Health Informatics: Medical Decision Making PDF
Document Details
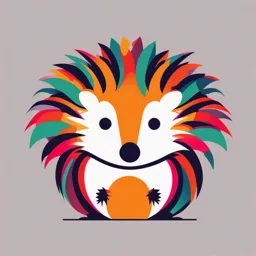
Uploaded by DivineHummingbird2604
Özgür Tosun
Tags
Summary
These lecture notes cover health informatics with a focus on medical decision making. Topics include an introduction to medical decision making, evidence-based medicine, the role of AI and machine learning, patient-centered decision making and case studies. The resource is authored by Özgür Tosun.
Full Transcript
Associate Prof. Dr. Özgür Tosun Health Informatics Medical Decision Making Introduction to Types of Medical Medical Decision Decisions Making Artificial Intelligence Co...
Associate Prof. Dr. Özgür Tosun Health Informatics Medical Decision Making Introduction to Types of Medical Medical Decision Decisions Making Artificial Intelligence Course Evidence-Based and Machine Medicine (EBM) Learning in Medical Outline Decision Making Patient-Centered Case studies Decision Making Definition Introduction to Medical Decision Making Medical decision making refers to the process of choosing among alternative courses of action in the management of a patient's health condition. This process involves evaluating the available evidence, considering potential risks and benefits, integrating patient preferences, and using clinical experience to make informed decisions. Significance Medical decision making is crucial to the Introduction delivery of effective, patient-centered care. to Medical Decision It has a direct impact on patient outcomes, Making quality of care, and resource allocation. Developing and improving decision-making skills are essential for healthcare professionals to optimize patient care and ensure evidence-based practice. Role of Medical Informatics in Decision Making Introduction to Medical Decision Making Medical informatics is the interdisciplinary field that focuses on the effective use of information and technology in healthcare. It plays a vital role in medical decision making by: Role of Medical Informatics in Decision Making 1.Facilitating access to relevant and up-to-date clinical information (e.g., electronic health Introduction records, clinical guidelines, research articles). 2.Developing and implementing clinical decision to Medical support systems (CDSS) to assist healthcare professionals in making informed decisions. Decision 3.Utilizing artificial intelligence (AI) and machine Making learning (ML) algorithms to analyze large datasets, identify patterns, and support diagnostic, therapeutic, and prognostic decisions. 4.Providing tools and methods for analyzing, visualizing, and communicating medical data to support collaborative decision making among healthcare professionals and between providers and patients. The Decision-Making Process in Healthcare The medical decision-making process Introduction typically follows these steps: to Medical Gathering and organizing relevant clinical Decision data (e.g., medical history, physical examination findings, diagnostic test results). Making Identifying the patient's problem(s) or clinical question(s) that need to be addressed. Generating a list of possible diagnoses, interventions, or management strategies. Evaluating the available evidence related to each option, including the risks, benefits, and likelihood of success. The Decision-Making Process in Healthcare The medical decision-making process Introduction typically follows these steps: to Medical Incorporating patient preferences, values, and unique circumstances into the decision-making Decision process. Selecting the most appropriate course of action Making based on the available evidence, clinical experience, and patient preferences. Implementing the chosen intervention or management plan and monitoring its effectiveness. Continuously updating and revising decisions based on new information, patient response, and clinical outcomes. Types of Medical Decisions Diagnostic decisions Therapeutic decisions Prognostic decisions Diagnostic Decisions Diagnostic decisions involve determining the cause of a patient's signs, symptoms, or clinical findings. This process typically involves the following steps: Collecting relevant clinical information: Healthcare professionals gather information through patient history, physical examination, and diagnostic tests. Formulating a differential diagnosis: Based on the collected information, a list of possible diagnoses is generated. Refining the differential diagnosis: Additional tests or consultations may be needed to narrow down the list of potential diagnoses. Establishing a definitive diagnosis: The healthcare professional arrives at a final diagnosis, which serves as the basis for further management decisions. Diagnostic Decisions Example: A patient presents with fever, headache, and neck stiffness. The healthcare provider collects a detailed history, performs a physical examination, and orders initial tests like blood work and a lumbar puncture. Based on the findings, the differential diagnosis may include bacterial meningitis, viral meningitis, or another central nervous system infection. Additional testing and clinical data help the provider arrive at a definitive diagnosis, such as bacterial meningitis, which will guide the therapeutic decisions. Diagnostic Decisions-How medical Informatics Assist Decision Making for the Previous Example Diagnostic Decisions (Bacterial Meningitis Example) Electronic Health Records (EHRs): EHRs can help healthcare providers access a patient's medical history, previous test results, and other relevant information, aiding in the formulation of a differential diagnosis. Clinical Decision Support Systems (CDSS): CDSS can provide evidence-based guidance and diagnostic algorithms, helping clinicians consider a broad range of potential diagnoses and prioritize the most likely ones. Artificial Intelligence (AI) and Machine Learning (ML): AI and ML can be used to analyze large datasets, identify patterns, and develop predictive models for specific diseases or conditions, assisting with diagnostic decision-making. Telemedicine and Remote Consultations: Telemedicine can facilitate consultations with specialists or other healthcare professionals, allowing for quicker, more accurate diagnostic decisions. Therapeutic Decisions Therapeutic decisions involve selecting the most appropriate treatment or intervention for a patient's condition. This process includes: Identifying potential treatment options: Based on the diagnosis, healthcare professionals consider various interventions, which may include medications, surgery, or lifestyle modifications. Evaluating the risks and benefits of each option: Providers weigh the potential benefits of each treatment against the risks and side effects, taking into account the available evidence. Incorporating patient preferences and values: Patients' values, preferences, and unique circumstances are considered when choosing among treatment options. Implementing the chosen treatment plan: The selected intervention is initiated, and the patient's response is monitored. Therapeutic Decisions Example: A patient diagnosed with type 2 diabetes needs to make therapeutic decisions about their treatment plan. The healthcare provider and patient discuss various options, such as lifestyle modifications (diet and exercise), oral hypoglycemic medications, or insulin therapy. They consider the risks and benefits of each option, taking into account the patient's preferences and individual circumstances. The chosen treatment plan may include a combination of lifestyle changes and oral medications, which will be monitored for effectiveness and adjusted as needed. Diagnostic Decisions-How medical Informatics Assist Decision Making for the Previous Example Therapeutic Decisions (Type 2 Diabetes Example) Evidence-Based Medicine (EBM) resources: Medical informatics can provide healthcare providers with easy access to up-to-date clinical guidelines, research articles, and other EBM resources, helping them make informed therapeutic decisions. Clinical Decision Support Systems (CDSS): CDSS can offer personalized treatment recommendations based on a patient's unique characteristics, such as age, comorbidities, and medication history, as well as provide alerts for potential drug interactions or contraindications. Patient Education and Engagement Tools: Medical informatics can provide tools and resources to help patients better understand their condition and treatment options, promoting shared decision-making and improved adherence to therapy. Remote Monitoring and Telehealth: Telehealth technology can enable healthcare providers to monitor patients' progress, adjust treatments as needed, and provide ongoing support to improve therapeutic outcomes. Prognostic Decisions Prognostic decisions involve predicting the likely course and outcome of a patient's condition, considering factors such as disease progression, potential complications, and the patient's overall health. This process includes: Gathering relevant clinical data: Healthcare professionals use diagnostic test results, clinical findings, and patient history to assess a patient's condition. Assessing disease progression and potential complications: Providers evaluate the natural course of the disease and the likelihood of complications or comorbidities. Estimating the patient's prognosis: Based on the gathered data, healthcare professionals make predictions about the patient's likely clinical outcomes and survival. Communicating prognostic information to the patient: Providers share the prognosis with the patient, which may inform further therapeutic or advance care planning decisions. Prognostic Decisions Example: A patient is diagnosed with advanced-stage lung cancer. The healthcare team gathers clinical data, such as the tumor stage, histology, and presence of metastases, to assess the patient's condition. They estimate the patient's prognosis based on this information and discuss the findings with the patient. Prognostic Decisions-How medical Informatics Assist Decision Making for the Previous Example Prognostic Decisions (Advanced-Stage Lung Cancer Example) Clinical Data Repositories and Registries: Access to large databases of patient outcomes can help healthcare professionals identify factors that influence prognosis, facilitating more accurate prognostic estimates. AI and ML Algorithms: AI and ML can analyze complex datasets and develop predictive models to estimate a patient's prognosis based on specific clinical factors. Clinical Decision Support Systems (CDSS): CDSS can integrate prognostic information with other clinical data, such as treatment options and potential side effects, to help healthcare providers make more informed decisions about patient care. Communication and Visualization Tools: Medical informatics can provide tools to help healthcare professionals effectively communicate prognostic information to patients, including visual aids and interactive decision aids that facilitate shared decision-making. Definition and Principles EBM is the conscientious, explicit, and judicious use of current best evidence in making decisions about the care of individual patients. The practice of EBM involves integrating Evidence- individual clinical expertise with the best available external clinical evidence from Based systematic research and considering patient values and preferences. Medicine The key principles of EBM include (EBM) i. ii. Asking clear, focused clinical questions. Searching for the best available evidence. iii. Critically appraising the evidence for its validity, importance, and applicability. iv. Applying the evidence to individual patient care and integrating it with clinical expertise and patient preferences. Importance of EBM in Medical Decision Making EBM improves the quality and consistency of healthcare decisions by ensuring that they are based on the Evidence- best available evidence. Practicing EBM can lead to better Based patient outcomes, reduced healthcare costs, and more efficient use of Medicine resources. (EBM) EBM helps healthcare professionals stay up-to-date with the latest research and advances in their field, fostering continuous learning and professional development. The Hierarchy of Evidence The hierarchy of evidence is a ranking system used to evaluate the strength and quality of clinical research studies. The hierarchy typically includes the following levels (from highest to lowest quality): Evidence- 1. Systematic reviews and meta-analyses 2. Randomized controlled trials (RCTs) Based 3. Cohort studies 4. Case-control studies Medicine 5. Cross-sectional studies 6. Case series and case reports (EBM) 7. Expert opinion, consensus statements, and guidelines Higher levels of evidence provide stronger support for medical decision making, but the relevance and applicability of the evidence to a specific patient situation must also be considered. Steps in Practicing EBM To practice EBM effectively, healthcare professionals should follow these steps: 1) Formulating clinical questions (using the PICO format: Patient, Intervention, Evidence- Comparison, Outcome) 2) Finding the best available evidence (using databases such as PubMed, Cochrane Based Library, or guidelines from professional organizations) Medicine 3) Appraising the evidence for its validity, importance, and applicability (considering (EBM) study design, risk of bias, and relevance to the patient population) 4) Applying the evidence to clinical practice (integrating the evidence with clinical expertise and patient preferences to make informed medical decisions) 1- Introduction to the Clinical Scenario: A 55-year-old patient with newly Evidence- diagnosed hypertension visits Based your clinic. The patient has no other comorbidities and no Medicine known history of cardiovascular (EBM)-An disease. Your goal is to determine the most appropriate Example initial antihypertensive medication for this patient. 2- Formulating a Clinical Question: Using the PICO format, you can frame your clinical question as follows: 1. P (Patient): 55-year-old patient with newly diagnosed hypertension and no other Evidence- comorbidities 2. I (Intervention): Initial antihypertensive medication (e.g., thiazide diuretic) Based 3. C (Comparison): Other classes of antihypertensive medications (e.g., ACE Medicine inhibitors, beta-blockers, calcium channel blockers) 4. O (Outcome): Blood pressure control, (EBM)-An reduction in cardiovascular events, and side effect profile Example The clinical question could be: "In a 55-year-old patient with newly diagnosed hypertension and no other comorbidities, which class of antihypertensive medication is most effective for blood pressure control, reducing cardiovascular events, and minimizing side effects?" 3- Finding the Best Available Evidence: Search databases such as PubMed or the Cochrane Library using relevant Evidence- keywords, such as "hypertension," Based "antihypertensive medication," "treatment," "blood pressure control," Medicine and "cardiovascular events." Identify systematic reviews, meta- (EBM)-An analyses, or high-quality RCTs comparing the efficacy and safety of Example different antihypertensive medication classes in patients with hypertension. 4- Appraising the Evidence: Review the identified studies, assessing their quality and relevance: Study design: Are the studies systematic reviews, meta-analyses, or RCTs? Evidence- Risk of bias: Do the studies have a low risk of bias (e.g., adequate Based randomization, blinding, and low attrition)? Relevance to the patient population: Do Medicine the studies include patients similar to your 55-year-old patient with (EBM)-An hypertension and no other comorbidities? Example Synthesize the findings from the appraised studies, focusing on blood pressure control, reduction in cardiovascular events, and side effect profiles of different antihypertensive medication classes. 5- Applying the Evidence to Clinical Practice: Based on the appraised evidence, identify the antihypertensive medication class that appears most effective and safe for your Evidence- patient (e.g., thiazide diuretics, ACE inhibitors, or calcium channel blockers). Based Consider the patient's preferences, values, and unique circumstances (e.g., cost, dosing Medicine frequency, potential side effects) when selecting the specific medication. (EBM)-An Initiate the chosen antihypertensive medication and monitor the patient's blood Example pressure and response to treatment, adjusting the medication as needed based on clinical outcomes and any new evidence that emerges. 1- Develop a habit of asking clinical questions Integrating Encourage yourself and your colleagues to formulate clear, focused clinical questions Evidence- when encountering diagnostic, therapeutic, or prognostic dilemmas. This will help guide your Based search for relevant evidence and ensure that your decisions are informed by the latest Medicine research. into Daily 2- Establish efficient search strategies Become familiar with databases such as Clinical PubMed, Cochrane Library, and guideline repositories to search for the best available Practice and evidence. Bookmark frequently used resources and learn how to use search filters Decision and advanced search features to find high- Making quality studies efficiently. 3- Develop critical appraisal skills Acquire the skills to assess the validity, Integrating importance, and applicability of research Evidence- studies. This may involve attending workshops, participating in journal clubs, or Based using online resources to enhance your understanding of research methods and study Medicine designs. 4- Utilize Clinical Decision Support Systems (CDSS) into Daily and EBM tools Clinical Incorporate CDSS and EBM tools into your practice to facilitate access to evidence-based Practice and guidelines, clinical prediction rules, and risk calculators. These resources can help you Decision make more informed decisions and provide personalized care based on the best available Making evidence. 5- Engage in continuous learning and professional development Integrating Dedicate time for staying current with research in your field by regularly reading Evidence- clinical journals, attending conferences, Based or participating in online courses or webinars. This will help you keep up with Medicine new developments and ensure that your practice remains evidence-based. into Daily 6- Collaborate with colleagues and specialists Clinical Engage in discussions with colleagues and Practice and consult with specialists when appropriate. Sharing knowledge and Decision experiences can help broaden your perspective and improve your ability to Making critically appraise and apply evidence. 7- Encourage patient involvement and shared decision-making Integrating Involve patients in the decision-making process by discussing the available Evidence- evidence, risks, benefits, and potential outcomes of different treatment options. Based This approach fosters shared decision- Medicine making and ensures that patient values and preferences are considered. into Daily 8- Reflect on clinical outcomes and learn from Clinical experience Routinely evaluate the outcomes of your Practice and clinical decisions and reflect on how well the evidence-based approach has Decision worked. Use these insights to refine your Making decision-making process and improve your practice. Artificial Artificial Intelligence (AI) and Intelligence Machine Learning (ML) are (AI) and Machine playing an increasingly Learning important role in medical (ML) in decision making, offering the Medical potential to enhance diagnostic Decision accuracy, optimize treatment Making selection, and improve patient outcomes. 1- AI and ML in Diagnostic Decisions AI algorithms can analyze medical images, such as X-rays, MRIs, and CT scans, to detect patterns Artificial indicative of specific diseases or conditions. For example, AI-based systems have been developed Intelligence to identify lung nodules suggestive of cancer in (AI) and chest CT scans, with accuracy levels comparable Machine to or exceeding those of human radiologists. Learning ML models can help predict the likelihood of certain diseases based on electronic health record (ML) in (EHR) data, including demographic information, Medical laboratory results, and medical history. For Decision instance, AI systems have been developed to predict the risk of developing diabetes, Making cardiovascular disease, or other chronic conditions, enabling early intervention and prevention efforts. 2- AI and ML in Therapeutic Decisions AI can be used to personalize treatment plans by analyzing large datasets of patient outcomes and identifying the most effective treatments for specific patient subgroups. For example, AI-driven precision oncology platforms have been developed to match Artificial cancer patients with targeted therapies Intelligence (AI) based on their tumor's genetic profile, improving treatment efficacy and reducing and Machine side effects. Learning (ML) in ML algorithms can optimize drug dosing, taking into account factors such as age, Medical Decision weight, organ function, and genetic makeup, Making to minimize the risk of adverse reactions and maximize therapeutic benefits. An example is the use of AI in anticoagulation management, where ML models can predict the optimal warfarin dose for individual patients, reducing the risk of bleeding complications. 3- AI and ML in Prognostic Decisions AI can help predict patient outcomes and identify individuals at high risk of adverse Artificial events, such as hospital readmissions, complications, or mortality. For example, Intelligence ML algorithms have been developed to (AI) and predict the risk of sepsis in ICU patients, allowing for early intervention and Machine potentially reducing morbidity and Learning mortality rates. (ML) in AI can also be used to develop personalized prognostic models for Medical specific diseases, enabling more accurate Decision estimates of disease progression, treatment response, and survival. For Making instance, AI systems have been developed to predict cancer patients' response to immunotherapy based on their tumor's genetic and immune features, facilitating more informed decisions about treatment strategies. Patient-centered decision making refers to the process of involving patients in their Patient- healthcare decisions, ensuring that their values, preferences, Centered and personal circumstances are taken into account. This Medical approach often involves shared decision making, where Decision healthcare professionals and patients collaborate to make Making informed choices about diagnostic and treatment options. Patient-Centered Decision Making This approach recognizes that patients are experts in their own lives and values, and Patient- that their input is essential for making healthcare Centered decisions that align with their goals and preferences. Medical Shared decision making involves a two-way exchange Decision of information, where healthcare professionals Making provide evidence-based recommendations, and patients express their preferences, concerns, and expectations. Incorporating Patient Preferences and Values To incorporate patient preferences and values in Patient- medical decisions, healthcare professionals should: Encourage patients to Centered express their concerns, values, and expectations. Present evidence-based Medical information on the benefits, risks, and Decision potential outcomes of different options. Provide decision aids or Making other tools to help patients understand the implications of their choices. Be empathetic and supportive, acknowledging patients' emotions and validating their feelings. Strategies to Improve Patient-Provider Communication and Facilitate Shared Decision Making Establish rapport: Create a comfortable environment for open Patient- communication by being attentive, approachable, and respectful. Use plain language: Communicate Centered complex medical information in a way that patients can understand, avoiding jargon and technical terms. Medical Active listening: Show genuine interest in the patient's concerns and preferences, using verbal and Decision nonverbal cues to demonstrate your engagement. Making Ask open-ended questions: Encourage patients to share their thoughts and feelings by asking questions that invite elaboration and reflection. Validate emotions: Acknowledge the patient's emotions and provide reassurance, empathy, and support. Strategies to Improve Patient-Provider Communication and Facilitate Shared Decision Making Elicit patient preferences: Ask patients about their priorities, Patient- values, and goals, and explore how these factors might influence their healthcare decisions. Centered Provide decision aids: Utilize tools such as brochures, videos, or Medical online resources to help patients understand the potential outcomes, benefits, and risks of Decision different options. Encourage questions and discussion: Invite patients to ask Making questions, express their concerns, and engage in a collaborative discussion about their healthcare choices. Confirm understanding: Ensure that patients have a clear understanding of the information provided and the implications of their decisions. Real-Life Example Scenario A 60-year-old patient is diagnosed with localized prostate cancer. The healthcare professional discusses Patient- the available treatment options, which include active surveillance, surgery, and radiation therapy. Centered Each option has potential benefits and risks, and the best choice is Medical also depended on the patient's priorities and preferences. The healthcare professional Decision provides information on the expected outcomes, side effects, Making and recovery times for each option, and the patient shares his concerns about preserving sexual function and maintaining a good quality of life. Together, they decide that active surveillance is the most suitable approach for the patient, given his priorities and the specifics of his cancer. Medical informatics can play a significant role in supporting patient-centered decision making in the case of the 60-year-old patient with prostate cancer. 1- Electronic Health Records (EHRs) EHRs can provide healthcare professionals Patient- with a comprehensive overview of the patient's medical history, laboratory results, and imaging studies, helping them assess the Centered patient's overall health and suitability for various treatment options. 2- Clinical Decision Support Systems (CDSS) Medical CDSS can help healthcare professionals access evidence-based guidelines and recommendations for prostate cancer Decision management, ensuring that they are considering the latest research when discussing treatment options with the patient. Making 3- Patient Education and Decision Aids Medical informatics tools can offer tailored patient education materials and decision aids, such as interactive modules or videos, that provide clear and accessible information on prostate cancer and its treatment options. These resources can help the patient better understand their condition and the potential benefits and risks associated with each treatment option. Medical informatics can play a significant role in supporting patient-centered decision making in the case of the 60-year- old patient with prostate cancer. 4- Risk Prediction Models Patient- ML algorithms can analyze the patient's clinical data to generate Centered personalized risk predictions for cancer progression or the likelihood of treatment success. This Medical information can help the healthcare professional, and patient make more informed decisions about the most appropriate treatment approach. Decision 5- Telemedicine and Remote Consultations Medical informatics can facilitate Making telemedicine and remote consultations, enabling the patient to seek a second opinion from a specialist or discuss their treatment options with their healthcare professional from the comfort of their home. Medical informatics can play a significant role in supporting patient-centered decision making in the case of the 60-year- old patient with prostate cancer. Patient- 6- Patient-Reported Outcome Measures (PROMs) Medical informatics systems can be Centered used to collect and analyze patient- reported outcome measures, which Medical can provide valuable insights into the real-world impact of different treatment options on patients' Decision quality of life, functional status, and symptom burden. Making 7- Online Support Communities Medical informatics can help connect the patient with online support communities where they can share their experiences, learn from others who have faced similar decisions, and receive emotional support during their decision-making process. Medical informatics can play a significant role in supporting patient- centered decision making in the case of the 60-year-old patient with prostate cancer. Patient- By incorporating medical informatics into the decision- Centered making process for the 60-year- old patient with prostate cancer, healthcare professionals can Medical provide more personalized, evidence-based, and patient- centered care. Medical Decision informatics tools can help both the healthcare professional and Making the patient access relevant information, assess risks, and make more informed decisions about the most suitable treatment approach. Case Study 1: Predicting Sepsis in ICU Patients In this case study, a hospital has implemented a Machine Learning (ML) algorithm to predict sepsis in ICU patients, based on Electronic Health Record (EHR) data. The Case algorithm continuously analyzes patients' vital signs, laboratory results, and clinical notes to identify Study 1 early signs of sepsis. When a patient is identified as high-risk, the healthcare team receives an alert and can quickly initiate appropriate interventions. This real-time prediction system has the potential to reduce morbidity and mortality rates by enabling early detection and treatment of sepsis. Case Study 2: Personalized Diabetes Management A primary care physician is treating a patient with type 2 diabetes. To optimize the patient's blood glucose control and minimize the risk of complications, the physician uses a Clinical Decision Case Support System (CDSS) that incorporates AI-driven analytics. The CDSS analyzes the patient's demographic information, Study 2 medical history, and laboratory results to recommend personalized treatment goals and management strategies. This includes medication adjustments, lifestyle changes, and monitoring plans tailored to the patient's unique needs and preferences. By utilizing medical informatics, the physician can provide more personalized and effective care to their patient. Case Study 3: Telemedicine for Rural Patients with Chronic Conditions A rural patient with multiple chronic conditions, such as hypertension and heart failure, faces challenges accessing specialized care due to distance and limited resources. Their healthcare provider uses telemedicine and remote Case monitoring technologies to manage the patient's conditions more effectively. The patient's vital signs, symptoms, and Study 3 medication adherence are monitored remotely using connected devices, and the data is transmitted to the healthcare provider for analysis. The healthcare provider can then adjust treatment plans based on this information and communicate with the patient via video consultations. Medical informatics enables better management of the patient's conditions, reduces the need for travel, and improves access to care.