Neural Networks and Deep Learning Lecture 02 - PDF
Document Details
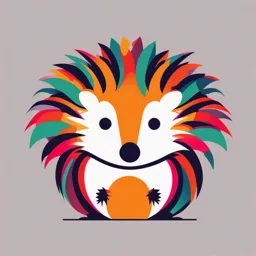
Uploaded by InnocuousAntagonist2066
null
Dr. Zein Al Abidin IBRAHIM
Tags
Summary
This lecture introduces fundamental concepts of neural networks and deep learning. It covers topics like neural networks, activation functions, the McCulloch-Pitts model, and various types of neural networks, such as MLPs, RNNs, CNNs, and LSTMs.
Full Transcript
MLAI504 501 NEURAL NETWORKS AND MLAI504 – 2024/2025 DEEP LEARNING Dr. Zein Al Abidin IBRAHIM [email protected] NEURAL NETWORKS: A QUICK OVERVIEW DEEP LEARNING BRAIN 3 WHAT IS NEURAL NETWORKS ? ▪...
MLAI504 501 NEURAL NETWORKS AND MLAI504 – 2024/2025 DEEP LEARNING Dr. Zein Al Abidin IBRAHIM [email protected] NEURAL NETWORKS: A QUICK OVERVIEW DEEP LEARNING BRAIN 3 WHAT IS NEURAL NETWORKS ? ▪ Idea → inspired from human Brain ▪ A brain is composed of a vary large number of processing units → called neurons. ▪ Number approximately = 1011 = 10 milliard neurons ▪ Neurons works in parallel ▪ Computational power of brain is very high ▪ In addition → large connectivity of neurons ▪ Connections called synapses ▪ Neurons have connections to around 104 other neurons. ▪ Brain take approximately 100-200ms to perform perceptual recognition 4 WHAT IS ANN? ▪ NN → massive number of parallel distributed processing units ▪ Can store experiential knowledge (experiences) ▪ Similar to brain in two main things: 1. Knowledge → acquired from its environment through a learning phase. 2. Interneuron connection weights used to store the acquired knowledge. 5 LOOKING INSIDE HUMAN BRAIN 6 HOW TO MODEL A NEURON ▪ Another representation for the bias is to consider it as an additional neuron, the summation this time Starts from zero. ▪ 𝑣𝑘 = σ𝑚 𝑗=0 𝑤𝑘𝑗 𝑥𝑗 ▪ 𝑦𝑘 = 𝜑(𝑣𝑘 ) ▪ 𝑥0 = +1, 𝑤𝑘0 = 𝑏𝑘 Knowledge 7 SOME ACTIVATION FUNCTIONS 8 MCCULLOCH-PITTS MODEL ▪ The first computational model of a neuron was proposed by Warren MuCulloch (neuroscientist) and Walter Pitts (logician) in 1943. ▪ Inputs → Boolean ▪ Output → Boolean ▪ Activation → thresholding ▪ What we can do with → OR, AND, > ▪ No learning from data ▪ Just a theoretical model 9 MCCULLOCH-PITTS MODEL 10 ONE-INPUT NEURON: EXAMPLE ▪ 1D pattern p ▪ The associated weight = w ▪ The bias value is b ▪ The output is n ▪ The value n passes through the activation function f to generate the output a ▪ Ex: w=3, p=2, b=-1.5 ▪ a=f(3(2)-1.5)=f(4.5) 11 MULTIPLE-INPUT NEURON: EXAMPLE 12 THE NEURON IN THE SPACE ▪ Output of the neuron can be written: ▪ 𝑦 = 𝑤 𝑇 𝑥 with w=[w0, w1,….wd] & x=[1, x1, ….,xd]T ▪ Weights we need to be learned from training data such that the patterns of the training data are correctly classified ▪ Ex: when d = 1 one-dimension patterns ▪ y = w x + w0 is the equation of a line ▪ The line separate the space in two zones positive-side & negative-side ▪ Find weights in such a way →for any new input x, assign it to one of two classes depending in which side is. 13 HOW TO REPRESENT A NEURAL NETWORKS: DIRECTED GRAPHS kel layer b2lba majmo3a mn neurons , neuron be nfs layer msh mrtebten be b3d …… … 14 HOW TO REPRESENT NEURAL NETWORKS: DIRECTED GRAPHS ▪ The directed graph can be complete (known as fully- connected or dense) or incomplete. ▪ Fully-connected → each neuron in layer n is connected to each neuron in layer n+1 (see figure below). ▪ Incomplete (known as partially complete)→ a neuron in layer n is connected to some neurons in layer n+1 (used in CNN, RNN, …). ▪ referred to as an architectural graph 15 SOME NETWORK ARCHITECTURES (1/2) ▪ Here are some network architectures: ▪ Feedforward Neural Networks (Multilayer Perceptron (MLP)) ▪ Used in simple classification and regression tasks where the input data is structured. ▪ RNN → Recurrent Neural Networks ▪ Used in NLP for time-series prediction, speech recognition, and any task where order and context over sequences are important. ▪ CNN→ Convolutional Neural Networks ▪ Used in image and video recognition, object detection, segmentation, and any task involving visual data. ▪ LSTM → Long Short-Term Memory Networks ▪ Similar to RNNs but more efficient for longer sequences and temporal dependencies. Used extensively in NLP tasks, machine translation, and speech processing. ▪ Transformer Networks ▪ Transformer networks use attention mechanisms to process input data, enabling them to capture global dependencies in sequences of data. ▪ Used in NLP tasks such as translation, summarization, and language modeling 16 (e.g., BERT, GPT). Used also in image processing (Vision Transformers). SOME NETWORK ARCHITECTURES (2/2) ▪ Here are some network architectures: ▪ GAN → Generative Adversarial Networks ▪ Consists of two neural networks, a generator and a discriminator, that compete against each other in a zero-sum game. ▪ The generator creates fake data, and the discriminator tries to distinguish between real and fake data. ▪ Used for image generation, style transfer, data augmentation, and synthetic data generation for various applications. ▪ Autoencoders ▪ Consists of an encoder and a decoder. The encoder compresses the input into a latent-space representation, and the decoder reconstructs the input from this representation. ▪ Used for dimensionality reduction, image denoising, anomaly detection, and generating data (e.g., variational autoencoders). ▪ Graph Neural Networks (GNN) ▪ designed to work with graph-structured data. They use nodes, edges, and adjacency matrices to process data. ▪ Used for Social network analysis, molecular biology, recommendation systems, and any application involving network or graph-structured data. ▪ Capsule Networks (CapsNets) ▪ attempt to model hierarchical relationships in data more effectively than traditional CNNs. ▪ Used for image classification, object segmentation, and tasks requiring spatial hierarchies in the data. 17 ▪... FEEDFORWARD NETWORK 18 SINGLE-LAYER AND MULTI-LAYER lezem 23mel vectorization (5astn bel network) Weight matrix 1 Weight matrix 2 Weight matrix 3 19 (lzem ytl3 1 be2e zeros bel output) EXAMPLE – USE CASE car o 2ham she hidden layer train 0 bicycle 1 ▪ Consider a handwritten-digit recognition problem. Ex: eza binary class fe ykon 1 output spam/not ▪ Input → pixels of the image each pixel as separate feature spam ▪ Output → one of the 10 digits (10 neuron) ▪ Training set → large variety of handwritten digits that are representative of a real-world situation. ▪ Design of the network ▪ Input dimension = number of pixels in the image. ▪ Output = 10 neurons each represent one of the 10 digits 784*100* 100*100 *100*10 0-9 output 20 BUILDING PRIOR INFORMATION INTO NN DESIGN ▪ Examples of how to build prior information into the NN design. ▪ We have ad hoc procedures: ▪ Restricting the network architecture which is achieved through the use of local connections known as receptive fields. ▪ Constraining the choice of synaptic weights which is implemented through the use of weight sharing. kermel msh kel b7aje la kel weights fe local connection w nsabet weight Partially connected feedforward network. 21 EXAMPLE: LOCAL CONNECTIONS AND WEIGHTS SHARING WEIGHTS SHARING LIKE IN CNN NO NEED TO HAVE DIFFERENT WIGHTS 22 BUILDING INVARIANCES INTO NN DESIGN ▪ Problem to consider during NN design: ▪ Network trained to detect objects → what if objects appear rotated, translated, scaled, different colors than during training? ▪ → The classifier should be invariant to these transformations. ▪ How to do that? ▪ Invariant by structure ▪ Invariance by training ▪ Invariant by feature space 23 BUILDING INVARIANCES INTO NN DESIGN ▪ Invariant by structure ▪ Weights of neurons are created so that transformed versions of the same input are forced to produce the same output. ▪ Disadvantage → the number of synaptic connections becomes prohibitively large even for images of moderate size. ▪ Invariance by training ▪ Train the network to recognize the image and its rotations (different aspect) views. ▪ Data Augmentation → Generate more samples from your dataset by applying several type of transformations (rotated, scaled, translated, …). ▪ Disadvantages → Computational cost, overfitting, … 24 BUILDING INVARIANCES INTO NN DESIGN ▪ Invariant by feature space ▪ Extract features to characterize the essential information content of patterns that are invariant to transformations of the input. ▪ Advantages ▪ The number of features applied may be reduced to realistic levels. ▪ The requirements imposed to the network are relaxed. ▪ Invariance for all objects with respect to known transformation is assured. ▪ Disadvantage ▪ Not easy and should pass by feature engineering step to study the set of features. 25 LEARNING PROCESS ▪ Learning can be with a teacher or without a teacher. ▪ Learning with a teacher can be categorized into supervised and reinforced learning. ▪ Learning with a Teacher → Main steps ▪ Pattern in dataset are fed into the network ▪ The error is calculated as the difference between the predicted and real label. ▪ Weights are updated in backward manner relative to their amount. ▪ Updates is done until reaching an optimum value 26 LEARNING PROCESS ▪ Learning with a Teacher ▪ Updating the weights is done in the direction of the gradient ▪ The update is done in one of the three ways: ▪ Batch-training → one update after processing a batch of samples. ▪ Stochastic training → one update after processing one individual sample. ▪ Mini-batch training → one update after processing a small subset of data Weights updating Error function 27 LEARNING PROCESS 28