AI Programming Languages PDF
Document Details
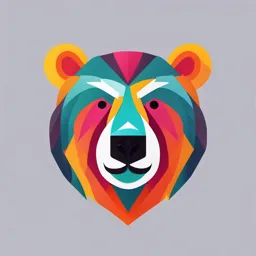
Uploaded by SnazzyLucchesiite9861
Tags
Summary
This document discusses various programming languages used in artificial intelligence (AI) development. It highlights Python, Java, and Prolog as popular choices, emphasizing their strengths and characteristics. The document also mentions the importance of understanding the strengths and specifics of each AI programming language for project development.
Full Transcript
LANGUAGES USED IN AI Artificial Intelligence is a very imperative technology to develop and construct innovative computer programs and systems, which can be used to mimic various intelligence processes like knowledge, learning and reasoning. There are numerous programming languages out there, each...
LANGUAGES USED IN AI Artificial Intelligence is a very imperative technology to develop and construct innovative computer programs and systems, which can be used to mimic various intelligence processes like knowledge, learning and reasoning. There are numerous programming languages out there, each with its own merits and areas of strength. Which programming language we use for your AI project depends on specific requirements. Some AI programming languages excel at handling large swathes of data and crunching big numbers, others shine at natural language programming. Understanding the strengths and specifics of each language will help us determine the perfect fit for our project. Lets us discuss the popularly used languages to develop AI projects in this section. 1. PYTHON Python tends to top the list of best AI programming languages, no matter how you slice it up. The fact that it has been around for so long and has consistently performed well as a general purpose programming language that can be used for front-end or beck-end development. It is easy to learn, produces clear, streamlined code, and works well for new as well as experienced developers. With a clearly defined syntax and simple English keywords, Python is highly readable, and easy to learn. It is one of the most powerful and easy programming languages that anyone can start to learn. Python is initially developed in the early stage of 1991. Most of the developers and programmers choose Python as their favorite programming language for developing Artificial Intelligence solutions. Python is worldwide popular among all developers and experts because it has more career opportunities than any other programming language. Python also comes with some default sets of standards libraries and also provides better community support to its users. Further, Python is a platform-independent language and also provides an extensive framework for Deep Learning, Machine Learning, and Artificial Intelligence. Python is also a portable language as it is used on various platforms such as Linux, Windows, Mac OS, and UNIX. Features of Python It is easy to learn than any other programming language. It is also a dynamically-typed language. Python is an Object-oriented language. It provides extensive community support and a framework for ML and DL. Open-source. Large standard sets of libraries. Interpreted language. 2. JAVA One of the most widely used programming languages in the world, Java has unique qualities that make it a top contender among the best AI development languages in 2022. In fact, it has been so for a long time now. Java is an object-oriented programming language that offers easy debugging and simple syntax. Having a proven track record in software development, mobile app development and now even AI development, Java continues to win over developers with every new generation. It is also the most widely used programming language by all developers and programmers to develop machine learning solutions and enterprise development. Similar to Python, Java is also a platform- independent language as it can also be easily implemented on various platforms. Further, Java is an object-oriented and scalable programming language. Java allows virtual machine technology that helps to create a single version of the app and provides support to your business. The best thing about Java is once it is written and compiled on one platform, then you do not need to compile it again and again. This is known as WORA (Once Written Read/Run Anywhere) principle. Features of Java Java has so many features which make Java best in industry and to develop artificial intelligence applications: Portability Cross-platform. Easy to learn and use. Easy-to-code Algorithms. Built-in garbage collector. Swing and Standard Widget Toolkit. Simplified work with large-scale projects. Better user interaction. Easy to debug. 3. PROLOG Prolog has been around for a long time, as long as since 1972. However, instead of calling it an old language, experts would call it a well-aged, mature AI programming language. It is a logical, declarative programming language developed for natural language processing. How good it is at that job can be understood by the fact that IBM Watson uses Prolog in parsing natural language in fielding human-generated questions. It is one of the oldest programming languages used for Artificial Intelligence solutions. Prolog stands for "Programming in Logic", which was developed by French scientist Alain Colmerauer in 1970. For AI programming in Prolog, developers need to define the rules, facts, and the end goal. After defining these three, the prolog tries to discover the connection between them. Programming in AI using Prolog is different and has several advantages and disadvantages. Features of Prolog Supports basic mechanisms such as Pattern Matching, Tree-based data structuring, and Automatic backtracking. Prolog is a declarative language rather than imperative. 4. R A number of AI applications involve big data and crunching of big numbers. Did you know that a special language was developed just for the purpose of statistical computing? That’s right, R was created by statisticians just for performing computations and crunch massive data sets with ease in a matter of seconds. Today, R is a powerful language used for machine learning programming applications, and any artificial intelligence applications that involve extensive computation or data analysis. is one of the great languages for statistical processing in programming. However, R supports free, open-source programming language for data analysis purposes. It may not be the perfect language for AI, but it provides great performance while dealing with large numbers. Some inbuilt features such as built-in functional programming, object-oriented nature, and vectorial computation make it a worthwhile programming language for AI. Features of R programming R is an open-source programming language, which is free of cost, and also you can add packages for other functionalities. R provides strong & interactive graphics capability to users. It enables you to perform complex statistical calculations. It is widely used in machine learning and AI due to its high-performance capabilities 5. C++ An highly reliable object-oriented programming language that has vast applications in AI development is C++. Its rapid processing speed makes it of particular interest to complex machine learning models as it allows them to run with high efficiency. C++ boasts some extremely useful attributes. It generates a compact code allowing modules to run with high efficiency. It is a statically typed language which means type errors don’t appear during runtime. C++ also offers dynamic load balancing, adaptive caching, memory management and a host of other features that make it a highly favored AI programming language. Features of C++ C++ is one of the fastest languages, and it can be used in statistical techniques. It can be used with ML algorithms for fast execution. Most of the libraries and packages available for Machine learning and AI are written in C++. It is a user friendly and simple language. 6. LISP LISP is probably the oldest AI programming language. In fact, the very term Artificial Intelligence was coined by John McCarthy, the inventor of LISP. It is one of the most flexible programming languages with automatic garbage collection, quick prototyping abilities, dynamic object creation, and support for symbolic expressions. LISP was used to express the earliest AI programs such as ELIZA. The inventor of LISP programming was John McCarthy, who coined the term Artificial Intelligence. LISP is one of the most efficient programming languages for solving specific problems. Currently, it is mainly used for machine learning and inductive logic problems. Features of LISP The program can be easily modified, similar to data. Make use of recursion for control structure rather than iteration. Garbage Collection is necessary. We can easily execute data structures as programs. An object can be created dynamically. 7. JULIA JULIA IS by MIT in 2012, Julia is a relatively new AI programming language designed to effectively handle expansive numerical analysis and handle large data sets with ease. The engineers at MIT designed Julia keeping in mind all the requirements of modern AI development. It possesses remarkable speed, powerful computational capacity, easy script like syntax and much more, helping developers make the best AI programming. Julia is a comparatively new language, which is mainly suited for numerical analysis and computational science. It contains several features that can be very helpful in AI programming. Features of Julia Common numeric data types. Arbitrary precision values. Robust mathematical functions. Built-in package manager. Ability to work for both parallel and distributed computing. Support for multiple dispatches. Support for C functions. 8. HASKEL Haskell is a purely functional, modern AI programming language with far reaching advantages in Artificial intelligence programming. It has advanced features such as type classes that enable type- safe operator overloading. ll these features make Haskell ideal for research, teaching and industrial applications. Its flexibility and error handling capacity, made Haskell one of the safest AI programming language. UNIT 1: LECTURE 8- TYPES OF AI Types of Artificial Intelligence: Artificial Intelligence can be divided in various types, there are mainly two types of main categorization which are based on capabilities and based on functionally of AI. Following is flow diagram which explain the types of AI. AI type-1: Based on Capabilities 1. Weak AI or Narrow AI: Narrow AI is a type of AI which is able to perform a dedicated task with intelligence.The most common and currently available AI is Narrow AI in the world of Artificial Intelligence. Narrow AI cannot perform beyond its field or limitations, as it is only trained for one specific task. Hence it is also termed as weak AI. Narrow AI can fail in unpredictable ways if it goes beyond its limits. Apple Siriis a good example of Narrow AI, but it operates with a limited pre-defined range of functions. IBM's Watson supercomputer also comes under Narrow AI, as it uses an Expert system approach combined with Machine learning and natural language processing. Some Examples of Narrow AI are playing chess, purchasing suggestions on e-commerce site, self-driving cars, speech recognition, and image recognition. 2. General AI: General AI is a type of intelligence which could perform any intellectual task with efficiency like a human. The idea behind the general AI to make such a system which could be smarter and think like a human by its own. Currently, there is no such system exist which could come under general AI and can perform any task as perfect as a human. The worldwide researchers are now focused on developing machines with General AI. As systems with general AI are still under research, and it will take lots of efforts and time to develop such systems. 3. Super AI: Super AI is a level of Intelligence of Systems at which machines could surpass human intelligence, and can perform any task better than human with cognitive properties. It is an outcome of general AI. Some key characteristics of strong AI include capability include the ability to think, to reason,solve the puzzle, make judgments, plan, learn, and communicate by its own. Super AI is still a hypothetical concept of Artificial Intelligence. Development of such systems in real is still world changing task. Artificial Intelligence type-2: Based on functionality 1. Reactive Machines Purely reactive machines are the most basic types of Artificial Intelligence. Such AI systems do not store memories or past experiences for future actions. These machines only focus on current scenarios and react on it as per possible best action. IBM's Deep Blue system is an example of reactive machines. Google's AlphaGo is also an example of reactive machines. 2. Limited Memory Limited memory machines can store past experiences or some data for a short period of time. These machines can use stored data for a limited time period only. Self-driving cars are one of the best examples of Limited Memory systems. These cars can store recent speed of nearby cars, the distance of other cars, speed limit, and other information to navigate the road. 3. Theory of Mind Theory of Mind AI should understand the human emotions, people, beliefs, and be able to interact socially like humans. This type of AI machines are still not developed, but researchers are making lots of efforts and improvement for developing such AI machines. 4. Self-Awareness Self-awareness AI is the future of Artificial Intelligence. These machines will be super intelligent, and will have their own consciousness, sentiments, and self-awareness. These machines will be smarter than human mind. Self-Awareness AI does not exist in reality still and it is a hypothetical concept. Types of artificial intelligence Another perspective on the types of AI has been classified according to the application of AI as follows. Analytic AI Powered with machine learning (including its most advanced deep learning techniques), analytic AI scans tons of data for dependencies and patterns to ultimately produce recommendations or provide a business with insights, thus contributing to data-driven decision-making. Sentiment analysis and supplier risk assessment are just a few examples of analytic AI in action. If you’d like to get a complete picture of how such a solution works, our experts have summarized the insights gained from their experience with two of the use cases – inventory optimization and demand forecasting. Functional AI Functional AI is very similar to analytic AI – it also scans huge amounts of data and searches for patterns and dependencies in it. However, instead of giving recommendations, functional AI takes actions. For instance, being the part of the IoT cloud, it can spot a machine-breakdown pattern in the sensor data received from a certain machine, and trigger a command to turn this machine off. Another example: robots that Amazon uses Play Video to the pickers, thus speeding up the picking process. Interactive AI This type of AI allows businesses to automate communication without compromising on interactivity. To envisage this type of AI, think of chatbots and smart personal assistants whose abilities can vary from answering pre-built questions to understanding the conversation context. Interactive AI can serve another purpose – improving a company’s internal processes. For example, one of our projects was dedicated to creating a chatbot to facilitate the corporate process of vacation booking. Text AI Businesses that use text AI can enjoy text recognition, speech-to-text conversion, machine translation, and content generation capabilities. Even if a company is not Google or Amazon, or any other giant company that provides text AI as a service, it can still take advantage of this AI type. For example, the company can use text AI to power an internal corporate knowledge base. Contrary to a traditional knowledge base that rests upon a search by keywords, an AI-powered one can find the document containing the most relevant answer even if the document doesn’t have full keywords. This is possible thanks to semantic search and natural language processing, which allow AI to build semantic maps and recognize synonyms to understand the context of the user’s question. Visual AI With visual AI, businesses can identify, recognize, classify and sort objects or convert images and videos into insights. A computer system that helps an insurer to estimate damage based on damaged car photos or a machine that grades apples based on their color and size are the examples of visual AI. This type of AI covers computer vision or augmented reality fields. What is knowledge representation? Humans are best at understanding, reasoning, and interpreting knowledge. Human knows things, which is knowledge and as per their knowledge they perform various actions in the real world. But how machines do all these things comes under knowledge representation and reasoning. Hence we can describe Knowledge representation as following: Knowledge representation and reasoning (KR, KRR) is the part of Artificial intelligence which concerned with AI agents thinking and how thinking contributes to intelligent behavior of agents. It is responsible for representing information about the real world so that a computer can understand and can utilize this knowledge to solve the complex real world problems such as diagnosis a medical condition or communicating with humans in natural language. It is also a way which describes how we can represent knowledge in artificial intelligence. Knowledge representation is not just storing data into some database, but it also enables an intelligent machine to learn from that knowledge and experiences so that it can behave intelligently like a human. What to Represent: Following are the kind of knowledge which needs to be represented in AI systems: Object: All the facts about objects in our world domain. E.g., Guitars contains strings, trumpets are brass instruments. Events: Events are the actions which occur in our world. Performance: It describe behavior which involves knowledge about how to do things. Meta-knowledge: It is knowledge about what we know. Facts: Facts are the truths about the real world and what we represent. Knowledge-Base: The central component of the knowledge-based agents is the knowledge base. It is represented as KB. The Knowledgebase is a group of the Sentences (Here, sentences are used as a technical term and not identical with the English language). TYPES OF KNOWLEDGE 1. Declarative Knowledge: Declarative knowledge is to know about something. It includes concepts, facts, and objects. It is also called descriptive knowledge and expressed in declarativesentences. It is simpler than procedural language. 2. Procedural Knowledge It is also known as imperative knowledge. Procedural knowledge is a type of knowledge which is responsible for knowing how to do something. It can be directly applied to any task. It includes rules, strategies, procedures, agendas, etc. Procedural knowledge depends on the task on which it can be applied. 3. Meta-knowledge: Knowledge about the other types of knowledge is called Meta-knowledge. 4. Heuristic knowledge: Heuristic knowledge is representing knowledge of some experts in a filed or subject. Heuristic knowledge is rules of thumb based on previous experiences, awareness of approaches, and which are good to work but not guaranteed. 5. Structural knowledge: Structural knowledge is basic knowledge to problem-solving. It describes relationships between various concepts such as kind of, part of, and grouping of something. It describes the relationship that exists between concepts or objects. The Relationship Between Knowledge and AI In simplest terms, AI provides the mechanisms that enable machines to “gain knowledge.” It allows them to acquire, process, and use knowledge to perform tasks that display “intelligent” behavior, such as: Perception Learning Knowledge representation and reasoning Planning Execution There are significant differences between knowledge and intelligence. Knowledge is the collection of skills and information a person’s acquired through experience. Knowing how to apply that knowledge to problem-solving and decision-making is intelligence. AI knowledge management is a strategic process where an organization creates value from intangible assets. What Is the Knowledge Base in AI? A great illustration of knowledge-based AI is AI-powered customer service. When finding a solution to a customer’s problem, customer support agents often search multiple sources of information and seek advice from one or more experts. AI simplifies the process by using keywords and phrases to quickly scour dozens, if not hundreds, of various types of information to speedily answer an agent’s question. By placing all information from different siloed teams in a centralized repository, knowledge bases in AI make it incredibly easy for agents to retrieve relevant, useful intelligence. Customers no longer need to be transferred from department to department in search of someone who has the answer to their question because all agents have access to the same accurate and relevant information. fundamental characteristics of an AI-powered knowledge base The fundamental characteristics of an AI-powered knowledge base include: Accurate and Relevant Content. AI extracts insights and patterns from large sets of data and then makes predictions based on that information. It then provides users with real-time and pertinent information that addresses their needs. A Consistent Voice. All agents have access to the same relevant content, which means they’re all working with the same information and providing a consistent customer experience. Faster Service. An AI-powered knowledge base significantly speeds business activities, with customers enjoying faster service from agents. Simplification. Robust doesn’t have to mean complex. Easy-to-navigate dashboards and interfaces make it easy to create and maintain even the most intricate knowledge base. Improved Collaboration. Knowledge base articles are at their best when customers and agents work together. AI gathers feedback and recommendations from agents, customers, and community users to continuously update and improve content. 4 Key Benefits of Knowledge Base in Artificial Intelligence Knowledge management systems boost productivity, minimize risk, and increase your competitive advantage. A knowledge base in AI delivers many important benefits, including: 1. Simplifying Knowledge Discovery. Until a few years ago, organizations only had access to two knowledge management practices: capturing and sharing knowledge. Today’s systems add discovering and maintaining knowledge to the mix. Knowledge workers can now use multiple tools, including cloud-based solutions, to share their knowledge and expertise with others. AI makes the entire process easier with new technologies like semantic search, natural language processing, and machine learning. 2. Connecting Data From Disparate Sources. People working in different departments don’t necessarily capture and share information in the same way. For instance, sales reps may use a CRM tool, and support teams use a ticketing system. AI helps organizations connect and combine knowledge across multiple processes. 3. Keeping Your Knowledge Base Content Up-to-Date. Over time, a tremendous amount of information ends up stored in a knowledge base. Eventually, some of it becomes outdated, but no one bothers to update or dispose of it. AI supports knowledge maintenance by reminding employees to update knowledge regularly. 4. Providing Important Knowledge Management Metrics. It’s challenging to prove a knowledge management system is fulfilling its promise. AI makes it far easier to track performance metrics like first call resolution, average wait time, and average call abandonment rate. Knowledge-Based Agent in Artificial intelligence An intelligent agent needs knowledge about the real world for taking decisions and reasoning to act efficiently. Knowledge-based agents are those agents who have the capability of maintaining an internal state of knowledge, reason over that knowledge, update their knowledge after observations and take actions. These agents can represent the world with some formal representation and act intelligently. Knowledge-based agents are composed of two main parts: o Knowledge-base and o Inference system. A knowledge-based agent must able to do the following: An agent should be able to represent states, actions, etc. An agent Should be able to incorporate new percepts An agent can update the internal representation of the world An agent can deduce the internal representation of the world An agent can deduce appropriate actions. Knowledge base: Knowledge-base is a central component of a knowledge-based agent, it is also known as KB. It is a collection of sentences (here 'sentence' is a technical term and it is not identical to sentence in English). These sentences are expressed in a language which is called a knowledge representation language. The Knowledge-base of KBA stores fact about the world. TECHNIQUES OF KNOWLEDGE REPRESENTATION There are mainly four ways of knowledge representation which are given as follows: 1. Logical Representation Logical representation is a language with some concrete rules which deals with propositions and has no ambiguity in representation. Logical representation means drawing a conclusion based on various conditions. This representation lays down some important communication rules. It consists of precisely defined syntax and semantics which supports the sound inference. Each sentence can be translated into logics using syntax and semantics. Syntax: Syntaxes are the rules which decide how we can construct legal sentences in the logic. It determines which symbol we can use in knowledge representation. How to write those symbols. Semantics: Semantics are the rules by which we can interpret the sentence in the logic. Semantic also involves assigning a meaning to each sentence. Logical representation can be categorised into mainly two logics: 1. Propositional Logics 2. Predicate logics Note: We will discuss Prepositional Logics and Predicate logics in later chapters. Advantages of logical representation: 1. Logical representation enables us to do logical reasoning. 2. Logical representation is the basis for the programming languages. Disadvantages of logical Representation: 1. Logical representations have some restrictions and are challenging to work with. 2. Logical representation technique may not be very natural, and inference may not be so efficient. Note: Do not be confused with logical representation and logical reasoning as logical representation is a representation language and reasoning is a process of thinking logically. 2. Semantic Network Representation Semantic networks are alternative of predicate logic for knowledge representation. In Semantic networks, we can represent our knowledge in the form of graphical networks. This network consists of nodes representing objects and arcs which describe the relationship between those objects. Semantic networks can categorize the object in different forms and can also link those objects. Semantic networks are easy to understand and can be easily extended. This representation consist of mainly two types of relations: 1. IS-A relation (Inheritance) 2. Kind-of-relation Example: Following are some statements which we need to represent in the form of nodes and arcs. Statements: 1. Jerry is a cat. 2. Jerry is a mammal 3. Jerry is owned by Priya. 4. Jerry is brown colored. 5. All Mammals are animal. In the above diagram, we have represented the different type of knowledge in the form of nodes and arcs. Each object is connected with another object by some relation. Drawbacks in Semantic representation: 1. Semantic networks take more computational time at runtime as we need to traverse the complete network tree to answer some questions. It might be possible in the worst case scenario that after traversing the entire tree, we find that the solution does not exist in this network. 2. Semantic networks try to model human-like memory (Which has 1015 neurons and links) to store the information, but in practice, it is not possible to build such a vast semantic network. 3. These types of representations are inadequate as they do not have any equivalent quantifier, e.g., for all, for some, none, etc. 4. Semantic networks do not have any standard definition for the link names. 5. These networks are not intelligent and depend on the creator of the system. Advantages of Semantic network: 1. Semantic networks are a natural representation of knowledge. 2. Semantic networks convey meaning in a transparent manner. 3. These networks are simple and easily understandable. 3. Frame Representation A frame is a record like structure which consists of a collection of attributes and its values to describe an entity in the world. Frames are the AI data structure which divides knowledge into substructures by representing stereotypes situations. It consists of a collection of slots and slot values. These slots may be of any type and sizes. Slots have names and values which are called facets. Facets: The various aspects of a slot is known as Facets. Facets are features of frames which enable us to put constraints on the frames. Example: IF-NEEDED facts are called when data of any particular slot is needed. A frame may consist of any number of slots, and a slot may include any number of facets and facets may have any number of values. A frame is also known as slot-filter knowledge representation in artificial intelligence. Frames are derived from semantic networks and later evolved into our modern-day classes and objects. A single frame is not much useful. Frames system consist of a collection of frames which are connected. In the frame, knowledge about an object or event can be stored together in the knowledge base. The frame is a type of technology which is widely used in various applications including Natural language processing and machine visions. Slots Filter Name Peter Profession Doctor Age 25 Marital status Single Weight 78 Advantages of frame representation: 1. The frame knowledge representation makes the programming easier by grouping the related data. 2. The frame representation is comparably flexible and used by many applications in AI. 3. It is very easy to add slots for new attribute and relations. 4. It is easy to include default data and to search for missing values. 5. Frame representation is easy to understand and visualize. Disadvantages of frame representation: 1. In frame system inference mechanism is not be easily processed. 2. Inference mechanism cannot be smoothly proceeded by frame representation. 3. Frame representation has a much generalized approach. 4. Production Rules Production rules system consist of (condition, action) pairs which mean, "If condition then action". It has mainly three parts: The set of production rules Working Memory The recognize-act-cycle In production rules agent checks for the condition and if the condition exists then production rule fires and corresponding action is carried out. The condition part of the rule determines which rule may be applied to a problem. And the action part carries out the associated problem-solving steps. This complete process is called a recognize-act cycle. The working memory contains the description of the current state of problems-solving and rule can write knowledge to the working memory. This knowledge match and may fire other rules. If there is a new situation (state) generates, then multiple production rules will be fired together, this is called conflict set. In this situation, the agent needs to select a rule from these sets, and it is called a conflict resolution. Propositional Logic Introduction to propositional Logic Propositional logic (PL) is the simplest form of logic where all the statements are made by propositions. A proposition is a declarative statement which is either true or false. It is a technique of knowledge representation in logical and mathematical form. Example 1. a) It is Sunday. 2. b) The Sun rises from West (False proposition) 3. c) 3+3= 7(False proposition) 4. d) 5 is a prime number. Basic facts of propositional logic Propositional logic is also called Boolean logic as it works on 0 and 1. In propositional logic, we use symbolic variables to represent the logic, and we can use any symbol for a representing a proposition, such A, B, C, P, Q, R, etc. Propositions can be either true or false, but it cannot be both. Propositional logic consists of an object, relations or function, and logical connectives. These connectives are also called logical operators. The propositions and connectives are the basic elements of the propositional logic. Connectives can be said as a logical operator which connects two sentences. A proposition formula which is always true is called tautology, and it is also called a valid sentence. A proposition formula which is always false is called Contradiction. Statements which are questions, commands, or opinions are not propositions such as "Where is Rohini", "How are you", "What is your name", are not propositions. Syntax of propositional logic The syntax of propositional logic defines the allowable sentences for the knowledge representation. There are two types of Propositions: 1. Atomic Propositions 2. Compound propositions Atomic Proposition: Atomic propositions are the simple propositions. It consists of a single proposition symbol. These are the sentences which must be either true or false. Example 1. 2+2 is 4, it is an atomic proposition as it is a true fact. 2. "The Sun is cold" is also a proposition as it is a false fact. Compound proposition: Compound propositions are constructed by combining simpler or atomic propositions, using parenthesis and logical connectives. Example: a) "It is raining today, and street is wet." b) "Ankit is a doctor, and his clinic is in Mumbai." Logical Connectives Logical connectives are used to connect two simpler propositions or representing a sentence logically. We can create compound propositions with the help of logical connectives. There are mainly five connectives, which are given as follows: 1. Negation: A sentence such as ¬ P is called negation of P. A literal can be either Positive literal or negative literal. 2. Conjunction: A sentence which has ∧ connective such as, P ∧ Q is called a conjunction. Example: Rohan is intelligent and hardworking. It can be written as, P= Rohan is intelligent, Q= Rohan is hardworking. → P∧ Q. 3. Disjunction: A sentence which has ∨ connective, such as P ∨ Q. is called disjunction, where P and Q are the propositions. Example: "Ritika is a doctor or Engineer", Here P= Ritika is Doctor. Q= Ritika is Doctor, so we can write it as P ∨ Q. 4. Implication: A sentence such as P → Q, is called an implication. Implications are also known as if-then rules. It can be represented as If it is raining, then the street is wet. Let P= It is raining, and Q= Street is wet, so it is represented as P → Q 5. Biconditional: A sentence such as P⇔ Q is a Biconditional sentence, example If I am breathing, then I am alive P= I am breathing, Q= I am alive, it can be represented as P ⇔ Q. Following is the summarized table for Propositional Logic Connectives: Operators Logical equivalence: Logical equivalence is one of the features of propositional logic. Two propositions are said to be logically equivalent if and only if the columns in the truth table are identical to each other. Let's take two propositions A and B, so for logical equivalence, we can write it as A⇔B. In below truth table we can see that column for ¬A∨ B and A→B, are identical hence A is Equivalent to B Properties of Operators: Commutativity: o P∧ Q= Q ∧ P, or o P ∨ Q = Q ∨ P. Associativity: o (P ∧ Q) ∧ R= P ∧ (Q ∧ R), o (P ∨ Q) ∨ R= P ∨ (Q ∨ R) Identity element: o P ∧ True = P, o P ∨ True= True. Distributive: o P∧ (Q ∨ R) = (P ∧ Q) ∨ (P ∧ R). o P ∨ (Q ∧ R) = (P ∨ Q) ∧ (P ∨ R). DE Morgan's Law: o ¬ (P ∧ Q) = (¬P) ∨ (¬Q) o ¬ (P ∨ Q) = (¬ P) ∧ (¬Q). Double-negation elimination: o ¬ (¬P) = P. Limitations of propositional Logic Limitations of propositional logic We cannot represent relations like ALL, some, or none with propositional logic. Example: 1. All the girls are intelligent. 2. Some apples are sweet. Propositional logic has limited expressive power. In propositional logic, we cannot describe statements in terms of their properties or logical relationships. First order predicate Logic In propositional logic, we can only represent the facts, which are either true or false. PL is not sufficient to represent the complex sentences or natural language statements. The propositional logic has very limited expressive power. Consider the following sentence, which we cannot represent using PL logic. "Some humans are intelligent", or "Sachin likes cricket." To represent the above statements, PL logic is not sufficient, so we required some more powerful logic, such as first-order logic. First-Order logic: First-order logic is another way of knowledge representation in artificial intelligence. It is an extension to propositional logic. FOL is sufficiently expressive to represent the natural language statements in a concise way. First-order logic is also known as Predicate logic or First-order predicate logic. First-order logic is a powerful language that develops information about the objects in a more easy way and can also express the relationship between those objects. First-order logic (like natural language) does not only assume that the world contains facts like propositional logic but also assumes the following things in the world: o Objects: A, B, people, numbers, colors, wars, theories, squares, pits, wumpus,...... o Relations: It can be unary relation such as: red, round, is adjacent, or n-any relation such as: the sister of, brother of, has color, comes between o Function: Father of, best friend, third inning of, end of,...... As a natural language, first-order logic also has two main parts: 1. Syntax 2. Semantics Syntax of First-Order logic: The syntax of FOL determines which collection of symbols is a logical expression in first-order logic. The basic syntactic elements of first-order logic are symbols. We write statements in short-hand notation in FOL. Basic Elements of First-order logic: Following are the basic elements of FOL syntax: Constant 1, 2, A, John, Mumbai, cat,.... Variables x, y, z, a, b,.... Predicates Brother, Father, >,.... Function sqrt, LeftLegOf,.... Connectives ∧, ∨, ¬, ⇒, ⇔ Equality == Quantifier ∀, ∃ Atomic sentences: Atomic sentences are the most basic sentences of first-order logic. These sentences are formed from a predicate symbol followed by a parenthesis with a sequence of terms. We can represent atomic sentences as Predicate (term1, term2,......, term n). Example: Ravi and Ajay are brothers: => Brothers(Ravi, Ajay). Chinky is a cat: => cat (Chinky). Complex Sentences: Complex sentences are made by combining atomic sentences using connectives. First-order logic statements can be divided into two parts: Subject: Subject is the main part of the statement. Predicate: A predicate can be defined as a relation, which binds two atoms together in a statement. Consider the statement: "x is an integer.", it consists of two parts, the first part x is the subject of the statement and second part "is an integer," is known as a predicate. Quantifiers in First-order logic: A quantifier is a language element which generates quantification, and quantification specifies the quantity of specimen in the universe of discourse. These are the symbols that permit to determine or identify the range and scope of the variable in the logical expression. There are two types of quantifier: 1. Universal Quantifier, (for all, everyone, everything) 2. Existential quantifier, (for some, at least one). Universal Quantifier: Universal quantifier is a symbol of logical representation, which specifies that the statement within its range is true for everything or every instance of a particular thing. The Universal quantifier is represented by a symbol ∀, which resembles an inverted A. If x is a variable, then ∀x is read as: For all x For each x For every x. Example: All man drink coffee. Let a variable x which refers to a cat so all x can be represented in UOD as below: ∀x man(x) → drink (x, coffee). Existential Quantifier: Existential quantifiers are the type of quantifiers, which express that the statement within its scope is true for at least one instance of something. It is denoted by the logical operator ∃, which resembles as inverted E. When it is used with a predicate variable then it is called as an existential quantifier. It will be read as: There are all x where x is a man who drink coffee. If x is a variable, then existential quantifier will be ∃x or ∃(x). And it will be read as: There exists a 'x.' For some 'x.' For at least one 'x.' Example: Some boys are intelligent. ∃x: boys(x) ∧ intelligent(x) It will be read as: There are some x where x is a boy who is intelligent. Some Examples of FOL using quantifier: 1. All birds fly. In this question the predicate is "fly(bird)." And since there are all birds who fly so it will be represented as follows. ∀x bird(x) →fly(x). 2. Every man respects his parent. In this question, the predicate is "respect(x, y)," where x=man, and y= parent. Since there is every man so will use ∀, and it will be represented as follows: ∀x man(x) → respects (x, parent). 3. Some boys play cricket. In this question, the predicate is "play(x, y)," where x= boys, and y= game. Since there are some boys so we will use ∃, and it will be represented as: ∃x boys(x) → play(x, cricket). 4. Not all students like both Mathematics and Science. In this question, the predicate is "like(x, y)," where x= student, and y= subject. Since there are not all students, so we will use ∀ with negation, so following representation for this: ¬∀ (x) [ student(x) → like(x, Mathematics) ∧ like(x, Science)]. 5. Only one student failed in Mathematics. In this question, the predicate is "failed(x, y)," where x= student, and y= subject. Since there is only one student who failed in Mathematics, so we will use following representation for this: ∃(x) [ student(x) → failed (x, Mathematics) ∧∀ [¬(x==y) ∧ student → ¬failed (x, Mathematics)].