scoring scheme** of **similarity** of amino acid residues and an algorithm to establish the alignment. The aim is that the **combined** use of the **algorithm** with the **scoring scheme** generates the best alignment in terms of the biology and has the potential to be **extended** to database scoring. *Scoring Scheme* The simplest way is to **score 1** for identical amino acids and 0 for different ones (similarly, identical bases can be scored). However, for proteins, **evolution** imposes **constraints** on types of amino acid changes that generally occur to modify, but not destroy protein function. Residues tend to keep their chemical property, e.g. the tendency to be **buried** (i.e. non-polar or hydrophobic residues). The maintenance of chemical property is called conservative substitution. **Point Accepted Mutation (PAM)** This was developed by Dayhoff in around 1978 (founder of bioinformatics). This is based on counting the number of times residue types changed in aligned sequences of closely homologous sequences, it can be extended to more distant relationships by assuming the matrix can be multiplied by itself. **PAM 250** was developed to model sequences with **20% identity**. First, it **quantifies the odds** that one residue is mutated from another from: \ [\$\$odds\\ score = \\ \\frac{\\text{observed\\ probability\\ of\\ amino\\ acid\\ pair\\ exchanging}}{\\text{probability\\ of\\ exchange\\ due\\ to\\ chance}}\$\$]{.math.display}\ **NOTE**: For a residue that is not mutating, the odds score represents odds that the residue resists mutation.  As we need to multiply the odds along the sequence, it is easiest to take a log and add, hence **log odds score**. The matrix represents the **chemical properties** so hydrophobic residues that substitute score are favourable as they are often observed. However, the problem is that this was based on examination of **close homologs** and we need the matrix for difficult cases of remote homology. This matrix captures what we can **expect chemically**. **BLOSUM62** **BLO**cks **S**ubstitution **M**atrix. This was derived by Henikoff and Henikoff in the early 90s. It is based on aligned sequences of protein families called BLOCKS. BLOSUM62 includes clustered sequences in BLOCKS where pairwise identity \> 62%. It looked at the **conserved blocks** and **ignored the loops** to try and amplify the signal over the noise. It has a similar calculation to the PAM250 matrix, however BLOSUM62 is the most **accurate** and **sensitive** and so it is the **most widely used** matrix and included in the BLAST/PSIBLAST family of database searching algorithms. *Gap Penalties* Penalise gaps (**indels**). Penalty = o + e ⋅ l Where o = the gap **opening** constant (10) e = gap **extension** constant (1) l = **length** of gap extension o \> e as the **evolutionary event** is making the gap and we often see long gaps. #### Alignment Methods *Protein Domains* Often a protein sequence is formed from parts known as domains, where each domain is a **different** homologous family. Domains are the **evolutionary unit**. *Local vs Global Alignment* **Needleman-Wunsch Algorithm** This is a general algorithm for sequence comparison, it **maximises a similarity score** to give a maximum match. Finds the best **GLOBAL** alignment of any two sequences. Maximum match = largest number of residues of one sequence that can be matched with another allowing for all possible **indels**. The N-W involves an iterative matrix method of calculation: \- all **possible** pairs of **residues** (one from each sequence) are represented in a **2-dimensional array**. \- all possible alignments are represented by **pathways** through this array. Three Main Steps: 1\. Assign **similarity values** 2\. For each cell, look at all **possible pathways** back to the beginning of the sequence (allowing for indels) and give that **cell** the value of the **maximum scoring pathway**. 3\. Construct an **alignment** (pathway) **back** from the highest scoring cell to give the highest scoring alignment. *Similarity Values* S~ij~ is the **numerical value** that is assigned to every cell in the array depending on the similarity/dissimilarity of the two residues. It uses the **BLOSUM62** matrix for this.  *Constructing the Alignment* The alignment score is **cumulative** by **adding** along a path through the array. The best alignment has the **highest score** i.e. the maximum match. Maximum match = the largest number resulting from summing the cell values of every pathway, the maximum match will always be somewhere in the **outer row** or **column** shown. The alignment is constructed by working backwards from the maximum match, as you **trace back** the best path through the matrix introducing **gap penalties**. When a gap penalty is introduced, the next step is: **Best of** { Just continue the alignment Add gap in vertical sequence Add gap in horizontal sequence } **Smith-Waterman Algorithm** Instead of looking at each sequence in its entirety this compares segments of all possible lengths (**LOCAL** alignments) and chooses whichever **maximises** the **similarity measure**. **Dynamic Programming** Following its introduction by Needleman and Wunsch (1970), dynamic programming has become the method of choice for **''rigorous'' alignment of DNA and protein** sequences. For a number of useful alignment-scoring schemes, this method is guaranteed to produce an alignment of two given sequences having the highest possible score. It also **allows gaps**. #### Database Searching Query sequence + Database of sequences Comparison algorithm list of similar protein sequences infer homologous similar structure and often similar function. *Fast Pairwise Search Algorithms* Single query aligned independently to any similar database entry, but it must perform a **local search**. The Smith-Waterman is guaranteed to find a mathematically **optimal solution** but is too slow for searching except on specialist parallel processing computers. Various **fast methods** have been developed based on finding short local matches and then building up the alignment. These methods are good, but they are **not guaranteed** to find a mathematically **optimal** solution. FATSA -- popular method developed in 1985 but is no longer widely used. **BLAST** -- this is now the **major** sequence search tool in protein and DNA bioinformatics. **BLAST\ **This is a highly **sophisticated** approach developed by Altschul in 1990 and is a very fast local search program (**50x the speed** of the Smith-Waterman algorithm). 1\. First it finds short segments or **seeds** (known as **words**) in the query that have **matches** in the database using the **BLOSUM62** score. 2\. Then it **extends** suitable seeds to form **HSPs** (high scoring pairs) using ungapped and gapped alignments. The significance of a HSP match of a given length is evaluated by precise statistics. BLAST is also used for **DNA/DNA** and **Protein/6 frame DNA translation**. **PSI-BLAST** has also been developed that uses multiple sequences. *Accuracy of Database Searching* Need a **cut off score** to assign positives and negatives -- do this by P and E values. *Reliability of a Match* P(S) is the **probability** of achieving a score S or a better score by **chance** (P is a **cumulative** score). Also use a related measure which is the expectation of an error in the database scan (**E-value**). E(S) is the expected number of chance occurrences of scores equal or better than S. *E-Values* E-value is the **expected number** of matches that are **errors** if you searched and took all matches up to and including S. Essentially the E-value is the estimated **number of false positives** found using S as the cut off. Most search programs return one or both of these values, and values do consider the size of the database searched and the score of the match. **BLAST** also considers the **length of the match** as short matches are easier to find. For matches **\< 20 residues** you must be very cautious in suggesting true homology, and you **cannot** infer short matches will have a similar **3D structure**. Confident if P or E \< **10-3** but these are estimated values and could be wrong. Also, you **cannot compare** E-values from different programs as they all calculate them differently. Note that P is a probability and so P \ 1 means it is favourable. Don't use energy calculations because the conformation won't be correct, as there will be conformational changes. Step 3 -- Search for **clusters** of similar complex **geometry** with **low energy**. Step 4 -- **Refinement** Step 5 -- **Functional** residues information (this can be added at any stage) \- This is found from **literature** CAPRI and CASP These are ways to do a **blind evaluation** of docking. If unbound or homology-built molecules are docked without knowing solution, the results must be **evaluated**. Often the knowledge of a binding site is employed as well as **human inspection**. **NOTE**: **ClusPro** is a powerful **ab initio** protein docking server. Template-Based Prediction As we have **known structures** for complexes, we can use an approach similar to template-based prediction of protein structure, where the idea is to inherit a structure of an unknown complex of a **known template**. If A' is homologous to A and B' is homologous to B, and AB have a known X-ray structure of their complex, then the assumption is that they will **bind** in a **homologous** way. If the A'/B' interface is favourable when evaluated in 3D then the **prediction** is that they will **interact**. Template library is a **library of complexes**, then do a **sequence search** of the two sequences against the template library. Need to match both chain A and chain B, then you can make the 3D model. Step 1 -- **Sequence Search** \- Start with **sequence** protein A and protein B \- Based on sequence similarity, **search** the library of complexes in PDB for a complex A'/B' \- **Align** sequences A to A' and B to B' \- Sequence search can be via **BLAST, PSI-BLAST** or **Hidden Markov Models**. Step 2 -- **Model Construction** \- On 3D structure of the complex, **change the sequence** from A' to A and B' to B \- **Adjust any loops** where there is an indel \- **Refine** the complex Step 3 -- **Alternate Model Selection** \- Sometimes there can be **several suitable templates** as several have similar sequence identity. \- Construct **several models** \- **Score** models (similar to ab initio docking) \- Choose the **best model** \- NB this this one approach -- there are several variations of template-based modelling. *Accuracy and Coverage* \- If both queries **\>30% identity** to the templates in the complex, then they are typically **acceptable** models according to the CAPRI criteria and can be obtained for 2/3 of predicted complexes. \- For \~450,000 human protein-protein interactions, **8%** have **X-ray or NMR** structure, and a further **20-40%** by **template docking** -- we still have a long way to go. Deep Learning The concept of **correlated mutations** also extends to homo and **hetero complexes**. We can join two sequences of two chains and then run AlphaFold. This is an active area of research where the results are very encouraging, we will know more about its accuracy after CASP15. *AF2Complex* You can add **multiple sequences together** and run them all through **AlphaFold2** as if it is one protein, to build a model. *ColabFold* It will fold the **individual sequences** and **dock** them together -- fold and dock. Note that **heterodimers** work **better** as the **correlated mutations** tend to be a stronger signal. Even though you can get some remarkably **good predictions**, this is still a work in progress. *CASP15 Results* **Huge improvement** of score from CASP12-CASP15. *AlphaFold3* There is a substantive **improvement in protein-protein docking**, and a very large improvement in docking antibodies to proteins seeing as there is **no co-evolution** to guide docking. Awaiting blind trails in CASP16 (December 2024). *Best Approaches* Typically use careful identification of **multiple sequence alignment** followed by **AlphaFold Multimer** or similar. Manual groups also used rtemplates if AlphaFold was not successful, and also some groups used scoring interfaces. ### Lecture 4 -- Structural Bioinformatics #### Protein Function Gene Ontology The enzyme classification system is only enzyme functions, whereas gene ontology gives **functions beyond enzymes.** It is a controlled vocabulary that can be applied to all organisms, and can be used to **describe gene products** (proteins and RNA) in any organism. All descriptions are supported by some level of **evidence**. It captures information about 3 important features of function: \- **What** does the gene product do? -- Molecular function \- **Why** does it perform these activities? -- Biological process \- **Where** does it act? -- Cellular component 3 Gene Ontologies \- **Molecular Function** = biological function \- **Biological Process** = biological goal/objective (higher level function) \- broad biological goals, such as mitosis or purine metabolism \- **Cellular Component** = active location *Evidence Codes* The **annotation source** is important, it enables you to assess how confident the annotation is. GO associates annotations with an evidence code that indicates its score. Sources for Annotations **- Papers** **- Experiments** \- Computational analysis \- Automated predictions/analysis The first two are the most **reliable** as they have the most **robust** information, and we would only use these as **training data** for an algorithm. Uses of GO Enhanced predictors of protein function return **prediction of GO terms**. Common features in a set of over-expressed genes can be reported as belonging to a common GO group. If you do RNA-seq, look if you have a set of **highly expressed genes** and whether they all belong to a **similar** **GO term**, this can give you can idea of **related function** or where the activity is. **Clustering** terms is often used to get a picture of an integrated view of protein function. Function Prediction There are about 240 million sequences, where the time taken experimentally to determine function can be several years. Thus, to predict function, we use methods that **exploit protein homology** and protein families. *Approaches:* General homology search \- **BLAST** (single sequence information) \- **PSIBLAST** (multiple sequence information) \- **HMMs** scan (multiple sequence information in HMM) \- **Pfam** library *Method:* Query sequence + Database of sequences Comparison algorithm (BLASTP, PSIBLAST, HMM) list of similar protein sequences infer homologues, similar structure and often similar function. *Types of Homologous Proteins* **Orthologs** These are homologues created by **speciation**, and typically have the **same function** in **different species**. You can **transfer the function** with a high degree of **confidence**, as they usually have almost identical functions and the same 4 digits of EC if they are enzymes. Also, will have a closer **sequence identity** than paralogs. Example: **Human chymotrypsin** and **Bovine chymotrypsin** -- preferentially cleave peptides after aromatic Leu, have the same 4 digit EC code and have **85%** sequence identity. **Paralogues** These are homologues created by **gene duplication** **within a species** from which they **evolve independently**, these typically have **related** functions. Example: **Human trypsinogen** / **Human chymotrypsin**, have different 4^th^ digit of EC and only **38%** identity. *%ID and Functional Transfer* To some extent these concepts about EC numbers apply to all types of function but there are no clear-cut rules, and function is challenging to quantify. Start with a query sequence and perform a BLASTP search: \- If you find a protein from **another species** with **\>\~50% identity** then it could easily be an **orthologue** with the same function. \- If you find a protein from **another species** with **\>\~30% identity** then it could be a **paralogue** and have a related function. \- If you find a protein from the **same species** with **\>\~30%** identity then it could be a **paralogue** and have a related function. These concepts are incorporated into software, where it is safe to **transfer function** between **orthologues**. Domains Another complication of automatic transfer is that proteins have domains. **Homology-Based Predictions** Search programs identify **local similarities**. Two sequences may share a region/domain of similarity but also other domains that are **different**. As not all domains are shared the function the function is probably different. This is the **danger** of automated predictions based on **homology**, so you should do a **full-length match** and not just a local match you get from BLAST or PSIBLAST. **Pfam** is a **domain-based** approach and can **circumvent** this problem by searching specific domain libraries. **Specific Domain Libraries** Specific libraries with **functional annotation** can be searched via **InterPro** at EBI. Avoid problem of **inheriting function** from a homologue where we only **match one domain** by looking at: \- Prosite **motifs** (short patterns good for enzyme active sites) \- Profite **profiles** (more extensive profiles -- e.g. a carrier protein will have a sequence pattern for the function that extends across the sequence) \- **Pfam** (HMMs of domains) However, all of this requires that the **family has been added**. It is generally **easier to interpret** than homology transfer, but there is **less coverage**. **Advanced Sequence-Based Approaches** Groups develop approaches based on sequence concepts but integrating **different sources of information**. One concept is to say that proteins that **interact** will have **similar functions** (i.e. use the **interactome**), an example of this is the STRING database. Other approaches integrate many sources of information e.g. NetGo. String Database This identifies proteins that **interact** with a **query protein**. It uses different sources of information with different reliability. It gets known interactions from curated **databases** that have been **experimentally determined**. It also contains **predicted** interactions from a variety of bioinformatics methods and **text mining** (finding in literature). NetGo2.0 This is a **machine learning** approach, where you start with the query sequence and it uses k-nearest neighbour using the BLAST result, InterPro features, frequency of amino acids and k-nearest neighbour of STRING. This integrates lots of **sources of information** and did quite well. There is a blind test for function prediction known as **CAFA**, where they try to **predict GO terms**, and NetGo2.0 performed well. NetGo3.0 Added in a **protein language model** that replaces some of the earlier components, made very **little difference** -- didn't transform the accuracy. **Structure-Based Approaches** Match 3D **structures** (**superpose** them) and if there are similar **functional residues** this suggests related function. This way you can find hits that you can't identify by sequence because they have such a **remote relationship.** Example: **Integrase** and **Ribonuclease** **H**, where their active sites match so we can transfer the function. NOTE: Structure-based approaches **enhance your confidence** in functional annotation. *Structural Searching* Take a structure, **align** it with a database of structures and you get a **score**. Use **structural searching** to identify similar fold (**DALI**, CATH or Foldseek), as a similar 3D structure may suggest a related function. If the **functional site** in the **match** is known, then examine if there are similar residues in your newly determined protein. This gives a **higher degree of confidence** of functional transfer, even though you can never be certain. *FoldSeek* As structure prediction methods are generating millions of publicly available protein structures, searching these databases is becoming a **bottleneck**. Foldseek **aligns** the structure of a query protein against a database by describing tertiary amino acid interactions within proteins as sequences over a structural alphabet. Foldseek **decreases computation times** by four to five orders of magnitude with 86%, 88% and 133% of the sensitivities of Dali, TM-align and CE, respectively. *Convergence of Active Sites* Cannot do this if you have **convergent evolution**. For example, even with **gamma-chymotrypsin** and **subtilisin**, structure-based approaches looking at the **global fold** would not find this relationship. People have tried to search for the **local 3D patterns**, but it gives a lot of **false positives**. The way you would tackle this would be via **STRING**, where you look at the interactions, but it wouldn't give you any **specific data**. #### Prediction Non-Globular Regions Apart from the ends, the protein chain in the membrane is in a **non-polar** (hydrophobic) environment, thus, the **sidechains** tend to be **hydrophobic**. Main-chain cannot have NH and CO groups not forming hydrogen bonds -- they do not like to be **uncompensated** for in a hydrophobic environment, so they form an **alpha-helix**. However, note that not all membrane bound proteins are formed of alpha-helical segments. *Bacteriorhodopsin* This was the first membrane protein revealed by Cryo-EM and is a classic example of a 7 TM GPCR, with **7 alpha helices.** *Porin* This is a **beta-barrel**, and is the only other structure where you can **satisfy all the H bonding** other than alpha-helices. Although, they are found more in **bacteria**. *Transmembrane Helices* These span the hydrophobic section of the **membrane** about 35-50A wide. This section is not translocated (**fixed**) that abuts membrane often contains +ve charges. Each residue in an alpha helix advances the structure by **1.8A**, and transmembrane helices tend to be between **20-30 residues long**. Methods to Identify TM Regions **Hydrophobic Residue Search** Early methods searched for **runs** of very non-polar (hydrophobic) residues along a sequence. Then use a scale of how hydrophobic each residue is using either the **Hopp and Woods scale** or the **Kyte and Doolitle scale**, calculated typically over a window of **11 residues**. *Hydrophobic Plots*  The red line is the threshold. Here there are two well predicted membrane spanning regions. *Signal Peptides* Signal peptides refer to the sequence at the **start of proteins** ranging from **15-60 residues**, that direct the protein to the **correct cellular location**, these are typically **cleaved** off. They often have a **hydrophobic region** followed by a pattern typical of the **cleavage** site, however, in predictions we need to try and **distinguish** transmembrane regions from signal peptides. **DeepTMHMM** Until recently, prediction methods developed **HMMs** based on aligned sequences. The state of the art now is DeepTMHMM, which is a **deep learning** approach that predicts transmembrane structures and signal peptides. The algorithm predicts how the sequence **maps** onto the different potential states from the N- to the C- terminus, with a **stepwise approach**. *Low Complexity Regions* Regions with composition **biased** strongly to a small number of amino acids are very uninformative, but occur in the sequences of a **significant** number of proteins (often in disordered regions). They **distort** the statistical significance scores of alignments, they **pollute the PSSM** and lead you into the **wrong part of space**. The program **SEG** is often used, where it replaces low complexity regions with lower case in **BLAST** searches at NCBI. *Coiled-Coils* These are two or three **intertwined alpha helices** and be a short segment or far longer. They are identified using COILS. *Disordered Proteins* Some Globular proteins have small regions which are disordered and **cannot** be identified with crystallography or NMR, often at the **N- or C- terminus** or a **long loop**. Other proteins do not adopt a single structural conformation but are instead highly **flexible**, this flexibility allows **protein recognition**. Often, proteins become **structured** when a protein **binds** to another protein. *Prediction of Disorder* Based on principles, they tend to have a **lower** fraction of **hydrophobic** residues than the folded protein with a hydrophobic core. Prediction is machine learning based, as most programs are now based on **neural networks** or **support vector** **machines**. Examples are PONDR-FIT and **DISOPRED2**. You can also use AlphaFold prediction where the confidence given by **pLDDT \< 70%**. *fIDPnn Disorder Prediction* flDPnn produced the most accurate **predictions of disorder** (AUC = 0.814) and the fully disordered proteins (i.e., proteins for which disorder covers at least 95% of their sequences) in CAID. Moreover, flDPnn generates **putative functions** for the predicted IDRs covering the four most commonly annotated functions, including **protein-binding**, **DNA-binding**, **RNA-binding**, and **linkers.** **NOTE**: AlphaFold pLDDT indicates disorder. DisProt - Database of Disordered Proteins DisProt is the **gold standard** database for intrinsically disordered proteins and regions, providing valuable information about their **functions**. They show that DisProt\'s curated annotations strongly correlate with disorder predictions inferred from **AlphaFold2 pLDDT** (predicted Local Distance Difference Test) confidence scores. **Drug Discovery Pipeline** Definitions - **Target**\ A protein or molecule whose activity is modified to achieve therapeutic effects. - **Hit**\ A small molecule identified through biological or computational screening with the desired effect (typically IC₅₀ ≤ 1 µM). - Hits may come from commercially available compounds or custom chemical libraries. - **Limitation**: If purchasable, the compound lacks novelty, meaning you cannot secure a '*composition of matte'r* patent for it. - **Lead**\ A chemically optimized version of a hit, designed to: - Enhance therapeutic efficacy. - Minimize adverse effects, including toxicity and poor absorption. Clinical Development Phases 1. **Clinical Phase I** - **Purpose**: Determine a safe dosage and assess side effects. - **Participants**: 20--80 individuals (often healthy volunteers). - **Method**: Gradual dose escalation with close monitoring of absorption and adverse effects. 2. **Clinical Phase II** - **Purpose**: Refine Phase I results, focusing on side effects and initial effectiveness. - **Participants**: 100--300 patients (usually with the target condition). - **Outcome**: Identifies potential therapeutic benefits. 3. **Clinical Phase III** - **Purpose**: Definitively prove effectiveness and further evaluate safety. - **Participants**: Thousands of patients in large-scale trials. - **Method**: Randomized comparison with placebos or existing treatments. - **Outcome**: Provides the robust data needed for regulatory approval. Post-Clinical Stages - **Regulatory Phase** - Submission of data to obtain approval for the drug's use and marketing. - **Sales and Monitoring Phase (Phase IV)** - Purpose: Post-marketing surveillance to monitor long-term safety and effectiveness. Patent Timing Challenge - **Risk of Early Patenting**: The drug might fail clinical trials, leading to wasted resources. - **Risk of Late Patenting**: Competitors may develop and release similar drugs, undercutting exclusivity. **Computer-Based Drug Design** There are three types of modelling: - You know the **activities** of a set of ligands but do not know the structure of the receptor. - You know the **structure of the receptor** but not of the active ligands. - You know the **structure of the complex** of the receptor and the ligand(s). Ligand Screening *Step 1 -- Derive a QSAR* **QSAR** = Quantitative Structure Activity Relationship = A **mathematical relationship** relating activity to the structure of a molecule. Start with a set of ligands with known activity to derive a QSAR. *Step 2 -- Use the QSAR to score new molecules.* *Step 3 -- Dock ligands into the receptor using virtual screening.* **Input**: Structure of the target protein + Database of small molecules Dock into site and test the high scoring molecules. **Output**: Structure determination + new inhibitor design. Docking Methods *Traditional Methods* - Search by adjusting the conformation of the **ligand** and the targets side chains (**NOT** its main chain). - Score using a **simplified atom/atom score** of energy calculations. - We don't want precise Van der Waals interactions as they tend to fail when they can't model conformational change. - Examples are AutoDock Vina and GLIDE. *Advanced ML Methods* - Some use ML to derive better **scoring functions** -- DeepDock. - Some use ML for **searching and scoring** -- DiffDock - DiffDock uses diffusion models, it takes the known complexes, diffuse it with noise and then denoise it. Evaluation of Ligand Docking -- Redocking Incorrect Approach This involves using the X-ray structure of a protein-ligand complex to test if the docking algorithm can predict the correct pose of the ligand. Typically, the algorithm explores different conformations of the ligand while keeping the protein structure mostly rigid, allowing only side-chain adjustments. This **\"lock-and-key\"** approach is **overly simplistic** and does not reflect biological reality, as proteins often undergo **backbone conformational changes** when binding different ligands. Another issue, particularly prevalent in newer machine learning (ML) approaches, is the practice of training on one protein and testing on a **homologous** protein. This method does not constitute de novo docking but instead **exploits structural** similarity, which undermines the evaluation of the model\'s true predictive capability. Correct Approach In contrast, the correct approach begins by using the **apo structure** of the protein, which is the **unbound** state, to model the **holo** structure, the **protein-ligand complex**. The docking process should then evaluate how accurately the ligand can be docked into the binding site. This approach often requires the use of **softer potentials** to accommodate the **flexibility of the protein**. Importantly, homologous proteins should be **excluded** from both training and testing datasets to prevent artificially high-performance metrics that do not reflect real-world docking challenges. A more refined strategy involves using a protein already known to bind one inhibitor and attempting to dock a different molecule into the **same site**. This method better approximates docking into an unbound state, providing a more realistic assessment of the docking algorithm\'s predictive accuracy. **NOTE**: When you see ligand docking programs, you must ask these questions about re-docking and homology. **Additional Note** If you use AF2/3 to generate your protein structure, because it has learned both the bound and unbound structures, you tend to get a **hybrid conformation**. This means you don't actually know if youre docking to the bound or the unbound form. This is an advantage of using **template-based approaches** like Phyre2.2. **Sample Results from AlphaFold3** AF3 yields **impressive results on docking**. It was used to dock a clinical stage inhibitor to it ligand - AF3 achieves accurate predictions but docking tools Vina and Gold do not. Discovery of Pfizer's Nirmatrelvir In March 2020, the **3CL protease** was identified as a suitable target for SARs-CoV-2. Pfizer already had an inhouse viable drug against this enzyme from an earlier SARS outbreak. However, this drug has **5 hydrogen bond donors** and so is very **polar**. If administered as a pill it would get trapped in the gut and so could only be used intravenously. The structure of the molecule was examined to see the parts that would bind to the receptor, and then removed the hydrogen bond donor that was not critical to this interaction. This identified **Nirmatrelvir**, with good **antiviral** activity and can be given orally to rats. They also decided to add **ritonavir**, which has no activity against SARS-CoV-2, but does bind to **metabolising enzymes**, thereby preventing nirmatrelvir from being broken down. The result was **Paxlovid**, which was approved by the FDA in July 2022. If given to Covid-19 patients within 3 days of symptoms, then hospital admissions or death was 89% lower than placebo. Due to the emergency situation of covid, the drug discovery timeline of Paxlovid was **2.25 years** compared to an average 13 years.