Lecture 1 - Data Governance and Management (Part 1) PDF
Document Details
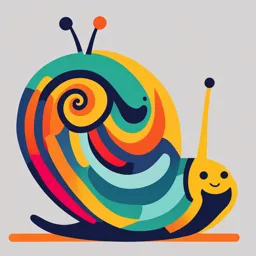
Uploaded by ConscientiousRecorder1975
National College of Ireland
John Bohan
Tags
Summary
This lecture provides an overview of data governance and management, including the definition of data governance, its importance in organizations, and the role it plays in achieving business goals. It also explains different aspects of data governance, such as data quality, integrity, and provenance, and the processes and methodologies for implementing data governance.
Full Transcript
Data Governance and Ethics WEEK 1: DATA GOVERNANCE AND MANAGEMENT (PART 1) [email protected] Updated John Bohan 27/05 1 Overview of course content 1. Data Governance and Management (Weeks 1-3) 2. Privacy and Data Protection (Weeks 4-6) 3. Ethical Issu...
Data Governance and Ethics WEEK 1: DATA GOVERNANCE AND MANAGEMENT (PART 1) [email protected] Updated John Bohan 27/05 1 Overview of course content 1. Data Governance and Management (Weeks 1-3) 2. Privacy and Data Protection (Weeks 4-6) 3. Ethical Issues Pertaining to Data (Weeks 7, 9-10) 4. Fairness, Accountability, and Transparency of Algorithmic Systems (Weeks11-13) 2 2 Data Governance and Management Week 1: DG Concepts Scope of DG and reasons for growth in interest Definitions, Terminology (Data Governance, Data Management) Introduction to DMBOK wheel Week 2: Application of concept Roles and responsibilities, DG operating model, Management of data strategies, policies, processes and procedures Week 3: DG Implementation Ladley’s 8-phase implementation process for DG Data Governance, Data Quality, Data Provenance, Data risk identification and management Models and tools for data governance 3 Week 1 Concepts – definition of DG (as part of broad system of DM), reason for growth in interest, relationship to management, governance as governance, partnership Ladley Ch 2,4 Application - data quality, integrity, provenance; roles and responsibilities, operating model Ladley Chap 3 Implementation – DG methodology – 8 stages of implementation Ladley Chap 5 3 Data Governance and Management (Part 1 – concepts and definitions) 4 4 What do we mean by corporate governance? Corporate governance is the structure of rules, practices, and processes used to direct and manage a company. A company's board of directors is the primary force influencing corporate governance. Directors The basic principles of corporate governance are accountability, transparency, fairness, and responsibility. Corporate governance goes beyond day-to-day management by considering for instance ethical behavior, corporate strategy and Management Owners risk management. Bad corporate governance can cast doubt on a company's operations and its ultimate profitability. Corporate Governance Definition: How It Works (investopedia.com) 5 5 Data and information management Good organisation data management is building block for information management, better decisions and ultimately organisational wisdom. Data Governance is the umbrella term application good corporate governance to data practices 6 IG Is Not a Project, But an Ongoing Program IG is an ongoing program, not a one-time project. I G provides an umbrella to manage and control information output and communications. Since technologies change so quickly, it is necessary to have overarching policies that can manage the various IT platforms that an organization may use.Compare it to a workplace safety program; every time a new location, team member, piece of equipment, or toxic substance is acquired by the organization, the workplace safety program should dictate how that is handled. If it does not, the workplace safety policies/procedures/training that are part of the workplace safety program need to be updated. Regular reviews are conducted to ensure the program is being followed and adjustments are made based on the findings. The effort never ends. The same is true for IG. IG is not only a tactical program to meet regulatory, compliance, and litigation demands. It can be strategic, in that it is the necessary underpinning for developing a management strategy that maximizes knowledge worker productivity while minimizing risk and costs. 6 Data Governance: What? Data governance (DG) is the process of ◦managing the availability, usability, integrity and security of the data in enterprise systems, ◦based on internal data standards and policies that also control data usage. 7 Having trusted and reliable records, reports, data, and databases enables managers to make key decisions with confidence.1 And accessing that information and business intelligence in a timely fashion can yield a long-term sustainable competitive advantage, creating more agile enterprises. To do this, organizations must standardize and systematize their handling of information. They must analyze and optimize how information is accessed, controlled, managed, shared, stored, preserved, and audited. They must have complete, current, and relevant policies, processes, and technologies to manage and control information, including who is able to access what information, and when, to meet external legal and regulatory demands and internal governance policy requirements. In short, IG is about information control and compliance. 7 Data Governance: Why? Effective data governance seeks to ◦ ensure that data quality is consistent and trustworthy ◦ ensure that data is not misused or abused. ◦ Increase the business value of data ◦ Reduce business, operational and administration costs ◦ Help to ensure the continued existence of the company through risk management and optimisation 8 In the past regulatory compliance has been big driver More recently, the need for innovation and adding value has increased the demand for DG Some companies make their living out of information and data! Enable better decision making reduce operational friction protect the needs of data stakeholders train management and staff to adopt common approaches to managing data and resolving issues Build standard repeatable data management and governance processes reduce costs and increase effectiveness through coordination efforts ensure transparency of processes 8 Data Governance: Other definitions emphasise different aspects of DG Statement of Authority: Exercise of authority and control (planning, monitoring and enforcement) over the management of data assets (DAMA DMBOK) Framework: A system of decision rights and accountabilities for information related processes, executed according to agreed upon models which describe who can take what actions, with what information and when, under what circumstances, using what methods etc Org. artefacts: The organization and implementation of policy's procedures , structure, roles and responsibilities which outline and enforce rules of engagement, decision rights and accountabilities for the effective management of information assets Org. capability: People and organizational capability, processes, controls and technologies and architecture Source: Alder, Why Data Governance? 9 9 Growth of Data The amount of data in the world is estimated to double every 18-24 months How much of it is used (or useful?) In 2019, every internet minute saw ◦ 4.5 million Google searches ◦ 55,000 Instagram posts ◦ 694,000 Netflix streams ◦ 4.5 million YouTube views Enormous increase in corporate data Source: https://www.domo.com/learn/data-never-sleeps-7 10 Estimates and projections vary, but it has been stated that 90 percent of the data existing worldwide today was created in the last two years5 and that every two days more information is generated than was from the dawn of civilization until 2003.6 This trend will continue: The global market for Big Data technology and services is projected to grow at a compound annual rate of 27 percent through 2017, about six times faster than the general information and communications technology (ICT) market.7 Smallwood IT, IG, risk, compliance, and legal representatives in organizations have a clear sense that most of the information stored is unneeded, raises costs, and poses risks. According to a survey taken at a recent Compliance, Governance and Oversight Counsel summit, respondents estimated that approximately 25 percent of information stored in organizations has real business value, while 5 percent must be kept as business records and about 1 percent is retained due to a litigation hold. “This means that [about] 69 percent of information in most companies has no business, legal, or regulatory value. 10 Data growth and the need for corporate governance The increase in data has been recognized as presenting opportunities from … ◦ improved business intelligence leading to ◦ improved efficiencies and quality control in manufacturing and service delivery ◦ more effective provision of supports within organizations such as inventory control , design etc ◦ better customer relationship management ◦ improved strategic and business planning … but also to higher risks from … ◦ data losses and data breaches leading to loss of reputation and financial losses including fines ◦ threats of hacking, ransomware, system failure 11 Focussing on corporate governance, increase has led to opportunies … risks 11 Example of poor corporate and research data governance https://www.theguardian.com/uk-news/2020/sep/24/cambridge- https://www.penguinrandomhouse.com/books/6 analytica-directorship-ban-alexander-nix?CMP=Share_AndroidApp_Other 04375/mindfck-by-christopher-wylie/ 12 12 Improve strategic direction of organisation Get better value out of customer and client information More effective operational processes Reduce costs by removing duplicated effort in data collection and Some examples maintenance All for better data analysis and better decisions of goals that a DG programme Reduce organisational friction might seek to Set clear data responsibilities between different business and support areas achieve Protect the needs of various data stakeholders within and without organisation Ensure organisational accountability and compliance Improve organisational capacity to address data issues through education and trainings Allow for transparency by adoption of standard, repeatable data processes 13 The business drivers will depend on many factors including the - Nature of the organisation and its mission (eg profit vs not-for-profit) - Its competitive challenges - Its scale - Its organisation structure (hierarchical vs flat, bureaucratic vs professional) 13 Data Governance: How? Effective data governance operates through policies, standards and tools that seek to ◦ Facilitate and improve the internal and external communication about data ◦ Provide for the identification and minimization of data-related risks ◦ Establish and control internal rules for data use ◦ Ensure the implementation of industry, national or international compliance requirements Maintain Expect Set data rules data consequences discipline 14 IG consists of the overarching polices and processes to optimize and leverage information while keeping it secure and meeting legal and privacy obligations in alignment with stated organizational business objectives. IT governance consists of following established frameworks and best practices to gain the most leverage and benefit out of IT investments and support accomplishment of business objectives. Data governance consists of the processes, methods, and techniques to ensure that data is of high quality, reliable, and unique (not duplicated), so that downstream uses in reports and databases are more trusted and accurate. 14 Governance as Government Defining data policies Like countries, organisations Setting standards and will develop a style of protocols governance that suits their Deciding on the appropriate circumstances Enterprise Data Architecture Legislature Data Governance Steering Committee Judiciary Executive Issue management Escalation of disputes Protecting and serving data structures and roles Dispute resolution Administrative responsibilities 15 The core word in governance is govern. Data governance can be understood in terms of political governance. It includes legislative-like functions (defining policies, standards, and the Enterprise Data Architecture), judicial-like functions (issue management and escalation), and executive functions (protecting and serving, administrative responsibilities). To better manage risk, most organizations adopt a representative form of data governance, so that all stakeholders can be heard. Each organization should adopt a governance model that supports its business strategy and is likely to succeed within its own cultural context. Organizations should also be prepared to evolve that model to meet new challenges. Models differ with respect to their organizational structure, level of formality, and approach to decision-making. Some models are centrally organized, while others are distributed. 15 Data Governance vs Data Management Data Management is the process of gathering, storing, organizing and maintaining data created and collected by an organization. Data Governance determines the overall practice of data management. It is regarded as the “management of data management” and envisages a separation between those governing and those managing separation between those governing and those managing “Doing things right” vs “Doing the right thing” Even if an organisation has no specific Data Government practices, things still get done. But are the right things being done? The issue is whether a clear structure and roles need to defined through agreed policies, operational processes and procedure Why the importance of documenting what these are? Induction and training of staff Accountability and audit External consumption (media, regulators) 16 Source: Ladley 2012 Chapter 2. Definitions and concepts | Data Governance (oreilly.com) 16 Data Governance: Who? Data Governance includes the people, processes and technologies needed to manage and protect the company’s data assets in order to guarantee generally understandable, correct, complete, trustworthy, secure and discoverable corporate data. 17 17 Data Governance as partnership DG requires a partnership between business and IT to Provide an effective and consistent means of distributing data to those who need it for business purposes Calibrate and empower access to appropriate use of data Providing quality assurance about data Avoid retaining or gathering redundant data 18 18 Data Governance should be business led with IT input not the other way around Data Governance should be business led with IT input not the other way around Like other management processes, it must balance strategic and operational needs. This balance can best be struck by following a set of principles that recognize salient features of data management and guide data management practice. An organisations management should start with identifying its data principles that might include ◦ Effective data management requires leadership commitment ◦ Data is an asset that needs to be planned and accounted for like other assets ◦ Managing data includes managing the risks associated with data not just the opportunities ◦ Data management requires an organisation-wide perspective that must drive Information Technology decisions, not the other way around 19 Data management shares characteristics with other forms of asset management, as seen in Figure 1. It involves knowing what data an organization has and what might be accomplished with it, then determining how best to use data assets to reach organizational goals. Like other management processes, it must balance strategic and operational needs. This balance can best be struck by following a set of principles that recognize salient features of data management and guide data management practice. Data is an asset with unique properties: Data is an asset, but it differs from other assets in important ways that influence how it is managed. The most obvious of these properties is that data is not consumed when it is used, as are financial and physical assets. The value of data can and should be expressed in economic terms: Calling data an asset implies that it has value. While there are techniques for measuring data’s qualitative and quantitative value, there are not 19 yet standards for doing so. Organizations that want to make better decisions about their data should develop consistent ways to quantify that value. They should also measure both the costs of low quality data and the benefits of high quality data. 19 Managing data means managing the quality of data: Ensuring that data is fit for purpose is a primary goal of data management. To manage quality, organizations must ensure they understand stakeholders’ requirements for quality and measure data against these requirements. It takes Metadata to manage data: Managing any asset requires having data about that asset (number of employees, accounting codes, etc.). The data used to manage and use data is called Metadata. Because data cannot be held or touched, to understand what it is and how to use it requires definition and knowledge in the form of Metadata. Metadata originates from a range of processes related to data creation, processing, and use, including architecture, modeling, stewardship, governance, Data Quality management, systems development, IT and business operations, and analytics It takes planning to manage data: Even small organizations can have complex technical and business process landscapes. Data is created in many places and is moved between places for use. To coordinate work and keep the end results aligned requires planning from an architectural and process perspective. Data management is cross-functional; it requires a range of skills and expertise: A single team cannot manage all of an organization’s data. Data management requires both technical and non-technical skills and the ability to collaborate. 20 Data management requires an enterprise perspective: Data management has local applications, but it must be applied across the enterprise to be as effective as possible. This is one reason why data management and data governance are intertwined. Data management must account for a range of perspectives: Data is fluid. Data management must constantly evolve to keep up with the ways data is created and used and the data consumers who use it. Data management is lifecycle management: Data has a lifecycle and managing data requires managing its lifecycle. Because data begets more data, the data lifecycle itself can be very complex. Data management practices need to account for the data lifecycle. Different types of data have different lifecycle characteristics: And for this reason, they have different management requirements. Data management practices have to recognize these 20 differences and be flexible enough to meet different kinds of data lifecycle requirements. Managing data includes managing the risks associated with data: In addition to being an asset, data also represents risk to an organization. Data can be lost, stolen, or misused. Organizations must consider the ethical implications of their uses of data. Data-related risks must be managed as part of the data lifecycle. 20 21 Data management requirements must drive Information Technology decisions: Data and data management are deeply intertwined with information technology and information technology management. Managing data requires an approach that ensures technology serves, rather than drives, an organization’s strategic data needs. Effective data management requires leadership commitment: Data management involves a complex set of processes that, to be effective, require coordination, collaboration, and commitment. Getting there requires not only management skills, but also the vision and purpose that come from committed leadership. 21 Data management and data governance 22 22 Data management and data governance Data Governance could be regarded as the “management of data management” It envisages a separation between those governing and those managing See figure 15 from DAMA- DMBOK See also https://www.boardeffect.com/blog/diff erence-between-governance- management/ 23 23 DAMA-DMBOK2 Data Management Framework The scope and focus of a particular data governance program will depend on organizational needs, but most programs include: Strategy: Defining, communicating, and driving execution of Data Strategy and Data Governance Strategy Policy: Setting and enforcing policies related to data and Metadata management, access, usage, security, and quality Standards and quality: Setting and enforcing Data Quality and Data Architecture standards Oversight: Providing hands-on observation, audit, and correction in key areas of quality, policy, and data management (often referred to as stewardship) Compliance: Ensuring the organization can meet data-related regulatory compliance requirements Issue management: Identifying, defining, escalating, and resolving issues related to data security, data access, data quality, regulatory compliance, data ownership, policy, standards, terminology, or data governance procedures Data management projects: Sponsoring efforts to improve data management practices Data asset valuation: Setting standards and processes to consistently define the business value ofdata assets 24 24 DAMA DMBOK “wheel” Professional discipline of data management addresses data and information as an “information asset”) (O’Keeffe et al 2018, Chapter 5) DAMA defines data governance as “The exercise of an authority and control (planning, monitoring and enforcement) over the management of data assets” Data governance enables an organization to determine proper action regarding data and data processing, clear definitions, decision making rights and responsibilities and then escalation path or mediation process where issues arise. 25 Data management association 25 Data management knowledge areas Data governance enables an organization to determine proper action regarding data and data processing, clear definitions, decision making rights and responsibilities and then escalation path or mediation process where issues arise. The Data Governance function guides all other data management functions. DG includes the people, processes and technologies required to ensure good quality data, its effective management and overall governance These can be grouped in a number of knowledge areas Should reflect the organisational circumstance; not a case of “one size fits all”. 26 “All organizations make decisions about data, regardless of whether they have a formal Data Governance function. Those that establish a formal Data Governance program exercise authority and control with greater intentionality (Seiner, 2014). Such organizations are better able to increase the value they get from their data assets.” (DMBOK p67) 1. Data Governance provides direction and oversight for data management by establishing a system of decision rights over data that accounts for the needs of the enterprise. (Chapter 3) 2. Data Architecture defines the blueprint for managing data assets by aligning with organizational strategy to establish strategic data requirements and designs to meet these requirements. (Chapter 4) 3. Data Modeling and Design is the process of discovering, analyzing, representing, and communicating data requirements in a precise form called the data model. (Chapter 5) 4. Data Storage and Operations includes the design, implementation, and support of stored data to 26 maximize its value. Operations provide support throughout the data lifecycle from planning for to disposal of data. (Chapter 6) 5. Data Security ensures that data privacy and confidentiality are maintained, that data is not breached, and that data is accessed appropriately. (Chapter 7). 26 6. Data Integration and Interoperability includes processes related to the movement and consolidation of data within and between data stores, applications, and organizations. (Chapter 8) 7. Document and Content Management includes planning, implementation, and control activities used to manage the lifecycle of data and information found in a range of unstructured media, especially documents needed to support legal and regulatory compliance requirements. (Chapter 9) 8. Reference and Master Data includes ongoing reconciliation and maintenance of core critical shared data to enable consistent use across systems of the most accurate, timely, and relevant version of truth about essential business entities. (Chapter 10) 9. Data Warehousing and Business Intelligence includes the planning, implementation, and control processes to manage decision support data and to enable knowledge workers to get value from data via analysis and reporting. (Chapter 11) 27 10. Metadata includes planning, implementation, and control activities to enable access to high quality, integrated Metadata, including definitions, models, data flows, and other information critical to understanding data and the systems through which it is created, maintained, and accessed. (Chapter 12) 11. Data Quality includes the planning and implementation of quality management techniques to measure, assess, and improve the fitness of data for use within an organization (Chapter 13) 27 Data Governance and Management Week 1: DG Concepts Scope of DG and reasons for growth in interest Definitions, Terminology (Data Governance, Data Management) Introduction to DMBOK wheel Week 2: Application of concept Roles and responsibilities, DG operating model, Management of data strategies, policies, processes and procedures Week 3: DG Implementation Ladley’s 8-phase implementation process for DG Data Governance, Data Quality, Data Provenance, Data risk identification and management Models and tools for data governance 28 Week 1 Concepts – definition of DG (as part of broad system of DM), reason for growth in interest, relationship to management, governance as governance, partnership Ladley Ch 2,4 Application - data quality, integrity, provenance; roles and responsibilities, operating model Ladley Chap 3 Implementation – DG methodology – 8 stages of implementation Ladley Chap 5 28 Questions? 29 29 Additional material CAN BE ADDED AT FUTURE STAGE 30 30 Data growth is at an exponential rate Source: http://ec1702991.blogspot.com/2018/12/3.html 31 http://ec1702991.blogspot.com/2018/12/3.html Kryder's law - The idea that data storage will double almost every year (13 months), as the storage capacity increases, storage will decrease in price. https://searchstorage.techtarget.com/definition/Kryders-Law growth of data - Data is increasing at an exponential rate, more data has been made in the past two years than ever before combined. It is estimated that by the year 2020, 1.7MB of new data will be made every second for every human on earth. Within 5 years there will be 50 billion smart connected devices on earth. also by 2020, at least 1/3 of all the world's data will pass through the cloud. The image below contains more examples of data growth as well as a graph for visual representation 31