Stochastic Processes Lecture 10 PDF
Document Details
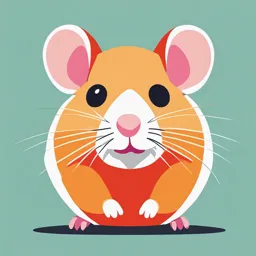
Uploaded by RenewedSerpentine1935
UET Peshawar
Dr. Safdar Nawaz Khan Marwat
Tags
Summary
This document is a lecture on stochastic processes, specifically focusing on queuing networks and closed networks. It details methods for analyzing interconnected queue-based service stations, including open and closed system models.
Full Transcript
Stochastic Processes CSE-5605 Dr. Safdar Nawaz Khan Marwat DCSE, UET Peshawar Lecture 10 [email protected] Queuing Networks Interconnected queue-based service stations Open system (arrivals and departures) Closed system (no arrivals and departures)...
Stochastic Processes CSE-5605 Dr. Safdar Nawaz Khan Marwat DCSE, UET Peshawar Lecture 10 [email protected] Queuing Networks Interconnected queue-based service stations Open system (arrivals and departures) Closed system (no arrivals and departures) Source: Prof. C. Görg, Communication Networks II, University of Bremen [email protected] 2 Closed Network Gordon and Newell showed in 1967 ❑ Product form solution can be extended for closed networks ❑ All assumptions relevant to Jackson networks hold ❑ Number of jobs in network is constant N i =1 k i = K Arrival rate at station i (throughput) ❑ With no external arrivals i = j =1 j p ji N Settings ❑ Short circuit source and sink ❑ Exactly K circulating jobs ❑ No queue needs more than K places Source: Prof. C. Görg, Communication Networks II, University of Bremen [email protected] 3 Closed Network (cont.) N queues and k jobs k can be greater than n Make a table of x’s and /’s ❑ E.g. the number of queues, N = 4 and jobs, k = 5 Queue 1 Queue 2 Queue 3 Queue 4 xx / / x / xx In summary, xx//x/xx N - 1 /’s and K x’s So the number of different arrangements would be 𝑁−1+𝐾 𝑁−1+𝐾 = 𝐾 𝑁−1 [email protected] 4 Closed Network (cont.) Three balls placed in an urn are labeled as 1, 2 and 3. Five draws are performed in such a way that ball is placed back in the urn after each draw. Find the number of possible outcomes of this random experiment. [email protected] 5 Closed Network (cont.) Open network can be treated as closed ❑ By taking arrival rate such that number of jobs in network remain K Number of different possible states ❑ Equal to number of possibilities to distribute K jobs among N stations 𝑁+𝐾−1 𝑛𝑠 = 𝑁−1 As ei = λi / λ, therefore ei given as N ei = e j p ji j =1 ❑ where ei denotes mean number of visits made to ith station ❑ εi denotes service rate of server at ith station Source: Prof. C. Görg, Communication Networks II, University of Bremen [email protected] 6 Closed Network (cont.) According to Gordon and Newell ❑ Stationary distribution at equilibrium has unique solution N ki p(k , k ,..., k ) = 1 x 1 2 N G( K ) b (k i =1 i i ) i where xi = ei / εi G(K) is normalization constant ❑ All state probabilities should sum up to 1 N ki G (K ) = xi i =1 bi (k i ) i=1 i N k = K Number of jobs in individual stations are inter-dependent Source: Prof. C. Görg, Communication Networks II, University of Bremen [email protected] 7 Closed Network (cont.) Help function bi(ki) is defines as k i ! ki ni bi (0) = 1 bi (ki ) = ni !ni ki − ni ki ni 1 ni = 1 For ni = 1, solution simplifies to N G (K ) = x ki i i=1 ki = K i =1 N Source: Prof. C. Görg, Communication Networks II, University of Bremen [email protected] 8 Example N = 3, K = 3 and ni = 1 ❑ Full mesh Transition probability matrix 0.6 0.3 0.1 P = 0.2 0.3 0.5 0.4 0.1 0.5 μ1 = 0.8/s, μ2 = 0.6/s, μ3 = 0.4/s, (εi = μi due to single server) Source: Prof. C. Görg, Communication Networks II, University of Bremen [email protected] 9 Example (cont.) Solution for stationary distribution at equilibrium 1 3 ki p(k1 , k 2 , k3 ) = xi G (3) i =1 where 3 G (3) = x ki i k1 + k 2 + k3 =3 i =1 For relative visit frequency e1 = e1 p11 + e2 p21 + e3 p31 e2 = e1 p12 + e2 p22 + e3 p32 e3 = e1 p13 + e2 p23 + e3 p33 Normalize every ei wrt reference station (1 in our case) ❑ Thus e1 = 1 whereas e2 = 0.533, e3 = 0.733 Source: Prof. C. Görg, Communication Networks II, University of Bremen [email protected] 10 Example (cont.) Determination of various xi ki x1 = 1; x = 1.25; x1 = 1.5625; x = 1.953; 0 1 2 3 1 1 x2 = 1; x = 0.889; x2 = 0.790; x = 0.702; 0 1 2 3 2 2 x3 = 1; x = 1.833; x3 = 3.361; x = 6.162; 0 1 2 3 3 3 Possibilities of distributing K = 3 jobs among N = 3 stations 3 + 3 − 1 5 ns = = = 10 3 −1 2 k1 | 3 2 1 1 2 0 0 0 0 1 The states are k2 | 0 1 2 1 0 3 2 1 0 0 k3 | 0 0 0 1 1 0 1 2 3 2 Source: Prof. C. Görg, Communication Networks II, University of Bremen [email protected] 11 Example (cont.) All states sum up to G(3) 𝐺 3 = 𝑥1 3 𝑥2 0 𝑥3 0 + 𝑥1 2 𝑥21 𝑥3 0 + 𝑥1 2 𝑥2 0 𝑥31 + 𝑥11 𝑥2 2 𝑥3 0 + 𝑥11 𝑥21 𝑥31 + 𝑥11 𝑥2 0 𝑥3 2 + 𝑥1 0 𝑥2 3 𝑥3 0 + 𝑥1 0 𝑥2 2 𝑥31 + 𝑥1 0 𝑥21 𝑥3 2 + 𝑥1 0 𝑥2 0 𝑥3 3 = 24.733 Hence determination of state probabilities possible 3 1 𝑝 𝑘1 , 𝑘2 , 𝑘3 = ෑ 𝑥𝑖 𝑘𝑖 24.733 𝑖=1 Boundary probability pi(ki) ❑ E.g. station 1 with 2 jobs 𝑥1 2 𝑥21 𝑥3 0 + 𝑥1 2 𝑥2 0 𝑥31 𝑝1 2 = 𝑝 2,1,0 + 𝑝 2,0,1 = = 0.172 𝐺 3 Source: Prof. C. Görg, Communication Networks II, University of Bremen [email protected] 12 Example (cont.) Mean jobs in station i 3 Li = ki pi (ki ) ki =1 ❑ E.g. station 1 L1 = 1. p(1,1,1) + p(1,2,0) + p(1,0,2) + 2. p(2,1,0) + p(2,0,1) + 3. p(3,0,0) = 0.8728 L2 = 0.541 L3 = 1.585 Source: Prof. C. Görg, Communication Networks II, University of Bremen [email protected] 13 Example (cont.) Station load (probability of at least one job in station) 3 1 = p(i, j , k ) with k , j (0,1,2) i =1 3 2 = p(i, j , k ) with i, k (0,1,2) j =1 3 3 = p(i, j , k ) with i, j (0,1,2) k =1 In general, when ni = 1 i i = 1 − pi (0) = i Source: Prof. C. Görg, Communication Networks II, University of Bremen [email protected] 14 Example (cont.) For station 1 1 = p(1,1,1) + p(1,2,0) + p(1,0,2) + p(2,1,0) + p(2,0,1) + p(3,0,0) = 1 − pi (0) = 0.5428 2 = 0.386, 3 = 0.797 Station arrival rate (throughput) ❑ λi = ni ρi εi ❑ However ni = 1, so εi = μi 1 = 0.435, 2 = 0.232, 3 = 0.319 Mean sojourn time using Little’s law, Vi = Li / λi V1 = 2.009, V2 = 2.337, V3 = 4.976 Source: Prof. C. Görg, Communication Networks II, University of Bremen [email protected] 15