Multivariate Regression Analysis Lecture 5 PDF
Document Details
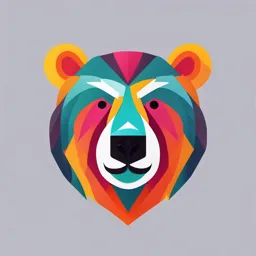
Uploaded by MasterfulNeptunium
Imam Abdulrahman Bin Faisal University
Tags
Summary
This lecture outlines multivariate regression analysis, a statistical technique for understanding relationships between multiple independent variables and a single dependent variable. It covers key components, applications in healthcare and business, and the need for this type of analysis. The lecture also explains how to build and interpret multivariate regression models, along with potential limitations and challenges in the analysis.
Full Transcript
I. Multivariate Regression Analysis: Definition: A statistical technique used to understand the relationship between multiple independent variables and a single dependent variable. It assesses how several factors collectively influence a specific outcome over time, making it particularly...
I. Multivariate Regression Analysis: Definition: A statistical technique used to understand the relationship between multiple independent variables and a single dependent variable. It assesses how several factors collectively influence a specific outcome over time, making it particularly useful for time series data. Key Components: One dependent variable, two or more independent variables (potentially correlated), coefficients indicating each independent variable's contribution to the dependent variable's variation, and an error term. The analysis accounts for potential time dependencies and autocorrelations. Applications: o Healthcare: Analyzing factors (age, treatment type, comorbidities) influencing patient outcomes, predicting disease progression, and supporting personalized medicine. o Business and Economics: Analyzing consumer behavior, understanding marketing strategy impact on sales, forecasting economic indicators (interest rates, employment levels), and making data-driven decisions. o Time Series Data: Forecasting future values based on historical data while considering multiple predictors. This involves incorporating lagged values of dependent and independent variables to capture temporal effects. Common in economics, finance, and environmental science. Need for Multivariate Regression: o Modeling complex relationships o Controlling for confounding variables o Improving predictive power o Comparing effects of different variables o Choosing the best model II. Building and Interpreting a Multivariate Regression Model: Building Process: Data preparation, selecting variables, model building, model evaluation, and model interpretation. Key Components of the Model: Dependent variable, independent variables, coefficients, and error term. Model Performance Metrics: R-squared, adjusted R-squared, and p-values for coefficients (significance). III. Limitations and Challenges: Assumptions: Linearity, independence of observations, homoscedasticity (constant variance of errors) must be met for valid results. Multicollinearity: Highly correlated independent variables distort results and complicate interpretation. It inflates the variance of coefficient estimates, leading to unreliable inferences. It's crucial to check correlations before modeling. Remedies include removing highly correlated predictors or combining them using techniques like principal component analysis. Autocorrelation: Correlation between residuals across time, leading to inefficient estimates and biased tests if not addressed. IV. EViews Application: Data Preparation: Ensuring time series data is stationary (constant mean and variance) using tools like differencing and logging. Conducting Regression: Using the 'Quick' menu and 'Estimate Equation' to define dependent and independent variables, then obtaining coefficient estimates, R-squared, and significance levels. Interpreting Output: Understanding coefficients, p-values, and diagnostic tests like the Durbin-Watson statistic (autocorrelation). Identifying Multicollinearity: Using diagnostic tools like the Variance Inflation Factor (VIF) and correlation matrix. A VIF > 10 often indicates a problem. Residual Normality (Jarque-Bera Test): Assessing normality of residuals; a significant result (p-value < 0.05) suggests deviation from normality, impacting reliability. EViews provides this test. Serial Correlation (Breusch-Godfrey LM Test): Detecting serial correlation; a significant result (p-value < 0.05) indicates the presence of serial correlation. EViews provides this test. Model Specification (Ramsey RESET Test): Checking for specification errors; a significant result (p-value < 0.05) indicates potential omitted variables or incorrect functional form. EViews provides this test.