GIS and Remote Sensing for Agriculture: Image Classification PDF
Document Details
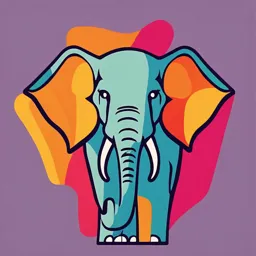
Uploaded by VisionaryLearning393
Universität Kassel
Satya Prakash
Tags
Summary
This document presents a lecture on image classification within the context of Geographic Information Systems (GIS) and remote sensing. It covers unsupervised and supervised classification methods, and processes such as K-means and ISODATA clustering. The document highlights key elements of image interpretation and accuracy assessment.
Full Transcript
Module M.SIA.I14M GIS and Remote Sensing for Agriculture L9: Image Classification Satya Prakash [email protected] GNR/FB 11 Image Classification Image is a continuous data representation The continuous image data n...
Module M.SIA.I14M GIS and Remote Sensing for Agriculture L9: Image Classification Satya Prakash [email protected] GNR/FB 11 Image Classification Image is a continuous data representation The continuous image data needs to be converted to discrete data that represent different interpretable few categories Land use and land cover (LULC) Soil types Crop types Impervious and non- impervious surfaces Elements of Visual Interpretation Location: Where an object is found in an image Tone: Relative brightness or color of objects Shape: The general form, structure, or outline of objects Size: The dimensions of objects; important for distinguishing between different features Pattern: The spatial arrangement of objects; can be random or systematic Texture: Surface characteristics (smooth/rough) Shadow: can provide information about the height and shape of features Association: The relationship between objects; understanding one feature can provide clues about another Domain of classification Spectral domain Categorise a pixel based on its pattern of spectral reflectance data Most common Easy to implement Spectral and spatial domain Addition to the spectral pattern of the pixel, consider the its relationship to neighbouring pixels More complex and difficult to implement Temporal domain Employ changes of the pixel value over time to categorise pixel Computationally intense Currently possible with long term satellite image archives 5 Density slicing - I Convert continuous single band image data into series of intervals or slices Single band images One band multispectral data Vegetation index image (e.g., NDVI) Used when pixel values have a direct relationship to a physical variable Only limited use when classes spectrally similar 6 Density slicing - II 7 Feature space 6 Principles of Remote Sensing, Klaus Tempfli, Normal Kerle, Gerrit Huurneman, Lucas Janssen Methods of classification Unsupervised Supervised Group pixels based on Group pixels based on spectral values using the data from training clustering algorithms data Algorithm examines Based on numerical unknown pixels and put pattern from training them into natural data, unknown pixels groups present in the put into the respective image class No knowledge about “Class Label” 9 Unsupervised Classification 10 Unsupervised classification Assumes no prior knowledge Clustering of image pixels based on natural grouping of pixels within the images When to apply? Difficult to collect ground/reference data Classify images from unknow regions Advantages Fast method Easy to apply Human error is reduced Algorithms are available in every image analysing software Disadvantages Limited control over the process Class labels may not represent physical meaning 11 K-means clustering - I Assign random class centra Compute the spectral distance (Euclidian distance) from each centre to each pixel Assign class membership to each according nearest class midpoint Compute new class centra for each group of pixels belonging to the same class Repeat last two steps until the centra no longer change (iterative optimization) 12 K-means clustering - II https://stanford.edu/~cpiech/cs221/handouts/kmeans.html 13 K-means clustering - III User has to define number classes (K) Difficult to define number of classes in advance Classes have to be merged, divided manually Correct informative class labels are assigned afterwards 14 ISODATA clustering Iterative Self-Organising Data Analysing Technique Similar procedure as K-Means clustering After each iteration; Merging: if two clusters are closer than defined threshold Splitting: if clusters are too large (based on standard deviation) Deletion: if clusters do not have enough member than defined threshold Thresholds have to be defined before start the clustering procedure 15 Supervised Classification 16 Supervised classification – I Lillesand, T. M., Kiefer, R. W., & Chipman, J. W. (2003). Remote sensing and image interpretation (Fifth Edit). Wiley. 17 Supervised classification – II Define training areas Representative Many Large Avoid mixed areas Remove excessive wavebands (if many bands are available) Apply multi-image enhancement techniques Create ”signature” for each class Min, max, mean, variance, covariance Classify Compare every pixel with class signature and assign a class to each pixel Evaluate classification accuracy Against training areas Against independent samples Redo classification until accuracy is satisfactory 18 Parallelepiped (Box) classifier Create “Boxes” in feature space for each class based on min and max from each band Every pixel is checked which box contains that pixel If a pixel falls into a box, then the pixel will get the class label as box it belongs Pixels that not belongs to any box will remain as unclassified pixels Pros Fast Assumes no statistical distribution Small samples are enough Cons Overlapping classes Undefined areas between classes Lillesand, T. M., Kiefer, R. W., & Chipman, J. W. (2003). Remote sensing and image interpretation (Fifth Edit). Wiley. 19 Minimum Distance to Mean (MDM) classifier Class centra is defined based on class mean Distance from each pixel to each class mean is computed The pixel is assigned class membership to the spectrally closest class (minimum distance) Pros Fast Small training samples are enough All pixels are classified Cons Does not consider the variability of the classes Lillesand, T. M., Kiefer, R. W., & Chipman, J. W. (2003). Remote sensing and image interpretation (Fifth Edit). Wiley. 20 Maximum Likelihood (MLH) classifier Widely used in supervised classification Not only consider the class centra, But also the shape, size, and orientation of the cluster based on statistical distance calculated from the mean value and covariance matrix of the cluster The distance is probability value A pixel is assigned to the class to which it has the highest probability Assumes classes follow a normal (Gaussian) distribution Pros Considers statistical distribution and covariance of classes Takes prior probably into account Cons Large number of samples are needed Lillesand, T. M., Kiefer, R. W., & Chipman, J. W. (2003). Remote sensing and image interpretation (Fifth Edit). Computational intensive Wiley. 21 Machine Learning Classifiers Random Forest Support Vector Machine Artificial Neural Network 22 Case study: Bengaluru 23 Source: GNR/FB11 24 Source: GNR/FB11 25 Source: GNR/FB11 Accuracy Assessment 26 How accurate the classification Classification is incomplete unless its accuracy has been assessed Visual evaluation Is pattern realistic? Any artifacts? Evaluating how the training areas were classified Statistical evaluation of the final map against an independent samples Spatial pattern of the samples should be representative Sources of errors Geometric errors Wrong labelling Incorrect atmospheric correction Undistinguishable classes Non-perfect classification method 27 Error (Confusion) Matrix (Contingency Table) Error matrix is a square matrix (n by n) with number of rows and columns equals to number of classes It compares, on a category by category basis, the relationship between known reference data and corresponding classification results Different accuracy metrics are calculated Overall accuracy Commission and omission error per class User and producer accuracy per class Kappa coefficient 28 Error (Confusion) Matrix (Contingency Table) 29 Overall Accuracy (OA) http://gis.humboldt.edu/OLM/Courses/GSP_216_Online/lesson6-2/error-matrix.html 𝑇𝑜𝑡𝑎𝑙 𝑛𝑢𝑚𝑏𝑒𝑟 𝑜𝑓 𝑐𝑜𝑟𝑟𝑒𝑐𝑡𝑙𝑦 𝑐𝑙𝑎𝑠𝑠𝑖𝑓𝑒𝑑 𝑝𝑥𝑒𝑙𝑠 𝑂𝐴 = × 100 𝑇𝑜𝑡𝑎𝑙 𝑛𝑢𝑚𝑏𝑒𝑟 𝑜𝑓 𝑝𝑖𝑥𝑒𝑙𝑠 (21+31+22) 𝑂𝐴 = × 100 = 77.9 % 95 30 Commission and Omission errors Commission error Omission error Related to incorrectly classified pixels in each Related to missed out pixel in each class class From actual water pixels, 5 were classified as 6 Forest pixels and 0 Urban pixels were Forest and 7 were classified as Urban classified as Water Total water pixels in reference data is 33 Total water pixels in classified result is 27 Omission error of water is 12/33 = 36 % Commission error of water is 6/27 = 22 % 31 User’s and Producer’s accuracy User’s accuracy Producer’s accuracy Accuracy from the point of view of map user Accuracy from the point of view of map producer Probability of an unknown pixel on the map of being correctly classified Probability of an unknown pixel in the field of being correctly classified Correctly classified water pixels are 21 Correctly classified water pixels are 21 Total water pixels in classified result is 27 Total water pixels in reference data is 33 User’s accuracy of water is 21/27 = 78 % Omission error of water is 21/33 = 64 % 32 Kappa Coefficient A measure to evaluate classification result compares to a random distribution of each category Simply, determine whether a classification is better than a chance 𝑁𝐴 − 𝐵 𝐾𝑎𝑝𝑝𝑎 𝐾 = 𝑁2 − 𝐵 A = sum of diagonal elements B = sum of the product (row total x column total for each class) N = total number of elements Kappa coefficient varies between -1 and +1 0 means there is no agreement 1 means a strong agreement between reference and classified data Usually above 0.75 indicates a good classification results 33 Kappa Coefficient http://gis.humboldt.edu/OLM/Courses/GSP_216_Online/lesson6-2/error-matrix.html A = 21 + 31 + 22 = 74 B = (27*33) + (37*39) + (31*23) = 891 + 1443 + 713 = 3047 N = 95 Kappa = (NA – B) / (N² – B) Kappa = ((95*74) – 3047) / (95^2 - 3047) = 3983/5978 = 0.67 34 Summary Classification can be categorised into unsupervised and supervised procedures Unsupervised classification does not require a prior knowledge about potential classes, however further information is needed for interpretation of classification results For supervised classification a priori knowledge is used to identify areas for training the classifier Training areas should be selected in a way that they represent spectral variation within the selection land cover class Classification is one of the major processes in remote sensing Evaluating classification accuracy is necessary to make resulting classification image applicable by others 35