IS423 Course Notes - Session 4 PDF
Document Details
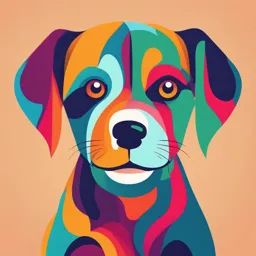
Uploaded by HealthfulTropicalIsland7725
Singapore Institute of Management (SIM)
2023
Randall E. Duran
Tags
Related
- FSI 3023 - Fintech and Financial Services PDF
- Lesson 9: Payment Services PDF
- DFS and RTGS - Digital Financial Services & Real-Time Gross Settlement PDF
- Financial Aspects of Digital Business PDF
- Trainee Manual - Digital Technology in Banking May 2024 PDF
- Personal Finance Chapter 4: The Banking Services of Financial Institutions PDF
Summary
These course notes discuss the evolution of business models and technology platforms for brokers over the past few decades. The shift towards electronic trading and algorithmic trading is highlighted, along with the increasing importance of real-time market data. The notes also cover various business activities and services offered by financial intermediaries.
Full Transcript
Adapted from: © 2023 Randall E. Duran Financial Services Technology, by Randall E. Duran, Cengage Learning Asia, 2017 IS423 Course Notes Session 4 Introd...
Adapted from: © 2023 Randall E. Duran Financial Services Technology, by Randall E. Duran, Cengage Learning Asia, 2017 IS423 Course Notes Session 4 Introduction Like exchanges, the business models and technology platforms of brokers have undergone major changes over the past few decades. Transactions have gradually migrated from voice-based execution to electronic trading, and at the exchange, from floor trading to automated matching. Trading volumes have multiplied and low latency trade execution services have become competitive offerings. One of the main drivers behind these changes has been the shift in asset managers’ responsibilities and expectations. Over time, the performance of asset managers has come under greater scrutiny. Since transaction costs can be a major drag on overall investment performance, brokers’ fees and their quality of trade execution became a major concern. Likewise, the widespread availability of real-time market data has provided “buy-side” traders with better visibility into market activity. At the same time, the orientation of sell-side firms has also changed. Automated trading platforms enable a single customer to send out thousands of orders per second to their broker. A new breed of buy-side firms emerged that were focused on algorithmic and high-frequency trading. For these customers, it was important to minimize the delay, or latency, between when the broker received the order and when it was delivered to the exchange. Moreover, these customers were attractive in that they tend to trade in large volumes and, thus, generated large amounts of commission fees. However, to acquire and retain them, brokers’ technology infrastructure had to be retrofitted and continually enhanced to keep up with ongoing changes in hardware, software, and trading markets. Besides updating their IT systems, brokers began offering additional services to help attract new, and retain existing, buy-side customers. Some brokers extended the availability of their in- house trading and portfolio management systems to their customers. Prime brokerage services, including centralized securities lending, financing, and global custody services, were another offering provided to customers. Brokers also ramped up their own proprietary trading (prop trading) operations, that used the broker’s own capital to trade in the market. Unlike the business of handling customer orders, prop trading was the equivalent of having a private hedge fund embedded within the broker. These changes occurred over an extended period and some financial intermediaries responded faster than others. This uneven growth also led to many technology-related challenges. The migration from phone calls, faxes, and paper confirmations to entirely electronic system-to-system communications required participants to simultaneously support all communication methods. Likewise, from the outset, there was no standard way for the sharing of electronic trade information between buy-side firms, sell- side firms, exchanges, clearing houses, and depositories. Business Activities A financial markets intermediary may be involved in a single business activity or many different activities. For instance, a financial institution might only provide equity brokerage services as a member of a single exchange. On the other hand, another financial institution might provide equity brokerage services in multiple geographies, support trading of listed derivatives, provide investment research, make markets in FX, as well as provide investment banking services. Figure 1 shows proportion of revenue generated by business unit for the ten largest investment banks. The following subsections will examine the common services that are engaged in by financial intermediaries. To help narrow the scope of discussion, this section will focus primarily on the activities of agency brokers and broker- dealers in the context of equity trading. Specifically, this section covers customer-focused services, prop trading, market making, and risk management. 1 Adapted from: © 2023 Randall E. Duran Financial Services Technology, by Randall E. Duran, Cengage Learning Asia, 2017 Figure 1. Investment banking revenue by business unit The types of customer services provided by a financial intermediary vary depending on the institution. Even so, there are brokerage-related services, such as order management, research, and advice, that are prevalent across many institutions. Order management and execution Due to capital and regulatory requirements, it is impractical for most buy-side firms to be exchange members. Hence, order management and execution are primary services that agency brokers provide to customers. Agency brokers act as agents for their clients, performing transactions on customers’ behalf and without serving as a counterparty in the transaction. Many agency brokers are exchange members. Alternatively, an agency broker who is not a member may execute customer orders via another broker who is a member of the exchange. Buy-side customers typically use nonmember brokers to help disguise their trading activity. For example, an asset manager may be concerned about leakage of information that a large order is pending in the market, if it is placed with a single broker. Therefore, it might choose to split the order and send the different parts to multiple brokers to manage the execution. Distributing the order helps obfuscate the source of the trading, and no single intermediary will know the scale of the overall transaction. Likewise, the “footprints” in the market left by the order’s execution will appear to be from multiple unrelated sources. Common services related to order management that agency brokers provide include: order execution—routing orders to execution venues; position tracking and reporting; algorithmic order execution; and smart order routing. With regard to position tracking and reporting, a “position” is the value, that is, the product of the quantity and price, of a cash or security holding. A small quantity of a high-priced asset can represent a large position, as can a large quantity of a low-priced asset. Research Many financial intermediaries have internal research teams, including securities analysts and economists, who provide analysis and opinions on a range of investment topics. Valuation assessments of companies, 2 Adapted from: © 2023 Randall E. Duran Financial Services Technology, by Randall E. Duran, Cengage Learning Asia, 2017 expectations for currency and interest rate movements, and macroeconomic evaluations of countries, regions, and markets are some of the areas that may be covered. Research is used to guide trading and asset allocation for financial intermediaries’ investments and trading. Financial intermediaries may also distribute research reports to customers as a value-added service. Typically, research will be provided to customers without charge as an incentive for customers to send some or all of their order flow to the broker. Advice Besides research, some financial intermediaries provide advisory services. Advice may be targeted at broad or detailed levels. For example, a financial intermediary may make high-level recommendations about allocation percentages across a range of asset classes such as domestic equities, international equities, government bonds, and cash. Alternatively, they may make a specific recommendation about the valuation of a company’s stock and issue a rating for it: buy, sell, or hold. Banks and brokers also provide advice that takes into account customers’ existing holdings and specific needs. In particular, customers may benefit from guidance on which financial instruments can help them achieve specific investment or risk hedging objectives. Financial intermediaries have begun, more recently, to recommend the use of specific algorithms to customers that execute large orders. Such advice may be based on in-depth knowledge of the algorithms’ design and behavior in various market conditions. Likewise, customers may need direction on how to set an algorithm’s parameters so as to achieve optimal results. Despite the fact that advisory services are intended to help customers, they have come under scrutiny. One concern has been that financial institutions’ investment banking relationships with companies have led to the provision of biased investment ratings on those companies. Another concern is that the advice provided to customers has been motivated and influenced, to some extent, by brokers’ goal of getting customers to buy products and services rather than addressing customers’ needs. Consequently, there have been numerous lawsuits around the world that have accused financial intermediaries of mis-selling products to customers. Financial markets intermediaries have tried to address concerns regarding their advisory services in several ways. Lengthy legal disclaimers have been added to banking agreements and research reports to help protect against lawsuits. Banks and brokers have also provided more training on regulatory requirements and client obligations to customer-facing staff, that is, front-office employees who interact directly with customers. Furthermore, some financial institutions have implemented stricter internal controls for business activities that provide financial advice or product recommendations to customers. In some cases, business process management systems (BPMS) have been used to help ensure that relationship managers (RMs) and brokers actually execute the processes as intended. Other Services Besides the core services described above, brokers offer a variety of services to address specific customer needs. Prime brokerage services are service packages designed to support smaller fund managers, especially hedge funds. Common services provided as part of and in conjunction with prime brokerage arrangements include: securities lending; centralized clearing; global custody; provision of trading and order management systems (OMS); operational support and consulting services; and leasing of office space. 3 Adapted from: © 2023 Randall E. Duran Financial Services Technology, by Randall E. Duran, Cengage Learning Asia, 2017 Many of these services may not have visible fees associated with them. Instead, the bank or broker will generate revenue indirectly from interest charges on customers’ margin account and fees related to the customers’ trading activity. However, other services, such as leasing and consultancy, may be billed on a monthly basis. Prime brokerage arrangements are attractive to customers, particularly new and smaller funds, because it provides a “one-stop shop” that enables them to get going quickly, with a minimal amount of overhead. Likewise, the per-transaction cost model minimizes the need for hedge funds to obtain upfront capital to set up infrastructure for their business operation. Trading System Architecture Over time, the development of equity order routing systems have followed different paths. Initially, high- touch order management systems were built to support manual order execution that requires people to be involved. For example, a fund manager might call his broker to ask the broker to manually “work” an order on the market to get a good execution price or to execute it using one of the broker’s algorithms. More recently, though, low-touch order routing platforms have become popular. In the low- touch case, a buy- side trader or an IT system that implements an algorithmic trading system would send orders straight into a direct market access (DMA) trading platform. The execution of these orders would then be managed electronically, and without human intervention. This section examines the functional components commonly used by banks and brokers to route and manage customer orders for exchange traded instruments. Two common service delivery configurations, DMA and colocation, are also reviewed. Order Routing System Components Order routing systems support the delivery and processing of orders as they flow from the end user to an execution venue. As shown in Figure 2, a number of functional components may be involved. OMS are one of the most common systems used by both brokers and their customers. OMS were originally designed to perform electronic recordkeeping for orders placed over the telephone. Order- capture and position screens replaced paper order tickets and position blotters in the front office. By managing trade information in a database, OMS were also able to support back-office functions such as accounting, reporting, and reconciliation. Execution management systems (EMS), on the other hand, were designed for electronic trading, where trading volumes are much higher and execution speed is critical. EMS manage the execution of orders electronically and with no manual intervention, sometimes by using algorithms to execute orders over an extended period of time. There is some debate over whether, ultimately, the functionality of OMS and EMS will merge, and that it will not be necessary to choose between the two types of systems. However, it may be the case that certain specialized capabilities cannot be provided by a “one size fits all” implementation, and that demand for such features will support the continued existence of both types of systems. Connectivity gateways provide interfaces, often based on standard protocol such as FIX, that enable customers to send orders electronically to the broker. The risk management system verifies that the customer orders will not exceed their margin or cash limits. Smart order routers determine to which execution venue(s) orders should be routed. Market access gateways, also referred to as line handlers, connect and communicate orders to different execution venues. Each connection, or “line,” to the exchange will have a limited capacity that is commonly referred to as its “throttle” limit. For example, a single connection to the exchange may support up to 100 messages per second, which includes messages related to orders, acknowledgements, and fills. Hence, to handle a peak processing rate of 250 orders per second, a broker would require multiple exchange connections. Alternatively, if only one line were used, orders and responses would queue up at peak times and be subject to processing delays, that is, latency. Even if throughput was not a concern, typically, at least two exchange connections would be maintained to provide resilience— if one telecommunication link to the exchange were to fail, the other would continue to provide connectivity, preventing service interruption. 4 Adapted from: © 2023 Randall E. Duran Financial Services Technology, by Randall E. Duran, Cengage Learning Asia, 2017 The connectivity between components may be achieved in a number of different ways. Components can communicate with one another directly over the network using relatively low-level TCP/IP communication protocols. Alternatively, in higher-performance environments, specialized middleware messaging products may be used to implement scalable, low-latency network communications. In ultra-high performance configurations, multiple components may be run within the same server and communicate with one another using interprocess communication (IPC) mechanisms, such as shared memory communication. Using IPC avoids the delay involved with sending messages over the network, often reducing latency by an order of magnitude. In all the figures shown, the cloud images that show “leased lines” refer to dedicated telecommunications links, which may be implemented using any number of technologies, such as multiprotocol label switching (MPLS) networks. Likewise, multiple stacked boxes indicate where multiple instances are run for load balancing purposes, as is the case with the market access gateways shown in Figure 2. It should be assumed that some form of active-active, hot-standby resilience, is used for all of the components, even though a single box is shown in the diagrams. Figure 2. Order routing architecture implemented by brokers Direct Market Access DMA is a service whereby customer orders are sent electronically to the broker, who then routes the order to the relevant exchange with minimal interruption. In Figure 2, DMA orders begin their journey in the lower left-hand corner, flow through a connectivity gateway, and are then routed by an EMS to execution venues. To minimize delay, DMA orders circumvent the broker’s OMS on their way to the execution venue. Also, DMA orders are not visible to the broker’s front office prior to execution because they do not have access to monitor order flow through the EMS. After execution, the trade details will be reported by the EMS to the OMS. Allowing the financial intermediary’s staff to only access post-trade 5 Adapted from: © 2023 Randall E. Duran Financial Services Technology, by Randall E. Duran, Cengage Learning Asia, 2017 information diminishes the chance of information leakage, that is, other market participants gaining knowledge of the order and making trades in advance of or in parallel with the customer. Brokers compete on the speed at which their DMA platforms can deliver orders to execution venues, and every microsecond counts. As a case in point, a broker has claimed as part of its public marketing one- way latencies of between 200 and 600 microseconds to various Asian stock exchanges. Specialized order routing components designed and tuned to deliver high performance are used to implement DMA. To avoid delays, these components only perform basic risk validation and compliance. For example, whereas an OMS would retrieve current margin or credit availability information from the risk management system as part of the pretrade validation process, calls to an external system would introduce a very long delay for DMA. Alternatively, the components used for DMA could retrieve customer risk information at the beginning of the day and only perform pretrade risk checks that approximate the customer’s current risk position based on their intraday transactions. While the latter method is less accurate, it significantly reduces the interaction between systems and, thus, the processing time. To help compensate for the reduced accuracy, risk-limit thresholds could also be lowered. Algorithmic Order Execution Institutional investors, such as pension, mutual, and hedge funds, must achieve sizable positions in individual equities, which makes it difficult for them to achieve preferable execution prices. If an institutional investor submits a large market order, just a small portion of it may take up all available liquidity at or near the current market price. The part of the order that cannot be filled by the available liquidity may be executed at suboptimal prices. Alternatively, placing a large limit order, instead of a market order, could signal the intention to buy or sell a large quantity of the security, thereby leading other market participants to react quickly and drive the market price in an unfavorable direction. Therefore, in practice, large orders are sliced into smaller orders and executed over a period of time. Historically, people at brokerages did this manually. In recent years, it has become more common to use computerized algorithms to perform the slicing and order execution function. This section introduces the general concepts related to algorithmic order execution. Two basic algorithms, time-weighted average pricing (TWAP) and volume-weighted average pricing (VWAP), will be used to illustrate the concepts and principles involved with algorithm trading. Liquidity seeking algorithms are also discussed. For brevity, in the discussion, the terms algorithmic order execution and algorithmic trading will be used interchangeably. Concepts Algorithmic order execution describes the use of formal and repeatable methods to execute orders in the market. Algorithms address tactical concerns, such as when and how orders should be placed in the market to execute a large order. They do not make strategic decisions, such as what to trade or why positions should be taken, which is handled by trading strategies. Algorithmic trading does not require computers. A person can perform algorithmic trading simply by following a set of rules, manually performing calculations, and typing trades into an OMS at the specified times for the calculated amounts. However, computers are commonly used for this purpose because they are more efficient, cost effective, and reliable for performing these operations. Typically, the EMS is the architecture component that is responsible for algorithmic order execution. Algorithmic order execution is used in conjunction with large orders for two purposes: to reduce the orders’ market impact and to minimize trading costs. In some cases, trading algorithms are designed to achieve or exceed a specific benchmark. Algorithms are characterized as being either schedule driven, designed to achieve volume participation, or opportunistic, or a combination of the two. Schedule- driven algorithms and volume participation algorithms are often designed to track a specific trade execution benchmark, such as TWAP and VWAP, respectively. On the other hand, opportunistic algorithms are liquidity seeking, that is, they aim to execute as much of the order as possible at times when there is ample liquidity available at or near the current market price. Algorithms’ execution strategy may also be 6 Adapted from: © 2023 Randall E. Duran Financial Services Technology, by Randall E. Duran, Cengage Learning Asia, 2017 categorized as passive, active, or aggressive, or some combination of the three. For example, an opportunistic algorithm could first try to aggressively fill a certain percentage of the order soon after the execution of algorithm was initiated. Next, it could place passive limit orders in the market. Then, if fills against the limit orders do not generate sufficient volume, the algorithm could periodically become active and execute market orders to utilize existing liquidity. Parameters are used to modify the behavior of algorithms. Start and end times, and price and volume limits are common parameters that are used in conjunction with algorithms. These parameters may be changed while the algorithm is executing the order based on market conditions. Likewise, the details of manual trades that have been executed simultaneously and separately from the algorithm to fill the order may be input to the algorithm so that it can adjust the amount of the order that must be filled and also calculate the average execution price based on both the automated and manually executed trades. Algorithms have been developed to achieve specific execution objectives and accommodate different market conditions. For example, some institutional investors are benchmarked in a way that requires them to try to match the price of their trades as closely as possible to the end-of-day closing price. Achieving this goal is difficult because trading volume is especially heavy at the end of the trading day and large volumes of market data must be processed in real time to determine the current price. Hence, specialized algorithms have been designed to execute trades in the last few minutes leading up to market close. These algorithms attempt to project what the closing price will be and then execute a series of trades during the final minutes that will be near the closing price. For large orders, it is not practical to execute them entirely in the final seconds of trading because there may not be sufficient liquidity to accommodate the order, causing the execution price to slide away from the market price. Buy-side execution management systems, the brokers’ EMS, and execution venues may provide algorithmic trading capabilities. Nonetheless, many fund managers use broker’s algorithmic trading systems, and brokers compete with one another using proprietary algorithms so as to attract customer order flow. Some brokers also customize their algorithms to address specific customer requirements or adapt them based on customer feedback. Time-weighted Average Pricing Algorithms TWAP algorithms are schedule-driven algorithms that implement a simple strategy to slice up an order over a fixed period of time. TWAP divides up the order evenly over a specified number of time periods. For example, a parent order to buy 150,000 shares of a given stock might be split evenly into twelve child orders of 12,500 shares each, which are then executed at half-hour intervals. The average price for the order’s execution should approximately be the average price of the stock at each half-hour interval. Figure 3 illustrates how a TWAP algorithm would distribute orders over the course of the trading day. The light grey bars show the aggregate volume over half-hour intervals for a hypothetical stock that trades 500,000 shares in a day, and the dark grey bars show how the TWAP child orders would compare to the total volume traded during that period. TWAP is easy to understand and implement, but it has its shortcomings. First, it does not take into account the pattern that trading volume follows throughout the day. Typically, the heaviest volume will be at market open and market close. As shown in Figure 3, this pattern causes TWAP trades to make up a small percentage of volume around the start of the day, and a larger percentage around the middle of the day when aggregate trading volumes are lower. Thus, orders placed in the middle of the day would be expected to have greater market impact than those at the beginning or end of the day. A second concern is that the predictability of the TWAP algorithms, for example, buy 12,500 shares every 30 minutes, leaves “footprints” in the time and sales data that may be detected by other market participants. With knowledge of the amount and timing of future TWAP orders, other traders could “game” the algorithm. They could buy just before each incremental TWAP buy order, wait for the TWAP order’s size to impact the price, and then sell after the order raised the price. Therefore, in practice, TWAP algorithms have been adapted to include variation and randomization to help avoid this problem. 7 Adapted from: © 2023 Randall E. Duran Financial Services Technology, by Randall E. Duran, Cengage Learning Asia, 2017 Figure 3. Proportion of volume traded using a TWAP algorithm Volume-weighted Average Pricing Algorithms In contrast to TWAP algorithms, VWAP algorithms are a type of volume participation algorithm that incorporates trading volumes into the order size calculations. VWAP order sizes are proportional to the expected trading volume during the given time period. Larger orders will be placed during time periods that have higher volumes traded. Figure 4 shows how a VWAP algorithm would distribute orders to buy a total of 150,000 shares over the course of the trading day. Since 150,000 shares would be 30% of the stock’s hypothetical daily volume (500,000 shares), a VWAP algorithm would target to buy approximately 30% of the traded volume during each thirty-minute period. VWAP addresses the shortcomings of TWAP by placing orders for variable percentages of the intraday trading volume rather than fixed amounts. Even so, VWAP has a problem of its own— determining what the trading volume will be over the course of the day. The total volume traded will vary every day and the actual trading volume for each time period during the day may only be known after the fact. VWAP algorithms deal with this problem by using historical data to estimate the expected daily volume and its temporal distribution. If the trading volume has consistently followed a similar pattern over the previous month, the assumption is made that the pattern will hold for the current day. Consider the case where a stock’s average trading volume between 9:30 am and 10:00 am was 12% of its total daily volume over the past 20 trading days. Based on this information, a VWAP algorithm would execute 12% of the total order during the same time period. VWAP algorithms are well suited when performance is measured using a VWAP benchmark. However, because price is not factored into either TWAP or VWAP algorithms, they may continue to trade into sharp spikes or drops in the market, leading to a poor execution price and further exacerbate price movements. Incorporating parameters into the algorithm that set trading price limits can help address this concern. VWAP algorithms are also problematic if the market moves in the wrong direction for the trade, that is, when the market drops steadily throughout the day as a large sell order is being executed. The portions of the order that are reserved for execution in the middle and at the end of the day will execute at a substantially worse price than those orders at the beginning of the day. In this situation, it is better to execute most, if not all, of the order early in the day, even if it temporarily perturbs the market. Hence, VWAP is best applied in flat market conditions as opposed to when there is directional trending. VWAP algorithms have been popular despite their drawbacks. VWAP is also a common benchmark used to gauge traders’ performance and, thus, using a VWAP algorithm helps ensure that an order’s execution price is in line with the benchmark. Likewise, VWAP algorithms help ensure that the size of trades in any given time period will not stand out. 8 Adapted from: © 2023 Randall E. Duran Financial Services Technology, by Randall E. Duran, Cengage Learning Asia, 2017 Figure 4. Proportion of volume traded using a VWAP algorithm Liquidity-seeking Algorithms TWAP and VWAP algorithms are two relatively simple methods used to execute orders. Other more complex trading algorithms are more dynamic and take available liquidity into consideration. Rather than mechanically slicing up and executing orders, liquidity-seeking algorithms use depth-of-market information to decide the timing and size of orders. Such algorithms often place market orders to try to immediately fill as much of the order as possible without substantially moving the price. The algorithm may then attempt to fill the remainder of the order “passively” using limit orders. Alternatively, new orders may be placed when additional liquidity becomes available within a target price range. To illustrate how liquidity-seeking algorithms work, consider a simple example of an order to buy 100,000 shares of a stock. Based on the depth-of-market data, as shown in Figure 5, the algorithm would initially place a market order to buy 30,000 shares to try to purchase the available shares for sale at $14.60 and $14.65. Additional liquidity is available in the exchange’s order book; however, purchasing the remaining shares at $15.00 would adversely affect the average execution price for the order. Instead, the algorithm might place a limit order to buy 10,000 shares at $14.55. When the limit order was filled, the algorithm would place new child limit orders until the entire parent order was filled. The algorithm might also place market orders to buy when shares were on offer again in an acceptable price range, for example, for sale at or below $14.70. Figure 5. An example of available stock liquidity shown by depth-of-market data This example was simplified to help explain the general concept. In practice, such algorithms can be quite complex and may take into account liquidity that is potentially available but not displayed in the market depth information, that is, hidden and iceberg orders. Hybrid algorithms, which mix liquidity-seeking and benchmark-tracking strategies, are also available. Algorithms may also make use of liquidity that is 9 Adapted from: © 2023 Randall E. Duran Financial Services Technology, by Randall E. Duran, Cengage Learning Asia, 2017 available across multiple execution venues, taking advantage of a trading system’s smart order routing capabilities, if they are available. Smart Order Routing Smart order routing is applicable when an order can be executed on more than one execution venue. In North American and European markets, multiple exchanges and dark pools are available to trade highly liquid stocks. A simple order routing approach would consistently send orders to a single venue or require the person placing the order to designate the target venue. Alternatively, smart order routing evaluates a number of factors and then decides how much of an order to send to different execution venues so as to achieve the optimal execution price. The best available price at each venue is one factor used to route orders, but this consideration is complicated by price information that may not be publicly available, such as hidden orders on lit execution venues and orders sitting in dark pools. Available liquidity is another factor to be considered. If the venue that has the best price only has a small amount that is available for trading at that price, it may not be the best routing destination. The likelihood of execution on different venues will also be relevant. For instance, the greater the time lag between when the price information is received from an execution venue and the time the order is processed by the venue, the greater the chance that the price will have moved by the time the order is received. Based on these and other considerations, a smart order routing component could decide to route an order to one or multiple execution venues, either sequentially or in parallel. Algorithmic trading may also incorporate smart order routing to optimize performance to handle situations where the available liquidity is fragmented across multiple venues. For instance, a liquidity- seeking trading algorithm might initially fill part of an order by sending child market orders to a subset of lit execution venues, exchanges, and ECNs. The order quantity sent to each venue would depend on the availability of shares at that venue within a specific price range. Then, the remaining amount of the parent order could be divided and placed using passive limit orders on both lit and dark execution venues. Architecture Considerations This section examines two architecture considerations that are important for order routing systems: application-level communication standards and complex event processing (CEP). Application-level Communication Standards Standards are needed to support interparty communication of orders and execution status information. The FIX protocol is a real-time message communication standard that has become the predominant standard for communication between asset managers’, brokers’, and exchanges’ trading systems. FIX is also commonly used for communications between components within a trading system, such as between the connection gateways, OMS, EMS, and market access gateways. Besides FIX, other proprietary protocols exist. In some cases, components may support both FIX and a proprietary protocol. Whereas FIX provides simple “plug-and-play” connectivity, accommodating proprietary protocols will typically require additional integration effort, but may also provide better performance. As a case in point, the Singapore Exchange supports trading access both via a FIX gateway as well as using its own proprietary API, whereby the proprietary API provides lower latency. FIX supports a wide variety of transaction types, such as the generation of new orders, cancelation of orders, and communication of execution reports. FIX messages are sent between market participants’ order and execution management systems. SWIFT messages, on the other hand, are primarily used for back-office communications within banks. Like SWIFT, FIX is encoded using a text-based ASCII format. Even so, FIX is unlike SWIFT in that FIX does not provide a network for message communication. FIX is independent of any specific transport layer communications protocol. Message transfers are usually implemented on top of TCP/IP, but are also sent over other protocols, such as Java Messaging Standard implementations or tunneled through Secure Sockets Layer encrypted connections. 10 Adapted from: © 2023 Randall E. Duran Financial Services Technology, by Randall E. Duran, Cengage Learning Asia, 2017 The FIX protocol Like many other communication protocols, the FIX protocol is defined by its: Message structure—every FIX message has three parts: a header, a body, and a trailer. The FIX header includes the version of the FIX message, the length of the message body, and the message type. The content contained within the message body will vary depending on the message type. The trailer primarily consists of a checksum that is used to verify the integrity of the message. Data dictionary—consists of field and message definitions, and acceptable field values. For example, FIX version 4.0 defines message type “D” as an “Order—Single” message that contains eight mandatory fields and 29 optional fields. One of the mandatory fields in the message is field number 54, which is the “Side” field. FIX 4.0 defines the valid values for the Side field to be 1, 2, 3, 4, 5, and 6, which correspond to buy, sell, buy minus, sell plus, sell short, and sell short exempt, respectively. Encoding format and syntax—define how field and message values are represented as physical messages. Within the header, body, and trailer of a FIX message, field values are encoded using tag- value pairs, for example, “54 = 1” (tag = field value). The tags are integer values whose meaning the FIX data dictionary defines. Some tag values are reserved for private use between the sending and receiving parties and can be used to extend and customize the protocol. Field values are delimited using the nonprintable ASCII 01 control character. Interaction semantics—describe valid message communication sequences. FIX provides two levels of interaction semantics: one at the session level and another at the application level. Session-level semantics define the valid sequence of logon, logout, heartbeat, and other message exchanges that are not business related. FIX relies upon sequence numbers to ensure that messages are not lost or duplicated. Session-level semantics define how the sender and receiver should recover dropped messages, while application-level semantics define the valid responses for specific message types, such as orders and cancelation requests. Figure 6 shows an example of an order message encoded using FIX protocol version 4.2. This message represents a single order sent from a fund manager to a broker. The message shown represents an order to buy 50,000 shares of General Electric common stock at a limit price of $25.50 that is good until end of day. In this example, the ASCII 01 field delimiters are represented using the “^” symbol. The Side field of the trade, shown highlighted, corresponds to the 15th field in the message and it is set to the Buy value, which is represented by the number “1.” The other fields and their values can be determined by referencing a FIX dictionary. 8=FIX.4.2^9=202^35=D^49=BUYSIDEID^56=SELLSIDEID^34=132^52=2010 0905-14:34:25^11=999999^1=123456^63=0^64=20100908^21=3^110=500 0^111=10000^55=GE^48=369604103^22=1^54=1^60=20100905-13:34:51^ 38=50000^40=1^44=25.50^15=USD^59=0^10=127 Figure 6. Example of a FIX message FIX versions While FIX is a standard, several versions of the standard are actively used. Common versions of the FIX protocol that are used include FIX 4.0, 4.1, 4.2, 4.3, 4.4, and 5.0. The encoding format and data dictionary are consistent between the FIX versions. The available list of fields, message types, and valid values has been expanded with each new version of the protocol. For example, field 54—defined by FIX as the “side of an order,” such as buy, sell, short sell, and cross—has nine valid values in FIX version 4.2, whereas in FIX version 4.4 sixteen different values are valid. The interaction semantics have also changed between protocol versions. 11 Adapted from: © 2023 Randall E. Duran Financial Services Technology, by Randall E. Duran, Cengage Learning Asia, 2017 FIX protocol destinations, such as brokers and exchanges, will advertise which FIX version(s) they support. For example, Saxo Bank, an equities, futures, and FX broker, supported FIX versions 4.3 and 4.4 (as of 2017), and the London Stock Exchange supported FIX 5.0 (as of 2017). FIXML and FAST FIXML and FAST are two standards that are derivatives of the FIX protocol. FIXML is an XML-based standard version of FIX that is defined by document type definition (DTD) schemas. FIX was originally designed to be compact so as to minimize transmission and processing overhead, which is important for time-sensitive pretrade processing. After the FIX standard was developed, XML became a popular means of encoding data. Use of XML was advantageous because it was flexible and widely supported. The disadvantage of using XML, however, was that XML-based messages were long and required more processing time. Accordingly, FIXML was developed to support post-trade activities that were less time sensitive, such as clearing functions. Figure 7 shows an example of a FIXML message represented using the FIX 4.4 Schema Version. While the FIXML version is easy to read, it is larger than the FIX message with content similar to that shown in Figure 6. Figure 7. Example of a FIXML message FAST, on the other hand, was adapted to make the FIX protocol more efficient to transmit and process. FAST uses implicit tagging so that the tag values can be omitted from the message; instead, message templates are used. Also, certain fields can be left out of a message. The value of an omitted field can be inferred by the message receiver to be a default value, defined in the message template, or the value of the field that was sent in a previous message of the same type. FAST also encodes the message in binary format instead of text format. This results in both shorter messages and messages that are more easily processed electronically. FAST was designed to provide a FIX-based binary protocol for streaming market data. Traditionally, market data distributors and exchanges have had their own proprietary protocols for delivering market data, making it difficult for market participants to connect to multiple data sources. Extending the FIX protocol to support market data could provide a common standard, but using text-based FIX was too inefficient for high volume market data. Hence, FAST was developed to address these concerns. By encoding information in binary form, FAST reduces the transmission overhead and eliminates the processing time required to convert from human readable text format to computer readable binary format. FAST is used by a number of exchanges, ECNs, and financial intermediaries to provide direct market data and rate feeds. 12 Adapted from: © 2023 Randall E. Duran Financial Services Technology, by Randall E. Duran, Cengage Learning Asia, 2017 FIX Engines Like most systems used in financial markets, it is not necessary to build systems to send and receive FIX messages; there are a number of off-the-shelf systems that provide this capability. In fact, it is generally impractical to build FIX parsing and encoding functionality. This subsection has provided a simplified view of the FIX protocol for ease of understanding. In practice, however, much more complexity must be handled. For instance, the FIX message structure allows for nested groups of repeating field groups, and FIX has specific rules about how encryption should be applied to FIX messages. There are dozens of available FIX engines, both commercial and open source, available that have already addressed these concerns. FIX engines are software components that do the following: manage the network connectivity of the FIX session, encode and parse the FIX messages, perform validation of the FIX messages received, and automatically handle the session interaction semantics, that is, sequence number tracking, message loss recovery, and sending periodic “heartbeat” messages to show that the session is active. A FIX engine acts as a gateway for applications to communicate with other market participants outside the organization using standardized FIX formats. FIX engines may come as components that are already integrated into an OMS. Alternatively, standalone FIX engines may be used, and then must be integrated with other OMS and EMS components. Typically, FIX engines will support encryption, persistence, multiple messaging transports, monitoring tools, and simultaneous sessions that use multiple versions of FIX. Additionally, high-availability configurations may be available along with prebuilt interfaces to ECNs and other market participants. For some market participants, such as high-frequency traders, performance of the FIX engine may also be a key consideration. To this end, there are some FIX engines that have been implemented using customized hardware to maximize performance, as opposed to software running on commodity server hardware. 13