Lecture 4: Estimating Population Mean - Confidence Intervals PDF
Document Details
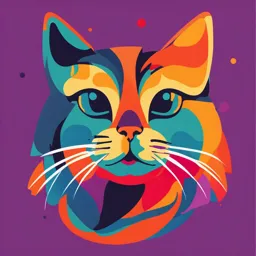
Uploaded by DeftSheep7841
Stony Brook University
Tags
Summary
This document details a lecture on estimating a population mean using confidence intervals in statistics. It introduces point estimates and discusses the concepts of error and confidence levels in estimating an unknown population parameter. The document utilizes examples to illustrate the calculations and interpretation of confidence intervals. The examples highlight different levels of confidence and how different confidence intervals affect the range of values.
Full Transcript
# Lecture 4: Estimating the Population Mean µ using Confidence Intervals (A,B) ## Introduction - We have a population of size N. - Population mean u is unknown. - We want to estimate u using the sample mean Xn obtained from a random sample. ## Definitions **Point Estimate:** An estimate of a po...
# Lecture 4: Estimating the Population Mean µ using Confidence Intervals (A,B) ## Introduction - We have a population of size N. - Population mean u is unknown. - We want to estimate u using the sample mean Xn obtained from a random sample. ## Definitions **Point Estimate:** An estimate of a population parameter given by a single number **Examples:** - Xn (sample mean) is a point estimate for u (population mean). - s (sample standard deviation) is a point estimate for σ (population standard deviation). ## Point Estimate Errors - There is some error when we estimate µ by Xn. - This error is represented by: X- µ = Std error = s / √n - n is the size of the sample - s is the standard deviation of the population. ## Confidence Intervals - A confidence interval (CI) for µ is an entire interval of plausible values for µ. - It's defined by a confidence level, e.g., 95%, 99%, etc. - Using Xn (our point estimate) as the center, we determine an interval of values for μ, (A,B), at the specified confidence level. ## Desirable Characteristics of Confidence Intervals - **Small confidence intervals:** we want to hone-in on the unknown mean μ. - **Large confidence levels:** we want to be more confident that the true population mean lies within the interval. ## Tradeoff between Confidence Level and Interval Size - As we try to increase the confidence level, the confidence interval gets wider. ## Case A: σ is known We want to create an interval estimate for the parameter µ when σ is known. ### Assumptions 1. **Normality:** The underlying population X is normally distributed, or large samples (n≥30) are taken. This ensures X is approximately normal due to the Central Limit Theorem. 2. **σ Known:** The population standard deviation σ is known. This is usually unrealistic as we are trying to estimate the population mean. 3. **Simple Random Sample:** The sample is a simple random sample. 4. **Independence:** The data values are independent. ### Formula for Confidence Interval The symmetric (1-α) CI for u is given by: Xn ± Za/2 * σ / √n Where: - Xn is the sample mean - Za/2 is the Z-score with area of α/2 to the right of it, under the Z curve. - σ is the population standard deviation - n is the sample size ### Example Let's consider an example with N = 250 students in a class. We are interested in the average weight of the students. - We take a random sample of n = 40 students. - The known population standard deviation σ = 6. - The sample mean X40 = 165 lbs. - We want to find a 90% confidence interval to estimate the population mean µ. #### Steps 1. **Confidence Level & Za/2:** We want a 90% confidence interval, so α = 0.1 and Za/2 = 1.645 (from the Z-table). 2. **Calculate Margin of Error:** E = Za/2 * σ / √n = 1.645 * 6 / √40 = 1.56 3. **Confidence Interval:** (Xn - E, Xn + E) = (165 - 1.56, 165 + 1.56) = (163.44, 166.56) #### Interpretation We are 90% confident that the true population mean weight lies in the interval (163.44, 166.56). ## Importance of the Interpretation - The 95% CI is presented as "We are 95% confident that the true parameter u lies in this interval. - This means that in repeated sampling, 95% of the intervals computed would include the population parameter µ. ## Example Continued Let's continue with the example from above and consider: 1. **60% confidence interval** 2. **98% confidence interval** 3. **Margin of sampling error for the 98% confidence interval** 4. **Length of the 98% confidence interval** The steps are similar to the steps for the 90% confidence interval but using different values for Za/2 and the confidence level. ## Conclusion - By understanding confidence intervals, we can obtain a range of plausible values for the unknown population mean. - This information is crucial for making informed decisions and drawing reliable conclusions in a variety of fields.