Lecture 1 - Introduction PDF
Document Details
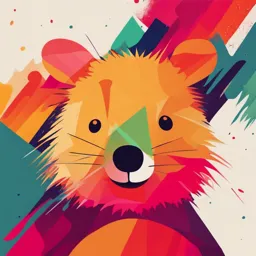
Uploaded by PopularForethought7405
UVA
Tags
Summary
This is an introduction course on deep learning, focusing on the historical development of the field.
Full Transcript
Frank Rosenblatt Charles W. Wightman A brief history of deep learning DEEP LEARNING ONE – 18 19 Pe...
Frank Rosenblatt Charles W. Wightman A brief history of deep learning DEEP LEARNING ONE – 18 19 Pe 58 r ce pt ro 19 ns 60 ,R Ad os a en lin bl e, at W t 19 id 69 ro Pe w r ce an pt d ro H 19 ns of 70 Ba ,M f Li ckp in n n ro sk ain pa y an 19 m ga aa tio d 74 Pa Ba n, pe ck rt pr UVA DEEP LEARNING COURSE – EFSTRATIOS GAVVES op 19 ag 86 at Ba io c EFSTRATIOS n, H kp in W EFSTRATIOS to r o p er First appearance (roughly) n a bo 19 an ga s GAVVES 97 LS d ti o W n GAVVES DEEP Sc TM hm , H illi , Ru – UVA id o c am m UVA hu h s el h 19 r DEEP 98 ar –LEARNING DEEP OC ber eite r t, H R, an af L d ONE f n eC 20 er LEARNING un 06 ,B LEARNING De Te ep ot h Le to u, - 19 COURSE ar Be COURSE 20 ni ng 09 ng io – ‹#›– 19 Im ,H an ag in to d en n, et Os 20 ,D 12 en in Al e g de ro an xn et , d et al. H ,L 20 af e 15 fn Cu Re er n sn ,B et ot (1 to to 54 u, Be y da lay ng DEEPER GO er io VISLab ,D s), M VISLab ee SR pm A in LEARNING AND OPTIMIZATIONS - 19 INTO DEEP d Rosenblatt: The Design of an Intelligent Automaton (1958) “a machine which senses, recognizes, remembers, and responds like a human mind” You think your wiring is chaotic? UVA DEEP LEARNING COURSE – EFSTRATIOS GAVVESEFSTRATIOS EFSTRATIOS GAVVES DEEP GAVVES –LEARNING – UVAUVA DEEP DEEP ONE LEARNING - 20 COURSE LEARNING COURSE – ‹#›– 20 DEEPER INTO DEEP VISLab VISLab LEARNING AND OPTIMIZATIONS - 20 Perceptrons o (McCulloch & Pitts: binary inputs & outputs, no weights/learning) o Rosenblatt proposed perceptrons for binary classifications o A model comprising one weight 𝑤! per input continuous 𝑥! o Multiply weights with respective inputs and add bias (b = w", 𝑥" = +1) & & 𝑦 = , 𝑤# 𝑥# + 𝑏 = , 𝑤# 𝑥# #$% #$" o If score 𝑦 positive then return 1, otherwise -1 UVA DEEP LEARNING COURSE – EFSTRATIOS GAVVESEFSTRATIOS EFSTRATIOS GAVVES DEEP GAVVES –LEARNING – UVAUVA DEEP DEEP ONE LEARNING - 21 COURSE LEARNING COURSE – ‹#›– 21 DEEPER INTO DEEP VISLab VISLab LEARNING AND OPTIMIZATIONS - 21 Training a perceptron o Main innovation: a learning algorithm for perceptrons Perceptron learning algorithm Comments 1. Set 𝑤! ← random 2. Sample new (𝑥" , 𝑙" ) New train image, label 3. Compute 𝑦" = ∑𝑤" 𝑥"! > 0 ⋅ : indicator function 4. If 𝑦" < 0, 𝑙" > 0 → 𝑤" = 𝑤" + η ⋅ 𝑥" Score too low. Increase weights! 5. If 𝑦" > 0, 𝑙" < 0 → 𝑤" = 𝑤" − η ⋅ 𝑥" Score too high. Decrease weights! 6. Go to 2 Repeat till happy J UVA DEEP LEARNING COURSE – EFSTRATIOS GAVVESEFSTRATIOS EFSTRATIOS GAVVES DEEP GAVVES –LEARNING – UVAUVA DEEP DEEP ONE LEARNING - 22 COURSE LEARNING COURSE – ‹#›– 22 DEEPER INTO DEEP VISLab VISLab LEARNING AND OPTIMIZATIONS - 22 From a single output to many outputs o Perceptron was originally proposed for binary decisions o What about multiple decisions, e.g. digit classification? o Append as many outputs as categories → Neural network 4-way neural network UVA DEEP LEARNING COURSE – EFSTRATIOS GAVVESEFSTRATIOS EFSTRATIOS GAVVES DEEP GAVVES –LEARNING – UVAUVA DEEP DEEP ONE LEARNING - 23 COURSE LEARNING COURSE – ‹#›– 23 DEEPER INTO DEEP VISLab VISLab LEARNING AND OPTIMIZATIONS - 23 From a single output to many outputs o Quiz: o How many weights w do we need if we have an image of size 200x200 pixels, with 3 colors (red, blue, green) as input and output 500 categories? o 1) 6K: ~ 1/10th of Gouda o 2) 60K: ~ Johan Cruijff Arena (biggest stadium in NL) o 3) 60M: ~ population of UK o 4) 60B: ~ 7.7x Earth’s population UVA DEEP LEARNING COURSE – EFSTRATIOS GAVVESEFSTRATIOS EFSTRATIOS GAVVES DEEP GAVVES –LEARNING – UVAUVA DEEP DEEP ONE LEARNING - 24 COURSE LEARNING COURSE – ‹#›– 24 DEEPER INTO DEEP VISLab VISLab LEARNING AND OPTIMIZATIONS - 24 XOR & 1-layer perceptrons o The original perceptron has trouble with simple non-linear tasks though o E.g., imagine a NN with two inputs that imitates the “exclusive-or” (XOR) ◦ 𝜏 is the threshold for either +1 or -1 prediction Input 1 Input 2 XOR 1 1 -1 1 ⋅ 𝑤# +1 ⋅ 𝑤$ < 𝜏 → 𝑤# + 𝑤$ < 𝜏 𝑤# + 𝑤$ > 2𝜏 1 0 +1 1 ⋅ 𝑤# +0 ⋅ 𝑤$ > 𝜏 → 𝑤# > 𝜏 Inconsistent 𝑤# + 𝑤$ < 𝜏 0 1 +1 0 ⋅ 𝑤# + 1 ⋅ 𝑤$ > 𝜏 → 𝑤$ > 𝜏 0 ⋅ 𝑤# + 0 ⋅ 𝑤$ < 𝜏 → 0 < 𝜏 0 0 -1 Output 𝑤! 𝑤" No line can separate the white from the black Input 1 Input 2 Minsky and Papert, “Perceptrons”, 1969 UVA DEEP LEARNING COURSE – EFSTRATIOS GAVVESEFSTRATIOS EFSTRATIOS GAVVES DEEP GAVVES –LEARNING – UVAUVA DEEP DEEP ONE LEARNING - 25 COURSE LEARNING COURSE – ‹#›– 25 DEEPER INTO DEEP VISLab VISLab LEARNING AND OPTIMIZATIONS - 25 Multi-layer perceptrons to the rescue o Minsky never said XOR cannot be solved by neural networks ◦ Only that XOR cannot be solved with 1-layer perceptrons o Multi-layer perceptrons (MLP) can solve XOR 𝑥# ◦ One layer’s output is input to the next layer 𝑎# ◦ Add nonlinearities between layers, e.g., sigmoids 𝑥$ ◦ Or even single layer with “feature engineering” 𝑎$ 𝑦# o Problem: how to train a multi-layer perceptron? 𝑥% o Rosenblatt’s algorithm not applicable. Why? 𝑎% 𝑥& UVA DEEP LEARNING COURSE – EFSTRATIOS GAVVESEFSTRATIOS EFSTRATIOS GAVVES DEEP GAVVES –LEARNING – UVAUVA DEEP DEEP ONE LEARNING - 26 COURSE LEARNING COURSE – ‹#›– 26 DEEPER INTO DEEP VISLab VISLab LEARNING AND OPTIMIZATIONS - 26 Multi-layer perceptrons to the rescue o Rosenblatt’s algorithm not applicable. Why? ◦ Learning depends on “ground truth” 𝑙! for updating weights ◦ For the intermediate neurons 𝑎# there is no “ground truth” 𝑥# ◦ The Rosenblatt algorithm cannot train intermediate layers 𝑎# 𝑥$ 𝑎$ 𝑦# 𝑥% 𝑎% 𝑥& UVA DEEP LEARNING COURSE – EFSTRATIOS GAVVESEFSTRATIOS EFSTRATIOS GAVVES DEEP GAVVES –LEARNING – UVAUVA DEEP DEEP ONE LEARNING - 27 COURSE LEARNING COURSE – ‹#›– 27 DEEPER INTO DEEP VISLab VISLab LEARNING AND OPTIMIZATIONS - 27 19 Pe 58 r ce pt ro 19 ns 60 ,R Ad os a en lin bl e, at W t 19 id 69 ro Pe w r ce an pt d ro H 19 ns of 70 ,M f Ba in ck sk pr op y an 19 ag d at Pa 74 Ba io n, p er ck pr Li t UVA DEEP LEARNING COURSE – EFSTRATIOS GAVVES op nn ag ain 19 86 at m Ba io aa c n EFSTRATIOS H kp in ,W r EFSTRATIOS to o p er n a bo 19 an ga s t GAVVES 97 LS d i GAVVES DEEP TM W o n, ill R – UVA ,H iam um UVA 19 oc s el h DEEP 98 hr ar –LEARNING DEEP OC e ite t, R, ra ONE Le nd 20 Sc LEARNING 06 Cu hm n LEARNING De , Bo id ep tto hu - 28 COURSE be Le u, r COURSE 20 ar Be ni ng The “AI winter” despite notable successes 09 – ‹#›– 28 Im ng io ,H an ag i n d en t o H 20 et ,D n ,O af fn 12 Al e ng sin er H exn et d er af e fn t, a l. o, Te 20 e r Le Cu h 15 Re n, sn Bo et tto (1 u, to 54 Be y da lay ng DEEPER GO er io VISLab ,D s), an M d VISLab ee SR pm A in LEARNING AND OPTIMIZATIONS - 28 INTO DEEP d The first “AI winter” (1969 ~1983) o What everybody thought ◦ “If a perceptron cannot even solve XOR, why bother?” o Results not as promised (too much hype!) → no further funding → AI Winter o Still, significant discoveries were made in this period ◦ Backpropagation à Learning algorithm for MLPs by Linnainmaa ◦ Recurrent networks à Varied-length inputs by Rumelhart ◦ CNNs à Neocognitron by Fukushima UVA DEEP LEARNING COURSE – EFSTRATIOS GAVVESEFSTRATIOS EFSTRATIOS GAVVES DEEP GAVVES –LEARNING – UVAUVA DEEP DEEP ONE LEARNING - 29 COURSE LEARNING COURSE – ‹#›– 29 DEEPER INTO DEEP VISLab VISLab LEARNING AND OPTIMIZATIONS - 29 The second “AI winter” (1995 ~ 2006) o Concurrently with Backprop and Recurrent Nets o Machine Learning models were proposed ◦ Similar accuracies with better math and proofs and fewer heuristics ◦ Better performance than neural networks with a few layers ◦ Kernel methods ◦ Support vector machines (SVMs) (Cortes; Vapnik,1995) ◦ Ensemble methods ◦ Decision trees (Tin Kam Ho, 1995), Random Forests (Breiman, 2001) o Manifold learning (~2000) ◦ Isomap, Laplacian Eigenmaps, LLE, LTSA o Sparse coding (Olshausen and Field, 1997) ◦ LASSO, K-SVD UVA DEEP LEARNING COURSE – EFSTRATIOS GAVVESEFSTRATIOS EFSTRATIOS GAVVES DEEP GAVVES –LEARNING – UVAUVA DEEP DEEP ONE LEARNING - 30 COURSE LEARNING COURSE – ‹#›– 30 DEEPER INTO DEEP VISLab VISLab LEARNING AND OPTIMIZATIONS - 30 The rise of deep learning (2006- present) DEEP LEARNING ONE – 31 19 Pe 58 r ce pt ro 19 ns 60 ,R Ad os a en lin bl e, at W t 19 id 69 Pe ro Pa ce r w an p e p tr d rt o n H 19 s, of 70 M f Ba i n Li ckp sk n n ro y an ain pa m ga d 19 aa tio 74 Ba n, ck pr UVA DEEP LEARNING COURSE – EFSTRATIOS GAVVES op 19 ag 86 at Ba io c EFSTRATIOS n, H kp in W EFSTRATIOS to r o p er n a bo 19 an ga s The thaw of the “AI winter” t GAVVES 97 d io LS W n GAVVES DEEP Sc TM ill , R – UVA hm , H iam um UVA 19 id o c s el h hu h DEEP 98 ar –LEARNING DEEP b e reit t, OC r er an ONE H R, af f n eC L d 20 LEARNING 06 er un LEARNING ,B De ot to - 32 COURSE ep u, COURSE 20 Le Be 09 ar ng – ‹#›– 32 Im ni io ng an ag en , H d et i n 20 ,D to n, 12 Al e ng Os an exn et in d et al. de H ,L ro 20 af e ,T fn Cu eh 15 Re e r n, sn Bo et tto (1 u, to 54 Be y da lay ng DEEPER GO er io VISLab ,D s), M VISLab ee SR pm A in LEARNING AND OPTIMIZATIONS - 32 INTO DEEP d The rise of deep learning o In 2006,Hinton and Salakhutdinov found multi-layer feedforward neural networks can be pretrained layer by layer. o Fine-tuned by backpropagation o Deep Belief Nets (DBNs), ◦ based on Boltzmann machines UVA DEEP LEARNING COURSE – EFSTRATIOS GAVVESEFSTRATIOS EFSTRATIOS GAVVES DEEP GAVVES –LEARNING – UVAUVA DEEP DEEP ONE LEARNING - 33 COURSE LEARNING COURSE – ‹#›– 33 DEEPER INTO DEEP VISLab VISLab LEARNING AND OPTIMIZATIONS - 33 Neural Networks: A decade ago o Lack of processing power o Lack of data o Overfitting o Vanishing gradients o Experimentally, training multi-layer perceptrons was not that useful “Are 1-2 hidden layers the best neural networks can do?” UVA DEEP LEARNING COURSE – EFSTRATIOS GAVVESEFSTRATIOS EFSTRATIOS GAVVES DEEP GAVVES –LEARNING – UVAUVA DEEP DEEP ONE LEARNING - 34 COURSE LEARNING COURSE – ‹#›– 34 DEEPER INTO DEEP VISLab VISLab LEARNING AND OPTIMIZATIONS - 34 Neural Networks: Today o Lack of processing power o Lack of data o Overfitting o Vanishing gradients o Experimentally, training multi-layer perceptrons was not that useful “Are 1-2 hidden layers the best neural networks can do?” UVA DEEP LEARNING COURSE – EFSTRATIOS GAVVESEFSTRATIOS EFSTRATIOS GAVVES DEEP GAVVES –LEARNING – UVAUVA DEEP DEEP ONE LEARNING - 35 COURSE LEARNING COURSE – ‹#›– 35 DEEPER INTO DEEP VISLab VISLab LEARNING AND OPTIMIZATIONS - 35 Deep Learning arrives o Easier to train one layer at a time → Layer-by-layer training o Training multi-layered neural networks became easier o Benefits of multi-layer networks, but single-layer easy of training Freeze layer 3 Freeze layer 2 Training layer 1 Input UVA DEEP LEARNING COURSE – EFSTRATIOS GAVVESEFSTRATIOS EFSTRATIOS GAVVES DEEP GAVVES –LEARNING – UVAUVA DEEP DEEP ONE LEARNING - 36 COURSE LEARNING COURSE – ‹#›– 36 DEEPER INTO DEEP VISLab VISLab LEARNING AND OPTIMIZATIONS - 36 Deep Learning arrives o Easier to train one layer at a time → Layer-by-layer training o Training multi-layered neural networks became easier o Benefits of multi-layer networks, but single-layer easy of training Freeze layer 3 Training layer 2 Freeze layer 1 Input UVA DEEP LEARNING COURSE – EFSTRATIOS GAVVESEFSTRATIOS EFSTRATIOS GAVVES DEEP GAVVES –LEARNING – UVAUVA DEEP DEEP ONE LEARNING - 37 COURSE LEARNING COURSE – ‹#›– 37 DEEPER INTO DEEP VISLab VISLab LEARNING AND OPTIMIZATIONS - 37 Deep Learning arrives o Easier to train one layer at a time → Layer-by-layer training o Training multi-layered neural networks became easier o Benefits of multi-layer networks, but single-layer easy of training Training layer 3 Freeze layer 2 Freeze layer 1 Input UVA DEEP LEARNING COURSE – EFSTRATIOS GAVVESEFSTRATIOS EFSTRATIOS GAVVES DEEP GAVVES –LEARNING – UVAUVA DEEP DEEP ONE LEARNING - 38 COURSE LEARNING COURSE – ‹#›– 38 DEEPER INTO DEEP VISLab VISLab LEARNING AND OPTIMIZATIONS - 38 19 Pe 58 r ce pt ro 19 ns 60 ,R Ad os a en lin bl e, at W t 19 id 69 Pe ro Pa cer w an p e p tr d rt o n H 19 s, of 70 M f Ba i n Li ckp sk n n ro y an ain pa m ga d 19 aa tio 74 Ba n, ck pr UVA DEEP LEARNING COURSE – EFSTRATIOS GAVVES op 19 ag 86 at Ba io c EFSTRATIOS n, H kp in W EFSTRATIOS to r o p er n a bo 19 s Deep Learning Renaissance an ga GAVVES 97 LS d ti o W n GAVVES DEEP Sc TM hm , H illi , Ru – UVA id o c am m UVA hu h s el h 19 r DEEP 98 ar –LEARNING DEEP OC ber eite r t, H R, an af L d ONE f n eC 20 er LEARNING un 06 ,B LEARNING De Te ep ot h Le to u, - 39 COURSE ar Be COURSE 20 ni ng 09 ng io – ‹#›– 39 Im ,H an ag in to d en n, et Os 20 ,D 12 en in Al e g de ro an xn et , d et al. H ,L 20 af e 15 fn Cu Re er n sn ,B et ot (1 to to 54 u, Be y da lay ng DEEPER GO er io VISLab ,D s), M VISLab ee SR pm A in LEARNING AND OPTIMIZATIONS - 39 INTO DEEP d Turns out: Deep Learning is Big Data Hungry! o In 2009 the ImageNet dataset was published [Deng et al., 2009] ◦ Collected images for all 100K terms in Wordnet (16M images in total) ◦ Terms organized hierarchically: “Vehicle”à“Ambulance” o ImageNet Large Scale Visual Recognition Challenge (ILSVRC) ◦ 1 million images, 1,000 classes, top-5 and top-1 error measured CNN based, non-CNN based UVA DEEP LEARNING COURSE – EFSTRATIOS GAVVESEFSTRATIOS EFSTRATIOS GAVVES DEEP GAVVES –LEARNING – UVAUVA DEEP DEEP ONE LEARNING - 40 COURSE LEARNING COURSE – ‹#›– 40 DEEPER INTO DEEP VISLab VISLab LEARNING AND OPTIMIZATIONS - 40 ImageNet: side notes o Most commonly used version: ImageNet-12: 1K categories, ~1.3M images, ~150GB o Explore them here: https://knowyourdata-tfds.withgoogle.com/#tab=STATS&dataset=imagenet2012 o (Important to also “see” the data, do not just throw a neural network at it!) Also check out: On the genealogy of machine learning datasets: A critical history of ImageNet. Denton et al. 2021 UVA DEEP LEARNING COURSE – EFSTRATIOS GAVVESEFSTRATIOS EFSTRATIOS GAVVES DEEP GAVVES –LEARNING – UVAUVA DEEP DEEP ONE LEARNING - 41 COURSE LEARNING COURSE – ‹#›– 41 DEEPER INTO DEEP VISLab VISLab LEARNING AND OPTIMIZATIONS - 41 ImageNet 2012 winner: AlexNet More weights than samples in the dataset! Krizhevsky, Sutskever & Hinton, NeurIPS 2012 UVA DEEP LEARNING COURSE – EFSTRATIOS GAVVESEFSTRATIOS EFSTRATIOS GAVVES DEEP GAVVES –LEARNING – UVAUVA DEEP DEEP ONE LEARNING - 42 COURSE LEARNING COURSE – ‹#›– 42 DEEPER INTO DEEP VISLab VISLab LEARNING AND OPTIMIZATIONS - 42 Why now? Datasets of everything (video, multi-modal, robots etc. ) Imagenet: 1,000 classes from ??? real images, 1M images Object recognition with CNN OCR with CNN Bank cheques Backpropagation Perceptron Parity, negation problems 1. Better hardware Mark I Perceptron 3. Better algorithms 2. Bigger data Potentiometers UVA DEEP LEARNING COURSE – EFSTRATIOS GAVVESEFSTRATIOS EFSTRATIOS GAVVES DEEP GAVVES –LEARNING – UVAUVA DEEP DEEP ONE LEARNING - 44 COURSE LEARNING COURSE – ‹#›– 44 DEEPER INTO DEEP VISLab VISLab LEARNING AND OPTIMIZATIONS - 44 The current scaling of models. o BERT model (354M parameters) ~ now $2K o RoBERTa (1000 GPUs for a week) ~ now $350K o GPT-3 (175B parameters, 1500 GPUs for 2 months) ~ $3M o … o PaLM ◦ 6144 TPUs, ~$25M ◦ 3.2 million kWh ~~1000 Households for a year (side note: Image models are “still” in range of o With news/hype about AI, important to stay critical. UVA DEEP LEARNING COURSE – EFSTRATIOS GAVVESEFSTRATIOS EF