Lecture 9 Treskova PDF
Document Details
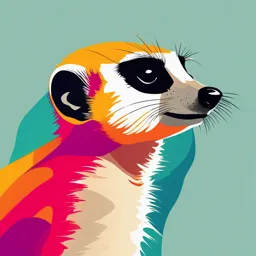
Uploaded by WarmBiedermeier
Heidelberg University
2025
Marina Treskova
Tags
Summary
This document is a lecture on integrated data analysis and outbreak prediction focusing on avian flu. It details the methodology and major results. The document also covers the concepts of SHAP vs Importance and the model's climate sensitivity.
Full Transcript
Lecture 8: Integrated data analysis and outbreak prediction: avian flu Marina Treskova, PhD Head of Research Group Eco-Epidemiology Heidelberg Institute of Globa...
Lecture 8: Integrated data analysis and outbreak prediction: avian flu Marina Treskova, PhD Head of Research Group Eco-Epidemiology Heidelberg Institute of Global Health & Interdisciplinary Centre for Scientific Computing Heidelberg University www.hei-planet.com 01/23/2025 Spillover is a key step in zoonotic disease emergence 01/23/2025 Zoonotic spillover A. Direct transmission B. Vertebrate intermediate host C. Invertebrate intermediate host D. Environment Ellwanger & Chies. 2021. Genetics and Molecular Biology. https://doi.org/10.1590/1678-4685-GMB-2020-0355 3 01/23/2025 Known zoonoses The number of included diseases is 203, of them: 70 Viral diseases 47 Bacterial diseases 74 Parasitic diseases The total number of outbreak events is 13985 01/23/2025 WHO priority diseases “Disease X” https://www.who.int/activities/prioritizing-diseases-for-research-and-development-in-emergency-contexts 01/23/2025 Clusters of zoonotic spillover 01/23/2025 Zoonoses Public Health. 2021;68:563–577. https://doi.org/10.1111/zph.12846 6 Over half of known human pathogenic diseases can be aggravated by climate change Source: Mora et al (2022). Nature Climate Change 01/23/2025 Land use and ecosystem degradation Source: Robinson et al. Lancet Planet Health 2022 01/23/2025 Biodiversity loss and dilution effect Lyme disease example High biodiversity „dilutes“ the potential of transmission 01/23/2025 https://sitn.hms.harvard.edu/flash/2022/biodiversity-loss-can- increase-the-spread-of-zoonotic-diseases/ Social drivers 01/23/2025 Rocklöv, J., Dubrow, R. Climate change: an enduring challenge for vector-borne disease prevention and control. Social drivers (cnt) 01/23/2025 One Health approach to zoonoses and prevention Prevention of zoonotic spillover: From relying on response to reducing the risk at source, the One Health High-Level Expert Panel (OHHLEP) 01/23/2025 Avian Flu model Background ResearchQuestion Themodels Main Results SHAPvsImportance 01/23/2025 13 Avian flu Avian Flu (also known as avian influenza) is an infectious viral disease primarily affecting birds but can occasionally infect humans and other animals. The disease is caused by influenza type A viruses, which are classified based on the surface proteins hemagglutinin (H) and neuraminidase (N). Common subtypes include H5N1, H7N9, and H5N6. https://rr-middleeast.woah.org/en/our-mission/one-health/highly-pathogenic-avian-influenza/ 01/23/2025 ResearchQuestion Avian Influenza (AI) outbreaks are on an increasing trajectory Spillover to humans’ risk is anticipated to grow How predicatable are these outbreaks taking into account several variables? 01/23/2025 15 TheFeatures Temperature, Precipitation NDVI, NDWI Bioclima Variables (bio1-bio19) NUTS3 SEX,AGE(65) Export, Imports GDP 01/23/2025 16 TheModels Each Model is constructed to answer specific question Model I Model II Model III - What eco-climatic and - Include wild - Include wild socio-economic bird outbreaks bird outbreaks but variables drive AIV switch off the outbreaks labels - How predictive are they? 17 01/23/2025 Model 1 49 Features 2006-2021 01/23/2025 18 Model 2 256 Features 2006-2021 01/23/2025 19 Model 3 256 Features 2006-2021 01/23/2025 20 Model Metrics 21 Main Results Test data set distribution Model prediction 94%Accuracy 01/23/2025 22 Main Results M1 The model is climate sensitive - Temperature, - Ndvi, and - Ndwi being the leading variables 01/23/2025 23 Main Results M2 24 Main Results M3 In the absence of wild bird outbreaks, Trade may have an influence in poultry disease spread. 01/23/2025 25 Main Results M1 49 Features 26 Main Results M2 256 Features 27 Main Results M3 256 Features minus wild bird labels 01/23/2025 28 Conclusion - Our model predicts AIV in Europe with an accuracy of 94% - The model is climate sensitive - The key climate indicators: - The temperature of the coldest month, - The mean temperature of quarter two and - The minimum temperature of quarter three. 01/23/2025 29 SHAP vs. Importance SHAP Feature Importance: o Based on game theory and the concept of Shapley values. o Measures the contribution of each feature by considering all possible feature combinations and the marginal contribution of each feature to the prediction. o Provides a global explanation (average importance across all samples) and local explanations (importance for individual predictions). o Takes into account feature interactions and correlations. XGBoost Standard Feature Importance: o Derived from the structure of the decision trees in the model. o Typically computed using one of three metrics: 1.Weight (Frequency): The number of times a feature is used to split the data across all trees. 2.Gain: The average improvement in the objective function (e.g., reduction in error) achieved by splits on a feature. 3.Cover: The average proportion of samples affected by splits on a feature. o Focuses on the structure and split metrics rather than interaction effects. 01/23/2025 30 SHAP vs. Importance Interpretation SHAP Feature Importance: o Provides a more nuanced explanation of feature impact by considering their interactions with other features. o Produces consistent and fair attributions based on Shapley value properties (additivity, symmetry, and efficiency). o Gives a decomposition of individual predictions, which helps understand why a particular prediction was made. XGBoost Standard Feature Importance: o Represents how often or how effectively a feature is used in the splits. o Does not account for feature interactions or correlations. o Tends to provide a high-level view, which may not always capture the true importance of correlated features. 01/23/2025 31 SHAP vs. Importance Sensitivity to Feature Correlation SHAP: o Handles correlated features better because it distributes the importance fairly among correlated features. o The Shapley value calculation considers how each feature contributes in the context of others. XGBoost: o Can overestimate the importance of features that are highly correlated or dominate splits in the tree structure. o May lead to biased feature importance metrics in the presence of multicollinearity. 01/23/2025 32 SHAP vs. Importance Granularity SHAP: o Offers both local explanations (for individual predictions) and global feature importance (aggregate across all data points). o Facilitates understanding at the instance level, making it highly interpretable for specific cases. XGBoost: o Provides only global feature importance metrics. o Limited in explaining individual predictions. 01/23/2025 33 Questions? www.hei-planet.com