Econometrics Lecture 1A PDF
Document Details
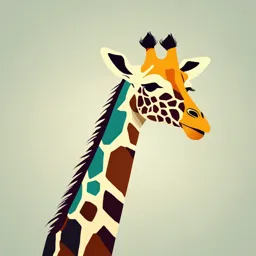
Uploaded by Hazim.Zamri
UNSW
Hasin Yousaf
Tags
Summary
This document presents a lecture on econometrics, covering topics like different types of economic data, random variables, and samples. It includes examples, tables, and references to Wooldridge chapters.
Full Transcript
ECONOMETRICS 1 LECTURE 1A Hasin Yousaf WEEK #1 TOPICS Econometrics What is econometrics? Types of economic data Random Variables and Samples Key references Course outline Wooldridge Chapters 1-2.1 2 ECONOMETRI...
ECONOMETRICS 1 LECTURE 1A Hasin Yousaf WEEK #1 TOPICS Econometrics What is econometrics? Types of economic data Random Variables and Samples Key references Course outline Wooldridge Chapters 1-2.1 2 ECONOMETRICS What is econometrics? Econometrics = use of statistical methods to analyze economic data (...but recently used for other type of data) Econometricians typically analyze: Experimental data (as in other sciences) Non-experimental data (predominantly) Typical goals of econometric analysis Estimating relationships between economic variables Testing economic theories & hypotheses Forecasting economic variables Evaluating & implementing government & business policy 3 GOALS OF AN ECONOMETRIC MODEL Example: We have data for different countries and years of the unemployment rate and inflation The goals we could have are: Estimating the relationship: When unemployment is high is inflation also high? Testing an economic theory: High inflation causes unemployment to go up Forecasting: How high inflation will be high next year? Evaluation: If the Australian government increases taxes what happened to unemployment Different econometric model are appropriate for different goals 4 ECONOMIC DATA Different kinds of economic data Cross-sectional Time series Pooled cross sections Panel/Longitudinal data Econometric methods depend on nature of data used Use of inappropriate methods may lead to misleading results 5 ECONOMIC DATA: CROSS-SECTIONAL Cross-sectional data sets Sample of individuals, households, firms, states, countries, or other units of interest at given point of time/in given period Observations typically more or less independent E.g. pure random sampling from a population Sometimes pure random sampling is violated leading to dependencies Units refuse to respond in surveys Sampling is characterized by clustering (e.g. household) 6 ECONOMIC DATA: CROSS-SECTIONAL... Cross-sectional data set on wages & other characteristics Indicator variables (1 = yes, 0 = no) 7 Observation number Hourly wage Years of Years of education experience ECONOMIC DATA: TIME SERIES Time series data sets Observations for an individual, firm, country,... collected over time E.g. stock prices, exchange rates, consumer price index, gross domestic product, annual homicide rates, automobile sales, … Observations are typically serially correlated Ordering of observations conveys important information Understanding the path of dependency over time is usually the main object of interest Typical features of time series data Trends, cycles & seasonality Data frequency (daily, weekly, monthly, quarterly, annually, …) important for features Typically in applied macroeconomics, finance,... 8 ECONOMIC DATA: TIME SERIES... Time series data on minimum wages & related variables Average minimum Average Unemployment Gross national wage for the given year coverage rate rate product 9 ECONOMIC DATA: POOLED CROSS SECTIONS Pooled cross sections Two or more cross sections are combined in one data set Cross sections are drawn independently of each other Often used to evaluate policy changes Evaluate effect of change in property taxes on house prices Random sample of house prices for year 1993 New random sample of house prices for year 1995 Compare before reform (1993) to after reform (1995) 10 ECONOMIC DATA: POOLED CROSS SECTIONS... Pooled cross sections on housing prices Property tax Size of house in square feet Number of bedrooms Number of bathrooms Before reform After reform 11 ECONOMIC DATA: PANEL Panel or longitudinal data Same cross-sectional units followed over time Panel data have cross-sectional & time series dimension Provide considerable opportunities in modelling Can account for time-invariant unobservables Can model lagged responses (as can time series data) Example: City crime statistics: each city is observed in two years Can control for time-invariant unobserved city characteristics Effect of police on crime rates may exhibit time lag 12 ECONOMIC DATA: PANEL... Two-year panel data on city crime statistics Each city has two time series observations Number of police in 1986 Number of police in 1990 13 DIFFERENCE BETWEEN RANDOM VARIABLE AND SAMPLE Some boring but useful definitions Example Coin Toss: 𝑋𝑋 represent the possible events before tossing the coin 𝐻𝐻𝐻𝐻𝐻𝐻𝐻𝐻𝐻𝐻 𝑤𝑤𝑤𝑤𝑤𝑤𝑤 𝑝𝑝𝑝𝑝𝑝𝑝𝑝𝑝𝑝𝑝𝑝𝑝𝑝𝑝𝑝𝑝𝑝𝑝𝑝𝑝𝑝𝑝 0.5 𝑋𝑋 = 𝑇𝑇𝑇𝑇𝑇𝑇𝑇𝑇𝑇𝑇 𝑤𝑤𝑤𝑤𝑤𝑤𝑤 𝑝𝑝𝑝𝑝𝑝𝑝𝑝𝑝𝑝𝑝𝑝𝑝𝑝𝑝𝑝𝑝𝑝𝑝𝑝𝑝𝑝𝑝 0.5 X is a random variable 14 DIFFERENCE BETWEEN RANDOM VARIABLE AND SAMPLE What is a random variable? Function that describes the possible outcomes and probabilities of a random event. Distribution of possible values of a variable I will try always to write it capital letters (X, W, COIN) 15 DIFFERENCE BETWEEN RANDOM VARIABLE AND SAMPLE Imagine now that we toss the coin N times. The observations are called sample Write it as {𝑤𝑤1 , 𝑤𝑤2 , … , 𝑤𝑤𝑁𝑁 } This is just a recording of the observation of tossing the coin 𝑁𝑁 times I will try always to write it lower case letters and with a subscript indicating which observation (𝑤𝑤1 , 𝑥𝑥𝑖𝑖 , 𝑐𝑐𝑐𝑐𝑐𝑐𝑐𝑐𝑗𝑗 …) 16 DIFFERENCE BETWEEN RANDOM VARIABLE AND SAMPLE Any observable phenomenon can be described as a random variable In this course we will be particularly interested in economic quantities ex: wages of Australian full-time workers Random Variable (Wage): describe the population distribution of full-time workers in Australia Sample 𝑤𝑤𝑎𝑎𝑎𝑎𝑎𝑎1 , 𝑤𝑤2 , … , 𝑤𝑤𝑎𝑎𝑎𝑎𝑎𝑎𝑁𝑁 : Thee list of our N observations of wages 17 FINAL THOUGHTS Need to know: Difference between different type of data Random Variable Vs. Sample Please check that you have access to STATA trough MyAccess 18