Lecture 5- Factorial between subjects ANOVA 2023-2024.pptx
Document Details
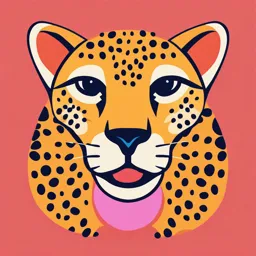
Uploaded by WillingOstrich
Full Transcript
Factorial between-subjects ANOVA (Lecture 5) 1 Factorial Analysis of Variance 2 I told you ANOVA is more flexible than ttests Compare more than two groups simultaneously In one-way ANOVA, all conditions are levels of the same independent variable (e.g. profession). What if you want to examine...
Factorial between-subjects ANOVA (Lecture 5) 1 Factorial Analysis of Variance 2 I told you ANOVA is more flexible than ttests Compare more than two groups simultaneously In one-way ANOVA, all conditions are levels of the same independent variable (e.g. profession). What if you want to examine effect of more than one independent variable (IV)? Factorial Analysis of Variance 3 Let’s look at situations where you have more than one IV (where you manipulate more than one thing). A between-subjects design with more than one IV is called a factorial design. Factorial ANOVA will have different participants in each condition. Learning objectives 4 Describe the factorial ANOVA technique. Conduct and interpret factorial ANOVA. Describe two-way interaction effects. For reporting results see ANOVA tutorial 5. Aims and objectives 5 Two-way between-subjects ANOVA (two IVs). Example of how a two-way between subjects ANOVA differs from a one-way between-subjects ANOVA. Discuss partitioning the variance for factorial ANOVA. Introduce the concept of an interaction. Reading the output and interpreting interaction graphs What is two-way betweensubjects ANOVA? 6 Depending on the number of IVs (or factors) that you manipulate the ANOVA is called: twoway, three-way, etc… What’s the name of ANOVA where four IVs have been manipulated? You can tell from the ANOVA name what the experimental design is. We don’t often see ANOVA with more than three IVs. It would be too difficult to interpret! Factorial Analysis of Variance 7 Benefit of Factorial Designs: We can look at how variables interact (how the variable work in combination). Interaction: "The effect of one independent variable may depend on the level of the other independent variable" May be more interesting than main effects (the effects of a variable in isolation). Interaction example 8 Effect of partying (sleep deprivation) and lecture type (stats vs. clinical psychology) on number of minutes sleeping in class. IV1 – sleep deprivation IV2 – lecture type DV – sleeping in class (in minutes) (two-way between subjects ANOVA) Benefit of Factorial Designs Let’s look at the effect of sleep deprivation alone (IV1), lumping the two ‘lecture types’ together. Partying the night before appears to make people sleepy. dozing (minutes) 9 Benefit of Factorial Designs Let’s look at the effect of lecture type (IV2), lumping the sleep deprivation groups together. People seem to be sleeping more in stats lectures. dozing (minutes) 10 Benefit of Factorial Designs these are called the main effects of the two IVs. based on the marginal means marginal means: the means for one IV averaged across all levels of the other IV But to understand the whole story, let’s look at the individual cell means, the means for each lecture/sleep deprivation combination... 20 12.5 dozing (minutes) dozing (minutes) 11 22.5 10 An interaction effect! 12 35 30 25 20 15 10 5 0 (minutes) dozing dosing (in minutes) stats clinical It appears partying the night before has a much higher effect on sleeping during a stats lecture! The effect on sleep in the clinical lecture was much smaller. This is an example of an interaction. Defining an Interaction 13 The effect of one independent variable may depend on the level of the other independent variable. An independent variable has a different effect on the dependent variable depending on the values of another independent variable. The effect of the two variables is “not additive”. Without an interaction we literally add the effects of the two IVs on the DV. But most things in the real world do not behave additively IVs may interfere, cooperate, compete, etc. If we listen to two of our favourite songs at the same time, we don’t get double the pleasure… An “It depends!” effect. Main effects in the presence of a significant interaction. Interpret with caution! The presence of an interaction may affect the generality of the main effects (even if they are significant). 35 30 25 20 15 10 5 0 (minutes) dozing dosing (in minutes) 14 stats clinical Numerical example 15 Psychologist tests how gender (boy vs. girl) & schooling (mixed vs. single sex) affects maths problem solving. She tested the problemssolving ability of boys and girls in mixed vs. single sex classrooms (a total of 4 child gender x classroom type combinations). Numerical example 16 Two-way between-subjects ANOVA IV1: child gender (two levels – boys vs girls) IV2: school type (two levels – mixed vs. single sex) DV: number of problems solved. Numerical example 17 Does performance differ between boys and girls (main effect of gender)? Does performance differ between single-sex and mixed schools (main effect of classroom type)? Does the effect of gender depend on which school type was tested (a gender x classroom type interaction)? Maths performance example: dataset 1 18 Boys IV1: Gender IV2: School Subject 1 Subject 2 Subject 3 Subject 4 Subject 5 Subject 6 Subject 7 Subject 8 Subject 9 Subject 10 Girls Mixed Single Mixed Single 12 17 14 13 15 17 15 13 18 16 15 12 13 14 17 16 13 18 15 17 15 30 17 16 18 20 18 16 21 19 19 21 16 18 20 18 16 17 20 15 The sources of variance in the data 19 Total variability (between all scores and grand mean) is split into: Variability between the group ‘averages’, i.e., variability explained by our independent variables (IV): (Sums of SquaresModel) Error variability not explained by our IVs (Sums of SquaresResidual). Partitioning the total variance in factorial ANOVA 20 (SS total) As usual JASP will do the grunt work for you… (SS gender) (SS classroom) (SS interaction) Partitioning the total variance in factorial ANOVA 21 Let’s start with the total variance: Total variance (SSTotal)= variability of each child’s score around the grand mean (ignoring group membership). Grand mean = 16.75 Partitioning the total variance in factorial ANOVA 22 SSbetween treatment = variability of the cell means around the grand mean.. (15 – 16.75)2, then (19-16.75)2 , etc. Break between-treatment variability further: SS gender : variability of marginal means for gender around grand mean. SS class: variability of marginal means for gender around grand mean. Ss interaction: what’s left of the betweentreatment variance. SS interaction = SSbetween treatment – SSgender – SSclass Error (residual) variance: whatever is left of the total variance SS error = SSTotal – SSbetween treatments Notice how adding extra factors and the interaction term shrinks the SSerror 23 (1) analysing only ‘gend (2) let’s add ‘class’ (3) let’s add the interact (full class x gender desig Notice how adding extra factors and the interaction term increases the sensitivity of our design (it shrinks the error term!) error error error 24 Checking for homogeneity of variance… 25 If p>.05 , we assume the groups have similar variances and you can Two-way ANOVA summary table 26 Same example, different data 27 Boys Mixe Singl d e 12 15 17 20 14 17 13 16 15 18 17 20 15 18 13 16 18 21 16 19 Girls Mixed Single 19 21 16 18 20 18 16 17 20 15 15 12 13 14 17 16 13 18 15 17 ANOVA summary table 28 29 An interaction, but no main effects More problems solved by boys in single sex than mixed schools, and more problems were solved by girls in mixed than single sex schools Recognizing main effects and interactions graphically 30 With two IVs we could have: Main effect of IV1, and nothing else (below parallel horizontal lines indicate an effect of gender). Main effects of IV1 and IV2, but no interaction (lines are parallel but not horizontal. Interaction, but no main effects (the lines form a cross) You can have other combinations as well, e.g., only one main effect and an interaction, etc. Types of interactions 31 Ordinal interactions nonparallel lines slope in the same direction but do not cross treatment has a more intense effect in one condition than another May be spurious if data is “ordinal” rather Example: Effect than interval floor or ceiling effects of exercise and caffeine on calories consumed… 32 Ordinal interactions assume interval data… We assume these two increases are numerically comparable… This is not necessarily the case with ordinal data Ranked data where distances between data points are unknown. E.g., we could rank people by their level of hunger but difference between them may not be comparable. E. g., Person A hungrier than Person B hungrier than Person C, but is A hungrier than B by as Spurious ordinal interaction due to ceiling effect 33 Spurious ordinal interaction due to floor effect 34 Cross-over interactions 35 E.g., when a factor has one effect in one condition and the opposite kind of effect in another condition not vulnerable to ordinal data issues… Effect of exercise and caffeine on calories consumed… Posthocs in factorial ANOVA 36 To interpret the main effects as with 1-way ANOVA (but only when the IV has more than two levels). Interaction posthocs aid interpretation of a significant interaction. Comparing cell means across factors. E.g., does caffeine have a significant effect in the exercise condition? ? What next? 37 What if the same participants are tested across multiple levels of two IVs. This would be a two-way repeated measures design What if the same participants are tested across multiple levels of one IV but contribute to only one level of another IV (e.g. gender) mixed design Readings 38