Lecture 11 - Estimating Abundance in Animal Populations PDF
Document Details
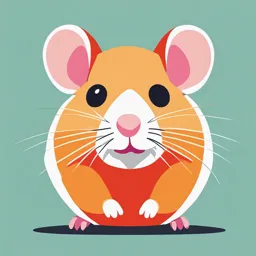
Uploaded by ProficientSetting
2024
Prof S W Makhabu
Tags
Related
- Lecture 17 Study Guide - Ecology PDF
- Using Experimentation to Understand the 10-Year Snowshoe Hare Cycle (2018) PDF
- Using Experimentation to Understand the 10-Year Snowshoe Hare Cycle (PDF)
- Animal Surveying Techniques PDF - SC/BIOL/ENVB 2080
- Conserving Populations & Species PDF
- Animal Ecology (Bartolomeus) PDF
Summary
This document is a lecture on estimating abundance in animal populations. It discusses different methods, including absolute and relative density estimations as well as mark-and-recapture techniques (Petersen and Schnabel methods).
Full Transcript
BDB 211 POPULATION AND COMMUNITY ECOLOGY Lecture 11 Estimating abundance in Animal Populations 23 September 2024 Prof S W Makhabu Learning Objective To learn and master the methods used in estimating abundance in animal populat...
BDB 211 POPULATION AND COMMUNITY ECOLOGY Lecture 11 Estimating abundance in Animal Populations 23 September 2024 Prof S W Makhabu Learning Objective To learn and master the methods used in estimating abundance in animal populations INTRODUCTION The central question of many ecologists when they see a population is “How many individuals are there? If there is a wish to harvest some of them, one necessary bit of information is the size of the population If you are deciding to spray a pesticide of aphids on a crop, you need to know their population If you wish to measure the impact of lion predation on a zebra population, you need to know the size of both the lion and zebra populations The cornerstone of many ecological studies is an estimate of the abundance of a particular population This is true for both population ecology, in which interest centres on one species, and community ecology, in which interest centres on many species and, thus, the estimation problem is more complex You need to decide whether estimation of population is necessary Deciding whether estimation of population is necessary INTRODUCTION Abundance can be measured in two ways: a. Absolute density b. Relative density Absolute density is the number of organisms per unit area or volume e.g. A red deer density of four deer per square kilometre is an absolute density Relative density is the density of one population to that of another population e.g. blue grouse may be more common in a block of recently burned woodland than in a block of mature woodland Relative density may be adequate for many ecological problems, and it should always be used when adequate because it is much easier and cheaper to determine than absolute density Absolute density must be obtained for any detailed population study in which one attempts to relate population density to reproductive rate or any other vital statistic. The analysis of harvesting strategies also demands information on absolute numbers. All community studies which estimate energy flow or nutrient cycles also require reliable estimates of absolute density Estimating Abundance Techniques Mark-and- recapture Techniques One way to estimate the size of a population is to capture and mark individuals from the population, release them, and then resample to see what fraction of individuals carry marks John Graunt first used this simple principle to estimate the human population of London in 1662 The first ecological use of mark-and-recapture was carried out by Danish fisheries biologist C. G. J. Petersen in 1896 (Ricker, 1975) Tagging of fish was first used to study movements and migration of individuals, but Petersen realized that tagging could also be used to estimate population size and to measure mortality rates Fisheries biologists were well advanced over others in applying these methods Lincoln (1930) used mark-recapture to estimate the abundance of ducks from band returns, and Jackson (1933) was the first entomologist to apply mark-recapture methods to insect populations The strength of mark-and-recapture techniques is that they can be used to provide information on birth, death, and movement rates in addition to information on absolute abundance The weakness of these techniques is that they require considerable time and effort to get the required data and to be accurate, they require a set of very restrictive assumptions about the properties of the population being studied Estimating Abundance Techniques Mark-and- recapture Techniques Mark-and-recapture techniques may be used for open or closed populations A closed population is one that does not change in size during the study period, that is, one in which the effects of births, deaths, and movements are negligible An open population is the more usual case, a population that changes in size and composition from births, deaths, and movements Different methods must be applied to open and closed populations: i. Closed populations, single, marking – Petersen method ii. Closed populations, multiple markings – Schnabel method iii. Open populations, multiple census – Jolly-Seber method Petersen Method The Petersen method is the simplest mark-and-recapture method because it is based on a single episode of marking animals, and a second single episode of recapturing individuals. The basic procedure is to mark a number of individuals over a short time, release them, and then to recapture individuals to check for marks. All individuals can be marked in the same way. The second sample must be a random sample for this method to be valid; that is, all individuals must have an equal chance of being captured in the second sample, regardless of whether they are marked or not. The data obtained are M = Number of individuals marked in the first sample C = Total number of individuals captured in the second sample R = Number of individuals in second sample that are marked. From these three variables, we need to obtain an estimate of Ň = Size of population at time of marking By a proportionality argument, we obtain: Petersen Method Nˆ = C M R Petersen Method Confidence Intervals for the Petersen Method How reliable are these estimates of population size? To answer this critical question, a statistician constructs confidence intervals around the estimates. A confidence interval is a range of values which is expected to include the true population size a given percentage of the time. Typically the given percentage is 95% but you can construct 90% or 99% confidence intervals, or any range you wish. The high and low values of a confidence interval are called the confidence limits. Clearly, one wants confidence intervals to be as small as possible, and the statistician's job is to recommend confidence intervals of minimal size consistent with the assumptions of the data at hand. Confidence Intervals for the Petersen Method Confidence intervals are akin to gambling. You can state that the chances of flipping a coin and getting "heads" is 50%, but after the coin is flipped, it is either "heads" or "tails". Similarly, after you have estimated population size by the Petersen method and calculated the confidence interval, the true population size (unfortunately not known to you!) will either be inside your confidence interval or outside it. You cannot know which, and all the statistician can do is tell you that on the average 95% of confidence intervals will cover the true population size. Alas, you only have one estimate, and on the average does not tell you whether your one confidence interval is lucky or unlucky. Confidence intervals are an important guide to the precision of your estimates. If a Petersen population estimate has a very wide confidence interval, you should not place too much faith in it. If you wish you can take a larger sample next time and narrow the confidence limits. But remember that even when the confidence interval is narrow, the true population size may sometimes be outside the interval. Several techniques of obtaining confidence intervals for Petersen estimates of population size are available, and the particular one to use for any specific set of data depends upon the size of the population in relation to the samples we have taken. Seber (1982) gives the following general guide: 1. If the fraction of marked animals (R/C) is less than 0.10, and a. The number of recaptures (R) is less than 50, use Poisson confidence intervals. b. The number of recaptures (R) is above 50, use the normal approximation to obtain confidence intervals. 2. If the fraction of marked animals (R/C) is more than 0.10, use binomial confidence intervals. Confidence Intervals for the Petersen Method Assumptions of Petersen Method For Nˆ in formula (2.2) or (2.3) to be an accurate estimate of population size the following five assumptions must hold: 1. The population is closed, so that N is constant. 2. All animals have the same chance of getting caught in the first sample. 3. Marking individuals does not affect their catchability. 4. Animals do not lose marks between the two sampling periods. 5. All marks are reported upon discovery in the second sample. If the first assumption is to hold in a biological population, it is essential that the Petersen method be applied over a short period of time. One of the crucial assumptions of mark-recapture work is that marked and unmarked animals are equally catchable. Random sampling is critical if we are to obtain accurate Petersen estimates, but it is difficult to achieve in field programs. SCHNABEL METHOD Schnabel (1938) extended the Petersen method to a series of samples in which there is a 2nd, 3rd, 4th...nth sample. Individuals caught at each sample are first examined for marks, then marked and released. Marking occurs in each of the sampling times. Only a single type of mark need be used, since throughout a Schnabel experiment we need to distinguish only two types of individuals: marked = caught in one or more prior samples; and unmarked = never caught before. We determine for each sample t: Ct = total number of individuals caught in sample t Rt = number of individuals already marked when caught in sample t Ut = number of individuals marked for first time and released in sample t Normally Ct = Rt + Ut but if there are accidental deaths involving either marked or unmarked animals, these are subtracted from the Ut value*. SCHNABEL METHOD SCHNABEL METHOD The number of marked individuals in the population continues to accumulate as we add further samples, and we define: M = number of marked individuals in the population t just before the t − th sample is taken t −1 Mt = Ut i =1 so that, for example, M6 = U1 + U2 + U3 + U4 + U5 Table 2.2 gives an example of some Schnabel-type data obtained on a sunfish population. Given these counts of marked and unmarked individuals, we need to derive an estimate of population size N for a closed population. Ricker (1975) gives several methods, and Seber (1982, Chap. 4) discusses this estimation problem in detail. We will describe here two methods of estimation, the original Schnabel method and the Schumacher and Eschmeyer method, which is the most robust and useful ecological model according to Seber (1982). Assumptions of the Schnabel Method The Schnabel method makes all the same assumptions that the Petersen method makes - that the population size is constant without recruitment or losses, that sampling is random, and all individuals have an equal chance of capture in any given sample. Our previous discussion of the limitations these assumptions impose are thus all relevant here. The major advantage of the multiple sampling in a Schnabel experiment is that it is easier to pick up violations of these assumptions. What methods are available for counting wildlife? There are three main methods for counting wildlife. These are ! total counts, ! sample counts ! index counts. The choice of how to do the count, whether on foot, from a vehicle or from an aircraft, will depend on the species to be counted, the size and relief of the area, the resources available and the objective of the count.