Principal Components Analysis and Factor Analysis PDF
Document Details
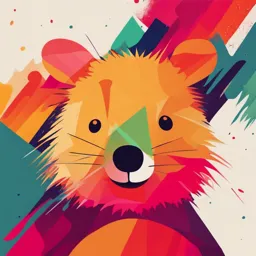
Uploaded by MemorableHonor
Tags
Summary
This document is a lecture/presentation on principal components analysis (PCA) and factor analysis (FA). It provides an overview of the techniques, their applications, and some examples. It covers topics from introductory concepts to more advanced methods.
Full Transcript
Principal Components Analysis and Factor Analysis Introduction Principal Components Analysis Factor Analysis What to Watch Out for PCA and FA 1 Introduction Principal Components Analysis (PCA) and Factor Analysis (FA) are statistical techniques used to identify a relatively small number of...
Principal Components Analysis and Factor Analysis Introduction Principal Components Analysis Factor Analysis What to Watch Out for PCA and FA 1 Introduction Principal Components Analysis (PCA) and Factor Analysis (FA) are statistical techniques used to identify a relatively small number of factors that can be used to represent relationships among sets of many interrelated variables. These techniques (PCA and FA) differ from regression analysis in that we do not have a dependent variable to be explained by a set of independent variables. In very general terms, both can be seen as approaches to summarising and uncovering any patterns in a set of multivariate data. The details behind each method are, however, quite different. In PCA the major objective is to select a number of components that explain as much of the total variance as possible. The values of the PCs for a given individual are relatively simple to compute and interpret. On the other hand, the factors obtained in FA are selected mainly to explain the interrelationships among the original variables. 2 Principal Components Analysis Principal Components Analysis is amongst the oldest and most widely used multivariate technique. The technique can be summarized as a method of transforming the original variables into new, uncorrelated variables (called the principal components ), each of which is defined to be a particular linear combination of the original variables. In other words, principal components analysis is a transformation from the observed variables, X1, …, XP, to variables C1, …, CP (i.e. principal components). We express this as follows (so called the principal components model): C1 = a11 X1 + a12 X2 + … + a1P XP C2 = a21 X1 + a22 X2 + … + a2P XP … … … (1) CP = aP1 X1 + aP2 X2 + … + aPP XP 3 Principal Components Analysis (cont.) The coefficients defining each new variable Ci (i.e. principal component), are chosen so that the following conditions hold: Var (C1) Var (C2) … Var (CP) The values of any two principal components are uncorrelated For any principal component the sum of the squares of the coefficients is one: (ai1)2 + (ai2)2 + … + (aiP)2 = 1, i=1,2,…P. In other words, C1 is the linear combination of X variables with the largest variance. Subject to the condition that it is uncorrelated with C1, C2 is the linear combination of X variables with the largest variance. Similarly, C3 has the largest variance subject to the condition that it is uncorrelated with C1 and C2; etc. It can be proved that the Var(Ci) in fact are the eigenvalues (i) of the variance-covariance matrix of the X variables and these P variances add up to the original total variance. That is, Var(C1) + Var (C2) + … + Var(CP) = 1 + 2 + … + P = Var (X1) + Var (X2) + …+ Var (XP) (Proofs - refer to on pages 432~433) 4 PC Model in Matrix Terms Let XT = (X1 , X2 , … , XP ) and aiT = (ai1, ai2, … , aiP ), i=1,2, …, P C1 = a11 X1 + a12 X2 + … + a1P XP = a1TX C2 = a21 X1 + a22 X2 + … + a2P XP = a2TX ……………. CP = aP1 X1 + aP2 X2 + … + aPP XP = aPTX That is, First PC = a1TX that maximizes Var(a1TX)= a1Ta1 Subject to a1Ta1=1 Second PC = a2TX that maximizes Var(a2TX ) Subject to a2Ta2 = 1 and Cov(a1TX, a2TX) = 0 The ith PC = aiT X that maximizes Var(aiT X ) Subject to aiT ai = 1 and Cov(ajTX, aiTX) = 0 for j