Econ 440 Lecture 2 PDF
Document Details
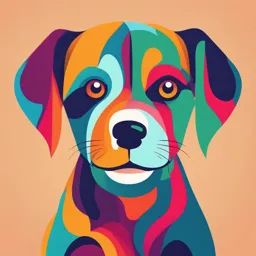
Uploaded by EnrapturedDragon
Texas A&M University
2024
Ragan Petrie
Tags
Summary
This document is a lecture covering econ concepts including quizzes, review materials, different types of experimental designs, and the study of probability. The notes are from August 22, 2024, and are from a Texas A&M University class.
Full Transcript
Econ 440 Lecture 2 Prof. Ragan Petrie Texas A&M University August 22, 2024 Quiz 0 - answer key on Canvas (Module 1) (1) A consumer has utility over consumption today (c1 ) and consumption tomorrow (c2 ) equal to 12 ln(c1 ) + 12 ln(c2 ) 1. If c1 = 10 and c2 = 20, what is the marginal r...
Econ 440 Lecture 2 Prof. Ragan Petrie Texas A&M University August 22, 2024 Quiz 0 - answer key on Canvas (Module 1) (1) A consumer has utility over consumption today (c1 ) and consumption tomorrow (c2 ) equal to 12 ln(c1 ) + 12 ln(c2 ) 1. If c1 = 10 and c2 = 20, what is the marginal rate of substitution between consumption today and consumption tomorrow? 2. What is the economic interpretation of this number? (2) Let y = u(x) = −3(x − 2)2 for x > 0 ∂u 1. What is ∂x =? 2. What is the value of x that maximizes u(x)? (3) Suppose you have 100 observations of GPAs from male and female college freshman, i.e. x1 , x2 , x3 ,..., x100. The average GPA across all freshman is x̄, and the standard deviation is d. 1. You want to run a t-test to test whether the average is different from zero. What would be the t-statistic? 2. You want to run a regression to test whether the average GPA for men and women are different. What would be the regression specification that you would run and how would you assess whether GPA’s are different? Be specific. Quiz 0 - Review Resources ▶ Economics Department Video Resources ▶ Basic Explanation of Functions ▶ Slopes and Inverse Demand ▶ Cobb-Douglas and Leontieff Production Functions ▶ Systems of equations and solving for equilibrium ▶ Consumer and Producer Surplus and Deadweight Loss ▶ Percent changes and elasticity ▶ Derivatives ▶ Optimization ▶ TAMU Virtual Math Learning Center Experimental Design Why Do We Need Experiments? ▶ Suppose we observe the following pattern in observational data: ▶ Can we conclude that cigarette lighters cause cancer? ▶ No, correlation is not causation ▶ More likely that there is a third variable (smoking) that causes the other two Experiments Give Us Control ▶ Experiments allow the researcher to control all the variables (or at least control them more than in observational data) ▶ Experiments also allow the researcher to determine causality using random assignment ▶ For example, if we randomly gave some people more cigarette lighters to have around the house, we would probably see that they have no causal relationship with cancer risk Building Blocks ▶ Every experiment needs some participants, also know as subjects or decision-makers ▶ The most basic unit in an experiment is a task or choice ▶ For example, deciding whether or not to buy a food item ▶ A treatment is a series of one or more similar tasks ▶ For example, choosing whether or not to buy many food items or buy one at a time ▶ An experiment consists of one or more treatments ▶ For example, could have one treatment where all food items are 25 cents, and another where all food items are 75 cents Treatment Variables ▶ Many experiments consist of only one treatment ▶ But many others have more than one treatment ▶ The parts that differ between the treatments are called treatment variables ▶ A common experimental design is to have two treatment variables that can each take on two levels ▶ This is called a 2-by-2 design ▶ Example: ▶ We are interested in responses to requests for donations to the local animal shelter ▶ Vary whether picture of a dog is included, and vary whether a specific amount of money is asked for Amount Specified Amount Not Specified Photo Treatment A Treatment B No Photo Treatment C Treatment D Four treatments Amount Specified Amount Not Specified Photo Would you give $10 to Would you give to help abandoned dogs help abandoned dogs find a home? find a home? Would you give $10 to Would you give to No Photo help abandoned dogs help abandoned dogs find a home? find a home? Within- vs Between-Subjects Design ▶ In a between-subjects design, each subject completes only one treatment ▶ In a within-subjects design, each subject completes multiple treatments ▶ What are the advantages/disadvantages of each designs? ▶ A within-subjects design can suffer from order effects: the order in which the subjects do the treatments can matter ▶ However, a within-subjects design needs fewer subjects and gives more control of subject characteristics Incentives ▶ Choices in experiments are typically incentivized with some kind of material incentive or payoff ▶ Incentives may be cash, consumption goods, social image ▶ Important to calibrate the size of the stakes to the task ▶ E.g., bad idea to only pay a few cents for correctly solving an entire crossword puzzle ▶ In some cases, hypothetical stakes may be appropriate ▶ e.g. “Would you take a job that could double your future income with probability 1/2 or reduce your future income by 1/2?” ▶ These type of questions are asked in the Panel Study of Income Dynamics (PSID) ▶ Notice there are no treatments. ▶ Could add treatments by adding info about state of economy prior to answering (i.e. economy forecasted to grow 2% annually next 5 years) Context ▶ Generally want to avoid contexts that include unnecessary complications or distractions for subjects ▶ For example, consider food choice experiment ▶ Primarily interested in testing the law of demand ▶ Avoid confounds such as making decisions publicly observable ▶ Unless, of course, this is the treatment variable in which I’m interested ▶ Controlling context is easier in lab experiments than field experiments ▶ Related issue: experimenter demand effect ▶ Subjects may be influenced by what they think the experimenter wants them to do ▶ Avoid using language that implies a value judgement or normative choice ▶ Deception used in psychology experiments so subjects do not know what the treatment is... but then you do not know what you are testing. Independence fictitious ▶ A famous (likely apocryphal) story: ▶ Graduate student is studying how fast lacerations heal on the skin of mice, depending on whether or not mice have a certain genetic mutation ▶ Advisor tells graduate student he should double his sample size for increased statistical power ▶ What is statistical power? ▶ More power reduces the probability of committing a Type II error (failing to detect an effect that is present, “false negative”) ▶ Doubling the number of mice is very expensive and time-consuming ▶ Graduate student is very clever: makes a second laceration on each mouse Independence ▶ Has the number of observations doubled? and why or why not? ▶ Not really: the two cuts on each mouse are probably not independent from another ▶ For example, if one cut heals quickly, I can expect the other cut will heal quickly too ▶ Thus the second cut does not add new information Pitfalls of Experiment Design ▶ Changing two treatment variables at the same time, i.e. always implementing a choice task when everyone is hungry or sleepy ▶ Poor choice of context, i.e. put the “desired” choice on upper-left side of screen ▶ Order effects not considered ▶ Observations not fully independent ▶ Poor choice of incentives and stakes Probability Why Do We Need Probability? 1. Social scientists are interested in making predictions about future behavior ▶ Sometimes the best prediction we can give is a likelihood of a certain event of interest happening 2. We need a benchmark to talk about rationality of behavior ▶ Probability judgement: Process of assigning a number to an event that represents one’s strength of belief that that event will occur ▶ The rules of probability come from very general assumptions but still give powerful restrictions on how probability judgement should behave Probability Basics ▶ Let Ω be the space of all possible outcomes ▶ E.g. if we are rolling a single die, Ω = {1, 2, 3, 4, 5, 6} ▶ A collection of one or more outcomes is an event, e.g. E ∈ Ω ▶ The event “roll a 3 or greater” is E = {3, 4, 5, 6} ▶ A probability function is a function P that assigns numbers between 0 and 1 (inclusive) to every possible event in Ω ▶ P({1}) = 61 ▶ P({3, 4, 5, 6}) = 2 3 Axioms of Probability ▶ An axiom is a mathematical rule that is assumed to be true ▶ We have just two axioms in probability theory: 1. P(Ω) = 1 ▶ You can think of this as “something is guaranteed to happen” ▶ E.g. P({1, 2, 3, 4, 5, 6}) = 1 in dice example 2. P(A or B) = P(A) + P(B) for any two mutually exclusive events A and B ▶ Called the addition axiom ▶ E.g. P({1}) + P({3, 4, 5, 6}) = 1 2 5 6 + 3 = 6 Where Do These Probabilities Come From? ▶ Frequentist perspective ▶ Probabilities represent long run averages ▶ E.g., P(4) = 16 because if I roll a die a very large number of times, 1 6 of the time I will roll a 4 ▶ Counting perspective ▶ Break event space into small enough pieces so that each one is equally likely to happen ▶ Probabilities of more complex events can then be built by addition rule, since pieces are mutually exclusive ▶ Example: what is the probability of flipping two heads in a row? ▶ Break down into 4 equally likely events: HH, HT , TH, TT ▶ Only 1 of these has two heads, so P(HH) = 1 4 Conditional Probabilities P(A and B) ▶ We can define conditional probability P(A|B) = P(B) ▶ This is the probability of A occurring given that we know B has occurred. ▶ Example: P(winter) = 14 but P(winter | snowed last weekend) > 1 4 ▶ We say two events A and B are independent if P(A|B) = P(A) ▶ Dice example: Probability of rolling a six is independent of what you rolled previously ▶ Multiplication rule: For any two events A and B then P(A and B) = P(A|B)P(B) ▶ Comes from rearranging definition of conditional probability ▶ Note that if A and B are independent, P(A and B) = P(A)P(B) Bayes’ Rule: Intuition ▶ Suppose you have the following information: ▶ The baseline breast cancer rate in women is 10% ▶ If a patient has breast cancer, a mammogram will return positive with 90% probability ▶ If a patient does not have breast cancer, a mammogram will return positive with 20% probability ▶ Question: What is the probability that a patient has cancer given that you see a positive mammogram result? ▶ Consider 100 women, all of whom we test for breast cancer ▶ 10 will have cancer (from the baseline rate) ▶ Of these, 9 will return positive, 1 will return negative ▶ 90 will not have cancer ▶ Of these, 18 will return positive (90 × 0.2) and 72 will return negative. ▶ So among the positive tests, only 9 1 27 = 3 are true positives for cancer Bayes’ Rule: Graphically Bayes’ Rule: Formalization ▶ Suppose we are considering two events A1 (having cancer) and B (having a positive test) ▶ From the definition of conditional probability, we have ▶ P(A1 |B) = P(A1 and B) P(B) ▶ P(B|A1 ) = P(B and A1 ) P(A1 and B) P(A1 ) = P(A1 ) ▶ Substituting one into the other: P(B|A1 )P(A1 ) P(A1 |B) = P(B) ▶ Suppose A2 is the event that A1 does not happen, ie A1 + A2 = Ω - A1 and A2 are mutually exclusive and comprise all possible outcomes ▶ Then P(B) = P(B and A1 ) + P(B and A2 ) - law of total probability ▶ This give us final version of Bayes’ rule: P(B|A1 )P(A1 ) P(A1 |B) = P(B|A1 )P(A1 ) + P(B|A2 )P(A2 )