Lecture 7 - DF 1.pdf
Document Details
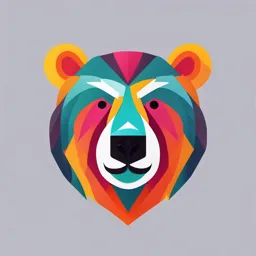
Uploaded by EyeCatchingSamarium
Tags
Full Transcript
OFFENSIVE AI LECTURE 7: DEEPFAKES - FACE Dr. Yisroel Mirsky [email protected] Today’s Agenda Introduction Threat Model Technical Background Facial Reenactment Face Replacement Dr. Yisroel Mirsky 2 3 Introduction Dr. Yisroel Mirsky Can we trust our senses? Why should we Care? 5 We like our...
OFFENSIVE AI LECTURE 7: DEEPFAKES - FACE Dr. Yisroel Mirsky [email protected] Today’s Agenda Introduction Threat Model Technical Background Facial Reenactment Face Replacement Dr. Yisroel Mirsky 2 3 Introduction Dr. Yisroel Mirsky Can we trust our senses? Why should we Care? 5 We like our fun Nicholas Cage videos Dr. Yisroel Mirsky Why should we Care? 6 The Good The Bad… 2022 Also 2022 Dr. Yisroel Mirsky Why should we Care? 7 Impersonation and falsification of media is dangerous! Social Engineering is the most common attack vector (humans are the often the weakest link!) Dr. Yisroel Mirsky Why should we Care? 8 Dr. Yisroel Mirsky 9 Introduction What is a Deepfake? Deep learning Synthetic “Any believable media generated by a deep neural network” Video (faces, scenes,...) Images (faces, medical,...) Audio (voice, music,...) Records (financial, logs,...) Deepfakes are not limited to human imagery Dr. Yisroel Mirsky 10 Introduction Deepfakes of Humans Attack Models: Impersonation Example Goals: Perform defamation Embarrassment, Pornography Threat Actors: state actor employee ...anybody Cause discredibility Spread misinformation Tamper Evidence Steal Money (Scams -abuse inherent trust) Obtain Information (Social Engineering) Cause an action (e.g., worker flip switch -also SE) Scope: Offline Online (realtime) Dr. Yisroel Mirsky 11 Introduction Deepfake Information Trust Chart Intention to mislead I. Hoax https://www.engadget.com/n etherlands-deepfake-videochat-navalny-212606049.html II. Propaganda Tampering of Evidence: Misdirection Medical, forensic, court, … Generated discourse to amplify events / facts, … Scams & Fraud: Trickery via spoofing, falsifying audit records, generating artwork, … Harming Credibility: Political Warfare: Tone change of articles, content loosely based on facts, conspiracy… Revenge porn, political sabotage via generated videos or articles, … https://www.businessinsider.co m/video-boris-johnsonendorses-jeremy-corbyn-inconvincing-deepfake-2019-11 https://www.wired.com/story/z elensky-deepfake-facebooktwitter-playbook/ https://www.technologyreview. com/2021/02/12/1018222/deepf ake-revenge-porn-coming-ban/ https://www.motherjo nes.com/politics/2019 /03/deepfake-gabonali-bongo/ https://www.forbes.com/sites/jess edamiani/2019/09/03/a-voicedeepfake-was-used-to-scam-aceo-out-of243000/?sh=665c7f9d2241 Corruption: Increased xenophobia, … https://deepai.org/ machine-learningmodel/torch-srgan IV. Trusted III. Entertainment Altering Published Movies: Authentic Content: Comedy, satire, … Credible Multimedia / Data Editing & Special Effects: https://shunsuke saito.github.io/P IFuHD/ Generating actors in movies, … https://www.theverge.com/20 19/5/10/18540953/salvadordali-lives-deepfake-museum Art & Demonstration: Animating dead characters, generated portraits, technology demos, … Truth https://www.engadget.com/lucasfil m-hires-shamook-054904077.html Dr. Yisroel Mirsky 12 Introduction Deepfake technology is free! It has also been commercialized… Dr. Yisroel Mirsky 13 Introduction When did it all start? 2014: Ian Goodfellow develops GANs 2017: Reddit user ‘Deepfakes’ swaps faces of women in explicit videos 2018: BuzzFeed demonstrated misinformation risk with Obama video 2019 – Today: Rapid development of deepfakes... 2014 2015 2016 2017 2018 … 2021 2022 14 Deepfake Basics Faces Dr. Yisroel Mirsky 15 Introduction Deepfakes of Humans 𝑠: source identity, 𝑡: target identity 𝑥𝑠 : source image, 𝑥𝑡 : target image, 𝑥𝑔 : generated image Four categories: Re-enactment Replacement Editing Synthesis Dr. Yisroel Mirsky Dr. Yisroel Mirsky 16 Introduction Deepfakes of Humans Re-enactment Generated (dubbing) (face or body) 𝑥𝑔 Dr. Yisroel Mirsky 17 Introduction Deepfakes of Humans Replacement Generated Drives: (dubbing) (face or body) 𝑥𝑔 Dr. Yisroel Mirsky 18 Face Replacement Swap Technology Reenactment Technology 𝑥𝑠 driver pose 𝑥𝑡 identity creating 𝑥𝑔 result 𝑥𝑠 driver identity 𝑥𝑡 identity Both technologies can be used in video impersonation creating 𝑥𝑔 result Dr. Yisroel Mirsky 19 Introduction Deepfakes of Humans Editing Generated 𝑥𝑔 Dr. Yisroel Mirsky 20 Introduction Deepfakes of Humans Synthesis Thispersondoesnotexist.com Dr. Yisroel Mirsky 21 Deepfake Basics Feature Representations How to present facial information to a model? 𝑥𝑠 ? → 𝐺 𝑥𝑔 Direct Representation Bad: carries distracting information about 𝑠 over to 𝑔 Requires paired training – we need to decouple identity! Dr. Yisroel Mirsky 22 Deepfake Basics 𝑥𝑠 ? → 𝐺 𝑥𝑔 2D colour image OR Multi channel bitmap Feature Representations How to present facial information to a model? Intermediate Representations Action Units (AU) 2D image to 3D morphable model (3DMM) UV maps Segmentation ▪ Uses pretrained DNN to perform semantic segmentation of image ▪ Result 𝑠 has 𝑛 channels (one for each segment type) Bad: still leaks identity Dr. Yisroel Mirsky 23 Deepfake Basics 𝑥𝑠 ? → 2D image OR Multi channel bitmap Feature Representations How to present facial information to a model? Intermediate Representations Action Units (AU) 2D image to 3D morphable model (3DMM) UV maps Segmentation Landmarks ▪ Extracts coordinates for a set number of landmarks ▪ Presented as a vector ▪ Usually extracted analytically (OpenCV) 𝐺 𝑥𝑔 Dr. Yisroel Mirsky 24 Deepfake Basics 𝑥𝑠 ? → 2D image OR Multi channel bitmap Feature Representations How to present facial information to a model? Intermediate Representations Action Units (AU) 2D image to 3D morphable model (3DMM) UV maps Segmentation Landmarks Facial Boundaries ▪ Similar to Landmarks except coordinates are joined visually in the 2D image ▪ Presented as a multichannel 2D image (each channel is a different facial feature) 𝐺 𝑥𝑔 Dr. Yisroel Mirsky 25 Deepfake Basics Common Loss Functions Each Loss Function provides a different learning signal Loss can be applied to specific outputs (or activations) to focus the training Cross Entropy Loss L1 Loss ℒ1 : 𝑥 − 𝑥𝑔 L2 Loss ℒ2 : 𝑥 − 𝑥𝑔 ℒ𝐶𝐸 : 1 2 Dr. Yisroel Mirsky 26 Deepfake Basics Common Loss Functions Can’t use ℒ1 or ℒ2 Perceptual Loss ℒ 𝑝𝑒𝑟𝑐 : For comparing images at the semantic level Compare same features, regardless of location Compare ID regardless of pose Dr. Yisroel Mirsky 27 Deepfake Basics Common Loss Functions Perceptual Loss ℒ 𝑝𝑒𝑟𝑐 : Method: Get the inner layer representation of 𝑥𝑡 and 𝑥𝑔 using model 𝐼 Measure the ℒ1 or ℒ2 of these feature maps 𝐼 is pretrained on the same domain Feature Matching Loss ℒ 𝐹𝑀 is when ℒ𝑝𝑒𝑟𝑐 is applied on a discriminator 𝑥𝑡 𝑥𝑠 → Weights of 𝐼 are locked 𝐺 𝑥𝑔 𝐼 e.g., VGGFace ℒ1 applied to the representations of 𝑥 and 𝑥𝑔 at internal layer (then back propag Dr. Yisroel Mirsky 28 Deepfake Basics Generative Neural Networks DF usually are made using a combination of 6 networks: 1. Convolutional Neural Networks (𝐶𝑁𝑁) 2. Encoder-Decoder Networks (𝐸𝐷) 3. Generative Adversarial Networks (𝐺𝐴𝑁) 4. Image-to-Image Translation (pix2pix) CycleGAN Recurrent Neural Networks (𝑅𝑁𝑁) Dr. Yisroel Mirsky 29 Deepfake Basics Generative Neural Networks Encoder Decoder (ED) 𝐸𝑛 encodes (compresses) a sample into an embedding 𝑒 𝐷𝑒 decodes (decompresses) embeddings 𝑒 captures the essence of 𝑥 depending on the loss function Common En-De networks: Autoencoder, VAE Dafoe specific or Human generic embedding of Tom Cruise’s pose and expression Example: 𝑥 𝐸𝑛 𝑒 𝐷𝑒 𝑥𝑔 Used to compress large/complex concepts into smaller (digestible) feature vectors Goodfellow I, Generative Adversarial Networks. 2014 30 Deepfake Basics Generative Neural Networks Generative Adversarial Network (GAN) Proposed by Goodfellow in 2014 𝐺 and 𝐷 play out an adversarial game 𝐷 tries to distinguish between generated from real (𝑥𝑔 vs 𝑥) 𝐺 maps random variables (𝑧) to content (𝑥𝑔 ) which will fool 𝐷 Train G Train D Training a GAN Loop: 1. Get batch from 𝑍 2. 𝐺 ← ℒ 𝐷 𝐺 𝑍 , 1 3. Get batch Xg ∪ 𝑋 4. 𝐷 ← ℒ(𝐺 𝑍 ∪ 𝑋, 𝑌) force label 1 (“real”) ground truth (0:fake, 1:real) 𝑧 𝐺 𝑥 𝑥𝑔 Networks 𝐷 𝑦 Used to generate high fidelity (realistic) content Generative Discriminator Isola P, et al. Image-to-image translation with conditional adversarial networks. 2017 31 Deepfake Basics Generative Neural Networks Image-to-Image (pix2pix) 𝑐 Maps images from domain A to domain B Let’s 𝐷 see the context 𝑐 to help evaluate the mapping Example: landmarks to Dafoe Skip connections 𝑐 𝐺 𝑥 Networks 𝑥 𝑐 𝑥𝑔 𝐷 UNET 𝑐 𝑥𝑔 Used to generate content from content 𝑦 Generative Discriminator Zhu J, et al. Unpaired Image-to-Image Translation using Cycle-Consistent Adversarial Networks, 2017 32 Deepfake Basics Generative Neural Networks Networks CycleGAN Maps images from domain A to domain B and back Uses cycle consistency loss: 𝐻𝑏𝑎 𝐻𝑎𝑏 𝑥 𝑎 = 𝑥𝑎 𝐻𝑎𝑏 𝐻𝑏𝑎 𝑥 𝑏 = 𝑥𝑏 Generative Discriminator CycleGAN 𝑥 𝑏′ 𝐻𝑎𝑏 𝑥𝑎 𝑥ො𝑎 A B 𝑥𝑔𝑎 𝐷𝑎 𝑥𝑎′ 𝑥𝑔𝑏 𝑥ො𝑏 𝐻𝑏𝑎 𝑥𝑏 𝐷𝑏 Dr. Yisroel Mirsky 33 Deepfake Basics Face DF Creation Basics: The pipeline Pipeline varies depending on the scope of 𝑥𝑔′ (whole scene, head, or just face) Dr. Yisroel Mirsky 34 DF Design Patterns Ways to Drive a Face 1. Let 𝑀 learn the mapping itself from a direct representation Example Model: Train Execute 𝑠 𝑥𝑠 𝐸𝑛 𝑥𝑡 𝐸𝑛𝑡 𝑒𝑠 𝑒𝑡 𝐷𝑒 𝑎 𝑥𝑔𝑠 𝐷𝑒 𝑏 𝑥𝑔𝑡 Problem: Artifacts in 𝑥𝑔 because 𝐷𝑒 𝑡 trained disjoint from 𝐸𝑛 𝑠 Dr. Yisroel Mirsky 35 DF Design Patterns Ways to Drive a Face 2. Train 𝐸𝑛 to disentangle identity from expression, then modify/swap encoding before 𝐷𝑒 Example Model: Train Execute VAE 𝑥 𝐸𝑛 𝑒 𝐷𝑒 𝑥𝑔 𝑒 identity 𝑥𝑡 𝐸𝑛 expression 𝑥𝑠 𝐸𝑛...... Problem: Which part of the embedding controls which attribute? 𝐷𝑒 𝑥𝑔 Dr. Yisroel Mirsky 36 DF Design Patterns Ways to Drive a Face 3. Add additional encoding (e.g., AU, LANDMARK, embedding) before 𝐷𝑒 Example Model: Execute Train Inner activation of FaceVGG Inner activation of FaceVGG 𝐼 𝑥𝑡 𝑒𝑡 𝑥 𝐼 𝑒𝑡 𝐷𝑒 𝐷𝑒 𝑥𝑔 𝑃 𝑝𝑠 𝑥𝑠 Face landmark predictor...... Problem: 𝑃 leaks the face shape of 𝑠 𝑃 𝑝𝑠 Face landmark predictor (normalized) 𝑥𝑔 Dr. Yisroel Mirsky 37 DF Design Patterns Ways to Drive a Face 4. Convert intermediate representation to that of 𝑡 before 𝐺 Example Model: Train 𝑥 𝑃 Execute 𝑝 Face landmark predictor 𝐷𝑒 𝑥𝑔 𝑥𝑠 𝑃 𝑝𝑠 𝑇𝑡 𝑝𝑡 𝐷𝑒 𝑥𝑔 Face landmark predictor E.g., trained in a self supervised manner to reconstruct landmark augmentations Dr. Yisroel Mirsky 38 DF Design Patterns Ways to Drive a Face 5. Create composite input from several representations, then refine concatenation with another network (e.g., pix2pix) Example Model: Dubbing Fried, O, et al. Text-based Editing of Talking-head Video. 2019 Execute 𝑥𝑠 𝑇 𝑥𝑠′ Refiner 𝑚 Pose transformer 𝑇 𝑥𝑡 Ugly Dafoe 𝑥𝑔 Refiners are also used to blend face in and handle occlusions Charming Dafoe Dr. Yisroel Mirsky 39 Current Challenges 5 Challenges with Creating Deepfakes 1. 2. 3. Generalization Data driven models for 𝑡 requires many HQ samples Trend: minimizing or use of few-shot learning Paired Training Data paring is required for supervised learning Trend: self supervised strategies, unpaired networks (e.g., CycleGAN), disentanglement Identity Leakage In both one-to-one and many-to-many (when training is self supervised) Trend: attention mechanisms, few-shot, feature conversion, AdaIN/skip connections to 𝐺 Dr. Yisroel Mirsky 40 Current Challenges Challenges with Creating Deepfakes 4. 5. 𝑥𝑠 𝑥𝑡 𝑥𝑔 Occlusions Dynamic obstructions (Hand, hair, inner mouth,...) cause artifacts when they appear (OOD) Trend: segmentation and inpainting on obstructed areas Temporal Coherence Many models operate per frame, resulting in artefacts and flickers Trends: input previous frame too Use temporal coherency loss Use RNNs Li Z, et al. FaceShifter: Towards High Fidelity And Occlusion Aware Face Swapping. 2020 41 Face Re-enactment Dr. Yisroel Mirsky Wu W, et al. Reenactgan: Learning to reenact faces via boundary transfer. 2018 42 Expression Re-enactment Any-to-One 𝑏: facial boundaries, 𝑏𝑥𝑔 : a boundary translated to domain 𝑥 ReenactGAN Generic Boundary Encoder 𝑥𝑠 𝐻1 𝐻2 𝐻∙𝑡 𝑏𝑠 𝑏𝑠 𝐷∙ Source Generic 𝑏𝑡′ CycleGAN 𝑏𝑠𝑔 𝑏𝑠′ 𝐻𝑡∙ 𝐷𝑡 𝑏𝑡𝑔 𝑏𝑡 Target Specific Target Specific Generator 𝐼 𝑥𝑔 𝐻𝑡 𝑥𝑡 VGG16 𝐷 𝑦 Jiangning Z, et al. FaceSwapNet: Landmark Guided Many-to-Many Face Reenactment. 2019 43 Expression Re-enactment Many-to-Many FaceSwapNet (boundary conversion) Landmark Converter 𝑥𝑠 𝑙𝑠 𝐸𝑛1 𝑙𝑡 𝐸𝑛2 Landmark Guided Generator AdaIN 𝑥𝑡′ 𝐻 𝑥𝑔 𝐷𝑒 𝑙𝑔 𝐸𝑛3 𝐷 𝐿𝐸 𝑥𝑡 Interpolate landmarks 𝑦 Caroline C, et al. Everybody dance now. 2019 44 Body Re-enactment Any-to-One Everybody Dance Now 𝑟: residual 1,2,4 (𝑖−1) 𝑙 (𝑖) 𝑡 (𝑖−1)′ 𝑥𝑡 Pose Predictor (𝑖) 𝑥𝑠 𝑃 𝑙𝑠(𝑖) 𝑝𝑜𝑠𝑒 𝑋𝑡 Normalize 𝑙𝑡 Body Generator (𝑖) 𝑙𝑔 𝐺𝐵 (𝑖) 𝑐𝑡 (𝑖)′ 𝑥𝑡 ÷ 𝐼 VGGM (𝑖)′ 𝑥𝑔 (𝑖−1)′ (𝑖−1) 𝑙𝑔 f 𝑥𝑡 (𝑖) 𝑐𝑔 Face Refiner 𝐺𝐹 𝐷1:3 𝑦1:3 1,2,4 𝑥𝑔 f ÷ 𝑟𝑔 𝑓 𝑙𝑡 𝑖 𝑓 𝑖 𝑓 𝑖 𝑓 f ∑ p 𝑥𝑔(𝑖) 𝑐𝑡 𝑖 𝑐𝑔 𝑓 𝐷𝐹 𝑦𝐹 45 Face Replacement Dr. Yisroel Mirsky Dr. Yisroel Mirsky 46 Face Replacement Face Replacement - face swap One of the most popular deepfakes Common Attacks: Revenge porn, Blackmail, Impersonation, Defamation Dr. Yisroel Mirsky 47 Face Replacement Approach 1: 1. Obtain image/video of a target scene, then 2. Inject source identity into it 𝑥𝑡 𝑠 ‘Cage’ Net 𝑥𝑔 Dr. Yisroel Mirsky 48 Face Replacement Approach 2: 1. Act out a desired scene, then 2. inject source identity into it 𝑥𝑡 𝑠 ‘Morgan’ Net 𝑥𝑔 https://github.com/iperov/DeepFaceLab 49 Face Replacement The Most Popular Design Pattern (one-to-one) Used by first Reddit deepfake, still used in tools like DeepFaceLab Dr. Yisroel Mirsky 50 Face Replacement The Most Popular Design Pattern (one-to-one) Used by first Reddit deepfake, still used in tools like DeepFaceLab Shared 𝐸𝑛 means no image pairing in training! Execute Train 𝑥𝑠 𝑥𝑡 𝑓 𝑓 𝑥𝑠 𝑥𝑡 OR 𝑓 𝑒𝑠 𝐸𝑛 𝑒 𝑡 𝐷𝑒𝑠 𝑥ො𝑠 𝑥𝑡 𝐷𝑒𝑡 𝑥ො𝑡𝑓 𝑥𝑡 𝑓 𝐸𝑛 𝑒𝑡 𝑓 𝐷𝑒𝑠 𝑥ො𝑔 p 𝑥𝑔 Dr. Yisroel Mirsky 51 Face Replacement Basic Loss 𝑓 𝑓 𝑓 𝑓 ℒ2 𝐸𝑛, 𝐷𝑒𝑠 : 𝑚𝑠 ∙ 𝑥𝑠 , 𝑚𝑠 ∙ 𝑥ො𝑠 𝑓 𝑓 𝑓 𝑓 ℒ2 𝐸𝑛, 𝐷𝑒𝑡 : 𝑚𝑡 ∙ 𝑥𝑡 , 𝑚𝑡 ∙ 𝑥ො𝑡 Current version uses more advanced losses like luminance loss and style 𝑥ො∙ 𝑓 Train 𝑥𝑠 𝑥𝑡 𝑥𝑡 OR 𝑓 𝑚∙ 𝑓 𝑓 𝑓 𝑥𝑠 ∙ 𝑒𝑠 𝐸𝑛 𝑒 𝑡 𝐷𝑒𝑠 𝑥ො𝑠 𝐷𝑒𝑡 𝑥ො𝑡𝑓 Blurred for soft edges = 𝑓 𝑥𝑔 Dr. Yisroel Mirsky 52 Face Replacement DeepFaceLab The Most Popular Face Replacement Tool Dr. Yisroel Mirsky 53 Face Replacement Training Data Making a Faceset: 1. Collect videos of 𝑠 and 𝑡 2. Extract frames 3. Use face recognition to locate faces 4. Align faces 5. Crop face (using segmentation) 6. Remove bad samples 1. Images that are not the selected identity 2. Bad alignments 3. obstructed faces 4. Noisy images Bao J, et al. Towards open-set identity preserving face synthesis. 2018 54 Face Replacement Another Approach (of many) OSIP-FS ED Feature Disentanglement 𝑦𝐼 𝐼 55 Face Synthesis https://thispersondoesnotexist.com Dr. Yisroel Mirsky Kamoun A, et al. Generative Adversarial Networks for face generation: A survey. 2022 56 Face Synthesis Overview When is face synthesis malicious? When it’s used to... Evade detectors (e.g., face reuse detec.) Lure victims Falsify Evidence Modify attributes Modify Articles (glasses, beard...) Dr. Yisroel Mirsky 57 Face Synthesis Most Common Malicious Use: Fake Profiles Attacks: Espionage Reconnaissance Scams: Romance, Vishing,... Predators Before Synthesis: scammers risked using fake images in their profiles 1. Someone may recognize the copied photo 2. Reverse image search reveals no online identity 3. After crime, source of photo may reveal attacker’s identity Now (DF): No risks, mass profile production,... Dr. Yisroel Mirsky 58 Face Synthesis Nightungale S, et al, AI-synthesized faces are indistinguishable from real faces and more trustworthy 2022 59 Face Synthesis Dr. Yisroel Mirsky 60 Face Synthesis StyleGAN (2018) Dr. Yisroel Mirsky 61 Face Synthesis Can modify codes here in execute mode StyleGAN (2018) - NVIDIA Given a distribution 𝑋 (e.g., images) StyleGAN... Automatically learns to separate high-level attributes E.g., Pose, identity,... Retains stochastic variations Old way: Latent code and content mapped mapped/ generated togther E.g., freckels, hair,... Enables “slide bar” adjustment to attributes Unsupervised training (*self supervised) Separate latent code mapping from generation We want to change style without affecting content AdaIN: Adaptive instance normalization Transfers style feature maps to content feature maps Dr. Yisroel Mirsky 62 Face Synthesis StyleGAN (2018) - NVIDIA Style Transfer: Option 1: Optimize 𝑧𝑠 with respect to a target image (as the ground truth output) Apply 𝑧𝑠 to the latent vector of the target image 𝑧𝑡 Option 2: Old way: Latent code and content mapped mapped/ generated togther Separate latent code mapping from generation We want to change style without affecting content Add a conditional input with 𝑧 (like pix2pix) AdaIN: Adaptive instance normalization Transfers style feature maps to content feature maps Analyzing and Improving the Image Quality of StyleGAN Tero Karras, Samuli Laine, Miika Aittala, Janne Hellsten, Jaakko Lehtinen, Timo Aila 63 Face Synthesis StyleGAN 2 (2019) StyleGAN 2 is used in thispersondoesnotexist.com thiscatdoesnotexist.com... https://youtu.be/0zaGYLPj4Kk 64 Face Synthesis StyleGAN 3 (2021) Dr. Yisroel Mirsky 65 Other Synthesis StyleGAN is not just for faces Cars, cities, objects, etc... Dr. Yisroel Mirsky 66 Mandatory Reading: Week 7 Pix2Pix HD: High-Resolution Image Synthesis and Semantic Manipulation with Conditional GANs https://arxiv.org/pdf/1711.11585.pdf